《认知心理学》课程教学资源(书籍文献)注意缺陷多动障碍的视觉注意网络研究新发现 Neural Correlates of the Dual-Pathway Model for ADHD in Adolescents
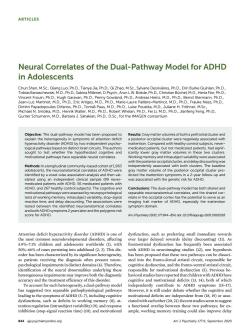
ARTICLES Neural Correlates of the Dual-Pathway Model for ADHD in Adolescents ent psycho nattention.Compared with healthy contol subjects.neve and Methods:Inalongitudinalcommunity-basedcc ndeper The paselin was as. symptom domain. AmJP3chGy2020177844-854dat101176/aoplaio.2020.19020183 Attention deficit hyperactivity disorder (ADHD)is immediate 50%66%of cases persisting into adulthood (2.3).This dis- with ADHD in neuroimaging studies (12),one hypothesis order has been characterized by its significant heterogeneity stinct d ns (There into the fronto-dor striatal circut identification of the neural abnormalities underlying these for motivational dysfunction(5).Previous be heterogeneous impairments may improve both the diagnostic havioral studies have reported that children with ADHDhave accuracy and motivational deficits(13,14).both of whic ependen has leading to the symptoms of ADHD(5-7).including cogitive motivational deficits are independent from(119)orasso dysfunctions,such as deficits in working memory (8),at- ciated witheachother(20,21).Recent studiesseem tosuggest a ru n(stop-signal rea ample Am J Psychiatry 177:9.September 2020
Neural Correlates of the Dual-Pathway Model for ADHD in Adolescents Chun Shen, M.Sc., Qiang Luo, Ph.D., Tianye Jia, Ph.D., Qi Zhao, M.Sc., Sylvane Desrivières, Ph.D., Erin Burke Quinlan, Ph.D., Tobias Banaschewski, M.D., Ph.D., Sabina Millenet, D.Psych., Arun L.W. Bokde, Ph.D., Christian Büchel, M.D., Herta Flor, Ph.D., Vincent Frouin, Ph.D., Hugh Garavan, Ph.D., Penny Gowland, Ph.D., Andreas Heinz, M.D., Ph.D., Bernd Ittermann, Ph.D., Jean-Luc Martinot, M.D., Ph.D., Eric Artiges, M.D., Ph.D., Marie-Laure Paillère-Martinot, M.D., Ph.D., Frauke Nees, Ph.D., Dimitri Papadopoulos Orfanos, Ph.D., Tomás Paus, M.D., Ph.D., Luise Poustka, M.D., Juliane H. Fröhner, M.Sc., Michael N. Smolka, M.D., Henrik Walter, M.D., Ph.D., Robert Whelan, Ph.D., Fei Li, M.D., Ph.D., Jianfeng Feng, Ph.D., Gunter Schumann, M.D., Barbara J. Sahakian, Ph.D., D.Sc., for the IMAGEN consortium Objective: The dual-pathway model has been proposed to explain the heterogeneity in symptoms of attention deficit hyperactivity disorder (ADHD) by two independent psychological pathways based on distinct brain circuits. The authors sought to test whether the hypothesized cognitive and motivational pathways have separable neural correlates. Methods: In a longitudinal community-based cohort of 1,963 adolescents, the neuroanatomical correlates of ADHD were identified by a voxel-wise association analysis and then validated using an independent clinical sample (99 nevermedicated patients with ADHD, 56 medicated patients with ADHD, and 267 healthy control subjects). The cognitive and motivational pathways were assessed by neuropsychological tests of working memory, intrasubject variability, stop-signal reaction time, and delay discounting. The associations were tested between the identified neuroanatomical correlates and both ADHD symptoms 2 years later and the polygenic risk score for ADHD. Results: Gray matter volumes of both a prefrontal cluster and a posterior occipital cluster were negatively associated with inattention. Compared with healthy control subjects, nevermedicated patients, but not medicated patients, had significantly lower gray matter volumes in these two clusters. Working memory and intrasubject variability were associated with the posterior occipital cluster, and delay discounting was independently associated with both clusters. The baseline gray matter volume of the posterior occipital cluster predicted the inattention symptoms in a 2-year follow-up and was associated with the genetic risk for ADHD. Conclusions: The dual-pathway model has both shared and separable neuroanatomical correlates, and the shared correlate in the occipital cortex has the potential to serve as an imaging trait marker of ADHD, especially the inattention symptom domain. Am J Psychiatry 2020; 177:844–854; doi: 10.1176/appi.ajp.2020.19020183 Attention deficit hyperactivity disorder (ADHD) is one of the most common neurodevelopmental disorders, affecting 5.9%–7.1% children and adolescents worldwide (1), with 50%–66% of cases persisting into adulthood (2, 3). This disorder has been characterized by its significant heterogeneity, as patients receiving the diagnosis often present neuropsychological impairments in distinct domains (4). Therefore, identification of the neural abnormalities underlying these heterogeneous impairments may improve both the diagnostic accuracy and the treatment efficiency of this disorder. To account for such heterogeneity, a dual-pathway model has suggested two separable pathophysiological pathways leading to the symptoms of ADHD (5–7), including cognitive dysfunctions, such as deficits in working memory (8), attention regulation (intrasubject variability) (9), and response inhibition (stop-signal reaction time) (10), and motivational dysfunction, such as preferring small immediate rewards over larger delayed rewards (delay discounting) (11). As frontostriatal dysfunction has frequently been associated with ADHD in neuroimaging studies (12), one hypothesis has been proposed that these two pathways can be dissociated into the fronto-dorsal striatal circuit, responsible for cognitive dysfunction, and the fronto-ventral striatal circuit, responsible for motivational dysfunction (5). Previous behavioral studies have reported that children with ADHD have cognitive and motivational deficits (13, 14), both of which independently contribute to ADHD symptoms (15–17). However, it is still under debate whether the cognitive and motivational deficits are independent from (18, 19) or associated with each other (20, 21). Recent studies seem to suggest a functional overlap between these two pathways; for example, working memory training could also improve delay 844 ajp.psychiatryonline.org Am J Psychiatry 177:9, September 2020 ARTICLES
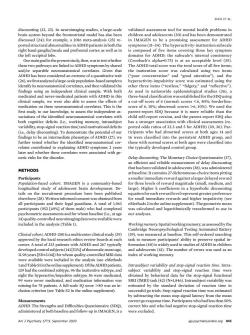
SHEN ET AL discounting (22.23).In neuroi ralidated a t tool for mental health problems in children and adolescents (30)and has been demonstrated discussed (24):for example.a 2016 meta-analysis (25)re in IMAGEN to be a promising assessment for ADHD 山四少 symptoms(31-34).The hyperactivity-inattention subscale com for ve items covering three key symptom Our main goal in the pr sent study then wastotest whether (Cronbach's alpha=0.75)is at an acceptable level (35) these two pathways are linked to ADHD symptoms by shared The ADHD total score was the total score of all five items; tomical co elate Given tha score was calculated using twe nd activity-i other three items restless.”fidgetv.”and "reflective" As used in nationwide epidemiological studies (36),a hree nd class ication was established fo the sDQu medication were 、This is the cu >6,109% first study to our knowledge to assess the independent as parent-report SDQ because it is more reliable than the sociations of the identihed neuroanatomical correlates with hild self-report version,and the parent-report SDQ also gnitive d working ass op-sgin nd 5 fo D)(30.36 mal s s at hoth a 14 and findings to be an intermediate phenotype of ADHD(27).we 6 were classified into the persistent adHd oup and those with normal scores at both ages were classified into elates ted to explair the typically developed control group were Delay discounting.the monetary choice ou aire (37 an efficient and reliable measurement of delay discounting METHODS in ains 27c e items pittin Participant “g3 logiudinalatudy2ofaoescemtbrhndeaopmtape sed cohor e levels of large).Higher k coefficients in a hyperbolic discounting ewhere (28).W con sent wa reward an high participants (9524%of them male)who had completed eat age our analyses. magingdata were available were included in the analysis (Ta able 1) Working memory.Spatial working memory,as as by th Clinical cohort.AdHd-200is a multice er clinical study (29 approved by the local research ethics review boards at each task to measure participants'ability to preserve spatial in- center.A total of 233 patients nith ADHD and 267 typically formation(40)is widely used in studies of ADHD in children ope cts41[53% (he number of available were included in the analysis (see 1and Table S1in theonlinesupplement).Ofthe ADHDpatients, Intrasubject variability and stop-signal reaction time.Intra- 129 had the combined subtyp subject variability and eight the hyperacti e subtype;56 v k42 tiona missing for78 patients A full-scale IO was an in stimated hy the standard deviation of reaction time in clusion criterion (see Table S2 in the online supplement). successful go trials.Stop-signal reaction time was estimated naire (SDO ytgoresponse time.Partieipants nal reasth .September 2020
discounting (22, 23). In neuroimaging studies, a large-scale brain system beyond the frontostriatal model has also been discussed (24); for example, a 2016 meta-analysis (25) reported structural abnormalities in ADHD patients in both the right basal ganglia/insula and prefrontal cortex as well as in the left occipital lobe. Ourmaingoalin the present study, then,was to testwhether these two pathways are linked to ADHD symptoms by shared and/or separable neuroanatomical correlates. Given that ADHD has been considered an extreme of a quantitative trait (26),wefirstanalyzedalarge-scale population-based sample to identify its neuroanatomical correlates, and then validated the findings using an independent clinical sample. With both medicated and never-medicated patients with ADHD in this clinical sample, we were also able to assess the effects of medication on these neuroanatomical correlates. This is the first study, to our knowledge, to assess the independent associations of the identified neuroanatomical correlates with both cognitive deficits (i.e., working memory, intrasubject variability, stop-signal reaction time) and motivational deficits (i.e., delay discounting). To demonstrate the potential of our findings to be an intermediate phenotype of ADHD (27), we further tested whether the identified neuroanatomical correlates contributed to explaining ADHD symptoms 2 years later and whether these correlates were associated with genetic risks for the disorder. METHODS Participants Population-based cohort. IMAGEN is a community-based longitudinal study of adolescent brain development. Details on the recruitment procedure have been published elsewhere (28).Written informed consent was obtained from all participants and their legal guardians. A total of 1,963 participants (952 [49%] of them male) who had completed psychometric assessments and for whom baseline (i.e., at age 14) quality-controlled neuroimaging data were available were included in the analysis (Table 1). Clinical cohort.ADHD-200 is a multicenter clinical study (29) approved by the local research ethics review boards at each center. A total of 233 patients with ADHD and 267 typically developed control subjects (141 [53%] of themmale;mean age, 11.98 years [SD=3.04]) for whom quality-controlled MRI data were available were included in the analysis (see eMethods 1 andTable S1in the online supplement). Of theADHD patients, 129 had the combined subtype, 96 the inattentive subtype, and eight the hyperactive/impulsive subtype; 56 were medicated, 99 were never medicated, and medication information was missing for 78 patients. A full-scale IQ score .80 was an inclusion criterion (see Table S2 in the online supplement). Measurements ADHD. The Strengths and Difficulties Questionnaire (SDQ), administered at both baseline and follow-up in IMAGEN, is a validated assessment tool for mental health problems in children and adolescents (30) and has been demonstrated in IMAGEN to be a promising assessment for ADHD symptoms (31–34). The hyperactivity-inattention subscale is composed of five items covering three key symptom domains for ADHD; the subscale’s internal consistency (Cronbach’s alpha=0.75) is at an acceptable level (35). The ADHD total score was the total score of all five items; the inattention score was calculated using two items (“poor concentration” and “good attention”), and the hyperactivity-impulsivity score was estimated using the other three items (“restless,” “fidgety,” and “reflective”). As used in nationwide epidemiological studies (36), a three-band classification was established for the SDQ using a cut-off score of 6 (normal: scores ,6, 80%; borderline: score of 6, 10%; abnormal: scores .6, 10%). We used the parent-report SDQ because it is more reliable than the child self-report version, and the parent-report SDQ also has a stronger association with clinical assessments (reported odds ratios of 32.3 and 5 for ADHD) (30, 36). Participants who had abnormal scores at both ages 14 and 16 were classified into the persistent ADHD group, and those with normal scores at both ages were classified into the typically developed control group. Delay discounting. The Monetary Choice Questionnaire (37), an efficient and reliable measurement of delay discounting that has been validated in adolescents (38), was administered at baseline. It contains 27 dichotomous-choice items pitting a smaller immediate reward against a larger delayed reward for three levels of reward magnitude (small, medium, and large). Higher k coefficients in a hyperbolic discounting equation for each rewardlevel represent greater preference for small immediate rewards and higher impulsivity (see eMethods 2 in the online supplement). The geometric mean was calculated and logarithmically transformed to use in our analyses. Working memory. Spatial working memory, as assessed by the Cambridge Neuropsychological Testing Automated Battery (39), was measured at baseline. This self-ordered searching task to measure participants’ ability to preserve spatial information (40) is widely used in studies of ADHD in children and adolescents (41). The number of errors was used as an index of working memory. Intrasubject variability and stop-signal reaction time. Intrasubject variability and stop-signal reaction time were obtained by behavioral data for the stop-signal functional MRI (fMRI) task (42) (N=1,846). Intrasubject variability was estimated by the standard deviation of reaction time in successful go trials. Stop-signal reaction time was estimated by subtracting the mean stop-signal latency from the mean correct go response time. Participants who had less than 50% correct hits and who had negative stop-signal reaction time were excluded. Am J Psychiatry 177:9, September 2020 ajp.psychiatryonline.org 845 SHEN ET AL

NEURAL CORRELATES OF THE DUAL-PATHWAY MODEL FOR ADHD IN ADOLESCENTS TABLE1.Characteristics of the study population in the IMAGEN cohort FWE d p th Characteristic or Measure Baseline (N=1.963) 48.5 728 480% Neuropsychological assc analysis.Sep- Mean ere con SD Mean SD Age (years 14.43 0.40 16.47 0.57 (working memory.intrasubject variability stop-signal reaction time,and delay dis- y-mpycre counting)and both ADHDsymptoms and gray Inattention score 192 lling tes ndedn N N terval was given by 5,000 bootstraps.Next,we ADHD included other variables as covar aesfor the aon ana ne varia a sig 941 fter controlling for other variables this as Abnorma 8.5 4.1 sociation is not independent ofother variables Mean SD out is contributed by some common facto between cognitive and motivational Stop-signal reaction time 186.43 61.90 Prospective association analysis.We extended GEN par ider significant associations between the baseline Structural MRI features and ADHD svmptoms 2 years later.In these re- acqui ition and and corresponding baselin Tweighted maiio-preparedaqu ymptoms, sand gray matt was collected using 3-T scanners and preprocessed using the performance (i.e,a significant AR2with p<0.05). s perto etween the pe Genetic Data Genotyping was carried out from blood drawn from total intracranial volume.and site.Sigificance of the results ntormation was col was given by 10,000 random permutations (reported as Beadchin Human Ge p-perm)and wasv edby the comparisons be enwe totaling 506.932 single-nucleotide the R packanp ize with polymorphisms available for establishing the polygenic risk ersistent ADHD goun)(46) score (PRS)for ADHD (see eMethods 4 in the online supplement) lates 3519 Statistical analysis the di (4Z Voxel-wise brain-wide association analysis.A whole-brain summary statistics were downloaded from the Psychiatric Genomics Consortium(http://www.med.unc.edu/pgc/results ay matt The primary analyses are ba on the IMAGEN.Age ore a total intr nial volun (48) and site were considered as covariates.IQ is not recom info(4).Associations of PRS with the neurop -hological mended as a variable to be controlled in cognitive studieso variables were tested by partial correlation analyses while ing for age te, ations with natter v ers were asses 846 aip.psychiatryonline org Am J Psychiatry 177-9.September 2020
Structural MRI The MRI acquisition protocols and quality controls in IMAGEN have been describedin detail (28). A high-resolution T1-weighted magnetization-prepared gradient echo sequence was collected using 3-T scanners and preprocessed using the VBM8 toolbox, as reported previously (43) (see eMethods 3 in the online supplement). Genetic Data Genotyping was carried out from blood drawn from IMAGEN participants (28). Genotype information was collected at 582,982 markers using the Illumina Human Genotyping BeadChip. After quality control, 1,790 cases were included in our sample, totaling 506,932 single-nucleotide polymorphisms available for establishing the polygenic risk score (PRS) for ADHD (see eMethods 4 in the online supplement). Statistical Analysis Voxel-wise brain-wide association analysis. A whole-brain analysis was conducted at the voxel level using the general linear model in SPM12 to identify clusters with gray matter volume associated with the ADHD total score at baseline in IMAGEN. Age, sex, handedness, total intracranial volume, and site were considered as covariates. IQ is not recommended as a variable to be controlled in cognitive studies of neurodevelopmental disorders, since it is often affected by the disorder (44). An uncorrected p threshold of 0.001 at voxel level, with a cluster-level family-wise error (FWE) corrected p threshold of 0.05, was applied to identify significant clusters (45). Neuropsychological association analysis. Separate partial correlation analyses were conducted between neuropsychological measures (working memory, intrasubject variability, stop-signal reaction time, and delay discounting) and both ADHD symptoms and gray matter volumes of the significant clusters, controlling for age, sex, handedness, total intracranial volume, and site. Confidence interval was given by 5,000 bootstraps. Next, we included other variables as covariates for the association analysis of one variable. If a significant association becomes insignificant after controlling for other variables, this association is not independent of other variables but is contributed by some common factor shared between cognitive and motivational deficits. Prospective association analysis. We extended our analysis to ADHD symptoms at age 16 in the IMAGEN participants. Hierarchical multiple regression was applied to identify significant associations between the baseline features and ADHD symptoms 2 years later. In these regression models with covariates and corresponding baseline symptoms, the behavioral variables and gray matter volumes of the significant clusters were entered one by one. A variable was retained in the model ifit significantly elevated the model performance (i.e., a significant DR2 with p,0.05). Analysis of covariance was performed between the persistent ADHD group and the typically developed control group in IMAGEN while controlling for sex, handedness, total intracranial volume, and site. Significance of the results was given by 10,000 random permutations (reported as p-perm) and was validated by the comparisons between wellmatched samples (healthy control subjects were selected by the R package MatchIt to match the sample size with the persistent ADHD group) (46). Polygenic analysis. The latest genome-wide association meta-analysis of 20,183 patients with ADHD and 35,191 control subjects was used as the discovery data set (47); the summary statistics were downloaded from the Psychiatric Genomics Consortium (http://www.med.unc.edu/pgc/resultsand-downloads). The primary analyses are based on the threshold of p,0.50, since it maximally captures phenotypic variance (48), using PRS software (PRSice; http://prsice. info/) (49). Associations of PRS with the neuropsychological variables were tested by partial correlation analyses while controlling for age, sex, and site, and its associations with gray matter volumes of the significant clusters were assessed by TABLE 1. Characteristics of the study population in the IMAGEN cohorta Characteristic or Measure Baseline (N=1,963) 2-Year Follow-Up (N=1,518) N %N% Male 952 48.5% 728 48.0% Mean SD Mean SD Age (years) 14.43 0.40 16.47 0.57 Hyperactivity-inattention subscale on parent SDQ Total score 2.97 2.29 2.39 2.05 Hyperactivity-impulsivity score 0.70 1.05 0.47 0.87 Inattention score 2.27 1.65 1.92 1.57 N %N % ADHD categories by hyperactivity-inattention total scoreb Normal 1,690 86.1 1,394 91.8 Borderline 107 5.5 64 4.1 Abnormal 166 8.5 62 4.1 Mean SD Delay discounting –1.98 0.61 Working memory 19.45 14.00 Intrasubject variabilityc 119.38 30.96 Stop-signal reaction timec 186.43 61.90 a ADHD=attention deficit hyperactivity disorder; SDQ=Strengths and Difficulties Questionnaire. b Scores were categorized as follows: normal: score ,6; borderline: score of 6; abnormal: score .6. c N=1,846. 846 ajp.psychiatryonline.org Am J Psychiatry 177:9, September 2020 NEURAL CORRELATES OF THE DUAL-PATHWAY MODEL FOR ADHD IN ADOLESCENTS

SHEN ET AL TABLE2.Associations of neuropsychological variables with ADHD symptoms and gray matter volumes of the signficant clusters ADHD Symptoms Gray Matter Volume Total Score Inattention Prefrontal Cluster Posterior occipital Cluster Measure 95%C 95%C1 95%C1 95%C 95%C1 Norking memory019*015.0240.09*0.05.0140.21*0.16025-0.04 0.08.0.01 -0.08*-0.12.-0.03 01001 n0y0.16*0.12.0.210.070.03.0120.180.13.0.22-0.04-0.09.0.005-0.07… -0.11-0.02 010*+0.05.0.150.05 oretedfo 0.001,0.10011*0.06.015-0.05-0.09.-0.002-0.05-0.09.-3.3e-4 0120.08,0170.080.03.013012*0.07,0.16-0.04-0.09,0.005-0.05 -010.-0.01 Gray mat ite and total ic p<0.05."p<0.01.p<0.00 additionally controlling for handedness and totalintracranial correlations were not confounded by one another(Table 2) volume. There was no significant correlation between ADHI and discounting rate was positively associated with working ADHD-200 clinical sample.Using a mask of the significant memory errors(r=0.13,df1952,p<0.00L,95%C1-0.08,0.17) ci) variability (r=0.09.df=1835, simificant clusters by comparing patients with control Neuroanatomical Correlates of Inattention in subjects;2)which ADHD patient subtype had the lowest gray a Population-Based Cohort control subjects:and3)whether medication had any remedial =3:3.357 voxels:peak t=-4.29.df-1950.cluster-level effect on the reduced gray matter volumes of the significant WE<0.001)and the posterior occipital cortex (x=-15, ers by group comparisons of ver-medicated patients =91.5,z=112 voxels;peakt 32.df=1 and site. and anterior insula,and the posterior occipital cluster was mainly in the left cuneus and extended to the left calcarine RESULTS tex (Figure 1).Thes not confounded by a的baine Ca地oiag became insignificant after controlling for the inattention memory,intrasubject variability,and delay discounting score but remained significant after controlling for the were positively associated with ADHD symptoms,and the hyperactivity-impulsivity score (prefrontal cluster:r=-0.08, .September 2020
additionally controlling for handedness and total intracranial volume. Validation. We applied the same preprocessing pipeline of structural neuroimaging data as that used in IMAGEN to the ADHD-200 clinical sample. Using a mask of the significant clusters identified in IMAGEN, the gray matter volume of each cluster was extracted for analyses. We tested 1) whether patients with ADHD had lower gray matter volumes of the significant clusters by comparing patients with control subjects; 2) which ADHD patient subtype had the lowest gray matter volumes of the significant clusters by comparing between two ADHD subtypes (hyperactive/impulsive subtype was excluded because of a small sample size of eight) and control subjects; and 3) whethermedication had any remedial effect on the reduced gray matter volumes of the significant clusters by group comparisons of never-medicated patients, medicated patients, and control subjects. All analyses were controlled for age, sex, handedness, total intracranial volume, and site. RESULTS Descriptive Statistics In the IMAGEN cohort at baseline (Table 1), working memory, intrasubject variability, and delay discounting were positively associated with ADHD symptoms, and the correlations were not confounded by one another (Table 2). There was no significant correlation between ADHD symptoms and stop-signal reaction time (p.0.05), and therefore it was not included in further analyses. Delay discounting rate was positively associated with working memory errors (r=0.13, df=1952, p,0.001, 95% CI=0.08, 0.17) and increased intrasubject variability (r=0.09, df=1835, p,0.001, 95% CI=0.04, 0.13). Neuroanatomical Correlates of Inattention in a Population-Based Cohort In IMAGEN at baseline, we found that higher ADHD total score was associated with lower gray matter volumes of two brain clusters in both the prefrontal cortex (x=219.5, y=49.5, z=3; 3,357 voxels; peak t=24.29, df=1950, cluster-level pFWE,0.001) and the posterior occipital cortex (x=21.5, y=91.5, z=15; 1,295 voxels; peak t=24.32, df=1950, cluster-level pFWE=0.025). The prefrontal cluster covered the left ventromedial prefrontal cortex, dorsal anterior cingulate cortex, and anterior insula, and the posterior occipital cluster was mainly in the left cuneus and extended to the left calcarine cortex (Figure 1). These associations were not confounded by either site (see Figure S1 in the online supplement) or IQ (see eResults 2 in the online supplement). These associations became insignificant after controlling for the inattention score but remained significant after controlling for the hyperactivity-impulsivity score (prefrontal cluster: r=20.08, TABLE 2. Associations of neuropsychological variables with ADHD symptoms and gray matter volumes of the significant clustersa ADHD Symptoms Gray Matter Volume Total Score HyperactivityImpulsivity Inattention Prefrontal Cluster Posterior occipital Cluster Measure r 95% CI r 95% CI r 95% CI r 95% CI r 95% CI Working memoryb 0.19*** 0.15, 0.24 0.09*** 0.05, 0.14 0.21*** 0.16, 0.25 20.04 20.08, 0.01 20.08*** 20.12, 20.03 Delay discountingb 0.13*** 0.08, 0.17 0.06** 0.02, 0.11 0.14*** 0.09.0.18 20.07** 20.11, 20.02 20.06* 20.10, 20.01 Intrasubject variabilityc 0.14*** 0.10, 0.19 0.09*** 0.04, 0.14 0.14*** 0.10, 0.19 20.05* 20.10, 20.01 20.06** 20.11, 20.01 Working memory corrected for delay discounting and intrasubject variability 0.16*** 0.12, 0.21 0.07** 0.03, 0.12 0.18*** 0.13, 0.22 20.04 20.09, 0.005 20.07** 20.11, 20.02 Delay discounting corrected for working memory and intrasubject variability 0.10*** 0.05, 0.15 0.05* 0.001, 0.10 0.11*** 0.06, 0.15 20.05* 20.09, 20.002 20.05* 20.09, 23.3e–4 Intrasubject variability corrected for working memory and delay discounting 0.12*** 0.08, 0.17 0.08** 0.03, 0.13 0.12*** 0.07, 0.16 20.04 20.09, 0.005 20.05* 20.10, 20.01 a ADHD symptoms were adjusted for age, sex, and site. Gray matter volume was adjusted for age, sex, handedness, site, and total intracranial volume. Confidence intervals were estimated by bootstrap 5,000 times. b N=1,963. c N=1,846. *p,0.05. **p,0.01. ***p,0.001. Am J Psychiatry 177:9, September 2020 ajp.psychiatryonline.org 847 SHEN ET AL

NEURAL CORRELATES OF THE DUAL-PATHWAY MODEL FOR ADHD IN ADOLESCENTS FIGURE1 Significa ain clusters asso ciated with ADHD total score in a population-based cohort had reduced ra matter vol umes of both the prefrontal as compared with the [SD=103]comparedwith6.23 mL[SD119:F=6.37,df ter (2.06 mL SD=0.351 com. paredwith2.19mL[SD=0.28]; ng the onata9e14Nl26i000 nificant results with even of005 larger effect sizes were found ster 9.5 95.2 29. 1950 4.32 0.001 asing matchcd-group 0251 No clust supplement). f=1949,p=0.002,95%CI=-0.03,-0.1 ate Phenotypes With mical Correlates of Inattention Selectively In the IMAGEN sample,we found that higher PRS for ADHD was associated with higher ADHD total score at baseline Variability,or Delay Discounting In IMAGEN at baseline,we found that a larger number of working memory errors was CL-02007.95%C002L0109 d0.6 matter volume of the posterior occipital cluster only (r=-0.07,df=1831,p=0.005)(Table 2).Similar to working .06,df=1777,p=0.009,95%C1=-0106,-0.015) memory,increased intrasubject variability was associate lower gray matte Validation using an adHd clinical cohort In the ADHD-200 sample,we confirmed that patients had delay discounting (r=0.05.df=1831.p=0.027(Table 2). ower gray matter volumes in both the prefrontal (3.86 mL SD-167 compared wi 40m king memory ith 1.28 ml SD0.291r928.dfL.49Lp-0.002:,0.019 (Figure2B. ter:r=-0.05,df=1831,p=0.049)(Table2). ese volumetric reductions were nonsignificant in patients ective Associations With Inattention 2 Ye sLate onding ADHD sympto clister:F=1292 dfl 354 00020035 age 14,working memory and delay discountingat age 14were xccipital cluster:F=729,df=1 354.p=0.007:p0.20) selectively associated with inattention (t=2.35, d1505 (Figure 2C-D). and hyperactivity-impulsivity(24. y-204d404.AR2-0002 p=0.042)and gray matter volume of the posterior oc cipital cluster (t=-3.55,df=1404,AR2=0.005,P<0.001) olumes of both clusters,the medicated patients(N56)had associate with inattention 2 years late lumes,and the never-medicated gray matter volumes 848 aip psychiatryonline.oro AmJPsychiatry 177:9.September 2020
df=1949, p,0.001, 95% CI=20.03, 20.13; posterior occipital cluster: r=20.07, df=1949, p=0.002, 95% CI=20.03, 20.11). Neuroanatomical Correlates of Inattention Selectively Associated With Working Memory, Intrasubject Variability, or Delay Discounting In IMAGEN at baseline, we found that a larger number of working memory errors was associated with lower gray matter volume of the posterior occipital cluster even after controlling for intrasubject variability and delay discounting (r=20.07, df=1831, p=0.005) (Table 2). Similar to working memory, increased intrasubject variability was associated with lower gray matter volume of the posterior occipital cluster even after controlling for working memory and delay discounting (r=20.05, df=1831, p=0.027) (Table 2). Greater delay discounting rate was associated with lower graymatter volumes of both clusters even after controlling for working memory and intrasubject variability (prefrontal cluster: r=20.05, df=1831, p=0.042; posterior occipital cluster: r=20.05, df=1831, p=0.049) (Table 2). Prospective Associations With Inattention 2 Years Later After controlling for the corresponding ADHD symptom at age 14, working memory and delay discounting at age 14 were selectively associated with inattention (t=2.35, df=1505, p=0.019) and hyperactivity-impulsivity (t=2.24, df=1505, p=0.025) at age 16. In the multivariate regression model, we found that bothworkingmemory (t=2.04, df=1404,DR2 =0.002, p=0.042) and gray matter volume of the posterior occipital cluster (t=23.55, df=1404, DR2 =0.005, p,0.001) at age 14 were associated with inattention 2 years later (Table 3). Adolescents with persistent ADHD symptoms (N=29) had reduced gray matter volumes of both the prefrontal cluster as compared with the typically developed control subjects (N=1,278; 5.63 mL [SD=1.03] compared with 6.23 mL [SD=1.19]; F=6.37, df=1, 1295, p-perm=0.012; partial eta-squared [h2 p]=0.005) and the posterior occipital cluster (2.06 mL SD=0.35] compared with 2.19mL [SD=0.28]; F=5.12,df=1,1295,p-perm=0.022; h2 p=0.004; see Figure S2 in the online supplement). Significant results with even larger effect sizes were found using matched-group comparisons (29 compared with 58; see eResults 3 in the online supplement). Associations of Neuropsychological and Neuroanatomical Intermediate Phenotypes With Polygenic Risk for ADHD In the IMAGEN sample, we found that higher PRS for ADHD was associated with higher ADHD total score at baseline (r=0.14, df=1779, p,0.001, 95% CI=0.097, 0.188), more working memory errors ( r=0.07, df=1779, p=0.002, 95% CI=0.026, 0.121), greater delay discounting rate (r=0.06, df=1779, p=0.007, 95% CI=0.021, 0.109), and lower gray matter volume of the posterior occipital cluster only (r=20.06, df=1777, p=0.009, 95% CI=20.106, 20.015). Validation Using an ADHD Clinical Cohort In the ADHD-200 sample, we confirmed that patients had lower gray matter volumes in both the prefrontal (3.86 mL [SD=1.67] compared with 4.40 mL [SD=1.56]; F=12.18, df=1, 491, p,0.001; h2 p=0.024) (Figure 2A) and the posterior occipital clusters (1.21 mL [SD=0.30] compared with 1.28 mL [SD=0.29]; F=9.28, df=1, 491, p=0.002;h2 p=0.019) (Figure 2B). These volumetric reductions were nonsignificant in patients with the combined subtype (N=129) and significant only in patients with the inattentive subtype (N=96; prefrontal cluster: F=12.92, df=1, 354, p,0.001; h2 p=0.035; posterior occipital cluster: F=7.29, df=1, 354, p=0.007; h2 p=0.20) (Figure 2C–D). Medication Effects In the ADHD-200 sample, we found that the typically developed control subjects (N=267) had the highest gray matter volumes of both clusters, the medicated patients (N=56) had intermediate gray matter volumes, and the never-medicated patients (N=99) had the lowest gray matter volumes. FIGURE 1. Significant brain clusters associated with ADHD total score in a population-based cohorta a The results were given by a voxel-wise whole brain analysis using the IMAGEN cohort at age 14 (N=1,963). Age, sex, handedness, total intracranial volume, and site were used as covariates. An uncorrected p threshold of 0.001 at voxel level, with a cluster-level family-wise error (FWE) corrected p threshold of 0.05, was applied to identify significant clusters. Two clusters were found to be negatively associated with the ADHD total score: the prefrontal cluster (x=219.5, y=49.5, z=3; 3,357 voxels; peak t=24.29, df=1950, cluster-level pFWE,0.001) and the posterior occipital cortex (x=21.5, y=91.5, z=15; 1,295 voxels; peak t=24.32, df=1950, cluster-level pFWE=0.025). No clusters were found to be positively associated with the ADHD total score. 848 ajp.psychiatryonline.org Am J Psychiatry 177:9, September 2020 NEURAL CORRELATES OF THE DUAL-PATHWAY MODEL FOR ADHD IN ADOLESCENTS
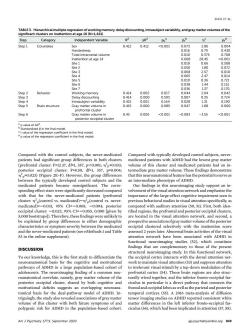
SHEN ET AL Step Category Independent Variable R2 △R2 B Step1 Covariates 0.412 0.412 <0.001 ioinaciamnulvoume ntion at age 14 Behavior Brain structure Gray matter volume in Step6 0.42 0.005 <0001 -0.083 -355 <0.001 posterior occipital cluster pvalue fR B in the final mode pvauecftheegresioncoeheentinhefmlmod Compared with the control subiects.the neve medicated Compared with typically developed control subiects.never patients had significant group differences in both clusters medicated patients with ADHD had the lowest gray matter (prefrontal cluster:F=12.37,df=1,357,p<0.001;np=0.033; volume of this cluster and medicated patients had an in- F=8.50, 357 and the medicated patients became nonsignificant.The corre. Our findings in this neuroimaging study support an in- sponding effect sizes were significantly decreased compared volvement of the visual attention network and emphasize the with that for the -0.031,95%CI=-0.068 0.004 d with audito attention (50.51.Fi occipital cluster:-0.02,%CI=-0.055,0.000 given by fieugoAhcpreOntaandposeriorocptaPdeies 5,000 bootstrapsD).Therefore,these findings wereunlikely to are located in the visual attention network,and second,a iation of gray m un neofthe posterio S3 in the online supplement). attention network have been associated with ADHD in unctionl neuroimaging studies (52),which constitute DISCUSSION nat are con se of the presen To our knowledge,this is the first study to differentiate the inital cortey inter with the do sal attention ne neuroanatomical basis for the cognitive and motivational work to maintain visual attention(53)and suppress attention to irrelevant visual stimuli by a top-down modulation of the (54).T n regions are also struc ciculus in particular is a direct pathway that connects the motivational deficits suggests an overlapping neuroana- frontal and occipital lobesas wellas the parietal and posterior tomical basis for the dual-pathway model of ADHD.In emporal cortices (55).A 2016 meta-analysis of diffusion the study also rev sociations of gray matte ensor imaging studies on ADH eported cons nt whi Am JPsychiatry 1779.September 2020
Compared with the control subjects, the never-medicated patients had significant group differences in both clusters (prefrontal cluster: F=12.37, df=1, 357, p,0.001; h2 p=0.033; posterior occipital cluster: F=8.50, df=1, 357, p=0.004; h2 p=0.023) (Figure 2E–F). However, the group differences between the typically developed control subjects and the medicated patients became nonsignificant. The corresponding effect sizes were significantly decreased compared with that for the never-medicated patients (prefrontal cluster: h2 p(control vs. medicated)2h2 p(control vs. nevermedicated)=20.031, 95% CI=20.068, 20.004; posterior occipital cluster: 20.022, 95% CI=20.055, 0.000 [given by 5,000 bootstraps]). Therefore, these findings were unlikely to be explained by group differences in either demographic characteristics or symptom severity between the medicated and the never-medicated patients (see eMethods 1 and Table S3 in the online supplement). DISCUSSION To our knowledge, this is the first study to differentiate the neuroanatomical basis for the cognitive and motivational pathways of ADHD in a large population-based cohort of adolescents. The neuroimaging finding of a common neuroanatomical correlate, namely, gray matter volume of the posterior occipital cluster, shared by both cognitive and motivational deficits suggests an overlapping neuroanatomical basis for the dual-pathway model of ADHD. Intriguingly, the study also revealed associations of gray matter volume of this cluster with both future symptoms of and polygenic risk for ADHD in the population-based cohort. Compared with typically developed control subjects, nevermedicated patients with ADHD had the lowest gray matter volume of this cluster and medicated patients had an intermediate gray matter volume. These findings demonstrate that this neuroanatomical feature has the potential to serve as an intermediate phenotype of ADHD. Our findings in this neuroimaging study support an involvement of the visual attention network and emphasize the importance of the large-effect cognitive impairment seen in previous behavioral studies in visual attention specifically, as compared with auditory attention (50, 51). First, both identified regions, the prefrontal and posterior occipital clusters, are located in the visual attention network, and second, a prospective association of graymatter volume of the posterior occipital clustered selectively with the inattention score assessed 2 years later. Abnormal brain activities of the visual attention network have been associated with ADHD in functional neuroimaging studies (52), which constitute findings that are complementary to those of the present structural neuroimaging study. In this functional network, the occipital cortex interacts with the dorsal attention network to maintain visual attention (53) and suppress attention to irrelevant visual stimuli by a top-down modulation of the prefrontal cortex (54). These brain regions are also structurally wired together, and the inferior fronto-occipital fasciculus in particular is a direct pathway that connects the frontal and occipital lobes as well as the parietal and posterior temporal cortices (55). A 2016 meta-analysis of diffusion tensor imaging studies on ADHD reported consistent white matter differences in the left inferior fronto-occipital fasciculus (56), which had been implicated in attention (57, 58). TABLE 3. Hierarchical multiple regression of working memory, delay discounting, intrasubject variability, and gray matter volumes of the significant clusters on inattention at age 16 (N=1,421) Step Category Independent Variable R2 DR2 pe a bf b tf c pf d Step 1 Covariates Sex 0.412 0.412 ,0.001 0.073 2.86 0.004 Handedness 0.016 0.79 0.430 Total intracranial volume 0.010 0.375 0.708 Inattention at age 14 0.608 28.45 ,0.001 Site 1 0.018 0.66 0.508 Site 2 0.050 1.80 0.072 Site 3 0.068 2.57 0.010 Site 4 0.065 2.47 0.014 Site 5 0.010 0.36 0.721 Site 6 0.038 1.44 0.151 Site 7 0.036 1.37 0.170 Step 2 Behavior Working memory 0.414 0.002 0.017 0.044 2.04 0.042 Step 3 Delay discounting 0.414 0.000 0.593 0.007 0.35 0.724 Step 4 Intrasubject variability 0.415 0.001 0.164 0.028 1.31 0.190 Step 5 Brain structure Gray matter volume in prefrontal cluster 0.415 0.000 0.685 0.047 1.88 0.060 Step 6 Gray matter volume in posterior occipital cluster 0.42 0.005 ,0.001 –0.083 –3.55 ,0.001 a p value of DR2 . b Standardized b in the final model. c t value of the regression coefficient in the final model. d p value of the regression coefficient in the final model. Am J Psychiatry 177:9, September 2020 ajp.psychiatryonline.org 849 SHEN ET AL

NEURAL CORRELATES OF THE DUAL-PATHWAY MODEL FOR ADHD IN ADOLESCENTS FIGURE 2.Group comparisons of gray matter volumes of the identified clusters in a clinical cohort 0.0 ADHD grou anvTedgoup ADHD group 03 ADHD. ubtype aro 。 -10 ults were based on the ADHD-200 cohort They-axis inall panels is the residualof gr sters reor s are the in gray aeotneeofhe oped group (N-0o)pane ADHD group (N-99 850 ajp.psychiatryonlineorg Am JPychiatry 177.9.September 2020
FIGURE 2. Group comparisons of gray matter volumes of the identified clusters in a clinical cohorta A B C D E F Typically developed group Typically developed group ADHD group –4 –2 0 2 4 ** Typically developed group ADHD group ** –4 –2 0 2 4 –4 –2 0 2 4 *** –1.0 –0.5 0.0 0.5 1.0 ** Residual of Prefrontal Gray Matter Volume Residual of Posterior Occipital Gray Matter Volume Residual of Prefrontal Gray Matter Volume Residual of Posterior Occipital Gray Matter Volume Residual of Prefrontal Gray Matter Volume Residual of Posterior Occipital Gray Matter Volume ADHD, combined subtype group ADHD, inattentive subtype group Typically developed group Typically developed group Typically developed group ADHD, combined subtype group ADHD, inattentive subtype group Medicated ADHD group Never-medicated ADHD group Medicated ADHD group Never-medicated ADHD group *** ** –1.0 –0.5 0.0 0.5 1.0 –1.0 –0.5 0.0 0.5 1.0 a The results were based on the ADHD-200 cohort. The y-axis in all panels is the residual of graymatter volumes of the identified clusters regressed on age, sex, handedness, total intracranial volume, and site. The bottom and top of the boxes are the minimum and maximum, and the band near the middle of the box is the median. Significant group comparisons are indicated with asterisks. Panel A depicts the difference in gray matter volume of the prefrontal cluster between typically developed control subjects (N=267) and ADHD patients (N=233). Panel B depicts the difference in gray matter volume of the posterior occipital cluster between the typically developed group (N=267) and ADHD patients (N=233). Panel C depicts the difference in gray matter volume of the prefrontal cluster among the typically developed group (N=267), the ADHD group with the combined subtype (N=129), and the ADHD group with the inattentive subtype (N=96). Panel D depicts the difference in gray matter volume of the posterior occipital cluster among the typically developed group (N=267), the ADHD group with the combined subtype (N=129), and the ADHD group with the inattentive subtype (N=96). Panel E depicts the difference in graymatter volume of the prefrontal cluster among the typically developed group(N=267), themedicated ADHD group (N556), and the never-medicated ADHD group (N599). Panel F depicts the difference in gray matter volume of the posterior occipital cluster among the typically developed group (N5267), the medicated ADHD group (N556), and the never-medicated ADHD group (N599). **p,0.01. ***p,0.001. 850 ajp.psychiatryonline.org Am J Psychiatry 177:9, September 2020 NEURAL CORRELATES OF THE DUAL-PATHWAY MODEL FOR ADHD IN ADOLESCENTS
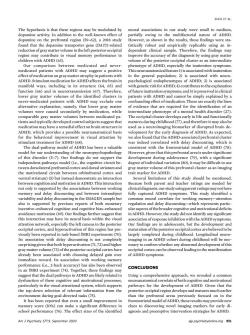
SHEN ET AL The hypothesis is that thes e small to mediun dopamine on the prefrontal region(59-62).a 2018 study However,as shown in the results,these findings were sta- stically gray ma ngs ma olume of the posterior occipital cluster as an intermediate Our comparison between medicated and never medica phenotype of ADHD,especially the inattention symptoms. ted patients with AD may sugge ati pos ure associated ADID Stimulant me s the brain in ical manifold ways,including in its structure (64.65)and function(66)and in neurotransmission(67).Therefore, of future inattention symptoms;and5)is preserved inclinical lower gray mae f th DHD and cannot b e simply explain Dy a nla tha ed fo ntermediate phenotyne of a mental health disorder (27) matter volumes bet n medicated pa develops early in life and functionally and typ lly dev ol subj suggest th 77, nd th .on ADHD.which of ADHD.As ement in visual attention by we also found that the ADHD-associated prefrontal cluster stimulant treatment for ADHD (68). was indeed correlated with delay dis ounting,which is ual-path HD has been ava nsisten vith the A 078 11 ith independent-pathways model (ie.the cognitive circuit be degree ofindividual variation (80).itma he m ntal cortex and orbito m⑤bti this tudy should be and motivation ADHD.Thisin m e and not only is supported by the associations between working clinical diagnosis.our ing and between intrasubjec fully captured ADHD symptoms. This study identified a g in the IM ample bu e ate for wor attennor supp hanced oitive hias-enhanced mahenoioc2,&rminStrie in ADHD.However,the study did not identify any significant this interaction atter netwo eciall in th are in a but the de ital and reported in task-based NR ments (70) gely completed during Its association with delay discounting is not com letel imaging in an ADHD cohort during childhood will be nec surprising given that both hyperactivation(71,72)and higher essary to confirm whether any abnormal development of this gray matter ave cortex can be observed leading to the manifestation immediate reward its association with workine performance (i.e.,2-back a ccuracy)has also been observed in an fMRI CONCLUSIONS at th ual path ays in A ted t which athways for the dev ment of ADHD Given that the sterior occipital region develops and matures much earlie envir rected tasks(5) bee even a sm se re sizes of the identified gosis and p ntion strategies for ADHD. .September 2020
The hypothesis is that these regions may be modulated by dopamine activity. In addition to the well-known effect of dopamine on the prefrontal region (59–62), a 2018 study found that the dopamine transporter gene (DAT1)-related reduction of gray matter volume in the left posterior occipital region may contribute to visual memory performance in children with ADHD (63). Our comparison between medicated and nevermedicated patients with ADHD may suggest a positive effect of medication on gray matter atrophy in patients with ADHD. Stimulant medication for ADHD affects the brain in manifold ways, including in its structure (64, 65) and function (66) and in neurotransmission (67). Therefore, lower gray matter volumes of the identified clusters in never-medicated patients with ADHD may exclude one alternative explanation, namely, that lower gray matter volumes were caused secondarily by medication, while comparable gray matter volumes between medicated patients and typically developed control subjects suggest that medication may have a remedial effect on brain structure in ADHD, which provides a possible neuroanatomical basis for the behavioral improvement in visual attention by stimulant treatment for ADHD (68). The dual-pathway model of ADHD has been a valuable model for our understanding of the neuropsychopathology of this disorder (5–7). Our findings do not support the independent-pathways model (i.e., the cognitive circuit between dorsolateral prefrontal cortex and dorsal striatum and the motivational circuit between orbitofrontal cortex and ventral striatum) (5) but instead demonstrate an interaction between cognition and motivation in ADHD. This interaction not only is supported by the associations between working memory and delay discounting and between intrasubject variability and delay discounting in the IMAGEN sample but also is supported by previous reports of both monetary incentive–enhanced cognition and cognitive bias–enhanced avoidance motivation (69). Our findings further suggest that this interaction may have its neural basis within the visual attention network, especially the left cuneus in the posterior occipital cortex, and hyperactivation of this region has previously been reported in task-based fMRI experiments (70). Its association with delay discounting is not completely surprising given that both hyperactivation (71, 72) and higher gray matter volume (73) of the posterior occipital cortex have already been associated with choosing delayed gain over immediate reward. Its association with working memory performance (i.e., 2-back accuracy) has also been observed in an fMRI experiment (74). Together, these findings may suggest that the dual pathways in ADHD are likely related to dysfunction of these cognitive and motivational processes, particularly in the visual attentional system, which supports the top-down selection of relevant information from the environment during goal-directed tasks (75). It has been reported that even a small improvement in memory score (10%) can make a significant difference in school performance (76). The effect sizes of the identified neural associations in our study were small to medium, partially owing to the multifactorial nature of ADHD. However, as shown in the results, these findings were statistically robust and empirically replicable using an independent clinical sample. Therefore, the findings may improve the accuracy of the diagnosis by using gray matter volume of the posterior occipital cluster as an intermediate phenotype of ADHD, especially the inattention symptoms. This neuroanatomical feature 1)is associated withinattention in the general population; 2) is associated with neuropsychological endophenotypes of ADHD; 3) is associated with genetic risk for ADHD; 4) contributes to the explanation of future inattention symptoms; and 5) is preserved in clinical patients with ADHD and cannot be simply explained by a confounding effect of medication. These are exactly the lines of evidence that are required for the identification of an intermediate phenotype of a mental health disorder (27). The occipital cluster develops early in life and functionally matures during childhood (77), and therefore it may also be used as a neuroimaging biomarker of disrupted brain development for the early diagnosis of ADHD. As expected, we also found that the ADHD-associated prefrontal cluster was indeed correlated with delay discounting, which is consistent with the frontostriatal model of ADHD (78). However, given that this prefrontal area is under significant development during adolescence (79), with a significant degree of individual variation (80), it may be difficult to use gray matter volume of this prefrontal cluster as an imaging trait marker for ADHD. Several limitations of this study should be mentioned. Because both parent and teacher ratings are needed for clinical diagnosis, our study using parent ratings may not have fully captured ADHD symptoms. This study identified a common neural correlate for working memory—attention regulation and delay discounting—which represents particular aspects of the broader cognitive and motivational deficits in ADHD. However, the study did not identify any significant association of response inhibition with the ADHD symptoms. Our findings are in adolescents, but the development and maturation of the posterior occipital cortex are believed to be largely completed during childhood. Longitudinal neuroimaging in an ADHD cohort during childhood will be necessary to confirm whether any abnormal development of this occipital cortex can be observed leading to the manifestation of ADHD symptoms. CONCLUSIONS Using a comprehensive approach, we revealed a common neuroanatomical correlate of both cognitive and motivational pathways for the development of ADHD. Given that the posterior occipital region develops and matures much earlier than the prefrontal areas previously focused on in the frontostriatal model of ADHD, these results may provide new clues to discovering novel imaging markers for early diagnosis and preemptive intervention strategies for ADHD. Am J Psychiatry 177:9, September 2020 ajp.psychiatryonline.org 851 SHEN ET AL
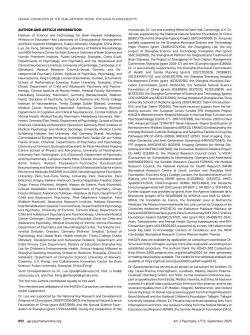
NEURAL CORRELATES OF THE DUAL-PATHWAY MODEL FOR ADHD IN ADOLESCENTS UTHOR AND ARTICLE INFORMATION h nts c 11 NIH ng to a a (L 0o/) K.(Feng):ar ve in on er for Rtair served as an ion.Eli )Prof.Li (feilie The first two authors contributed equally to this work or ph nbers and affiliations of the IMAGEN Consortium are listed in the d by the N arch and De 201 Hoso nd.D 17 dation of Shanghai (grant 17ZR1444400).During the preparation of this Education and Res MB月.the Ge undation (DFG 852 aip.psychiatryonline.org Am J Psychiatry 177-9.September 2020
AUTHOR AND ARTICLE INFORMATION Institute of Science and Technology for Brain-Inspired Intelligence, Ministry of Education–Key Laboratory of Computational Neuroscience and Brain-Inspired Intelligence, Fudan University, Shanghai, China (Shen, Luo, Jia, Feng, Sahakian); State Key Laboratory of Medical Neurobiology and MOE Frontiers Center for Brain Science, Institutes of Brain Science and Human Phenome Institute, Fudan University, Shanghai, China (Luo); Departments of Psychology and Psychiatry and the Behavioural and Clinical Neuroscience Institute, University of Cambridge, Cambridge, U.K. (Sahakian); Medical Research Council–Social, Genetic, and Developmental Psychiatry Centre, Institute of Psychiatry, Psychology, and Neuroscience, King’s College London (Desrivières, Quinlan, Schumann); School of Mathematical Sciences, Fudan University, Shanghai, China (Zhao); Department of Child and Adolescent Psychiatry and Psychotherapy, Central Institute of Mental Health, Medical Faculty Mannheim, Heidelberg University, Mannheim, Germany (Banaschewski, Millenet, Nees); Discipline of Psychiatry, School of Medicine and Trinity College Institute of Neuroscience, Trinity College Dublin (Bokde); University Medical Center Hamburg-Eppendorf, Hamburg, Germany (Büchel); Department of Cognitive and Clinical Neuroscience, Central Institute of Mental Health, Medical Faculty Mannheim, Heidelberg University, Mannheim, Germany (Flor, Nees); Department of Psychology, School of Social Sciences, University of Mannheim, Mannheim, Germany (Flor); Institute of Medical Psychology and Medical Sociology, University Medical Center Schleswig Holstein, Kiel University, Kiel, Germany (Nees); NeuroSpin, Commissariat à l’Énergie Atomique, Université Paris–Saclay, Gif-sur-Yvette, France (Frouin, Orfanos); Departments of Psychiatry and Psychology, University of Vermont, Burlington (Garavan); Sir Peter Mansfield Imaging Centre School of Physics and Astronomy, University of Nottingham, University Park, Nottingham, U.K. (Gowland); Department of Psychiatry and Psychotherapy, Campus Charité Mitte, Charité, Universitätsmedizin Berlin (Heinz, Walter); Physikalisch-Technische Bundesanstalt Braunschweig and Berlin (Ittermann); Institut National de la Santé et de la Recherche Médicale (INSERM) Unit 1000, Neuroimaging and Psychiatry, University Paris Sud–Paris Saclay, University Paris Descartes, Paris (Martinot, Artiges, Paillère-Martinot); Service Hospitalier Frédéric Joliot, Orsay, France (Martinot, Artiges); Maison de Solenn, Paris (Martinot); Groupe Hospitalier Nord Essonne, Department of Psychiatry, Orsay, France (Artiges); Assistance Publique–Hôpitaux de Paris, Department of Child and Adolescent Psychiatry, Pitié-Salpêtrière Hospital, Paris (Paillère-Martinot); Bloorview Research Institute, Holland Bloorview Kids Rehabilitation Hospital, Toronto (Paus); Departments of Psychology and Psychiatry, University of Toronto, Toronto (Paus); Department of Child and Adolescent Psychiatry and Psychotherapy, University Medical Center Göttingen, Göttingen, Germany (Poustka); Clinic for Child and Adolescent Psychiatry, Medical University of Vienna, Vienna (Poustka); Department of Psychiatry and Neuroimaging Center, Technische Universität Dresden, Dresden, Germany (Fröhner, Smolka); School of Psychology and Global Brain Health Institute, Trinity College Dublin (Whelan); Developmental and Behavioral Pediatric Department and Child Primary Care Department, Ministry of Education–Shanghai Key Lab for Children’s Environmental Health, Xinhua Hospital Affiliated to Shanghai Jiaotong University School of Medicine, Shanghai, China (Li, Sahakian); Department of Computer Science, University of Warwick, Coventry, U.K. (Feng); and Collaborative Innovation Center for Brain Science, Fudan University, Shanghai, China (Feng). Send correspondence to Dr. Luo (qluo@fudan.edu.cn), Prof. Li (feili@ shsmu.edu.cn), and Prof. Feng (jianfeng64@gmail.com). The first two authors contributed equally to this work The members and affiliations of the IMAGEN Consortium are listed in the online supplement. Dr. Luo was supported by the National Key Research and Development Program of China (grant 2018YFC0910503), the National Natural Science Foundation of China (grant 81873909), and the Natural Science Foundation of Shanghai (grant 17ZR1444400). During the preparation of this manuscript, Dr. Luo was a visiting fellow at Clare Hall, Cambridge, U.K. Dr. Jia was supported by the National Natural Science Foundation of China (81801773) and the Shanghai Pujiang Project (18PJ1400900). Dr. Feng was partially supported by the Shanghai Municipal Science and Technology Major Project (grant 2018SHZDZX01), the Zhangjiang Lab, the key project of Shanghai Science and Technology Innovation Plan (grant 16JC1420402), the Shanghai AI Platform for Diagnosis and Treatment of Brain Diseases, the Project of Zhangjiang Hi-Tech District Management Committee, Shanghai (grant 2016-17), and the 111 project (grant B18015). Dr. Li was partially supported by the Shanghai Municipal Commission of Health and Family Planning (grants 2017ZZ02026, 2018BR33, 2017EKHWYX-02, and GDEK201709), the Shanghai Shenkang Hospital Development Center (grant 16CR2025B), the Shanghai Municipal Education Commission (grant 20152234), the National Natural Science Foundation of China (grants 81930095, 81571031, 81761128035, and 81703249), the Shanghai Committee of Science and Technology (grants 17XD1403200 and 18DZ2313505), Xinhua Hospital of Shanghai Jiao Tong University School of Medicine (grants 2018YJRC03, Talent Introduction- 014, and Top Talent-201603). This work received support from the following sources: the European Union–funded FP6 Integrated Project IMAGEN (Reinforcement-Related Behavior in Normal Brain Function and Psychopathology) (LSHM-CT- 2007-037286), the Horizon 2020 funded ERC Advanced Grant “STRATIFY” (Brain Network–Based Stratification of Reinforcement-Related Disorders) (695313), ERANID (Understanding the Interplay Between Cultural, Biological, and Subjective Factors in Drug Use Pathways) (PR-ST-0416-10004), BRIDGET (JPND: Brain Imaging, Cognition Dementia, and Next Generation Genomics) (MR/N027558/1), the FP7 projects IMAGEMEND (602450; Imaging Genetics for Mental Disorders) and MATRICS (603016), the Innovative Medicine Initiative Project EU-AIMS (115300-2), the Medical Research Council Grant “c-VEDA” (Consortium on Vulnerability to Externalizing Disorders and Addictions) (MR/N000390/1), the Swedish Research Council FORMAS, the Medical Research Council, the National Institute for Health Research (NIHR) Biomedical Research Centre at South London and Maudsley NHS Foundation Trust and King’s College London, the Bundesministerium für Bildung und Forschung (BMBF grants 01GS08152; 01EV0711; eMED SysAlc01ZX1311A; Forschungsnetz AERIAL 01EE1406A), the Deutsche Forschungsgemeinschaft (DFG grants SM 80/7-1, SM 80/7-2, SFB 940/1). Further support was provided by grants from the Agence Nationale de la Recherche (project AF12-NEUR0008-01-WM2NA, and ANR-12-SAMA- 0004), the Fondation de France, the Fondation pour la Recherche Médicale, the Mission Interministérielle de Lutte contre les Drogues et les Conduites Addictives (MILDECA), the Assistance Publique Hôpitaux de Paris and INSERM (interface grant), Paris Sud University IDEX 2012, Science Foundation Ireland (16/ERCD/3797), NIH (grant RO1 MH085772-01A1; Axon, Testosterone, and Mental Health During Adolescence), and NIH Consortium grant U54 EB020403, supported by a cross-NIH alliance that funds Big Data to Knowledge Centers of Excellence and the NIHR Cambridge Biomedical Research Centre (Mental Health Theme). IMAGEN data are available by application to consortium coordinator Dr. Schumann (http://imagen-europe.com) after evaluation according to an established procedure. The authors thank the ADHD-200 Consortium (http://fcon_1000.projects.nitrc.org/indi/adhd200/) for their generosity in making data publicly available. The codes for the statistical analyses are available at https://github.com/qluo2018/DualPathwayADHD. Dr. Banaschewski has served as an adviser or consultant for Actelion, Eli Lilly, Hexal Pharma, Infectopharm, Lundbeck, Medice, Neurim Pharmaceuticals, Oberberg GmbH, and Shire; he has received conference support or speaking fees from Eli Lilly, Medice, Novartis, and Shire; he has been involved in clinical trials conducted by Shire and Vifor pharma; and he has received royalties from CIP Medien, Hogrefe, Kohlhammer, and Oxford University Press. Dr. Bokde has received support from the Health Research Board (Ireland) and the National Children’s Foundation Tallaght, Tallaght University Hospital, Ireland. Dr. Poustka has received speaking fees from Infectopharm and Shire; she receives funding from the Federal Ministry of Education and Research (BMBF), the German Research Foundation (DFG), 852 ajp.psychiatryonline.org Am J Psychiatry 177:9, September 2020 NEURAL CORRELATES OF THE DUAL-PATHWAY MODEL FOR ADHD IN ADOLESCENTS

SHEN ET AL of ear ademic skill deficits.JChild Psychol Psychiatry commercial interests cepeg3a820gn0y.2o2o orm C REFERENCES Willcutt EG:The prevalence of DSM-IV . istence ofattention nemory un n-deficit 22. vad J.de Graaf R.et al:Childhood predictors of adul neity in ADHD:neu nd symptom domains 24 ella yond he 25. or and Psychiatry 2016:73:815-82s a-analysis.JAMA 26 27. 3020 Mol Psychi 20101b 9.Kofler MJ,Rap MD Sa iontime variabilityin Psychol Rev 2013 nnaire:a re 10. S,et al.H 12 RubiaktCdord on sus "hot" in condu de 59-e87 h MD. nts.Biol Psy y2017:82:6 14. DR,Seth S,Ma ib ah n ti ent in psychiatry yond the Med 204:4 .Goodman:Psych L98 Barke )Dalen L Remington B:Do utive deficits and 7- 37 ang-R.Chan RC 38.Due 17 Am JPsychiatry 1779.September 2020
and the European Union and royalties from Hogrefe, Kohlhammer, and Schattauer. Dr. Sahakian has served as a consultant for Cambridge Cognition and Peak. The other authors report no financial relationships with commercial interests. Received February 18, 2019; revision received February 17, 2020; accepted March 18, 2020; published online May 7, 2020. REFERENCES 1. Willcutt EG: The prevalence of DSM-IV attention-deficit/ hyperactivity disorder: a meta-analytic review. Neurotherapeutics 2012; 9:490–499 2. BarkleyRA, FischerM, Smallish L, et al: The persistence of attentiondeficit/hyperactivity disorder into young adulthood as a function of reporting source and definition of disorder. J Abnorm Psychol 2002; 111:279–289 3. Lara C, Fayyad J, de Graaf R, et al: Childhood predictors of adult attention-deficit/hyperactivity disorder: results from the World Health Organization World Mental Health Survey Initiative. Biol Psychiatry 2009; 65:46–54 4. Wåhlstedt C, Thorell LB, Bohlin G: Heterogeneity in ADHD: neuropsychological pathways, comorbidity, and symptom domains. J Abnorm Child Psychol 2009; 37:551–564 5. Sonuga-Barke EJ: The dual pathway model of AD/HD: an elaboration of neuro-developmental characteristics. Neurosci Biobehav Rev 2003; 27:593–604 6. Sonuga-Barke EJ: Causal models of attention-deficit/hyperactivity disorder: from common simple deficits to multiple developmental pathways. Biol Psychiatry 2005; 57:1231–1238 7. Sonuga-Barke EJS: Psychological heterogeneity in AD/HD: a dual pathway model of behaviour and cognition. Behav Brain Res 2002; 130:29–36 8. Martinussen R, Hayden J, Hogg-Johnson S, et al: A meta-analysis of working memory impairments in children with attention-deficit/ hyperactivity disorder. J Am Acad Child Adolesc Psychiatry 2005; 44:377–384 9. Kofler MJ, RapportMD, Sarver DE, et al: Reaction time variability in ADHD: a meta-analytic review of 319 studies. Clin Psychol Rev 2013; 33:795–811 10. Crosbie J, Arnold P, PatersonA, et al: Responseinhibition andADHD traits: correlates and heritability in a community sample. J Abnorm Child Psychol 2013; 41:497–507 11. Sonuga-Barke EJ, Taylor E, Sembi S, et al: Hyperactivity and delay aversion, I: the effect of delay on choice. J Child Psychol Psychiatry 1992; 33:387–398 12. Rubia K: “Cool” inferior frontostriatal dysfunction in attention-deficit/ hyperactivity disorder versus “hot” ventromedial orbitofrontallimbic dysfunction in conduct disorder: a review. Biol Psychiatry 2011; 69:e69–e87 13. Sonuga-Barke E, Bitsakou P, ThompsonM: Beyond the dual pathway model: evidence for the dissociation of timing, inhibitory, and delayrelated impairments in attention-deficit/hyperactivity disorder. J Am Acad Child Adolesc Psychiatry 2010; 49:345–355 14. Coghill DR, Seth S, Matthews K: A comprehensive assessment of memory, delay aversion, timing, inhibition, decision making, and variability in attention deficit hyperactivity disorder: advancing beyond the three-pathway models. Psychol Med 2014; 44: 1989–2001 15. Sonuga-Barke EJ, Dalen L, Remington B: Do executive deficits and delay aversion make independent contributions to preschool attention-deficit/hyperactivity disorder symptoms? J Am Acad Child Adolesc Psychiatry 2003; 42:1335–1342 16. Yang B-R, Chan RC, Gracia N, et al: Cool and hot executive functions in medication-naive attention deficit hyperactivity disorder children. Psychol Med 2011; 41:2593–2602 17. Thorell LB: Do delay aversion and executive function deficits make distinct contributions to the functional impact of ADHD symptoms? A study of early academic skill deficits. J Child Psychol Psychiatry 2007; 48:1061–1070 18. SolantoMV, Abikoff H, Sonuga-Barke E, et al: The ecological validity of delay aversion and response inhibition as measures of impulsivity in AD/HD: a supplement to the NIMH multimodal treatment study of AD/HD. J Abnorm Child Psychol 2001; 29:215–228 19. Patros CHG, L Sweeney K, Mahone EM, et al: Greater delay discounting among girls, but not boys, with ADHD correlates with cognitive control. Child Neuropsychol 2018; 24:1026–1046 20. Karalunas SL, Huang-Pollock CL: Examining relationships between executive functioning and delay aversion in attention deficit hyperactivity disorder. J Clin Child Adolesc Psychol 2011; 40:837–847 21. Patros CHG, Alderson RM, Lea SE, et al: Visuospatial working memory underlies choice-impulsivity in boys with attention-deficit/ hyperactivity disorder. Res Dev Disabil 2015; 38:134–144 22. Bickel WK, Yi R, Landes RD, et al: Remember the future: working memory training decreases delay discounting among stimulant addicts. Biol Psychiatry 2011; 69:260–265 23. Wesley MJ, Bickel WK: Remember the future II: meta-analyses and functional overlap of working memory and delay discounting. Biol Psychiatry 2014; 75:435–448 24. Castellanos FX, Proal E: Large-scale brain systems in ADHD: beyond the prefrontal-striatal model. Trends Cogn Sci 2012; 16:17–26 25. Norman LJ, Carlisi C, Lukito S, et al: Structural and functional brain abnormalities in attention-deficit/hyperactivity disorder and obsessive-compulsive disorder: a comparative meta-analysis. JAMA Psychiatry 2016; 73:815–825 26. ThaparA: Discoveries on the genetics ofADHDin the 21st century: new findings and their implications. Am J Psychiatry 2018; 175:943–950 27. Meyer-Lindenberg A, Weinberger DR: Intermediate phenotypes and genetic mechanisms of psychiatric disorders. Nat Rev Neurosci 2006; 7:818–827 28. Schumann G, Loth E, Banaschewski T, et al: The IMAGEN study: reinforcement-related behaviour in normal brain function and psychopathology. Mol Psychiatry 2010; 15:1128–1139 29. Milham MP, Fair D, Mennes M, et al: a model to advance the translational potential of neuroimaging in clinical neuroscience. Front Syst Neurosci 2012; 6:62 30. Goodman R: The Strengths and Difficulties Questionnaire: a research note. J Child Psychol Psychiatry 1997; 38:581–586 31. Albaugh MD, Hudziak JJ, Ing A, et al: White matter microstructure is associated with hyperactive/inattentive symptomatology and polygenic risk for attention-deficit/hyperactivity disorder in a population-based sample of adolescents. Neuropsychopharmacology 2019; 44:1597–1603 32. Albaugh MD, Ivanova M, Chaarani B, et al: Ventromedial prefrontal volume in adolescence predicts hyperactive/inattentive symptoms in adulthood. Cereb Cortex 2019; 29:1866–1874 33. Albaugh MD, Orr C, Chaarani B, et al: Inattention and reaction time variability are linked to ventromedial prefrontal volume in adolescents. Biol Psychiatry 2017; 82:660–668 34. Bayard F, Nymberg Thunell C, Abé C, et al: Distinct brain structure and behavior related to ADHD and conduct disorder traits. Mol Psychiatry (Epub ahead of print, August 14, 2018) 35. Shrout PE: Measurement reliability and agreement in psychiatry. Stat Methods Med Res 1998; 7:301–317 36. Goodman R: Psychometric properties of the Strengths and Diffi- culties Questionnaire. J Am Acad Child Adolesc Psychiatry 2001; 40: 1337–1345 37. Kirby KN, Petry NM, Bickel WK: Heroin addicts have higher discount rates for delayed rewards than non-drug-using controls. J Exp Psychol Gen 1999; 128:78–87 38. Duckworth AL, SeligmanME: Self-discipline outdoes IQ in predicting academic performance of adolescents. Psychol Sci 2005; 16:939–944 39. Sahakian BJ, Owen AM: Computerized assessment in neuropsychiatry using CANTAB: discussion paper. J R Soc Med 1992; 85: 399–402 Am J Psychiatry 177:9, September 2020 ajp.psychiatryonline.org 853 SHEN ET AL
按次数下载不扣除下载券;
注册用户24小时内重复下载只扣除一次;
顺序:VIP每日次数-->可用次数-->下载券;
- 《认知心理学》课程教学资源(书籍文献)反射性注意的返回抑制起始时间可显著预测认知老化 Onset Time of Inhibition of Return Is a Promising Index for Assessing Cognitive Functions in Older Adults.pdf
- 《认知心理学》课程教学资源(书籍文献)聋人听觉皮层在感知觉功能重组中的作用 Reorganization of large-scale brain networks in deaf signing adults - The role of auditory cortex in functional reorganization following deafness.pdf
- 《认知心理学》课程教学资源(书籍文献)中国心理学家揭示面孔身份识别和表情识别的关联机制 Emerged human-like facial expression representation in a deep convolutional neural network.pdf
- 《认知心理学》课程教学资源(书籍文献)Science研究破解“脸盲”奥秘 A fast link between face perception and memory in the temporal pole.pdf
- 《认知心理学》课程教学资源(书籍文献)认知神经科学——关于心智的生物学(美)MICHAEL S.GAZZANIGA,Cognitive Neuroscience The Biology of the Mind(3rd Edition,彩色电子版).pdf
- 南方医科大学:《认知心理学》课程教学资源(课件讲稿)第一章 研究方法——认知神经科学(主讲:谭相良).pdf
- 《认知心理学》课程教学资源(书籍文献)The Student’s Guide to Cognitive Neuroscience,Fourth Edition,by Jamie Ward SY.pdf
- 《认知心理学》课程教学资源(书籍文献)Cognitive Neuroscience - The Biology of the Mind,Fourth Edition,Michael S. Gazzaniga, Richard B. Ivry, and George R. Mangun.pdf
- 《认知心理学》课程教学资源(书籍文献)认知科学与你的生活(原书第5版)美国名校学生最喜爱的心理学教材(美)凯瑟琳·加洛蒂(Kathleen M. Galotti)Cognitive Psychology - In and Out of the Laboratory,5th Edition.pdf
- 南方医科大学:《认知心理学》课程教学大纲 Cognitive psychology.pdf
- 《中国传统文化》课程教案讲义(打印版)第十六章 中外文化交流.pdf
- 《中国传统文化》课程教案讲义(打印版)第十一章 中国人生礼仪文化.pdf
- 《中国传统文化》课程教案讲义(打印版)第十章 中国饮食文化.pdf
- 《中国传统文化》课程教案讲义(打印版)第九章 中国服饰文化.pdf
- 《中国传统文化》课程教案讲义(打印版)第一章 文化简论.pdf
- 《中国传统文化》课程教学大纲(打印版)Introduction to Chinese Traditional Culture.pdf
- 《压力管理与心理健康》课程教学资源(教案讲义)第七章 消除压力,维护健康心理(打印版).pdf
- 《压力管理与心理健康》课程教学资源(教案讲义)第六章 压力自我调适的方法与技巧(打印版).pdf
- 《压力管理与心理健康》课程教学资源(教案讲义)第五章 认知重构与压力管理(打印版).pdf
- 《压力管理与心理健康》课程教学资源(教案讲义)第四章 人格特质与心理压力(打印版).pdf
- 《认知心理学》课程教学资源(书籍文献)神经与精神疾病(精神疾病的愉快体验加工和社会认知).pdf
- 南方医科大学:《认知心理学》课程教学资源(课件讲稿)第一章 认知心理——研究方法.pdf
- 南方医科大学:《认知心理学》课程教学资源(课件讲稿)第七章 记忆的认知神经机制.pdf
- 南方医科大学:《认知心理学》课程教学资源(课件讲稿)第三章 大脑半球的偏侧化(主讲:张瑞彬).pdf
- 南方医科大学:《认知心理学》课程教学资源(课件讲稿)第九章 社会认知的神经机制.pdf
- 南方医科大学:《认知心理学》课程教学资源(课件讲稿)第五章 物体识别的认知神经机制.pdf
- 南方医科大学:《认知心理学》课程教学资源(课件讲稿)第八章 情绪的认知神经机制.pdf
- 南方医科大学:《认知心理学》课程教学资源(课件讲稿)第六章 注意的认知神经机制.pdf
- 南方医科大学:《认知心理学》课程教学资源(课件讲稿)第十章 决策的认知神经机制.pdf
- 南方医科大学:《认知心理学》课程教学资源(课件讲稿)第四章 感知觉的认知神经机制——以观觉为例.pdf
- 南方医科大学:《认知心理学》课程教学资源(课件讲稿)记忆的认知神经机制-课件-王优.pdf
- 山东第一医科大学(泰山医学院):《大学生心理健康教育》课程教学大纲 Mental Health Education of College Student.doc
- 山东第一医科大学(泰山医学院):《大学生心理健康教育》课程教学资源(授课教案)第1章 绪论(负责人:冯宪萍).doc
- 山东第一医科大学(泰山医学院):《大学生心理健康教育》课程教学资源(授课教案)第2章 新生与环境适应.doc
- 山东第一医科大学(泰山医学院):《大学生心理健康教育》课程教学资源(授课教案)第3章 自我意识与大学生心理健康.doc
- 山东第一医科大学(泰山医学院):《大学生心理健康教育》课程教学资源(授课教案)第4章 情绪与大学生心理健康.doc
- 山东第一医科大学(泰山医学院):《大学生心理健康教育》课程教学资源(授课教案)第5章 人格与大学生心理健康.doc
- 山东第一医科大学(泰山医学院):《大学生心理健康教育》课程教学资源(授课教案)第6章 人际交往与大学生心理健康.doc
- 山东第一医科大学(泰山医学院):《大学生心理健康教育》课程教学资源(授课教案)第7章 爱情与性心理.doc
- 山东第一医科大学(泰山医学院):《大学生心理健康教育》课程教学资源(授课教案)第8章 学习心理.doc