《计算机科学》相关教学资源(参考文献)Spatial Mixing of Coloring Random Graphs
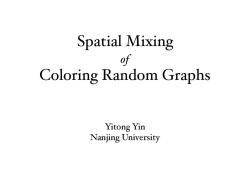
Spatial Mixing of Coloring Random Graphs Yitong Yin Nanjing University
Spatial Mixing of Coloring Random Graphs Yitong Yin Nanjing University
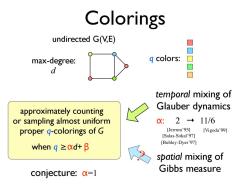
Colorings undirected G(V,E) max-degree: q colors: d 口 temporal mixing of approximately counting Glauber dynamics or sampling almost uniform 0:2→11/6 proper q-colorings of G [Jerrum'95] [Vigoda'99] [Salas-Sokal'97] [Bubley-Dyer'97] when q≥cd+B spatial mixing of conjecture:x=1 Gibbs measure
Colorings undirected G(V,E) approximately counting or sampling almost uniform proper q-colorings of G max-degree: q colors: d when q ≥αd+ temporal mixing of Glauber dynamics β spatial mixing of Gibbs measure ? α: 2 → 11/6 [Jerrum’95] [Salas-Sokal’97] [Bubley-Dyer’97] [Vigoda’99] conjecture: α=1
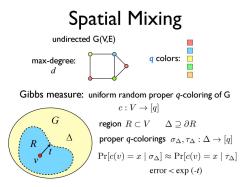
Spatial Mixing undirected G(V,E) 口 max-degree: q colors: d ▣ Gibbs measure:uniform random proper q-coloring of G c:V→[g region RCV △D∂R proper q-colorings,TA:△→[gl Pr[c(w)=x|o△]≈Pr[c(v)=x|T△] error exp (-t)
Spatial Mixing undirected G(V,E) q colors: Gibbs measure: uniform random proper q-coloring of G c : V ! [q] R G v t region R ⇢ V proper q-colorings error < exp (-t) max-degree: d ∆ , ⌧ : ! [q] ◆ @R Pr[c(v) = x | ] ⇡ Pr[c(v) = x | ⌧]
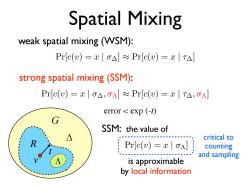
Spatial Mixing weak spatial mixing (WSM): Pr[c(w)=x|o△]≈Pr[C(v)=x|TA] strong spatial mixing (SSM): Pr[c(v)=A,]Pr[c(v)=xTA,OA] error exp (-t) SSM:the value of critical to Pr[c(v)=O] counting and sampling is approximable by local information
Spatial Mixing R G v t ⇤ weak spatial mixing (WSM): strong spatial mixing (SSM): error < exp (-t) Pr[c(v) = x | ⇤] is approximable by local information SSM: the value of critical to counting and sampling Pr[c(v) = x | ] ⇡ Pr[c(v) = x | ⌧] Pr[c(v) = x | , ⇤] ⇡ Pr[c(v) = x | ⌧, ⇤] ∆
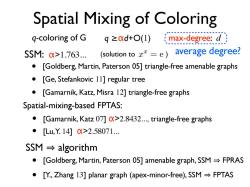
Spatial Mixing of Coloring q-coloring of G q≥0d+O(1) max-degree:d SSM:>1.763.. (solution to x=e) average degree? [Goldberg,Martin,Paterson 05]triangle-free amenable graphs [Ge,Stefankovic 11]regular tree [Gamarnik,Katz,Misra 12]triangle-free graphs Spatial-mixing-based FPTAS: ● [Gamarnik,Katz 07]>2.8432...,triangle-free graphs [Lu,Y.14]0>2.58071.. SSM→algorithm [Goldberg,Martin,Paterson 05]amenable graph,SSM=FPRAS [Y.,Zhang 13]planar graph (apex-minor-free),SSM=FPTAS
Spatial Mixing of Coloring • [Goldberg, Martin, Paterson 05] triangle-free amenable graphs • [Ge, Stefankovic 11] regular tree • [Gamarnik, Katz, Misra 12] triangle-free graphs q-coloring of G q ≥αd+O(1) max-degree: d • [Goldberg, Martin, Paterson 05] amenable graph, SSM 㱺 FPRAS • [Y., Zhang 13] planar graph (apex-minor-free), SSM 㱺 FPTAS • [Gamarnik, Katz 07] α>2.8432..., triangle-free graphs • [Lu, Y. 14] α>2.58071... SSM: α>1.763... xx (solution to ) = e SSM 㱺 algorithm Spatial-mixing-based FPTAS: average degree?
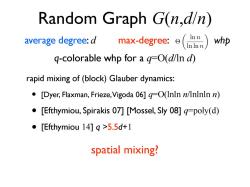
Random Graph G(n,d/n) average degree:d max-degree:whp q-colorable whp for a g=O(d/In d) rapid mixing of(block)Glauber dynamics: [Dyer,Flaxman,Frieze,Vigoda 06]g=O(Inln n/Inlnln n) [Efthymiou,Spirakis 07][Mossel,Sly 08]g=poly(d) [Efthymiou 14]g >5.5d+1 spatial mixing?
Random Graph G(n,d/n) • [Dyer, Flaxman, Frieze, Vigoda 06] q=O(lnln n/lnlnln n) • [Efthymiou, Spirakis 07] [Mossel, Sly 08] q=poly(d) • [Efthymiou 14] q >5.5d+1 average degree: d max-degree: ⇥ ✓ ln n ln ln n ◆ whp rapid mixing of (block) Glauber dynamics: q-colorable whp for a q=O(d/ln d) spatial mixing?
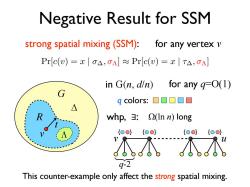
Negative Result for SSM strong spatial mixing(SSM): for any vertex v Pr[c(v)=x|o△,oA]≈Pr[C(v)=x|T△,OA] in G(n,dln) for any g=O(1) q colors:▣▣▣▣☐ whp,3:(In n)long 个不个不 This counter-example only affect the strong spatial mixing
Negative Result for SSM R G v t ⇤ strong spatial mixing (SSM): for any q=O(1) q colors: whp, ∃: Ω(ln n) long v u o q-2 { } { } { } { } in G(n, d/n) for any vertex v ∆ Pr[c(v) = x | , ⇤] ⇡ Pr[c(v) = x | ⌧, ⇤] This counter-example only affect the strong spatial mixing
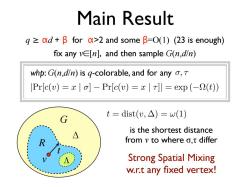
Main Result g=ad+B for a>2 and some B=0(1)(23 is enough) fix any vE[n],and then sample G(n,d/n) whp:G(n,d/n)is g-colorable,and for any o,T Pr[c(v)=x o]-Pr[c(v)=xT=exp(-(t)) t=dist(v,△)=w(1) is the shortest distance from v to where o,t differ Strong Spatial Mixing w.r.t any fixed vertex!
Main Result R G v t ⇤ q ≥ αd + β for α>2 and some β=O(1) (23 is enough) fix any v∈[n], and then sample G(n,d/n) whp: G(n,d/n) is q-colorable, and for any , ⌧ is the shortest distance from v to where σ,τ differ Strong Spatial Mixing w.r.t any fixed vertex! |Pr[c(v) = x | ] Pr[c(v) = x | ⌧ ]| = exp (⌦(t)) ∆ t = dist(v, ) = !(1)
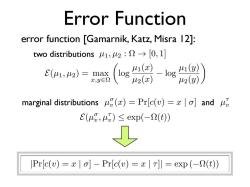
Error Function error function [Gamarnik,Katz,Misra 12]: two distributions ul,u2 :>0,1 E(1,u2)=max -log 1(g) x,y∈2 2(y) marginal distributions u()=Pr[c(v)=xo]and E(u,u)≤exp(-2(t) Pr[c(v)=xo]-Pr[c(v)=x]=exp(-2(t))
Error Function error function [Gamarnik, Katz, Misra 12]: two distributions µ1, µ2 : ⌦ ! [0, 1] marginal distributions µ v (x) = Pr[c(v) = x | ] and µ⌧ v |Pr[c(v) = x | ] Pr[c(v) = x | ⌧ ]| = exp (⌦(t)) E(µ v , µ⌧ v ) exp(⌦(t)) E(µ1, µ2) = max x,y2⌦ ✓ log µ1(x) µ2(x) log µ1(y) µ2(y) ◆
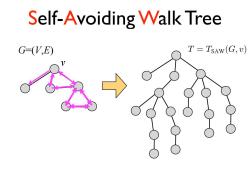
Self-Avoiding Walk Tree G-V.E) T=TSAW(G,U)
Self-Avoiding Walk Tree G=(V,E) v T = T;)?(G, v)
按次数下载不扣除下载券;
注册用户24小时内重复下载只扣除一次;
顺序:VIP每日次数-->可用次数-->下载券;
- 《计算机科学》相关教学资源(参考文献)Spatial mixing and the connective constant - Optimal bounds.pdf
- 《计算机科学》相关教学资源(参考文献)Simple average-case lower bounds for approximate near-neighbor from isoperimetric inequalities.pdf
- 《计算机科学》相关教学资源(参考文献)Counting hypergraph matchings up to uniqueness threshold.pdf
- 《计算机科学》相关教学资源(参考文献)Convergence of MCMC and Loopy BP in the Tree Uniqueness Region for the Hard-Core Model.pdf
- 《计算机科学》相关教学资源(参考文献)What can be sampled locally?.pdf
- 《计算机科学》相关教学资源(参考文献)On Local Distributed Sampling and Counting.pdf
- 《计算机科学》相关教学资源(参考文献)Dynamic Sampling from Graphical Models.pdf
- 《计算机科学》相关教学资源(参考文献)Dynamic inference in probabilistic graphical models.pdf
- 中国计算机学会学术著作丛书:《对等网络——结构、应用与设计 Peer-to-Peer Network Structure, Application and Design》PDF电子书(正文,共九章).pdf
- 电子科技大学:《嵌入式系统设计 Embedded Systems Design》课程教学资源(课件讲稿)Chapter 3 Hot topics in ES.pdf
- 电子科技大学:《嵌入式系统设计 Embedded Systems Design》课程教学资源(课件讲稿)Case 4.pdf
- 电子科技大学:《嵌入式系统设计 Embedded Systems Design》课程教学资源(课件讲稿)Case Analysis - Use DARTS to Design a S/W System of Robot Controller.pdf
- 电子科技大学:《嵌入式系统设计 Embedded Systems Design》课程教学资源(课件讲稿)Chapter 5 ask Management.pdf
- 电子科技大学:《嵌入式系统设计 Embedded Systems Design》课程教学资源(课件讲稿)Chapter 4 Task Management.pdf
- 电子科技大学:《嵌入式系统设计 Embedded Systems Design》课程教学资源(课件讲稿)Chapter 3 Software System.pdf
- 电子科技大学:《嵌入式系统设计 Embedded Systems Design》课程教学资源(课件讲稿)Chapter 2 Hardware System.pdf
- 电子科技大学:《嵌入式系统设计 Embedded Systems Design》课程教学资源(课件讲稿)Chapter 1 Overview(廖勇).pdf
- 南京大学:《随机算法 Randomized Algorithms》课程教学资源(课件讲稿)Universal Hashing.pdf
- 南京大学:《随机算法 Randomized Algorithms》课程教学资源(课件讲稿)Random Rounding.pdf
- 南京大学:《随机算法 Randomized Algorithms》课程教学资源(课件讲稿)Moments.pdf
- 《计算机科学》相关教学资源(参考文献)Approximate Counting via Correlation Decay in Spin Systems.pdf
- 《计算机科学》相关教学资源(参考文献)Approximate Counting via Correlation Decay on Planar Graphs.pdf
- 《计算机科学》相关教学资源(参考文献)Assigning Tasks for Efficiency in Hadoop.pdf
- 《计算机科学》相关教学资源(参考文献)Correlation Decay up to Uniqueness in Spin Systems.pdf
- 《计算机科学》相关教学资源(参考文献)Improved FPTAS for Multi-Spin Systems.pdf
- 《计算机科学》相关教学资源(参考文献)Cell-probe proofs and nondeterministic cell-probe complexity.pdf
- 《计算机科学》相关教学资源(参考文献)Cell-Probe Proofs.pdf
- 《计算机科学》相关教学资源(参考文献)Fast construction of overlay networks.pdf
- 《计算机科学》相关教学资源(参考文献)Ranged hash functions and the price of churn.pdf
- 《计算机科学》相关教学资源(参考文献)Dynamic and Distributed Algorithms for Sampling from Gibbs Distributions.pdf
- 《计算机科学》相关教学资源(参考文献)Fast Sampling Constraint Satisfaction Solutions via the Lovász Local Lemma.pdf
- 《计算机科学》相关教学资源(参考文献)Distributed Algorithms for MCMC Sampling.pdf
- 《计算机科学》相关教学资源(参考文献)Dynamic Sampling from Graphical Models.pdf
- 《计算机科学》相关教学资源(参考文献)Sampling & Counting for Big Data.pdf
- 《计算机科学》相关教学资源(参考文献)Introduction to the Correlation Decay Method.pdf
- 《计算机科学》相关教学资源(参考文献)Local Distributed Sampling from Locally-Defined Distribution.pdf
- 《计算机科学》相关教学资源(参考文献)Rectangle Inequalities for Data Structure Lower Bounds.pdf
- 《计算机科学》相关教学资源(参考文献)Sampling up to the Uniqueness Threshold.pdf
- 《计算机科学》相关教学资源(参考文献)What can be sampled locally?.pdf
- 《计算机科学》相关教学资源(参考文献)Correlation Decay up to Uniqueness in Spin Systems.pdf