南京大学:《组合数学》课程教学资源(课堂讲义)Cayley公式 Cayley's formula
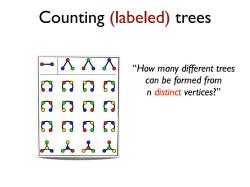
Counting (labeled)trees 八。入.八 "How many different trees can be formed from n distinct vertices?" 68 3 只又
Counting (labeled) trees “How many different trees can be formed from n distinct vertices?

Cayley's formula: There are n"-2 trees on n distinct vertices. 69 只又人又 Arthur Cayley
Cayley’s formula for the number of trees Chapter 30 Arthur Cayley One of the most beautiful formulas in enumerative combinatorics concerns the number of labeled trees. Consider the set N = {1, 2,...,n}. How many different trees can we form on this vertex set? Let us denote this number by Tn. Enumeration “by hand” yields T1 = 1, T2 = 1, T3 = 3, T4 = 16, with the trees shown in the following table: 434 34 34 343434 3 4 3434 34 34 343434 3 4 1 1 12 12 2 1 2 1212 12 1 1 2 3 2 21212 1212 12 12 121212 1 2 1 3 3 3 Note that we consider labeled trees, that is, although there is only one tree of order 3 in the sense of graph isomorphism, there are 3 different labeled trees obtained by marking the inner vertex 1, 2 or 3. For n = 5 there are three non-isomorphic trees: 5 60 60 For the first tree there are clearly 5 different labelings, and for the second and third there are 5! 2 = 60 labelings, so we obtain T5 = 125. This should be enough to conjecture Tn = nn−2, and that is precisely Cayley’s result. Theorem. There are nn−2 different labeled trees on n vertices. This beautiful formula yields to equally beautiful proofs, drawing on a variety of combinatorial and algebraic techniques. We will outline three of them before presenting the proof which is to date the most beautiful of them all. Arthur Cayley There are nn2 trees on n distinct vertices. Cayley’s formula:
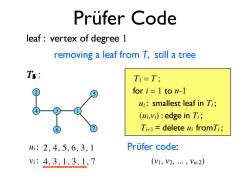
Prufer Code leaf:vertex of degree 1 removing a leaf from T,still a tree Ts: T1=T; for i=1 to n-1 ui:smallest leaf in Ti; (ui,vi):edge in Ti; Tit1=delete ui fromTi; :2,4,5,6,3,1 Prufer code: :4,3,1,3,1,7 (1,V2,…,Vn-2)
Prüfer Code 4 3 6 2 5 1 7 T1 = T ; ui : smallest leaf in Ti ; (ui,vi) : edge in Ti ; Ti+1 = delete ui fromTi ; for i = 1 to n-1 Prüfer code: (v1, v2, ... , vn-2) T : ui : vi : T23145 4, 3, 1, 3, 1, 7 2, 4, 5, 6, 3, 1 T6 leaf : vertex of degree 1 removing a leaf from T, still a tree
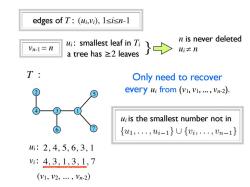
edges of T:(ui,vi),1sisn-1 ui:smallest leaf in Ti n is never deleted Vn-1=n a tree has≥2 leaves }> li≠n Only need to recover every ui from (v1,v1,...,Vn-2). ui is the smallest number not in {u1,.,u-1}U{v,,vn-1} u:2,4,5,6,3,1 w:4,3.1,3,1,7 (y1,V2,…,yn-2)
4 3 6 2 5 1 7 T : ui : vi : 4, 3, 1, 3, 1, 7 2, 4, 5, 6, 3, 1 (v1, v2, ... , vn-2) edges of T : (ui,vi), 1≤i≤n-1 vn-1 = n a tree has ≥2 leaves ui : smallest leaf in Ti } ui ≠ n n is never deleted Only need to recover every ui from (v1, v1, ... , vn-2). {u1,...,ui1} {vi,...,vn1} ui is the smallest number not in
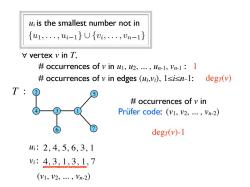
ui is the smallest number not in {u1,.,ui-1}U{i,.,n-1} V vertex v in T, occurrences of v in u1,u2,...un-1,Vn-1 1 occurrences of v in edges (ui,v),1sisn-1: degn(v) T: occurrences of v in 4 3 Prufer code:(v1,v2,...Vn-2) degn(v)-1 :2,4,5,6,3,1 :4,3,1,3,1,7 (V1,V2,…,Vn-2)
4 3 6 2 5 1 7 T : ui : vi : 4, 3, 1, 3, 1, 7 2, 4, 5, 6, 3, 1 (v1, v2, ... , vn-2) {u1,...,ui1} {vi,...,vn1} ui is the smallest number not in ∀ vertex v in T, # occurrences of v in u1, u2, ... , un-1, vn-1 : 1 # occurrences of v in edges (ui,vi), 1≤i≤n-1: degT(v) # occurrences of v in Prüfer code: (v1, v2, ... , vn-2) degT(v)-1
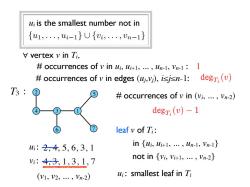
ui is the smallest number not in {u1,,u-1}U{,.,vn-1} V vertex v in Ti, occurrences of v in ui,ui+1,...un-1,Vn-1 1 occurrences of v in edges (uj,v),isjsn-1: degr(v) T3: #occurrences of v in (vi,...Vn-2) degr;(v)-1 leaf v of Ti: u:2,4,5,6,3,1 in ui,uitl,...un-1,Vn-1 :431,3,1,7 not in vi,vi+l,...Vn-2} (y1,V2,.,Vn-2) ui:smallest leaf in T
4 3 6 2 5 1 7 T3 : ui : vi : 4, 3, 1, 3, 1, 7 2, 4, 5, 6, 3, 1 (v1, v2, ... , vn-2) {u1,...,ui1} {vi,...,vn1} ui is the smallest number not in ∀ vertex v in Ti, # occurrences of v in ui, ui+1, ... , un-1, vn-1 : 1 # occurrences of v in edges (uj,vj), i≤j≤n-1: # occurrences of v in (vi, ... , vn-2) LMOTi (v) LMOTi (v) 1 leaf v of Ti : in {ui, ui+1, ... , un-1, vn-1} not in {vi, vi+1, ... , vn-2} ui : smallest leaf in Ti
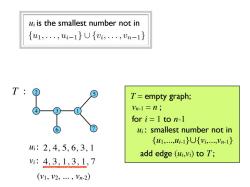
ui is the smallest number not in {u1,.,ui-1}U{i,…,n-1} T T=empty graph; Vn-1=N; for i=1 to n-1 ui:smallest number not in ul,...ui-1vis....vn-1h 2,4,5,6,3,1 add edge(ui,vi)to T; :4,3,13,1,7 (V1,V2,.,Vn-2)
4 3 6 2 5 1 7 T : ui : vi : 4, 3, 1, 3, 1, 7 2, 4, 5, 6, 3, 1 (v1, v2, ... , vn-2) {u1,...,ui1} {vi,...,vn1} ui is the smallest number not in T = empty graph; ui : smallest number not in add edge (ui,vi) to T ; for i = 1 to n-1 {u1,...,ui-1}∪{vi,...,vn-1} vn-1 = n ;
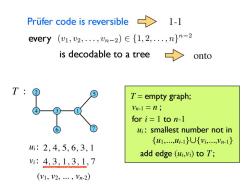
Prufer code is reversible 1-1 every(1,2,,n-2)∈{1,2,.…,n}n-2 is decodable to a tree > onto T T=empty graph; Vn-1 =n; for i=1 to n-1 ui:smallest number not in ul,...ui-1vis...Vn-1} u:2,4,5,6,3,1 add edge (ui,vi)to T; :4,3,1,3,1,7 (V1,V2,.,Vn-2)
4 3 6 2 5 1 7 T : ui : vi : 4, 3, 1, 3, 1, 7 2, 4, 5, 6, 3, 1 (v1, v2, ... , vn-2) T = empty graph; ui : smallest number not in add edge (ui,vi) to T ; for i = 1 to n-1 {u1,...,ui-1}∪{vi,...,vn-1} vn-1 = n ; Prüfer code is reversible 1-1 every (v1, v2,...,vn2) {1, 2,...,n}n2 is decodable to a tree onto
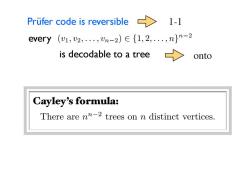
Prufer code is reversible > 1-1 every(,2,,un-2)∈{1,2,.,m}n-2 is decodable to a tree onto Cayley's formula: There are n"-2 trees on n distinct vertices
Prüfer code is reversible 1-1 every (v1, v2,...,vn2) {1, 2,...,n}n2 is decodable to a tree onto There are nn2 trees on n distinct vertices. Cayley’s formula:
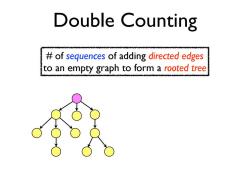
Double Counting of sequences of adding directed edges to an empty graph to form a rooted tree
# of sequences of adding directed edges to an empty graph to form a rooted tree Double Counting
按次数下载不扣除下载券;
注册用户24小时内重复下载只扣除一次;
顺序:VIP每日次数-->可用次数-->下载券;
- 南京大学:《组合数学》课程教学资源(课堂讲义)筛法 Sieve methods.pdf
- 南京大学:《组合数学》课程教学资源(课堂讲义)生成函数 Generating functions.pdf
- 南京大学:《组合数学》课程教学资源(课堂讲义)基本计数 Basic enumeration.pdf
- 南京大学:《组合数学》课程教学资源(课堂讲义)课程简介 Combinatorics Introduction(主讲:尹一通).pdf
- 南京大学:《高级优化 Advanced Optimization》课程教学资源(讲稿)Lecture 13 Advanced Topics - non-stationary online learning, universal online learning, online ensemble, base algorithm, meta algorithm.pdf
- 南京大学:《高级优化 Advanced Optimization》课程教学资源(讲稿)Lecture 12 Stochastic Bandits - MAB, UCB, linear bandits, self-normalized concentration, generalized linear bandits.pdf
- 南京大学:《高级优化 Advanced Optimization》课程教学资源(讲稿)Lecture 11 Adversarial Bandits - MAB, IW estimator, Exp3, lower bound, BCO, gradient estimator, self-concordant barrier.pdf
- 南京大学:《高级优化 Advanced Optimization》课程教学资源(讲稿)Lecture 10 Online Learning in Games - two-player zero-sum games, repeated play, minimax theorem, fast convergence.pdf
- 南京大学:《高级优化 Advanced Optimization》课程教学资源(讲稿)Lecture 09 Optimistic Online Mirror Descent - optimistic online learning, predictable sequence, small-loss bound, gradient-variance bound, gradient-variation bound.pdf
- 南京大学:《高级优化 Advanced Optimization》课程教学资源(讲稿)Lecture 08 Adaptive Online Convex Optimization - problem-dependent guarantee, small-loss bound, self-confident tuning, small-loss OCO, self-bounding property bound.pdf
- 南京大学:《高级优化 Advanced Optimization》课程教学资源(讲稿)Lecture 07 Online Mirror Descent - OMD framework, regret analysis, primal-dual view, mirror map, FTRL, dual averaging.pdf
- 南京大学:《高级优化 Advanced Optimization》课程教学资源(讲稿)Lecture 06 Prediction with Expert Advice - Hedge, minimax bound, lower bound; mirror descent(motivation and preliminary).pdf
- 南京大学:《高级优化 Advanced Optimization》课程教学资源(讲稿)Lecture 05 Online Convex Optimization - OGD, convex functions, strongly convex functions, online Newton step, exp-concave functions.pdf
- 南京大学:《高级优化 Advanced Optimization》课程教学资源(讲稿)Lecture 04 GD Methods II - GD method, smooth optimization, Nesterov’s AGD, composite optimization.pdf
- 南京大学:《高级优化 Advanced Optimization》课程教学资源(讲稿)Lecture 03 GD Methods I - GD method, Lipschitz optimization.pdf
- 南京大学:《高级优化 Advanced Optimization》课程教学资源(讲稿)Lecture 02 Convex Optimization Basics; Function Properties.pdf
- 南京大学:《高级优化 Advanced Optimization》课程教学资源(讲稿)Lecture 01 Introduction; Mathematical Background.pdf
- 南京大学:《数字图像处理》课程教学资源(课件讲义)11 图像特征分析.pdf
- 南京大学:《数字图像处理》课程教学资源(课件讲义)10 图像分割.pdf
- 南京大学:《数字图像处理》课程教学资源(课件讲义)09 形态学及其应用.pdf
- 南京大学:《组合数学》课程教学资源(课堂讲义)Pólya计数法 Pólya's theory of counting.pdf
- 南京大学:《组合数学》课程教学资源(课堂讲义)Ramsey理论 Ramsey theory.pdf
- 南京大学:《组合数学》课程教学资源(课堂讲义)存在性问题 Existence problems.pdf
- 南京大学:《组合数学》课程教学资源(课堂讲义)极值图论 Extremal graph theory.pdf
- 南京大学:《组合数学》课程教学资源(课堂讲义)极值集合论 Extremal set theory.pdf
- 南京大学:《组合数学》课程教学资源(课堂讲义)概率法 The probabilistic method.pdf
- 南京大学:《组合数学》课程教学资源(课堂讲义)匹配论 Matching theory.pdf
- 南京大学:《高级机器学习》课程教学资源(课件讲稿)01 基础(主讲:詹德川).pdf
- 南京大学:《高级机器学习》课程教学资源(课件讲稿)02 典型方法.pdf
- 《互联网营销理论与工具运用》课程教学资源(教案)项目一 走进互联网营销.pdf
- 《互联网营销理论与工具运用》课程教学资源(教案)项目二 互联网营销市场调研.pdf
- 《互联网营销理论与工具运用》课程教学资源(教案)项目三 互联网搜索引擎营销.pdf
- 《互联网营销理论与工具运用》课程教学资源(教案)项目四 互联网电子邮件营销.pdf
- 《互联网营销理论与工具运用》课程教学资源(教案)项目五 互联网社交媒体营销.pdf
- 《互联网营销理论与工具运用》课程教学资源(教案)项目六 互联网视频营销.pdf
- 《互联网营销理论与工具运用》课程教学资源(教案)项目七 互联网直播营销.pdf
- 《互联网营销理论与工具运用》课程教学资源(教案)项目八 互联网营销方案策划.pdf
- 《互联网营销理论与工具运用》课程教学资源(习题)题库1.pdf
- 《互联网营销理论与工具运用》课程教学资源(习题)题库2.pdf
- 《互联网营销理论与工具运用》课程教学资源(习题)题库3.pdf