西安电子科技大学:《神经网络与模糊系统 Neural Networks and Fuzzy Systems》课程教学资源(学科综述)模糊神经网络 Neuro-fuzzy Systems

Soft Computing integrating Evolutionary Neural and Fuzzy Systeme Neuro-fuzzy Systems Xinbo Gao School of Electronic Engineering Xidian University 2004,10
Neuro-fuzzy Systems Xinbo Gao School of Electronic Engineering Xidian University 2004,10
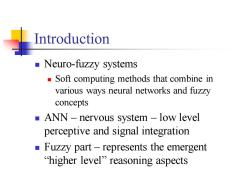
Introduction Neuro-fuzzy systems Soft computing methods that combine in various ways neural networks and fuzzy concepts ANN-nervous system -low level perceptive and signal integration Fuzzy part-represents the emergent higher level"reasoning aspects
Introduction ◼ Neuro-fuzzy systems ◼ Soft computing methods that combine in various ways neural networks and fuzzy concepts ◼ ANN – nervous system – low level perceptive and signal integration ◼ Fuzzy part – represents the emergent “higher level” reasoning aspects
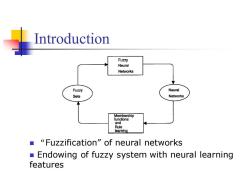
Introduction Fuzzy Neural Networks Fuzzy Neural Sets Networks Membership functions and Rule learning ■“Fuzzification"of neural networks Endowing of fuzzy system with neural learning features
Introduction ◼ “Fuzzification” of neural networks ◼ Endowing of fuzzy system with neural learning features

Introduction Co-operative-neural algorithm adapt fuzzy systems Off-line -adaptation On-line-algorithms are used to adapt as the system operates ■( Concurrent -where the two techniques are applied after one another as pre-or post-processing Hybrid-fuzzy system being representedas a network structure, making it possible to take advantage of learning algorithm inherited from ANNs
Introduction ◼ Co-operative-neural algorithm adapt fuzzy systems ◼ Off-line – adaptation ◼ On-line – algorithms are used to adapt as the system operates ◼ Concurrent – where the two techniques are applied after one another as pre- or post-processing ◼ Hybrid – fuzzy system being represented as a network structure, making it possible to take advantage of learning algorithm inherited from ANNs
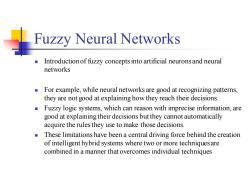
Fuzzy Neural Networks Introduction of fuzzy concepts into artificial neurons and neural networks For example,while neural networks are good at recognizing patterns, they are not good at explaining how they reach their decisions. Fuzzy logic systems,which can reason with imprecise information,are good at explaining their decisions but they cannot automatically acquire the rules they use to make those decisions. These limitations have been a central driving force behind the creation of intelligent hybrid systems where two or more techniques are combined in a manner that overcomes individual techniques
Fuzzy Neural Networks ◼ Introduction of fuzzy concepts into artificial neurons and neural networks ◼ For example, while neural networks are good at recognizing patterns, they are not good at explaining how they reach their decisions. ◼ Fuzzy logic systems, which can reason with imprecise information, are good at explaining their decisions but they cannot automatically acquire the rules they use to make those decisions. ◼ These limitations have been a central driving force behind the creation of intelligent hybrid systems where two or more techniques are combined in a manner that overcomes individual techniques
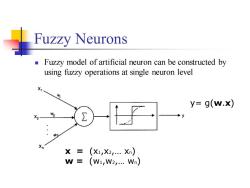
Fuzzy Neurons Fuzzy model of artificial neuron can be constructed by using fuzzy operations at single neuron level y=g(w.x) 2 % Xn X (X1,X2…Xn) W= (W1,W2,…Wn)
Fuzzy Neurons ◼ Fuzzy model of artificial neuron can be constructed by using fuzzy operations at single neuron level x = (x1,x2,… xn) w = (w1,w2,… wn) y= g(w.x)
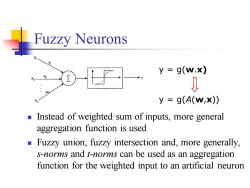
Fuzzy Neurons X y=g(w.x) y g(A(W,x)) Instead of weighted sum of inputs,more general aggregation function is used Fuzzy union,fuzzy intersection and,more generally. s-norms and t-norms can be used as an aggregation function for the weighted input to an artificial neuron
Fuzzy Neurons y = g(w.x) y = g(A(w,x)) ◼ Instead of weighted sum of inputs, more general aggregation function is used ◼ Fuzzy union, fuzzy intersection and, more generally, s-norms and t-norms can be used as an aggregation function for the weighted input to an artificial neuron

OR Fuzzy Neuron X AND W. X2AND W2 0R:[0,1]x[0,1]n->[0,1] OR y X AND Wn y=OR(X1 AND W1,X2 AND W2 ..Xn AND Wn) Transfer function g is linear If wk=0 then wk AND Xk-0 while if wk=1 then wk AND Xk=Xk independent of xk
OR Fuzzy Neuron ◼ Transfer function g is linear ◼ If wk=0 then wk AND xk=0 while if wk=1 then wk AND xk= xk independent of xk y=OR(x1 AND w1 , x2 AND w2 … xn AND wn) OR:[0,1]x[0,1]n->[0,1]
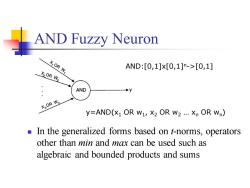
AND Fuzzy Neuron X OR W. AND:[0,1]x[0,1]n->[0,1] XOR W AND XnOR Wo y=AND(X1 OR W1,X2 OR W2 ..Xn OR Wn) In the generalized forms based on t-norms,operators other than min and max can be used such as algebraic and bounded products and sums
AND Fuzzy Neuron ◼ In the generalized forms based on t-norms, operators other than min and max can be used such as algebraic and bounded products and sums y=AND(x1 OR w1 , x2 OR w2 … xn OR wn) AND:[0,1]x[0,1]n->[0,1]
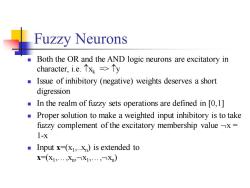
Fuzzy Neurons Both the OR and the aNd logic neurons are excitatory in character,i.e.Tx =Ty ■ Issue of inhibitory (negative)weights deserves a short digression ■ In the realm of fuzzy sets operations are defined in [0,1] Proper solution to make a weighted input inhibitory is to take fuzzy complement of the excitatory membership value-x= 1-x Input x=(x1,..x)is extended to X=(X1,…,XmX1,…,7Xn)
Fuzzy Neurons ◼ Both the OR and the AND logic neurons are excitatory in character, i.e. xk => y ◼ Issue of inhibitory (negative) weights deserves a short digression ◼ In the realm of fuzzy sets operations are defined in [0,1] ◼ Proper solution to make a weighted input inhibitory is to take fuzzy complement of the excitatory membership value x = 1-x ◼ Input x=(x1 ,..xn ) is extended to x=(x1 ,…,xn ,x1 ,…,xn )
按次数下载不扣除下载券;
注册用户24小时内重复下载只扣除一次;
顺序:VIP每日次数-->可用次数-->下载券;
- 西安电子科技大学:《神经网络与模糊系统 Neural Networks and Fuzzy Systems》课程教学资源(学科综述)人工神经网络 Artificial Neural Networks.ppt
- 西安电子科技大学:《神经网络与模糊系统 Neural Networks and Fuzzy Systems》课程教学资源(学科综述)有关人工智能的故事.doc
- 西安电子科技大学:《神经网络与模糊系统 Neural Networks and Fuzzy Systems》课程教学资源(学科综述)人工智能 AI.ppt
- 烟台理工学院:《机器人操作系统》课程教学资源(PPT课件讲稿)第1章 用于机器人的Ubuntu linux.ppt
- 烟台理工学院:《机器人学》课程教学资源(课件讲稿)第四章 机器人的逆向运动学.pdf
- 烟台理工学院:《机器人学》课程教学资源(课件讲稿)第六章 机器人的动力学.pdf
- 烟台理工学院:《机器人学》课程教学资源(课件讲稿)第五章 速度和静态力.pdf
- 烟台理工学院:《机器人学》课程教学资源(课件讲稿)第二章 空间描述和变换.pdf
- 烟台理工学院:《机器人学》课程教学资源(课件讲稿)第九章 机器人的线性控制.pdf
- 烟台理工学院:《机器人学》课程教学资源(课件讲稿)第三章 机器人的顺向运动学.pdf
- 烟台理工学院:《机器人操作系统》课程教学资源(PPT课件讲稿)第3章 机器人编程的Python基础知识.ppt
- 烟台理工学院:《机器人操作系统》课程教学资源(PPT课件讲稿)第2章 机器人编程的C++基础知识.ppt
- 烟台理工学院:《机器人学》课程教学资源(课件讲稿)第一章 机器人概述(主讲:杨智勇).pdf
- 烟台理工学院:《机器人操作系统》课程教学资源(PPT课件讲稿)绪论、第1章 极限与配合及检测.ppt
- 烟台理工学院:《机器人工程专业导论》理论课教学大纲 Introduction to robot engineering.doc
- 烟台理工学院:《竞赛机器人综合实训》课程教学大纲 Comprehensive training of competition robot.doc
- 烟台理工学院:《机器人组装与调试实习》课程教学大纲 Robot assembly and debugging practice.doc
- 烟台理工学院:《机器人建模与仿真》课程教学大纲 Robot Modeling and Simulation.doc
- 烟台理工学院:《机器人学》课程教学资源(课件讲稿)理论课教学大纲 Robotics.doc
- 烟台理工学院:《工业机器人》课程教学大纲 Industrial robot.doc
- 西安电子科技大学:《神经网络与模糊系统 Neural Networks and Fuzzy Systems》课程教学资源(学科综述)模糊系统与模糊逻辑 Fuzzy Theory.ppt
- 西安电子科技大学:《神经网络与模糊系统 Neural Networks and Fuzzy Systems》课程教学资源(学科综述)进化计算 SOFT COMPUTING Evolutionary Computing.ppt
- 《神经网络与模糊系统》课程教学资源(主题演讲)机器学习研究进展(南京大学:王珏).ppt
- 《神经网络与模糊系统》课程教学资源(主题演讲)选择性集成 Selective Ensemble(南京大学:周志华).ppt
- 西安电子科技大学:《神经网络与模糊系统 Neural Networks and Fuzzy Systems》课程PPT课件讲稿(2003)01.Neuronal Dynamics——Activations and Signals(主讲:高新波).ppt
- 西安电子科技大学:《神经网络与模糊系统 Neural Networks and Fuzzy Systems》课程PPT课件讲稿(2003)02. Neuronal Dynamics——Activation Models(1/2).ppt
- 西安电子科技大学:《神经网络与模糊系统 Neural Networks and Fuzzy Systems》课程PPT课件讲稿(2003)02. Neuronal Dynamics——Activation Models(2/2).ppt
- 西安电子科技大学:《神经网络与模糊系统 Neural Networks and Fuzzy Systems》课程PPT课件讲稿(2003)03. 突触动力学 - 非监督学习 Synaptic Dynamics I——Unsupervised Learning(1/2).ppt
- 西安电子科技大学:《神经网络与模糊系统 Neural Networks and Fuzzy Systems》课程PPT课件讲稿(2003)03. 突触动力学 - 非监督学习 Synaptic Dynamics I——Unsupervised Learning(2/2).ppt
- 西安电子科技大学:《神经网络与模糊系统 Neural Networks and Fuzzy Systems》课程PPT课件讲稿(2003)04. 突触动力学Ⅱ:有监督学习 Synaptic Dynamics II——Supervised Learning(2/2).ppt
- 西安电子科技大学:《神经网络与模糊系统 Neural Networks and Fuzzy Systems》课程PPT课件讲稿(2003)05. 结构和平衡 Architectures and Equilibria.ppt
- 西安电子科技大学:《神经网络与模糊系统 Neural Networks and Fuzzy Systems》课程PPT课件讲稿(2003)06. 模糊与概率 Fuzziness versus Probability.ppt
- 西安电子科技大学:《神经网络与模糊系统 Neural Networks and Fuzzy Systems》课程PPT课件讲稿(2003)07. 模糊联想记忆 Fuzzy Associative Memories(FAM).ppt
- 西安电子科技大学:《神经网络与模糊系统 Neural Networks and Fuzzy Systems》课程PPT课件讲稿(2003)08. 模糊与神经网络的比较——以倒车系统为例 Comparison of Fuzzy and Neural Truck Backer-Upper Control Systems.ppt
- 西安电子科技大学:《神经网络与模糊系统 Neural Networks and Fuzzy Systems》课程PPT课件讲稿(2003)09. 模糊图像变换编码 Fuzzy Image Transform Coding.ppt
- 西安电子科技大学:《神经网络与模糊系统 Neural Networks and Fuzzy Systems》课程PPT课件讲稿(2003)10. 模糊与卡尔曼滤波目标跟踪控制系统的比较 Comparison of Fuzzy and Kalman-Filter Target-Tracking Control Systems.ppt
- 西安电子科技大学:《神经网络与模糊系统 Neural Networks and Fuzzy Systems》课程PPT课件讲稿(2004)Chapter 02 ACTIVATIONS AND SIGNALS.ppt
- 西安电子科技大学:《神经网络与模糊系统 Neural Networks and Fuzzy Systems》课程PPT课件讲稿(2004)Chapter 03 Neuronal Dynamics 2:Activation Models.ppt
- 西安电子科技大学:《神经网络与模糊系统 Neural Networks and Fuzzy Systems》课程PPT课件讲稿(2004)Chapter 04 SYNAPTIC DYNAMICS 1:UNSUPERVISED LEARNING.ppt
- 西安电子科技大学:《神经网络与模糊系统 Neural Networks and Fuzzy Systems》课程PPT课件讲稿(2004)Chapter 05-1 第五章 突触动力学Ⅱ:有监督学习.ppt