南京大学:《组合数学》课程教学资源(课堂讲义)概率法 The probabilistic method
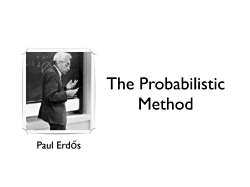
The Probabilistic Method Paul Erdos
The Probabilistic Method Paul Erdős
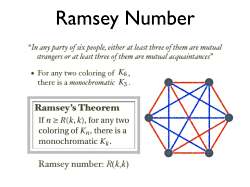
Ramsey Number "In any party of six people,either at least three of them are mutual strangers or at least three of them are mutual acquaintances" For any two coloring of K6, there is a monocbromatic K3. Ramsey's Theorem If n =R(k,k),for any two coloring of Kn,there is a monochromatic Kk. Ramsey number:R(k,k)
Ramsey Number • For any two coloring of , there is a monochromatic . “In any party of six people, either at least three of them are mutual strangers or at least three of them are mutual acquaintances” K6 K3 Ramsey’s Theorem If n R(k,k), for any two coloring of Kn, there is a monochromatic Kk . Ramsey number: R(k,k)
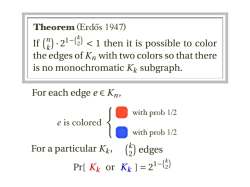
Theorem (Erdos 1947) If(W·2l-(⑤<1 then it is possible to color the edges of Kn with two colors so that there is no monochromatic Kk subgraph. For each edge ee Kn, with prob 1/2 eiscolored 8 with prob 1/2 For a particular Kk,(edges Pr[Kk or Kk]=2l-(⑤
If n k ⇥ · 21 k 2 ⇥ < 1 then it is possible to color the edges of Kn with two colors so that there is no monochromatic Kk subgraph. Theorem (Erdős 1947) e is colored with prob 1/2 with prob 1/2 For a particular Kk , k 2 ⇥ edges Pr[ or ] = 21 k 2 ⇥ Kk Kk For each edge e Kn
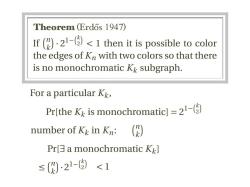
Theorem (Erdos 1947) If(W·2l-(均<1 then it is possible to color the edges of Kn with two colors so that there is no monochromatic Ki subgraph. For a particular Kk, Prlthe K&is monochromatic]=21-( number of Kk in Kn: ( Pr[3 a monochromatic Kk] ≤(阳·21-③<1
If n k ⇥ · 21 k 2 ⇥ < 1 then it is possible to color the edges of Kn with two colors so that there is no monochromatic Kk subgraph. Theorem (Erdős 1947) For a particular Kk , Pr[the Kk is monochromatic] = 21 k 2 ⇥ number of Kk in Kn: n k ⇥ Pr[ a monochromatic Kk ] ⇤ n k ⇥ · 21 k 2 ⇥ < 1
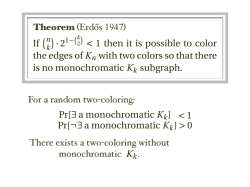
Theorem (Erdos 1947) If(份·2l-(0 There exists a two-coloring without monochromatic Kk
If n k ⇥ · 21 k 2 ⇥ 0 There exists a two-coloring without monochromatic . Kk
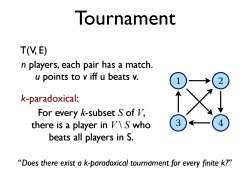
Tournament T(V,E) n players,each pair has a match. u points to v iff u beats v. k-paradoxical: For every k-subset S of V, there is a player in D\S who beats all players in S. "Does there exist a k-paradoxical tournament for every finite k?
Tournament n players, each pair has a match. u points to v iff u beats v. k-paradoxical: For every k-subset S of V, there is a player in V \ S who beats all players in S. T(V, E) “Does there exist a k-paradoxical tournament for every finite k?
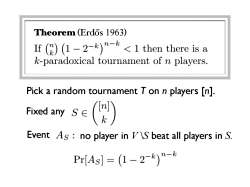
Theorem (Erdos 1963) If ()(1-k)<1 then there is a k-paradoxical tournament of n players. Pick a random tournament T on n players [n]. Event As:no player in \S beat all players in S. Pr[As]=(1-2k)-
If n k ⇥ 1 2k⇥nk < 1 then there is a k-paradoxical tournament of n players. Theorem (Erdős 1963) Pick a random tournament T on n players [n]. Fixed any S [n] k ⇥ Event AS : no player in V \S beat all players in S. Pr[AS] = 1 2k⇥nk
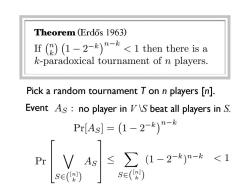
Theorem (Erdos 1963) If ()(1-2-)<1 then there is a k-paradoxical tournament of n players. Pick a random tournament T on n players [n]. Event As no player in \S beat all players in S. PrAs=((1-2k)”- Pr As≤∑(1-2)m-k<1 s∈() s∈(R)
If n k ⇥ 1 2k⇥nk < 1 then there is a k-paradoxical tournament of n players. Theorem (Erdős 1963) Pick a random tournament T on n players [n]. Event AS : no player in V \S beat all players in S. Pr[AS] = 1 2k⇥nk Pr < 1 ⇧ ⇤ ⌥ S( [n] k ) AS ⇥ ⌃ ⌅ ⇥ S⇥( [n] k ) (1 2k) nk
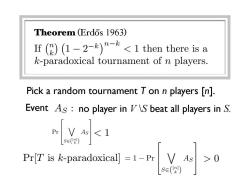
Theorem (Erdos 1963) If ()(1-2-k)"<1 then there is a k-paradoxical tournament of n players. Pick a random tournament T on n players [n]. Event As:no player in \S beat all players in S. Pr V As <1 se(g)」 s∈()
If n k ⇥ 1 2k⇥nk 0
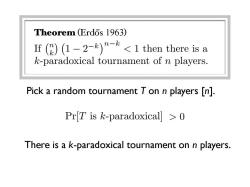
Theorem (Erd6s 1963) If ()(1--k)0 There is a k-paradoxical tournament on n players
If n k ⇥ 1 2k⇥nk 0 There is a k-paradoxical tournament on n players
按次数下载不扣除下载券;
注册用户24小时内重复下载只扣除一次;
顺序:VIP每日次数-->可用次数-->下载券;
- 南京大学:《组合数学》课程教学资源(课堂讲义)极值集合论 Extremal set theory.pdf
- 南京大学:《组合数学》课程教学资源(课堂讲义)极值图论 Extremal graph theory.pdf
- 南京大学:《组合数学》课程教学资源(课堂讲义)存在性问题 Existence problems.pdf
- 南京大学:《组合数学》课程教学资源(课堂讲义)Ramsey理论 Ramsey theory.pdf
- 南京大学:《组合数学》课程教学资源(课堂讲义)Pólya计数法 Pólya's theory of counting.pdf
- 南京大学:《组合数学》课程教学资源(课堂讲义)Cayley公式 Cayley's formula.pdf
- 南京大学:《组合数学》课程教学资源(课堂讲义)筛法 Sieve methods.pdf
- 南京大学:《组合数学》课程教学资源(课堂讲义)生成函数 Generating functions.pdf
- 南京大学:《组合数学》课程教学资源(课堂讲义)基本计数 Basic enumeration.pdf
- 南京大学:《组合数学》课程教学资源(课堂讲义)课程简介 Combinatorics Introduction(主讲:尹一通).pdf
- 南京大学:《高级优化 Advanced Optimization》课程教学资源(讲稿)Lecture 13 Advanced Topics - non-stationary online learning, universal online learning, online ensemble, base algorithm, meta algorithm.pdf
- 南京大学:《高级优化 Advanced Optimization》课程教学资源(讲稿)Lecture 12 Stochastic Bandits - MAB, UCB, linear bandits, self-normalized concentration, generalized linear bandits.pdf
- 南京大学:《高级优化 Advanced Optimization》课程教学资源(讲稿)Lecture 11 Adversarial Bandits - MAB, IW estimator, Exp3, lower bound, BCO, gradient estimator, self-concordant barrier.pdf
- 南京大学:《高级优化 Advanced Optimization》课程教学资源(讲稿)Lecture 10 Online Learning in Games - two-player zero-sum games, repeated play, minimax theorem, fast convergence.pdf
- 南京大学:《高级优化 Advanced Optimization》课程教学资源(讲稿)Lecture 09 Optimistic Online Mirror Descent - optimistic online learning, predictable sequence, small-loss bound, gradient-variance bound, gradient-variation bound.pdf
- 南京大学:《高级优化 Advanced Optimization》课程教学资源(讲稿)Lecture 08 Adaptive Online Convex Optimization - problem-dependent guarantee, small-loss bound, self-confident tuning, small-loss OCO, self-bounding property bound.pdf
- 南京大学:《高级优化 Advanced Optimization》课程教学资源(讲稿)Lecture 07 Online Mirror Descent - OMD framework, regret analysis, primal-dual view, mirror map, FTRL, dual averaging.pdf
- 南京大学:《高级优化 Advanced Optimization》课程教学资源(讲稿)Lecture 06 Prediction with Expert Advice - Hedge, minimax bound, lower bound; mirror descent(motivation and preliminary).pdf
- 南京大学:《高级优化 Advanced Optimization》课程教学资源(讲稿)Lecture 05 Online Convex Optimization - OGD, convex functions, strongly convex functions, online Newton step, exp-concave functions.pdf
- 南京大学:《高级优化 Advanced Optimization》课程教学资源(讲稿)Lecture 04 GD Methods II - GD method, smooth optimization, Nesterov’s AGD, composite optimization.pdf
- 南京大学:《组合数学》课程教学资源(课堂讲义)匹配论 Matching theory.pdf
- 南京大学:《高级机器学习》课程教学资源(课件讲稿)01 基础(主讲:詹德川).pdf
- 南京大学:《高级机器学习》课程教学资源(课件讲稿)02 典型方法.pdf
- 《互联网营销理论与工具运用》课程教学资源(教案)项目一 走进互联网营销.pdf
- 《互联网营销理论与工具运用》课程教学资源(教案)项目二 互联网营销市场调研.pdf
- 《互联网营销理论与工具运用》课程教学资源(教案)项目三 互联网搜索引擎营销.pdf
- 《互联网营销理论与工具运用》课程教学资源(教案)项目四 互联网电子邮件营销.pdf
- 《互联网营销理论与工具运用》课程教学资源(教案)项目五 互联网社交媒体营销.pdf
- 《互联网营销理论与工具运用》课程教学资源(教案)项目六 互联网视频营销.pdf
- 《互联网营销理论与工具运用》课程教学资源(教案)项目七 互联网直播营销.pdf
- 《互联网营销理论与工具运用》课程教学资源(教案)项目八 互联网营销方案策划.pdf
- 《互联网营销理论与工具运用》课程教学资源(习题)题库1.pdf
- 《互联网营销理论与工具运用》课程教学资源(习题)题库2.pdf
- 《互联网营销理论与工具运用》课程教学资源(习题)题库3.pdf
- 《互联网营销理论与工具运用》课程教学资源(讲义)课程标准.docx
- 《互联网营销理论与工具运用》课程教学资源(PPT课件)01 走进互联网营销.pptx
- 《互联网营销理论与工具运用》课程教学资源(PPT课件)02 互联网营销市场调研.pptx
- 《互联网营销理论与工具运用》课程教学资源(PPT课件)03 互联网搜索引擎营销.pptx
- 《互联网营销理论与工具运用》课程教学资源(PPT课件)04 互联网电子邮件营销.pptx
- 《互联网营销理论与工具运用》课程教学资源(PPT课件)05 互联网社交媒体营销.pptx