《计算机科学》相关教学资源(参考文献)Simple average-case lower bounds for approximate near-neighbor from isoperimetric inequalities

Simple Average-case Lower Bounds for Approximate Near-Neighbor from Isoperimetric Inequalities Yitong Yin Nanjing University
Simple Average-case Lower Bounds for Approximate Near-Neighbor fom Isoperimetric Inequalities Yitong Yin Nanjing University
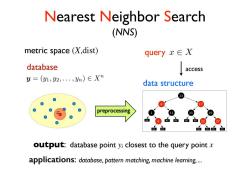
Nearest Neighbor Search (NNS) metric space (X,dist) query x∈X database access y=(y1,y2,,yn)∈Xn data structure preprocessing NIL NIL NIL NIL output:database point yi closest to the query point x applications:database,pattern matching,machine learning
Nearest Neighbor Search metric space (X,dist) database y = (y1, y2,...,yn) 2 Xn preprocessing data structure query x 2 X output: database point yi closest to the query point x (NNS) access applications: database, pattern matching, machine learning, ... x
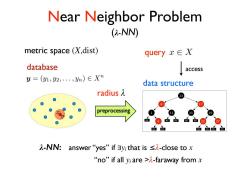
Near Neighbor Problem (a-NN) metric space (X,dist) query x∈X database access y=(y1,y2,,yn)∈Xn data structure radiusλ preprocessing 2 NIL NIL NIL 27 NIL NIL NIL -NN:answer“yes”if 3yi that is≤l-close tox “no”if all yiare>人-faraway fromx
Near Neighbor Problem database y = (y1, y2,...,yn) 2 Xn data structure query x 2 X (λ-NN) access x radius λ “no” if all yi are >λ-faraway from x λ-NN: answer “yes” if ∃yi that is ≤λ-close to x metric space (X,dist) preprocessing
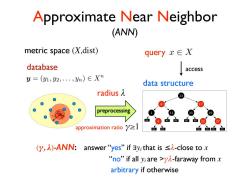
Approximate Near Neighbor (ANN) metric space (X,dist) query x∈X database access y=(y1,y2,,yn)∈Xm data structure radiusλ preprocessing NIL NIL NIL approximation ratio NIL NIL NIL (y,)-ANN:answer“yes”if yithat is≤λ-close tox "no"if all yiare >y-faraway fromx arbitrary if otherwise
Approximate Near Neighbor database y = (y1, y2,...,yn) 2 Xn data structure query x 2 X (ANN) access x radius λ “no” if all yi are >γλ-faraway from x approximation ratio γ≥1 arbitrary if otherwise (γ, λ)-ANN: answer “yes” if ∃yi that is ≤λ-close to x metric space (X,dist) preprocessing
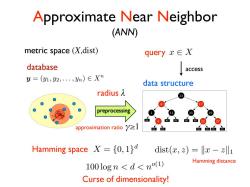
Approximate Near Neighbor (ANN) metric space (X,dist) query x∈X database access y=(y1,y2,,yn)∈Xn data structure radius入 preprocessing NIL NIL approximation ratio l NIL NIL NIL NIL NIL Hamming space={0,1}d dist(x,z)=x-z1 100logn<d≤no(1) Hamming distance Curse of dimensionality!
Approximate Near Neighbor database y = (y1, y2,...,yn) 2 Xn data structure query x 2 X (ANN) access x Hamming space X = {0, 1}d metric space (X,dist) dist(x, z) = kx zk1 Hamming distance Curse of dimensionality! preprocessing radius λ approximation ratio γ≥1 100 log n<d<no(1)
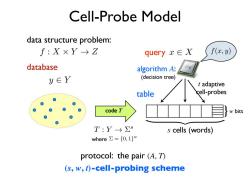
Cell-Probe Model data structure problem: f:X×Y→☑ query x∈X f(x,y) database algorithm A: CPU y∈Y (decision tree) t adaptive table cell-probes code T w bits T:Y→∑s s cells (words) where∑={0,1}w protocol:the pair (A,T) (s,w,t)-cell-probing scheme
code T table query x 2 X t adaptive cell-probes Cell-Probe Model } w bits ( s cells (words) algorithm A: ⌃ = {0, 1}w where (decision tree) protocol: the pair (A, T) database y 2 Y T : Y ! ⌃s f : X ⇥ Y ! Z data structure problem: (s, w, t)-cell-probing scheme f(x, y)
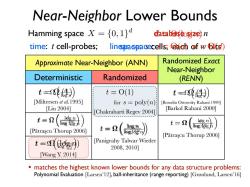
Near-Neighbor Lower Bounds Hamming space X ={0,1]d databaseosize)n time:t cell-probes;linepaspacecells;each of w bits) Approximate Near-Neighbor (ANN) Randomized Exact Near-Neighbor Deterministic Randomized (RENN) t=得) t=0(1) t=单 [Miltersen et al.1995] for s poly(n) Borodin Ostrovsky Rabani 1999] Liu2004] [Chakrabarti Regev 2004] Barkol Rabani 2000] Idg n 1og宽九 t=2 ldg n g玲照五 Patrascu Thorup 2006] t=() Patrascu Thorup 2006] t=刃 Panigrahy Talwar Wieder 2008,2010] Wang Y.2014] matches the highest known lower bounds for any data structure problems: Polynomial Evaluation [Larsen'12],ball-inheritance(range reporting)[Gronlund,Larsen'16]
Approximate Near-Neighbor (ANN) Randomized Exact Near-Neighbor Deterministic Randomized (RENN) Near-Neighbor Lower Bounds Hamming space X = {0, 1}d database size: n time: t cell-probes; space: s cells, each of w bits t = ⌦ ⇣ d log s ⌘ [Miltersen et al.1995] [Liu 2004] t = ⌦ ⇣ d log sw n ⌘ [Pătraşcu Thorup 2006] t = ⌦ ⇣ d log sw nd ⌘ [Wang Y. 2014] t = ⌦ ⇣ log n log sw n ⌘ [Panigrahy Talwar Wieder 2008, 2010] t = ⌦ ⇣ d log sw n ⌘ [Pătraşcu Thorup 2006] t = ⌦ ⇣ d log s ⌘ [Borodin Ostrovsky Rabani 1999] [Barkol Rabani 2000] for s = poly(n) [Chakrabarti Regev 2004] t = O(1) • matches the highest known lower bounds for any data structure problems: Polynomial Evaluation [Larsen’12], ball-inheritance (range reporting) [Grønlund, Larsen’16] linear space: s = Θ(n) w = Θ(d) d = ⇥(log n) t = ⌦ (1) t = ⌦ ⇣ log n log log n ⌘ t = ⌦ ⇣ log n log log n ⌘ t = ⌦ (log n) t = ⌦ ⇣ log n log log n ⌘ t = ⌦ (1)
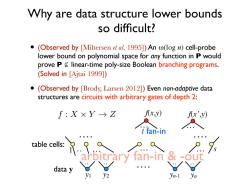
Why are data structure lower bounds so difficult? (Observed by Miltersen et al.1995])An (log n)cell-probe lower bound on polynomial space for any function in P would prove P g linear-time poly-size Boolean branching programs. (Solved in [Ajtai 1999]) (Observed by Brody,Larsen 2012])Even non-adaptive data structures are circuits with arbitrary gates of depth 2: f:XxY→Z fx,y) A,y) ifan-in data y y2 yn-1 n
Why are data structure lower bounds so difficult? • (Observed by [Miltersen et al. 1995]) An ω(log n) cell-probe lower bound on polynomial space for any function in P would prove P ⊈ linear-time poly-size Boolean branching programs. (Solved in [Ajtai 1999]) • (Observed by [Brody, Larsen 2012]) Even non-adaptive data structures are circuits with arbitrary gates of depth 2: f : X ⇥ Y ! Z data y y1 y2 yn-1 yn table cells: f(x,y) f(x’,y) arbitrary fan-in & -out t fan-in 1 s
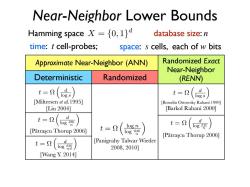
Near-Neighbor Lower Bounds Hamming space={0,1)d database size:n time:t cell-probes; space:s cells,each of w bits Approximate Near-Neighbor(ANN) Randomized Exact Near-Neighbor Deterministic Randomized (RENN) t=2 d log s t=2 () [Miltersen et al.1995] Borodin Ostrovsky Rabani 1999] Liu2004] [Barkol Rabani 2000] t=2( d log logn t=(e袋) Patrascu Thorup 2006] t=( Patrascu Thorup 2006] t=n() Panigrahy Talwar Wieder 2008,2010] [Wang Y.2014]
database size: n Approximate Near-Neighbor (ANN) Randomized Exact Near-Neighbor Deterministic Randomized (RENN) Near-Neighbor Lower Bounds Hamming space X = {0, 1}d time: t cell-probes; space: s cells, each of w bits t = ⌦ ⇣ d log s ⌘ [Miltersen et al.1995] [Liu 2004] t = ⌦ ⇣ d log sw n ⌘ [Pătraşcu Thorup 2006] t = ⌦ ⇣ d log sw nd ⌘ [Wang Y. 2014] t = ⌦ ⇣ log n log sw n ⌘ [Panigrahy Talwar Wieder 2008, 2010] t = ⌦ ⇣ d log sw n ⌘ [Pătraşcu Thorup 2006] t = ⌦ ⇣ d log s ⌘ [Borodin Ostrovsky Rabani 1999] [Barkol Rabani 2000]
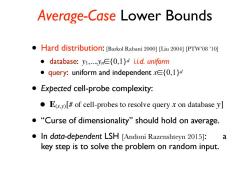
Average-Case Lower Bounds Hard distribution:[Barkol Rabani 2000][Liu 2004][PTW'08'10] ● database:y1,yn∈{0,1yd i.i..d.uniform ●query:uniform and independent xe∈{O,l}d Expected cell-probe complexity: E(.y)[of cell-probes to resolve query x on database y] "Curse of dimensionality"should hold on average. In data-dependent LSH [Andoni Razenshteyn 2015]: a key step is to solve the problem on random input
Average-Case Lower Bounds • Hard distribution: [Barkol Rabani 2000] [Liu 2004] [PTW’08 ’10] • database: y1,...,yn∈{0,1}d i.i.d. uniform • query: uniform and independent x∈{0,1}d • Expected cell-probe complexity: • E(x,y)[# of cell-probes to resolve query x on database y] • “Curse of dimensionality” should hold on average. • In data-dependent LSH [Andoni Razenshteyn 2015]: a key step is to solve the problem on random input
按次数下载不扣除下载券;
注册用户24小时内重复下载只扣除一次;
顺序:VIP每日次数-->可用次数-->下载券;
- 《计算机科学》相关教学资源(参考文献)Counting hypergraph matchings up to uniqueness threshold.pdf
- 《计算机科学》相关教学资源(参考文献)Convergence of MCMC and Loopy BP in the Tree Uniqueness Region for the Hard-Core Model.pdf
- 《计算机科学》相关教学资源(参考文献)What can be sampled locally?.pdf
- 《计算机科学》相关教学资源(参考文献)On Local Distributed Sampling and Counting.pdf
- 《计算机科学》相关教学资源(参考文献)Dynamic Sampling from Graphical Models.pdf
- 《计算机科学》相关教学资源(参考文献)Dynamic inference in probabilistic graphical models.pdf
- 中国计算机学会学术著作丛书:《对等网络——结构、应用与设计 Peer-to-Peer Network Structure, Application and Design》PDF电子书(正文,共九章).pdf
- 电子科技大学:《嵌入式系统设计 Embedded Systems Design》课程教学资源(课件讲稿)Chapter 3 Hot topics in ES.pdf
- 电子科技大学:《嵌入式系统设计 Embedded Systems Design》课程教学资源(课件讲稿)Case 4.pdf
- 电子科技大学:《嵌入式系统设计 Embedded Systems Design》课程教学资源(课件讲稿)Case Analysis - Use DARTS to Design a S/W System of Robot Controller.pdf
- 电子科技大学:《嵌入式系统设计 Embedded Systems Design》课程教学资源(课件讲稿)Chapter 5 ask Management.pdf
- 电子科技大学:《嵌入式系统设计 Embedded Systems Design》课程教学资源(课件讲稿)Chapter 4 Task Management.pdf
- 电子科技大学:《嵌入式系统设计 Embedded Systems Design》课程教学资源(课件讲稿)Chapter 3 Software System.pdf
- 电子科技大学:《嵌入式系统设计 Embedded Systems Design》课程教学资源(课件讲稿)Chapter 2 Hardware System.pdf
- 电子科技大学:《嵌入式系统设计 Embedded Systems Design》课程教学资源(课件讲稿)Chapter 1 Overview(廖勇).pdf
- 南京大学:《随机算法 Randomized Algorithms》课程教学资源(课件讲稿)Universal Hashing.pdf
- 南京大学:《随机算法 Randomized Algorithms》课程教学资源(课件讲稿)Random Rounding.pdf
- 南京大学:《随机算法 Randomized Algorithms》课程教学资源(课件讲稿)Moments.pdf
- 南京大学:《随机算法 Randomized Algorithms》课程教学资源(课件讲稿)Mixing.pdf
- 南京大学:《随机算法 Randomized Algorithms》课程教学资源(课件讲稿)Min-Cut.pdf
- 《计算机科学》相关教学资源(参考文献)Spatial mixing and the connective constant - Optimal bounds.pdf
- 《计算机科学》相关教学资源(参考文献)Spatial Mixing of Coloring Random Graphs.pdf
- 《计算机科学》相关教学资源(参考文献)Approximate Counting via Correlation Decay in Spin Systems.pdf
- 《计算机科学》相关教学资源(参考文献)Approximate Counting via Correlation Decay on Planar Graphs.pdf
- 《计算机科学》相关教学资源(参考文献)Assigning Tasks for Efficiency in Hadoop.pdf
- 《计算机科学》相关教学资源(参考文献)Correlation Decay up to Uniqueness in Spin Systems.pdf
- 《计算机科学》相关教学资源(参考文献)Improved FPTAS for Multi-Spin Systems.pdf
- 《计算机科学》相关教学资源(参考文献)Cell-probe proofs and nondeterministic cell-probe complexity.pdf
- 《计算机科学》相关教学资源(参考文献)Cell-Probe Proofs.pdf
- 《计算机科学》相关教学资源(参考文献)Fast construction of overlay networks.pdf
- 《计算机科学》相关教学资源(参考文献)Ranged hash functions and the price of churn.pdf
- 《计算机科学》相关教学资源(参考文献)Dynamic and Distributed Algorithms for Sampling from Gibbs Distributions.pdf
- 《计算机科学》相关教学资源(参考文献)Fast Sampling Constraint Satisfaction Solutions via the Lovász Local Lemma.pdf
- 《计算机科学》相关教学资源(参考文献)Distributed Algorithms for MCMC Sampling.pdf
- 《计算机科学》相关教学资源(参考文献)Dynamic Sampling from Graphical Models.pdf
- 《计算机科学》相关教学资源(参考文献)Sampling & Counting for Big Data.pdf
- 《计算机科学》相关教学资源(参考文献)Introduction to the Correlation Decay Method.pdf
- 《计算机科学》相关教学资源(参考文献)Local Distributed Sampling from Locally-Defined Distribution.pdf
- 《计算机科学》相关教学资源(参考文献)Rectangle Inequalities for Data Structure Lower Bounds.pdf
- 《计算机科学》相关教学资源(参考文献)Sampling up to the Uniqueness Threshold.pdf