《计算机科学》相关教学资源(参考文献)Dynamic Sampling from Graphical Models
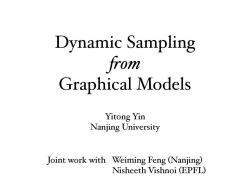
Dynamic Sampling from Graphical Models Yitong Yin Nanjing University Joint work with Weiming Feng (Nanjing) Nisheeth Vishnoi EPFL)
Dynamic Sampling fom Graphical Models Yitong Yin Nanjing University Joint work with Weiming Feng (Nanjing) Nisheeth Vishnoi (EPFL)
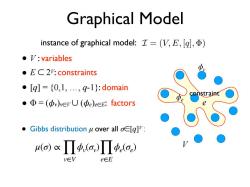
Graphical Model instance of graphical model:=(V,E,g,) oD:variables ●EC2:constraints [g]=(0,1,...g-1:domain ●pe constraint( ●Φ=(中v)eyU(中e)eeE:factors Gibbs distribution u over all oE[g]v: 4(o)x中,(o,)中(o。) v∈V e∈E
Graphical Model • Gibbs distribution µ over all σ∈[q]V : • V : variables • E ⊂ 2V: constraints • [q] = {0,1, …, q-1}: domain • Φ = (�v)v∈V ∪ (�e)e∈E: factors μ(σ) ∝ ∏ v∈V ϕv(σv) ∏ e∈E ϕe(σe) constraint e V ϕv ϕe instance of graphical model: I = (V,E, [q], )
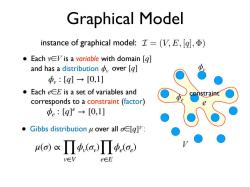
Graphical Model instance of graphical model:=(V,E,g,) ● Each vEV is a variable with domain [g] and has a distribution中,over[q] 中:[q]→[0,1] ●Each e∈E is a set of variables and corresponds to a constraint (factor) e constraint φe:[q]e→[0,1] ● Gibbs distribution u over all olg]: 4(o)x中,(o,)中(oe) v∈V e∈E
Graphical Model • Each v∈V is a variable with domain [q] and has a distribution • Each e∈E is a set of variables and corresponds to a constraint (factor) ϕe : [q] e → [0,1] μ(σ) ∝ ∏ v∈V ϕv(σv) ∏ e∈E ϕe(σe) constraint e ϕv over [q] V ϕv ϕe ϕv : [q] → [0,1] instance of graphical model: I = (V,E, [q], ) • Gibbs distribution µ over all σ∈[q]V :
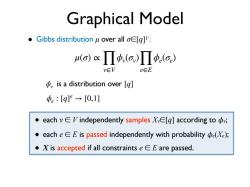
Graphical Model Gibbs distribution u over all oe[g]v: 4(o)cφ,(o,)Πp(a) v∈V e∈E is a distribution over [g] φe:[q]e→[0,1] ● each vE V independently samples XvE[g]according to ● each eEE is passed independently with probability e(Xe); X is accepted if all constraints e eE are passed
Graphical Model • Gibbs distribution µ over all σ∈[q]V : μ(σ) ∝ ∏ v∈V ϕv(σv) ∏ e∈E ϕe(σe) ϕv is a distribution over [q] ϕe : [q] e → [0,1] • each v ∈ V independently samples Xv∈[q] according to �v; • each e ∈ E is passed independently with probability �e(Xe); • X is accepted if all constraints e ∈ E are passed
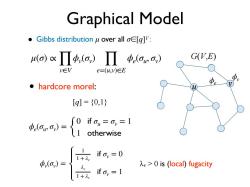
Graphical Model ·Gibbs distribution u over all o∈[q]': a(o)cΠ中,(o,)Π.(o,) G(V,E) v∈V e=(u,v)∈E ●hardcore morel: V [q]={0,1} grve otherwise if oy=0 A>0 is (local)fugacity if o=1
Graphical Model • Gibbs distribution µ over all σ∈[q]V : μ(σ) ∝ ∏ v∈V ϕv(σv) ∏ e=(u,v)∈E ϕe(σu, σv) ϕ ϕv e u v G(V,E) • hardcore morel: [q] = {0,1} ϕe(σu, σv) = { 0 if σu = σv = 1 1 otherwise ϕv(σv) = 1 1 + λv if σv = 0 λv 1 + λv if σv = 1 λv > 0 is (local) fugacity
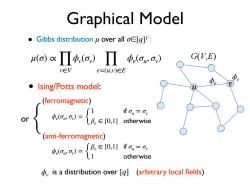
Graphical Model ●Gibbs distribution u over all o∈[q]': a(o)cΠ,(a,)Πg e(ou,O) G(V,E) v∈V e=(u,v)∈E Ising/Potts model: (ferromagnetic) if ou=oy or了 10.1 otherwise (anti-ferromagnetic) a,ow={eolee otherwise is a distribution over [g]( (arbitrary local fields)
Graphical Model • Gibbs distribution µ over all σ∈[q]V : μ(σ) ∝ ∏ v∈V ϕv(σv) ∏ e=(u,v)∈E ϕe(σu, σv) ϕ ϕv e u v G(V,E) • Ising/Potts model: ϕe(σu, σv) = { βe ∈ [0,1] if σu = σv 1 otherwise ϕe(σu, σv) = { 1 if σu = σv βe ∈ [0,1] otherwise ϕv is a distribution over [q] (ferromagnetic) (anti-ferromagnetic) or { (arbitrary local fields)
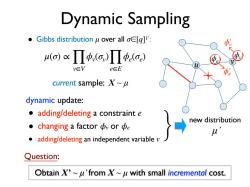
Dynamic Sampling ·Gibbs distribution u over all oe∈[q]': 4(o)x,(o,)中(oe) v∈V e∈E current sample:X~u dynamic update: adding/deleting a constraint e new distribution changing a factor y or e 儿 adding/deleting an independent variable v Question: Obtain X'~u'from X~u with small incremental cost
Dynamic Sampling • Gibbs distribution µ over all σ∈[q]V : ϕ ϕv e u v • adding/deleting a constraint e • changing a factor �v or �e • adding/deleting an independent variable v current sample: X ~ µ μ(σ) ∝ ∏ v∈V ϕv(σv) ∏ e∈E ϕe(σe) dynamic update: Obtain X’ ~ µ’ from X ~ µ with small incremental cost. Question: new distribution } µ’ ϕ′ e ϕ′ v
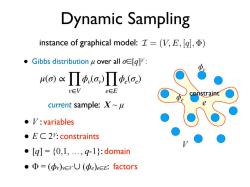
Dynamic Sampling instance of graphical model:=(V,E,g,) Gibbs distribution u over all oeg]: D u(o)xb(G)B.(G.) v∈V e∈E Ope constraint current sample:X~u ●V:variables 。EC2:constraints ·[q]={0,1,.,q-1}:domain ●Φ=(中v)eyU(中e)eeE:factors
Dynamic Sampling instance of graphical model: I = (V,E, [q], ) • V : variables • E ⊂ 2V: constraints • [q] = {0,1, …, q-1}: domain • Φ = (�v)v∈V ∪ (�e)e∈E: factors constraint e V ϕv ϕe • Gibbs distribution µ over all σ∈[q]V : current sample: X ~ µ μ(σ) ∝ ∏ v∈V ϕv(σv) ∏ e∈E ϕe(σe)
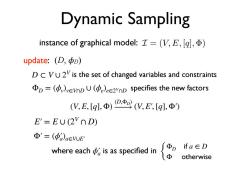
Dynamic Sampling instance of graphical model::Z=(V,E,[gl,Φ)) update:(D,D) D C VU2V is the set of changed variables and constraints ΦD=(φ,)vEVnD U(中e)e∈2 vD specifies the new factors (Y,E,[gl,Φ)Dg(Y,E,[ql,Φ) E'=EU(2V∩D) Φ'=(pa)a∈uE' where each is as specified in {8 ifa∈D otherwise
Dynamic Sampling instance of graphical model: I = (V,E, [q], ) update: (D, �D) (V, E, [q], Φ) (D,ΦD) (V, E′ , [q], Φ′) is the set of changed variables and constraints ΦD = (ϕv)v∈V∩D ∪ (ϕe)e∈2V∩D specifies the new factors D ⊂ V ∪ 2V E′ = E ∪ (2V ∩ D) Φ′ = (ϕ′ a)a∈V∪E′ where each ϕ′ a is as specified in { ΦD if a ∈ D Φ otherwise
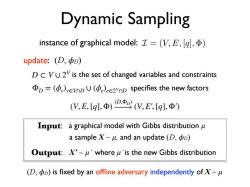
Dynamic Sampling instance of graphical model:Z =(V,E,g,) update:(D,D) DC VU2V is the set of changed variables and constraints D=()vEVD U(e)eE2vD specifies the new factors (V,E,Iql,)VEIql) Input:a graphical model with Gibbs distribution a sample ~u,and an update (D,D) Output:X'~u'where u'is the new Gibbs distribution (D,p)is fixed by an offline adversary independently of X~u
Dynamic Sampling Input: Output: a graphical model with Gibbs distribution µ a sample X ~ µ, and an update (D, �D) X’ ~ µ’ where µ’ is the new Gibbs distribution instance of graphical model: I = (V,E, [q], ) update: (D, �D) (V, E, [q], Φ) (D,ΦD) (V, E′ , [q], Φ′) is the set of changed variables and constraints ΦD = (ϕv)v∈V∩D ∪ (ϕe)e∈2V∩D specifies the new factors D ⊂ V ∪ 2V (D, �D) is fixed by an offline adversary independently of X ~ µ
按次数下载不扣除下载券;
注册用户24小时内重复下载只扣除一次;
顺序:VIP每日次数-->可用次数-->下载券;
- 《计算机科学》相关教学资源(参考文献)Dynamic inference in probabilistic graphical models.pdf
- 中国计算机学会学术著作丛书:《对等网络——结构、应用与设计 Peer-to-Peer Network Structure, Application and Design》PDF电子书(正文,共九章).pdf
- 电子科技大学:《嵌入式系统设计 Embedded Systems Design》课程教学资源(课件讲稿)Chapter 3 Hot topics in ES.pdf
- 电子科技大学:《嵌入式系统设计 Embedded Systems Design》课程教学资源(课件讲稿)Case 4.pdf
- 电子科技大学:《嵌入式系统设计 Embedded Systems Design》课程教学资源(课件讲稿)Case Analysis - Use DARTS to Design a S/W System of Robot Controller.pdf
- 电子科技大学:《嵌入式系统设计 Embedded Systems Design》课程教学资源(课件讲稿)Chapter 5 ask Management.pdf
- 电子科技大学:《嵌入式系统设计 Embedded Systems Design》课程教学资源(课件讲稿)Chapter 4 Task Management.pdf
- 电子科技大学:《嵌入式系统设计 Embedded Systems Design》课程教学资源(课件讲稿)Chapter 3 Software System.pdf
- 电子科技大学:《嵌入式系统设计 Embedded Systems Design》课程教学资源(课件讲稿)Chapter 2 Hardware System.pdf
- 电子科技大学:《嵌入式系统设计 Embedded Systems Design》课程教学资源(课件讲稿)Chapter 1 Overview(廖勇).pdf
- 南京大学:《随机算法 Randomized Algorithms》课程教学资源(课件讲稿)Universal Hashing.pdf
- 南京大学:《随机算法 Randomized Algorithms》课程教学资源(课件讲稿)Random Rounding.pdf
- 南京大学:《随机算法 Randomized Algorithms》课程教学资源(课件讲稿)Moments.pdf
- 南京大学:《随机算法 Randomized Algorithms》课程教学资源(课件讲稿)Mixing.pdf
- 南京大学:《随机算法 Randomized Algorithms》课程教学资源(课件讲稿)Min-Cut.pdf
- 南京大学:《随机算法 Randomized Algorithms》课程教学资源(课件讲稿)Markov Chain.pdf
- 南京大学:《随机算法 Randomized Algorithms》课程教学资源(课件讲稿)Lovász Local Lemma.pdf
- 南京大学:《随机算法 Randomized Algorithms》课程教学资源(课件讲稿)Identity Testing.pdf
- 南京大学:《随机算法 Randomized Algorithms》课程教学资源(课件讲稿)Finger printing.pdf
- 南京大学:《随机算法 Randomized Algorithms》课程教学资源(课件讲稿)Coupling.pdf
- 《计算机科学》相关教学资源(参考文献)On Local Distributed Sampling and Counting.pdf
- 《计算机科学》相关教学资源(参考文献)What can be sampled locally?.pdf
- 《计算机科学》相关教学资源(参考文献)Convergence of MCMC and Loopy BP in the Tree Uniqueness Region for the Hard-Core Model.pdf
- 《计算机科学》相关教学资源(参考文献)Counting hypergraph matchings up to uniqueness threshold.pdf
- 《计算机科学》相关教学资源(参考文献)Simple average-case lower bounds for approximate near-neighbor from isoperimetric inequalities.pdf
- 《计算机科学》相关教学资源(参考文献)Spatial mixing and the connective constant - Optimal bounds.pdf
- 《计算机科学》相关教学资源(参考文献)Spatial Mixing of Coloring Random Graphs.pdf
- 《计算机科学》相关教学资源(参考文献)Approximate Counting via Correlation Decay in Spin Systems.pdf
- 《计算机科学》相关教学资源(参考文献)Approximate Counting via Correlation Decay on Planar Graphs.pdf
- 《计算机科学》相关教学资源(参考文献)Assigning Tasks for Efficiency in Hadoop.pdf
- 《计算机科学》相关教学资源(参考文献)Correlation Decay up to Uniqueness in Spin Systems.pdf
- 《计算机科学》相关教学资源(参考文献)Improved FPTAS for Multi-Spin Systems.pdf
- 《计算机科学》相关教学资源(参考文献)Cell-probe proofs and nondeterministic cell-probe complexity.pdf
- 《计算机科学》相关教学资源(参考文献)Cell-Probe Proofs.pdf
- 《计算机科学》相关教学资源(参考文献)Fast construction of overlay networks.pdf
- 《计算机科学》相关教学资源(参考文献)Ranged hash functions and the price of churn.pdf
- 《计算机科学》相关教学资源(参考文献)Dynamic and Distributed Algorithms for Sampling from Gibbs Distributions.pdf
- 《计算机科学》相关教学资源(参考文献)Fast Sampling Constraint Satisfaction Solutions via the Lovász Local Lemma.pdf
- 《计算机科学》相关教学资源(参考文献)Distributed Algorithms for MCMC Sampling.pdf
- 《计算机科学》相关教学资源(参考文献)Dynamic Sampling from Graphical Models.pdf