西安电子科技大学:《神经网络与模糊系统 Neural Networks and Fuzzy Systems》课程PPT课件讲稿(2004)Chapter 02 ACTIVATIONS AND SIGNALS
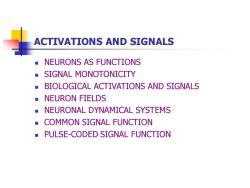
ACTIVATIONS AND SIGNALS ◆ NEURONS AS FUNCTIONS SIGNAL MONOTONICITY ■ BIOLOGICAL ACTIVATIONS AND SIGNALS NEURON FIELDS NEURONAL DYNAMICAL SYSTEMS COMMON SIGNAL FUNCTION PULSE-CODED SIGNAL FUNCTION
ACTIVATIONS AND SIGNALS ◼ NEURONS AS FUNCTIONS ◼ SIGNAL MONOTONICITY ◼ BIOLOGICAL ACTIVATIONS AND SIGNALS ◼ NEURON FIELDS ◼ NEURONAL DYNAMICAL SYSTEMS ◼ COMMON SIGNAL FUNCTION ◼ PULSE-CODED SIGNAL FUNCTION
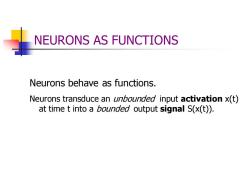
NEURONS AS FUNCTIONS Neurons behave as functions. Neurons transduce an unbounded input activation x(t) at time t into a bounded output signal S(x(t))
NEURONS AS FUNCTIONS Neurons behave as functions. Neurons transduce an unbounded input activation x(t) at time t into a bounded output signal S(x(t))
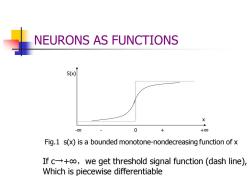
NEURONS AS FUNCTIONS S(x) X -00 0 + +00 Fig.1 s(x)is a bounded monotone-nondecreasing function of x If c+,we get threshold signal function (dash line) Which is piecewise differentiable
NEURONS AS FUNCTIONS S(x) x -∞ - 0 + +∞ Fig.1 s(x) is a bounded monotone-nondecreasing function of x If c→+∞,we get threshold signal function (dash line), Which is piecewise differentiable
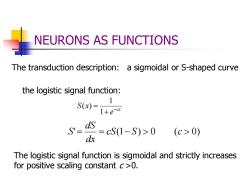
NEURONS AS FUNCTIONS The transduction description:a sigmoidal or S-shaped curve the logistic signal function: S(x)= l+e-cx S'= dS =cS(1-S)>0 (c>0) dx The logistic signal function is sigmoidal and strictly increases for positive scaling constant c>0
NEURONS AS FUNCTIONS The transduction description: a sigmoidal or S-shaped curve the logistic signal function: cx e S x − + = 1 1 ( ) ' = = cS(1− S) 0 (c 0) dx dS S The logistic signal function is sigmoidal and strictly increases for positive scaling constant c >0
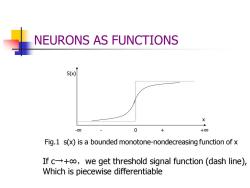
NEURONS AS FUNCTIONS S(x) X -00 0 + +00 Fig.1 s(x)is a bounded monotone-nondecreasing function of x If c+,we get threshold signal function (dash line) Which is piecewise differentiable
NEURONS AS FUNCTIONS S(x) x -∞ - 0 + +∞ Fig.1 s(x) is a bounded monotone-nondecreasing function of x If c→+∞,we get threshold signal function (dash line), Which is piecewise differentiable
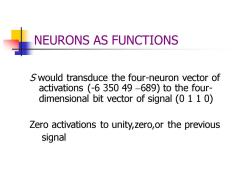
NEURONS AS FUNCTIONS Swould transduce the four-neuron vector of activations (-6 350 49 -689)to the four- dimensional bit vector of signal(0 1 1 0) Zero activations to unity,zero,or the previous signal
NEURONS AS FUNCTIONS S would transduce the four-neuron vector of activations (-6 350 49 –689) to the fourdimensional bit vector of signal (0 1 1 0) Zero activations to unity,zero,or the previous signal
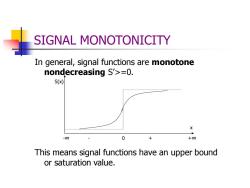
SIGNAL MONOTONICITY In general,signal functions are monotone nondecreasing S'>=0. S(x) X -00 0 +00 This means signal functions have an upper bound or saturation value
SIGNAL MONOTONICITY In general, signal functions are monotone nondecreasing S’>=0. This means signal functions have an upper bound or saturation value. S(x) x -∞ - 0 + +∞
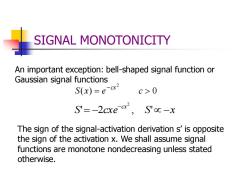
SIGNAL MONOTONICITY An important exception:bell-shaped signal function or Gaussian signal functions S(x)=e-cx2 c>0 S=-2cxe Soc-x The sign of the signal-activation derivation s'is opposite the sign of the activation x.We shall assume signal functions are monotone nondecreasing unless stated otherwise
SIGNAL MONOTONICITY An important exception: bell-shaped signal function or Gaussian signal functions 0 2 = − S x e c cx ( ) S cxe S x cx = − − − ' 2 , ' 2 The sign of the signal-activation derivation s’ is opposite the sign of the activation x. We shall assume signal functions are monotone nondecreasing unless stated otherwise
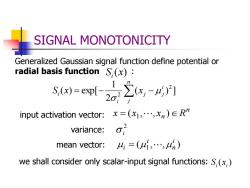
SIGNAL MONOTONICITY Generalized Gaussian signal function define potential or radial basis function S,(x): SU-ml2a2,门 input activation vector::x=(xi,…,xn)∈R" variance: mean vector: 4,=(4,,4) we shall consider only scalar-input signal functions:S,(x)
SIGNAL MONOTONICITY Generalized Gaussian signal function define potential or radial basis function : ( ) ] 2 1 ( ) exp[ 2 = − 2 − n j i j j i i S x x n x = (x1 , , xn )R ( , , ) i n i i = 1 input activation vector: variance: mean vector: 2 i S (x) i we shall consider only scalar-input signal functions: ( ) i i S x
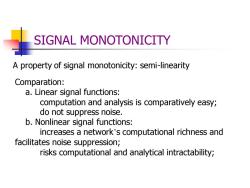
SIGNAL MONOTONICITY A property of signal monotonicity:semi-linearity Comparation: a.Linear signal functions: computation and analysis is comparatively easy; do not suppress noise. b.Nonlinear signal functions: increases a network's computational richness and facilitates noise suppression; risks computational and analytical intractability;
SIGNAL MONOTONICITY A property of signal monotonicity: semi-linearity Comparation: a. Linear signal functions: computation and analysis is comparatively easy; do not suppress noise. b. Nonlinear signal functions: increases a network’s computational richness and facilitates noise suppression; risks computational and analytical intractability;
按次数下载不扣除下载券;
注册用户24小时内重复下载只扣除一次;
顺序:VIP每日次数-->可用次数-->下载券;
- 西安电子科技大学:《神经网络与模糊系统 Neural Networks and Fuzzy Systems》课程PPT课件讲稿(2003)10. 模糊与卡尔曼滤波目标跟踪控制系统的比较 Comparison of Fuzzy and Kalman-Filter Target-Tracking Control Systems.ppt
- 西安电子科技大学:《神经网络与模糊系统 Neural Networks and Fuzzy Systems》课程PPT课件讲稿(2003)09. 模糊图像变换编码 Fuzzy Image Transform Coding.ppt
- 西安电子科技大学:《神经网络与模糊系统 Neural Networks and Fuzzy Systems》课程PPT课件讲稿(2003)08. 模糊与神经网络的比较——以倒车系统为例 Comparison of Fuzzy and Neural Truck Backer-Upper Control Systems.ppt
- 西安电子科技大学:《神经网络与模糊系统 Neural Networks and Fuzzy Systems》课程PPT课件讲稿(2003)07. 模糊联想记忆 Fuzzy Associative Memories(FAM).ppt
- 西安电子科技大学:《神经网络与模糊系统 Neural Networks and Fuzzy Systems》课程PPT课件讲稿(2003)06. 模糊与概率 Fuzziness versus Probability.ppt
- 西安电子科技大学:《神经网络与模糊系统 Neural Networks and Fuzzy Systems》课程PPT课件讲稿(2003)05. 结构和平衡 Architectures and Equilibria.ppt
- 西安电子科技大学:《神经网络与模糊系统 Neural Networks and Fuzzy Systems》课程PPT课件讲稿(2003)04. 突触动力学Ⅱ:有监督学习 Synaptic Dynamics II——Supervised Learning(2/2).ppt
- 西安电子科技大学:《神经网络与模糊系统 Neural Networks and Fuzzy Systems》课程PPT课件讲稿(2003)03. 突触动力学 - 非监督学习 Synaptic Dynamics I——Unsupervised Learning(2/2).ppt
- 西安电子科技大学:《神经网络与模糊系统 Neural Networks and Fuzzy Systems》课程PPT课件讲稿(2003)03. 突触动力学 - 非监督学习 Synaptic Dynamics I——Unsupervised Learning(1/2).ppt
- 西安电子科技大学:《神经网络与模糊系统 Neural Networks and Fuzzy Systems》课程PPT课件讲稿(2003)02. Neuronal Dynamics——Activation Models(2/2).ppt
- 西安电子科技大学:《神经网络与模糊系统 Neural Networks and Fuzzy Systems》课程PPT课件讲稿(2003)02. Neuronal Dynamics——Activation Models(1/2).ppt
- 西安电子科技大学:《神经网络与模糊系统 Neural Networks and Fuzzy Systems》课程PPT课件讲稿(2003)01.Neuronal Dynamics——Activations and Signals(主讲:高新波).ppt
- 《神经网络与模糊系统》课程教学资源(主题演讲)选择性集成 Selective Ensemble(南京大学:周志华).ppt
- 《神经网络与模糊系统》课程教学资源(主题演讲)机器学习研究进展(南京大学:王珏).ppt
- 西安电子科技大学:《神经网络与模糊系统 Neural Networks and Fuzzy Systems》课程教学资源(学科综述)进化计算 SOFT COMPUTING Evolutionary Computing.ppt
- 西安电子科技大学:《神经网络与模糊系统 Neural Networks and Fuzzy Systems》课程教学资源(学科综述)模糊系统与模糊逻辑 Fuzzy Theory.ppt
- 西安电子科技大学:《神经网络与模糊系统 Neural Networks and Fuzzy Systems》课程教学资源(学科综述)模糊神经网络 Neuro-fuzzy Systems.ppt
- 西安电子科技大学:《神经网络与模糊系统 Neural Networks and Fuzzy Systems》课程教学资源(学科综述)人工神经网络 Artificial Neural Networks.ppt
- 西安电子科技大学:《神经网络与模糊系统 Neural Networks and Fuzzy Systems》课程教学资源(学科综述)有关人工智能的故事.doc
- 西安电子科技大学:《神经网络与模糊系统 Neural Networks and Fuzzy Systems》课程教学资源(学科综述)人工智能 AI.ppt
- 西安电子科技大学:《神经网络与模糊系统 Neural Networks and Fuzzy Systems》课程PPT课件讲稿(2004)Chapter 03 Neuronal Dynamics 2:Activation Models.ppt
- 西安电子科技大学:《神经网络与模糊系统 Neural Networks and Fuzzy Systems》课程PPT课件讲稿(2004)Chapter 04 SYNAPTIC DYNAMICS 1:UNSUPERVISED LEARNING.ppt
- 西安电子科技大学:《神经网络与模糊系统 Neural Networks and Fuzzy Systems》课程PPT课件讲稿(2004)Chapter 05-1 第五章 突触动力学Ⅱ:有监督学习.ppt
- 西安电子科技大学:《神经网络与模糊系统 Neural Networks and Fuzzy Systems》课程PPT课件讲稿(2004)Chapter 05-2 Synaptic DynamicsII:Supervised Learning.ppt
- 西安电子科技大学:《神经网络与模糊系统 Neural Networks and Fuzzy Systems》课程PPT课件讲稿(2004)Chapter 06 Architecture and Equilibra 结构和平衡.ppt
- 西安电子科技大学:《神经网络与模糊系统 Neural Networks and Fuzzy Systems》课程PPT课件讲稿(2004)Chapter 07-1 Fuzziness vs. Probability.ppt
- 西安电子科技大学:《神经网络与模糊系统 Neural Networks and Fuzzy Systems》课程PPT课件讲稿(2004)Chapter 07-2 Fuzziness vs. Probability 模糊集合的模糊程度——模糊熵.ppt
- 西安电子科技大学:《神经网络与模糊系统 Neural Networks and Fuzzy Systems》课程PPT课件讲稿(2004)Chapter 08-1 Fuzzy Associative Memories.ppt
- 西安电子科技大学:《神经网络与模糊系统 Neural Networks and Fuzzy Systems》课程PPT课件讲稿(2004)Chapter 10 模糊图像变换编码.ppt
- 西安电子科技大学:《神经网络与模糊系统 Neural Networks and Fuzzy Systems》课程PPT课件讲稿(2004)Chapter 08-2 Fuzzy Associative Memories 模糊联想记忆 FUZZY ASSOCIATIVE MEMMORIESⅡ.ppt
- 西安电子科技大学:《神经网络与模糊系统 Neural Networks and Fuzzy Systems》课程PPT课件讲稿(2004)Chapter 09-2 模糊倒车控制系统——拖斗拖车.ppt
- 西安电子科技大学:《神经网络与模糊系统 Neural Networks and Fuzzy Systems》课程PPT课件讲稿(2004)Chapter 11 模糊与卡尔曼滤波目标跟踪控制系统的比较 Comparison of Fuzzy and Kalman-Filter Target-Tracking control system.ppt
- 西安电子科技大学:《神经网络与模糊系统 Neural Networks and Fuzzy Systems》课程PPT课件讲稿(2004)Chapter 09-1 模糊与神经网络倒车系统比较.ppt
- 西安电子科技大学:《神经网络与模糊系统 Neural Networks and Fuzzy Systems》课程PPT课件讲稿(2006)Chapter 02 NEURAL DYNAMIC1:ACTIVATIONHS AND SIGNALS(主讲:高新波).ppt
- 西安电子科技大学:《神经网络与模糊系统 Neural Networks and Fuzzy Systems》课程PPT课件讲稿(2006)Chapter 03-1 NEURONAL DYNAMICS 2:ACTIVATION MODELS.ppt
- 西安电子科技大学:《神经网络与模糊系统 Neural Networks and Fuzzy Systems》课程PPT课件讲稿(2006)Chapter 03-2 NEURONAL DYNAMICS 2:ACTIVATION MODELS.ppt
- 西安电子科技大学:《神经网络与模糊系统 Neural Networks and Fuzzy Systems》课程PPT课件讲稿(2006)Chapter 04-1 Synaptic Dynamics:Unsupervised Learning Part Ⅰ.ppt
- 西安电子科技大学:《神经网络与模糊系统 Neural Networks and Fuzzy Systems》课程PPT课件讲稿(2006)Chapter 04-2 Synaptic Dynamics:Unsupervised Learning Part Ⅱ.ppt
- 西安电子科技大学:《神经网络与模糊系统 Neural Networks and Fuzzy Systems》课程PPT课件讲稿(2006)Chapter 04-3 Part3 Differential Heb learning & Differential Competitive learning.ppt
- 西安电子科技大学:《神经网络与模糊系统 Neural Networks and Fuzzy Systems》课程PPT课件讲稿(2006)Chapter 05-1 突触动力学Ⅱ——有监督学习.ppt