西安电子科技大学:《神经网络与模糊系统 Neural Networks and Fuzzy Systems》课程PPT课件讲稿(2004)Chapter 08-2 Fuzzy Associative Memories 模糊联想记忆 FUZZY ASSOCIATIVE MEMMORIESⅡ

模糊联想记忆 FUZZY ASSOCIATIVE Presented by Yang Baisheng E.E.Dept. Xidian University
模糊联想记忆 FUZZY ASSOCIATIVE MEMMORIESⅡ Presented by Yang Baisheng E.E. Dept. Xidian University
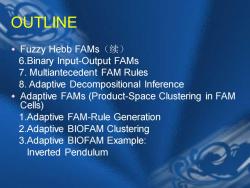
OUTLINE Fuzzy Hebb FAMs(续) 6.Binary Input-Output FAMs 7.Multiantecedent FAM Rules 8.Adaptive Decompositional Inference Adaptive FAMs (Product-Space Clustering in FAM Cells) 1.Adaptive FAM-Rule Generation 2.Adaptive BIOFAM Clustering 3.Adaptive BIOFAM Example: Inverted Pendulum
OUTLINE Fuzzy Hebb FAMs(续) 6.Binary Input-Output FAMs 7. Multiantecedent FAM Rules 8. Adaptive Decompositional Inference Adaptive FAMs (Product-Space Clustering in FAM Cells) 1.Adaptive FAM-Rule Generation 2.Adaptive BIOFAM Clustering 3.Adaptive BIOFAM Example: Inverted Pendulum
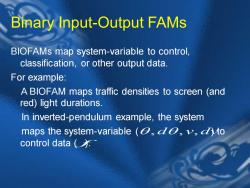
Binary Input-Output FAMs BIOFAMs map system-variable to control, classification,or other output data. For example: A BIOFAM maps traffic densities to screen (and red)light durations. In inverted-pendulum example,the system maps the system-variable (d,v,d)to control data(并
Binary Input-Output FAMs BIOFAMs map system-variable to control, classification, or other output data. For example: A BIOFAM maps traffic densities to screen (and red) light durations. In inverted-pendulum example, the system maps the system-variable ( ) to control data ( ). ,d,v,dv f
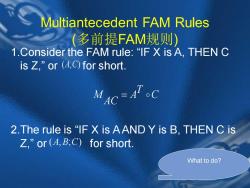
Multiantecedent FAM Rules (多前提FAM规则) 1.Consider the FAM rule:"IF X is A,THEN C isZ,”or(A,C)for short M4c=47.C 2.The rule is "IF X is AAND Y is B,THEN C is Z,”or(A,B,C)for short. What to do?
Multiantecedent FAM Rules (多前提FAM规则) 1.Consider the FAM rule: “IF X is A, THEN C is Z,” or for short. 2.The rule is “IF X is A AND Y is B, THEN C is Z,” or for short. C T A AC M = (A,B;C) (A,C) What to do?
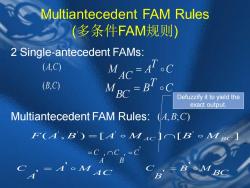
Multiantecedent FAM Rules (多条件FAM规则) 2 Single-antecedent FAMs: (A,C) MAC=A.C (B,C) MBC=BT.C Defuzzify it to yield the exact output. Multiantecedent FAM Rules:(4,B;C) F(A,B)=[AMACIO[BMBC] =C,∩C,=C B
Multiantecedent FAM Rules (多条件FAM规则) 2 Single-antecedent FAMs: Multiantecedent FAM Rules: ( , ) [ ] [ ] ' ' ' ' F A B = A M AC B MBC M AC A A C ' ' = ' ' ' C B C A = C = C ' = BC B M B C ' ' = C T B BC M = (B,C) (A,C) C T A AC M = (A,B;C) Defuzzify it to yield the exact output

Multiantecedent FAM Rules Suppose we present the exact inputs x,,y,to the single-FAM-rule system F that stores(A,B;C). We present the unit bit vectors and I to F as nonfuzzy set inputs.Then F(xy )=F(Ix,I) Property of =[I%MAc]O[Iy MEC] Hebb Matrix =a,∧C∩b,AC =mm(a,b,)ΛC
Multiantecedent FAM Rules Suppose we present the exact inputs , to the single-FAM-rule system that stores(A,B;C). We present the unit bit vectors and to as nonfuzzy set inputs.Then i x ( , ) ( , ) j Y i i j X F x y = F I I [ ] [ ] BC j AC Y i = I X M I M = ai C bj C = min( ai ,bj ) C j y F i X I j Y I F Property of Hebb Matrix
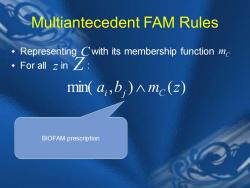
Multiantecedent FAM Rules Representing Cwith its membership function mc ◆For all z in Z min(a,b,)Λmc(2) BIOFAM prescription
Multiantecedent FAM Rules Representing with its membership function For all in : z min( a ,b ) m (z) i j C Z C mC BIOFAM prescription
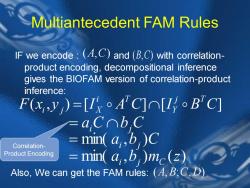
Multiantecedent FAM Rules IF we encode (4,C)and (B,C)with correlation- product encoding,decompositional inference gives the BIOFAM version of correlation-product inference: F(xy )=[IA'C]O[IB"C] a,C⌒b.C Correlation- min(a,,b,)C Product Encoding min(a;,b;)mc(z) Also,We can get the FAM rules:(4,B;C.D)
Multiantecedent FAM Rules Also, We can get the FAM rules: (A,B;C, D) F(x ,y ) [I A C] [I B C] j T Y i T i j X = = ai C bj C = min( ai ,bj )C min( a ,b )m (z) = i j C IF we encode : and with correlationproduct encoding, decompositional inference gives the BIOFAM version of correlation-product inference: (A,C) (B,C) CorrelationProduct Encoding
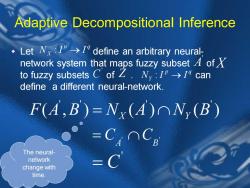
Adaptive Decompositional Inference Let Nx:I”→I'define an arbitrary neural. network system that maps fuzzy subset 4 of to fuzzy subsets C of Z.Ny:I can define a different neural-network. F(A,B)=Nx(A)∩N(B) =C∩Cg The neural- network change with C time
Adaptive Decompositional Inference Let define an arbitrary neuralnetwork system that maps fuzzy subset of to fuzzy subsets of . can define a different neural-network. n q X N : I → I ( , ) ( ) ( ) ' ' ' ' F A B = NX A NY B ' ' A B = C C ' = C p q Y N : I → I ' A X ' C Z The neuralnetwork change with time
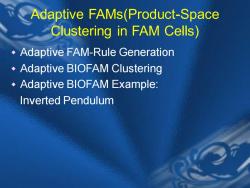
Adaptive FAMs(Product-Space Clustering in FAM Cells) Adaptive FAM-Rule Generation Adaptive BIOFAM Clustering Adaptive BIOFAM Example: Inverted Pendulum
Adaptive FAMs(Product-Space Clustering in FAM Cells) Adaptive FAM-Rule Generation Adaptive BIOFAM Clustering Adaptive BIOFAM Example: Inverted Pendulum
按次数下载不扣除下载券;
注册用户24小时内重复下载只扣除一次;
顺序:VIP每日次数-->可用次数-->下载券;
- 西安电子科技大学:《神经网络与模糊系统 Neural Networks and Fuzzy Systems》课程PPT课件讲稿(2004)Chapter 10 模糊图像变换编码.ppt
- 西安电子科技大学:《神经网络与模糊系统 Neural Networks and Fuzzy Systems》课程PPT课件讲稿(2004)Chapter 08-1 Fuzzy Associative Memories.ppt
- 西安电子科技大学:《神经网络与模糊系统 Neural Networks and Fuzzy Systems》课程PPT课件讲稿(2004)Chapter 07-2 Fuzziness vs. Probability 模糊集合的模糊程度——模糊熵.ppt
- 西安电子科技大学:《神经网络与模糊系统 Neural Networks and Fuzzy Systems》课程PPT课件讲稿(2004)Chapter 07-1 Fuzziness vs. Probability.ppt
- 西安电子科技大学:《神经网络与模糊系统 Neural Networks and Fuzzy Systems》课程PPT课件讲稿(2004)Chapter 06 Architecture and Equilibra 结构和平衡.ppt
- 西安电子科技大学:《神经网络与模糊系统 Neural Networks and Fuzzy Systems》课程PPT课件讲稿(2004)Chapter 05-2 Synaptic DynamicsII:Supervised Learning.ppt
- 西安电子科技大学:《神经网络与模糊系统 Neural Networks and Fuzzy Systems》课程PPT课件讲稿(2004)Chapter 05-1 第五章 突触动力学Ⅱ:有监督学习.ppt
- 西安电子科技大学:《神经网络与模糊系统 Neural Networks and Fuzzy Systems》课程PPT课件讲稿(2004)Chapter 04 SYNAPTIC DYNAMICS 1:UNSUPERVISED LEARNING.ppt
- 西安电子科技大学:《神经网络与模糊系统 Neural Networks and Fuzzy Systems》课程PPT课件讲稿(2004)Chapter 03 Neuronal Dynamics 2:Activation Models.ppt
- 西安电子科技大学:《神经网络与模糊系统 Neural Networks and Fuzzy Systems》课程PPT课件讲稿(2004)Chapter 02 ACTIVATIONS AND SIGNALS.ppt
- 西安电子科技大学:《神经网络与模糊系统 Neural Networks and Fuzzy Systems》课程PPT课件讲稿(2003)10. 模糊与卡尔曼滤波目标跟踪控制系统的比较 Comparison of Fuzzy and Kalman-Filter Target-Tracking Control Systems.ppt
- 西安电子科技大学:《神经网络与模糊系统 Neural Networks and Fuzzy Systems》课程PPT课件讲稿(2003)09. 模糊图像变换编码 Fuzzy Image Transform Coding.ppt
- 西安电子科技大学:《神经网络与模糊系统 Neural Networks and Fuzzy Systems》课程PPT课件讲稿(2003)08. 模糊与神经网络的比较——以倒车系统为例 Comparison of Fuzzy and Neural Truck Backer-Upper Control Systems.ppt
- 西安电子科技大学:《神经网络与模糊系统 Neural Networks and Fuzzy Systems》课程PPT课件讲稿(2003)07. 模糊联想记忆 Fuzzy Associative Memories(FAM).ppt
- 西安电子科技大学:《神经网络与模糊系统 Neural Networks and Fuzzy Systems》课程PPT课件讲稿(2003)06. 模糊与概率 Fuzziness versus Probability.ppt
- 西安电子科技大学:《神经网络与模糊系统 Neural Networks and Fuzzy Systems》课程PPT课件讲稿(2003)05. 结构和平衡 Architectures and Equilibria.ppt
- 西安电子科技大学:《神经网络与模糊系统 Neural Networks and Fuzzy Systems》课程PPT课件讲稿(2003)04. 突触动力学Ⅱ:有监督学习 Synaptic Dynamics II——Supervised Learning(2/2).ppt
- 西安电子科技大学:《神经网络与模糊系统 Neural Networks and Fuzzy Systems》课程PPT课件讲稿(2003)03. 突触动力学 - 非监督学习 Synaptic Dynamics I——Unsupervised Learning(2/2).ppt
- 西安电子科技大学:《神经网络与模糊系统 Neural Networks and Fuzzy Systems》课程PPT课件讲稿(2003)03. 突触动力学 - 非监督学习 Synaptic Dynamics I——Unsupervised Learning(1/2).ppt
- 西安电子科技大学:《神经网络与模糊系统 Neural Networks and Fuzzy Systems》课程PPT课件讲稿(2003)02. Neuronal Dynamics——Activation Models(2/2).ppt
- 西安电子科技大学:《神经网络与模糊系统 Neural Networks and Fuzzy Systems》课程PPT课件讲稿(2004)Chapter 09-2 模糊倒车控制系统——拖斗拖车.ppt
- 西安电子科技大学:《神经网络与模糊系统 Neural Networks and Fuzzy Systems》课程PPT课件讲稿(2004)Chapter 11 模糊与卡尔曼滤波目标跟踪控制系统的比较 Comparison of Fuzzy and Kalman-Filter Target-Tracking control system.ppt
- 西安电子科技大学:《神经网络与模糊系统 Neural Networks and Fuzzy Systems》课程PPT课件讲稿(2004)Chapter 09-1 模糊与神经网络倒车系统比较.ppt
- 西安电子科技大学:《神经网络与模糊系统 Neural Networks and Fuzzy Systems》课程PPT课件讲稿(2006)Chapter 02 NEURAL DYNAMIC1:ACTIVATIONHS AND SIGNALS(主讲:高新波).ppt
- 西安电子科技大学:《神经网络与模糊系统 Neural Networks and Fuzzy Systems》课程PPT课件讲稿(2006)Chapter 03-1 NEURONAL DYNAMICS 2:ACTIVATION MODELS.ppt
- 西安电子科技大学:《神经网络与模糊系统 Neural Networks and Fuzzy Systems》课程PPT课件讲稿(2006)Chapter 03-2 NEURONAL DYNAMICS 2:ACTIVATION MODELS.ppt
- 西安电子科技大学:《神经网络与模糊系统 Neural Networks and Fuzzy Systems》课程PPT课件讲稿(2006)Chapter 04-1 Synaptic Dynamics:Unsupervised Learning Part Ⅰ.ppt
- 西安电子科技大学:《神经网络与模糊系统 Neural Networks and Fuzzy Systems》课程PPT课件讲稿(2006)Chapter 04-2 Synaptic Dynamics:Unsupervised Learning Part Ⅱ.ppt
- 西安电子科技大学:《神经网络与模糊系统 Neural Networks and Fuzzy Systems》课程PPT课件讲稿(2006)Chapter 04-3 Part3 Differential Heb learning & Differential Competitive learning.ppt
- 西安电子科技大学:《神经网络与模糊系统 Neural Networks and Fuzzy Systems》课程PPT课件讲稿(2006)Chapter 05-1 突触动力学Ⅱ——有监督学习.ppt
- 西安电子科技大学:《神经网络与模糊系统 Neural Networks and Fuzzy Systems》课程PPT课件讲稿(2006)Chapter 05-2 Backpropagation Algorithm.ppt
- 西安电子科技大学:《神经网络与模糊系统 Neural Networks and Fuzzy Systems》课程PPT课件讲稿(2006)Chapter 10 模糊图像变换编码.ppt
- 西安电子科技大学:《神经网络与模糊系统 Neural Networks and Fuzzy Systems》课程PPT课件讲稿(2006)Chapter 05-3 突触动力学Ⅱ:有监督的学习.ppt
- 西安电子科技大学:《神经网络与模糊系统 Neural Networks and Fuzzy Systems》课程PPT课件讲稿(2006)Chapter 06 Architecture and Equilibria 结构和平衡.ppt
- 西安电子科技大学:《神经网络与模糊系统 Neural Networks and Fuzzy Systems》课程PPT课件讲稿(2006)Chapter 07-1 模糊与概率(一).ppt
- 西安电子科技大学:《神经网络与模糊系统 Neural Networks and Fuzzy Systems》课程PPT课件讲稿(2006)Chapter 07-2 模糊与概率(二).ppt
- 西安电子科技大学:《神经网络与模糊系统 Neural Networks and Fuzzy Systems》课程PPT课件讲稿(2006)Chapter 08-1 Fuzzy Associative Memories(1/3).ppt
- 西安电子科技大学:《神经网络与模糊系统 Neural Networks and Fuzzy Systems》课程PPT课件讲稿(2006)Chapter 08-2 Fuzzy Associative Memories(2/3).ppt
- 西安电子科技大学:《神经网络与模糊系统 Neural Networks and Fuzzy Systems》课程PPT课件讲稿(2006)Chapter 08-3 Fuzzy Associative Memories(3/3).ppt
- 西安电子科技大学:《神经网络与模糊系统 Neural Networks and Fuzzy Systems》课程PPT课件讲稿(2006)Chapter 11 模糊与卡尔曼滤波目标跟踪控制系统的比较 Comparison of Fuzzy and Kalman-Filter Target-Tracking control system.ppt