南京大学:《随机算法 Randomized Algorithms》课程教学资源(课件讲稿)Coupling
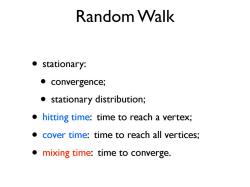
Random Walk stationary: ●convergence; stationary distribution; hitting time:time to reach a vertex; ● cover time:time to reach all vertices; mixing time:time to converge
Random Walk • stationary: • convergence; • stationary distribution; • hitting time: time to reach a vertex; • cover time: time to reach all vertices; • mixing time: time to converge
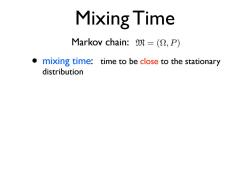
Mixing Time Markoy chain:t=(,P) ● mixing time:time to be close to the stationary distribution
Mixing Time Markov chain: M = (⌦, P) • mixing time: time to be close to the stationary distribution
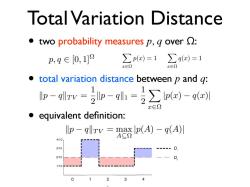
Total Variation Distance two probability measures p,g over p,9∈[0,1]0 ∑p(x)=1∑q(c)=1 x∈2 x∈2 total variation distance between p and g: Ilp-allrv jllp-all >lp(z)-a(s) x∈2 equivalent definition: ID-grv=Rlp(A)-g(A 4/10 3/10 D 2/10 1/10 0 2 3 4
Total Variation Distance • two probability measures p, q over Ω: • total variation distance between p and q: • equivalent definition: p, q 2 [0, 1]⌦ X x2⌦ p(x)=1 X x2⌦ q(x)=1 kp qkT V = 1 2 kp qk1 = 1 2 X x2⌦ |p(x) q(x)| COUPLING OF MARKOV CHAINS 4/10 r-----. I I I I I I r----- I I I - + - -:- --- -{ -- I I I ____ -' L __________ I 3/10 2/10 1/10 o 2 3 4 x Figure 11.1: Example of variation distance. The areas shaded by upward diagonal lines correspond to values x where DI (x) D 2(x). The total area shaded by upward diagonal lines must equal the total area shaded by downward diagonal lines, and the variation distance equals one of these two areas. we run the chain for a finite number of steps. If we want to use this Markov chain to shuffle the deck, how many steps are necessary before we obtain a shuffle that is to uniformly distributed? To quantify what we mean by "close to uniform", we must introduce a distance measure. Definition 11.1: The variation distance between two distributions D, and D2 on (f countable state space S is given by 1 liD, - D211 = 2: LID,(x) - D2(x)l. XES A pictorial example of the variation distance is given in Figure 11.1. The factor 1/2 in the definition of variation distance guarantees that the variation distance is between 0 and l. It also allows the following useful alternative characterization. Lemma 11.1: Foran), A S, let Di(A) = LXEA Di(x)jori = 1,2. Then A careful examination of Figure 11.1 helps make the proof of this lemma transparent. Proof: Let S+ S be the set of states such that D,(x) D2(X), and let S- S the set of states such that D2 (x) > D, (x). Clearly, and But since D,(S) = D2(S) = 1, we have D,(S+) + D,(S-) = D2(S+) + D2(S-) = 1, 272 kp qkT V = max A✓⌦ |p(A) q(A)|
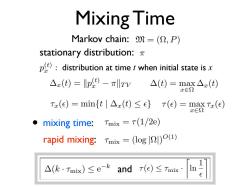
Mixing Time Markov chain:t=(,P) stationary distribution: p):distribution at time when initial state is △(t)=lp-π‖Tv △(t)=max△x(t) x∈2 Tx(e)=min{t|△x(t)≤e}T(e)=max T(e) x∈2 mixing time: Tmix =T(1/2e) rapid mixing: Tmix=(log2)0(1) △(k·Tmix)≤e-and(e)≤Tmix, In
Mixing Time Markov chain: M = (⌦, P) • mixing time: x(t) = kp(t) x ⇡kT V (t) = max x2⌦ x(t) ⌧x(✏) = min{t | x(t) ✏} ⌧ (✏) = max x2⌦ ⌧x(✏) ⌧mix = ⌧ (1/2e) stationary distribution: ⇡ p(t) x : distribution at time t when initial state is x rapid mixing: ⌧mix = (log |⌦|) O(1) (k · ⌧mix) ek ⌧ (✏) ⌧mix · ⇠ ln 1 ✏ ⇡ and
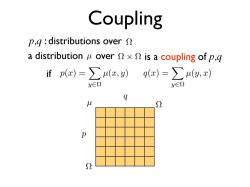
Coupling p,g:distributions over a distribution u over x is a coupling of p,q ifp(x)=>μ(,) q(c)=∑(y,x) y∈2 y∈2 9 0 2 p 2
Coupling p(x) = X y2⌦ µ(x, y) q(x) = X y2⌦ µ(y, x) a distribution µ over ⌦ ⇥ ⌦ is a coupling of p,q p,q : distributions over ⌦ if µ ⌦ ⌦ p q
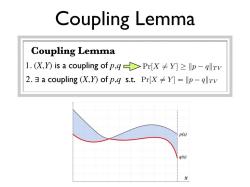
Coupling Lemma Coupling Lemma 1.(X,Y)is a coupling of p,q>Pr[X≠Y]≥lp-qlrv 2.3 a coupling (X,Y)of p,g s.t.Pr[XY]=llp-allrv p() g(x) x
Coupling Lemma 1. (X,Y) is a coupling of p,q Pr[X 6= Y ] kp qkT V 2. ∃ a coupling (X,Y) of p,q s.t. Pr[X 6= Y ] = kp qkT V Coupling Lemma
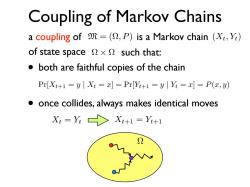
Coupling of Markov Chains a coupling of =(P)is a Markov chain (X,Y) of state space x such that: o l both are faithful copies of the chain Pr[X++1=y Xt=x]=Pr[Yi+1=yYi=x]=P(x,y) once collides,always makes identical moves Xi=Y>Xi+1=Yi+1
• both are faithful copies of the chain • once collides, always makes identical moves Coupling of Markov Chains ⌦ Pr[Xt+1 = y | Xt = x] = Pr[Yt+1 = y | Yt = x] = P(x, y) Xt = Yt Xt+1 = Yt+1 is a Markov chain (Xt, Yt) of state space a coupling of M = (⌦, P) ⌦ ⇥ ⌦ such that:
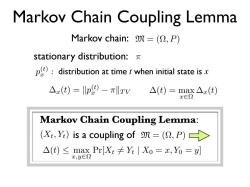
Markov Chain Coupling Lemma Markov chain:=(,P) stationary distribution: p):distribution at time t when initial state isx △z(t)=lp-πlrv △(t)=max△z(t) x∈2 Markov Chain Coupling Lemma: (X:,Yi)is a coupling of=(2,P) △(t)≤nax Pr[Xt≠Yt|Xo=x,Yo=y x,y∈2
Markov Chain Coupling Lemma Markov chain: M = (⌦, P) x(t) = kp(t) x ⇡kT V (t) = max x2⌦ x(t) stationary distribution: ⇡ p(t) x : distribution at time t when initial state is x (Xt, Yt) is a coupling of M = (⌦, P) (t) max x,y2⌦ Pr[Xt 6= Yt | X0 = x, Y0 = y] Markov Chain Coupling Lemma:
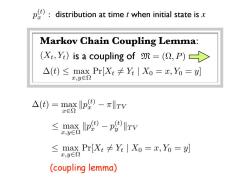
distribution at timewhen initial state isx Markov Chain Coupling Lemma: (X,Y)is a coupling of =(2,P) △(t)≤max Pr[Xt≠Y|Xo=x,Yo=y x,y∈2 △()=竖p-xy ≤max x,y∈2 llsv ≤max Pr[Xt卡Yt|Xo=x,Yo=y c,y∈2 (coupling lemma)
(Xt, Yt) is a coupling of M = (⌦, P) (t) max x,y2⌦ Pr[Xt 6= Yt | X0 = x, Y0 = y] Markov Chain Coupling Lemma: p(t) x : distribution at time t when initial state is x (t) = max x2⌦ kp(t) x ⇡kT V max x,y2⌦ kp(t) x p(t) y kT V max x,y2⌦ Pr[Xt 6= Yt | X0 = x, Y0 = y] (coupling lemma)
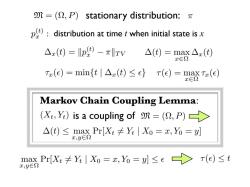
=(P)stationary distribution: p:distribution at time when initial state isx △x(t)=lp-πlTv △(t)=max△z(t) x∈2 Tr(e)=min{t|△x(t)≤}T(e)=max Ta(e) x∈2 Markov Chain Coupling Lemma: (X:,Y:)is a coupling of=(,P) △(t)≤max Pr[Xt≠YEXo=x,Yo= x,y∈2 max Pr[Xt≠Y|Xo=x,Y%=列≤e>1 T(e)≤t c,y∈2
(Xt, Yt) is a coupling of M = (⌦, P) (t) max x,y2⌦ Pr[Xt 6= Yt | X0 = x, Y0 = y] Markov Chain Coupling Lemma: max x,y2⌦ Pr[Xt 6= Yt | X0 = x, Y0 = y] ✏ ⌧ (✏) t M = (⌦, P) x(t) = kp(t) x ⇡kT V (t) = max x2⌦ x(t) ⌧x(✏) = min{t | x(t) ✏} ⌧ (✏) = max x2⌦ ⌧x(✏) stationary distribution: ⇡ p(t) x : distribution at time t when initial state is x
按次数下载不扣除下载券;
注册用户24小时内重复下载只扣除一次;
顺序:VIP每日次数-->可用次数-->下载券;
- 南京大学:《随机算法 Randomized Algorithms》课程教学资源(课件讲稿)Concentration.pdf
- 南京大学:《随机算法 Randomized Algorithms》课程教学资源(课件讲稿)Chernoff.pdf
- 南京大学:《随机算法 Randomized Algorithms》课程教学资源(课件讲稿)Balls and Bins.pdf
- 南京大学:《组合数学 Combinatorics》课程教学资源(课件讲稿)Ramsey Theory.pdf
- 南京大学:《组合数学 Combinatorics》课程教学资源(课件讲稿)The Probabilistic Method.pdf
- 南京大学:《组合数学 Combinatorics》课程教学资源(课件讲稿)Principle of Inclusion-Exclusion(PIE).pdf
- 南京大学:《组合数学 Combinatorics》课程教学资源(课件讲稿)Polya.pdf
- 南京大学:《组合数学 Combinatorics》课程教学资源(课件讲稿)Matching Theory.pdf
- 南京大学:《组合数学 Combinatorics》课程教学资源(课件讲稿)Generating Function.pdf
- 南京大学:《组合数学 Combinatorics》课程教学资源(课件讲稿)Extremal Sets.pdf
- 南京大学:《组合数学 Combinatorics》课程教学资源(课件讲稿)Extremal Combinatorics.pdf
- 南京大学:《组合数学 Combinatorics》课程教学资源(课件讲稿)Existence.pdf
- 南京大学:《组合数学 Combinatorics》课程教学资源(课件讲稿)Cayley.pdf
- 南京大学:《组合数学 Combinatorics》课程教学资源(课件讲稿)Basic Enumeration(主讲:尹一通).pdf
- 南京大学:《高级算法 Advanced Algorithms》课程教学资源(课件讲稿)Exercise Lecture For Advanced Algorithms(2022 Fall).pdf
- 南京大学:《高级算法 Advanced Algorithms》课程教学资源(课件讲稿)SDP-Based Algorithms.pdf
- 南京大学:《高级算法 Advanced Algorithms》课程教学资源(课件讲稿)Rounding Linear Program.pdf
- 南京大学:《高级算法 Advanced Algorithms》课程教学资源(课件讲稿)LP Duality.pdf
- 南京大学:《高级算法 Advanced Algorithms》课程教学资源(课件讲稿)Dimension Reduction.pdf
- 南京大学:《高级算法 Advanced Algorithms》课程教学资源(课件讲稿)Rounding Data.pdf
- 南京大学:《随机算法 Randomized Algorithms》课程教学资源(课件讲稿)Finger printing.pdf
- 南京大学:《随机算法 Randomized Algorithms》课程教学资源(课件讲稿)Identity Testing.pdf
- 南京大学:《随机算法 Randomized Algorithms》课程教学资源(课件讲稿)Lovász Local Lemma.pdf
- 南京大学:《随机算法 Randomized Algorithms》课程教学资源(课件讲稿)Markov Chain.pdf
- 南京大学:《随机算法 Randomized Algorithms》课程教学资源(课件讲稿)Min-Cut.pdf
- 南京大学:《随机算法 Randomized Algorithms》课程教学资源(课件讲稿)Mixing.pdf
- 南京大学:《随机算法 Randomized Algorithms》课程教学资源(课件讲稿)Moments.pdf
- 南京大学:《随机算法 Randomized Algorithms》课程教学资源(课件讲稿)Random Rounding.pdf
- 南京大学:《随机算法 Randomized Algorithms》课程教学资源(课件讲稿)Universal Hashing.pdf
- 电子科技大学:《嵌入式系统设计 Embedded Systems Design》课程教学资源(课件讲稿)Chapter 1 Overview(廖勇).pdf
- 电子科技大学:《嵌入式系统设计 Embedded Systems Design》课程教学资源(课件讲稿)Chapter 2 Hardware System.pdf
- 电子科技大学:《嵌入式系统设计 Embedded Systems Design》课程教学资源(课件讲稿)Chapter 3 Software System.pdf
- 电子科技大学:《嵌入式系统设计 Embedded Systems Design》课程教学资源(课件讲稿)Chapter 4 Task Management.pdf
- 电子科技大学:《嵌入式系统设计 Embedded Systems Design》课程教学资源(课件讲稿)Chapter 5 ask Management.pdf
- 电子科技大学:《嵌入式系统设计 Embedded Systems Design》课程教学资源(课件讲稿)Case Analysis - Use DARTS to Design a S/W System of Robot Controller.pdf
- 电子科技大学:《嵌入式系统设计 Embedded Systems Design》课程教学资源(课件讲稿)Case 4.pdf
- 电子科技大学:《嵌入式系统设计 Embedded Systems Design》课程教学资源(课件讲稿)Chapter 3 Hot topics in ES.pdf
- 中国计算机学会学术著作丛书:《对等网络——结构、应用与设计 Peer-to-Peer Network Structure, Application and Design》PDF电子书(正文,共九章).pdf
- 《计算机科学》相关教学资源(参考文献)Dynamic inference in probabilistic graphical models.pdf
- 《计算机科学》相关教学资源(参考文献)Dynamic Sampling from Graphical Models.pdf