南京大学:《随机算法 Randomized Algorithms》课程教学资源(课件讲稿)Lovász Local Lemma
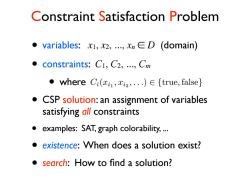
Constraint Satisfaction Problem ●variables:x1,x2,,xn∈D(domain) constraints:C1,C2,....Cm ●where C(ri,ri2,)∈{true,false} CSP solution:an assignment of variables satisfying all constraints examples:SAT,graph colorability,... existence:When does a solution exist? search:How to find a solution?
Constraint Satisfaction Problem • variables: x1, x2, ..., xn ∈ D (domain) • constraints: C1, C2, ..., Cm • where • CSP solution: an assignment of variables satisfying all constraints • examples: SAT, graph colorability, ... • existence: When does a solution exist? • search: How to find a solution? Ci(xi1 , xi2 ,...) 2 {true, false}
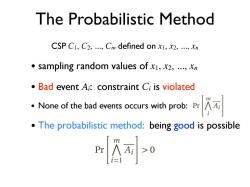
The Probabilistic Method CSP C1,C2,...Cm defined onx1,x2....xn sampling random values ofx1,x2,..., Bad event Ai:constraint Ci is violated None of the ad troPr The probabilistic method:being good is possible >0 Pr i=1
The Probabilistic Method • sampling random values of x1, x2, ..., xn • Bad event Ai: constraint Ci is violated • None of the bad events occurs with prob: • The probabilistic method: being good is possible Pr" ^ m i Ai # Pr" ^ m i=1 Ai # > 0 CSP C1, C2, ..., Cm defined on x1, x2, ..., xn
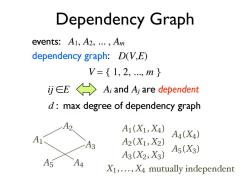
Dependency Graph events:A1,A2,...,Am dependency graph:D(V,E) V={1,2,,m} 访∈E> Ai and Aj are dependent d:max degree of dependency graph A2 A1(X1,X4) A1 A4(X4) A3 A2(X1,X2) A5(X3) A3(X2,X3) A5 A4 X1,...,X4 mutually independent
A1 A2 A3 A5 A4 X1,...,X4 mutually independent A1(X1,X4) A2(X1,X2) A3(X2,X3) A4(X4) A5(X3) events: A1, A2, ... , Am d : max degree of dependency graph dependency graph: D(V,E) V = { 1, 2, ..., m } ij ∈E Ai and Aj are dependent Dependency Graph
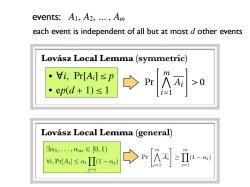
events:A1,A2,...,Am each event is independent of all but at most d other events Lovasz Local Lemma(symmetric) 。Vi,PrLA≤p Pr >0 ep(d+1)≤1 i=】 Lovasz Local Lemma(general) 3a1,..,am∈[0,1) i,PrA≤aaI1-a) →m公 i三1 j心i
events: A1, A2, ... , Am each event is independent of all but at most d other events 9↵1,..., ↵m 2 [0, 1) 8i,Pr[Ai] ↵i Y j⇠i (1 ↵j ) Pr " ^ m i=1 Ai # Y m i=1 (1 ↵i) Lovász Local Lemma (general) • ∀i, Pr[Ai] ≤ p • ep(d + 1) ≤ 1 Pr" ^ m i=1 Ai # > 0 Lovász Local Lemma (symmetric)
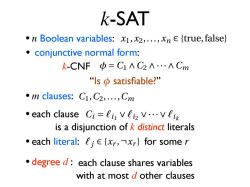
k-SAT .n Boolean variables:x1,x2,...,xnEtrue,false} conjunctive normal form: k-CNFΦ=C1∧C2∧·∧Cm “ils satisfiable?' ·n clauses::Cl,C2,,Cm .each clause Ci=iveiv...vli is a disjunction of k distinct literals ·each literal::lj∈{xr,xr}for some r degree d:each clause shares variables with at most d other clauses
k-SAT • n Boolean variables: x1,x2,...,xn 2 {true, false} • m clauses: C1,C2,...,Cm • each clause Ci = `i1 _`i2 _···_`ik • each literal: for some r • degree d : is a disjunction of k distinct literals `j 2 {xr ,¬xr } each clause shares variables with at most d other clauses k-CNF ¡ = C1 ^C2 ^···^Cm • conjunctive normal form: “Is φ satisfiable?
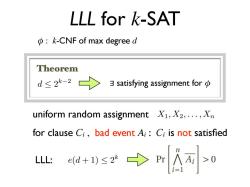
LLL for k-SAT k-CNF of max degree d Theorem d≤2k-2 > 3 satisfying assignment for uniform random assignment X1,X2,...,Xn for clause Ci,bad event Ai:Ci is not satisfied LLL: e(d+1)≤2kE >0
Theorem d 2k2 ∃ satisfying assignment for φ e(d + 1) 2k for clause Ci , bad event Ai : uniform random assignment X1, X2,...,Xn Ci is not satisfied φ : k-CNF of max degree d LLL: Pr ⇤ n i=1 Ai ⇥ > 0 LLL for k-SAT
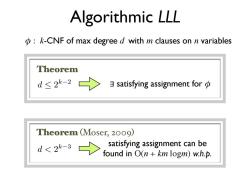
Algorithmic LLL k-CNF of max degree d with m clauses on n variables Theorem d≤2k-2 3 satisfying assignment for Theorem (Moser,2009) d<2k-3[ 〉 satisfying assignment can be found in O(n+km logm)w.h.p
satisfying assignment can be found in O(n + km logm) w.h.p. ∃ satisfying assignment for φ Algorithmic LLL Theorem d 2k2 Theorem (Moser, 2009) d < 2k3 φ : k-CNF of max degree d with m clauses on n variables
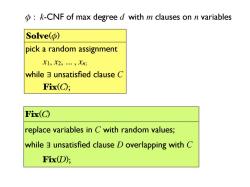
k-CNF of max degree d with m clauses on n variables Sove(Φ) pick a random assignment X1,X2,…,X; while 3 unsatisfied clause C Fix(C); Fix(C) replace variables in C with random values; while 3 unsatisfied clause D overlapping with C Fix(D);
Solve(φ) pick a random assignment x1, x2, ... , xn; while ∃ unsatisfied clause C Fix(C); Fix(C) replace variables in C with random values; while ∃ unsatisfied clause D overlapping with C Fix(D); φ : k-CNF of max degree d with m clauses on n variables
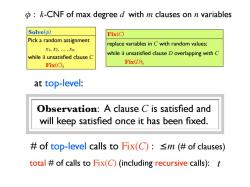
k-CNF of max degree d with mn clauses on n variables Solve() Fix(C) Pick a random assignment replace variables in C with random values; X1,x2,…,X: while 3 unsatisfied clause C while 3 unsatisfied clause D overlapping with C Fix(C); Fix(D); at top-level: Observation:A clause C is satisfied and will keep satisfied once it has been fixed. of top-level calls to Fix(C)<m(#of clauses) total of calls to Fix(C)(including recursive calls):t
Solve(φ) Pick a random assignment x1, x2, ... , xn; while ∃ unsatisfied clause C Fix(C); Fix(C) replace variables in C with random values; while ∃ unsatisfied clause D overlapping with C Fix(D); at top-level: Observation: A clause C is satisfied and will keep satisfied once it has been fixed. # of top-level calls to Fix(C) : ≤m (# of clauses) total # of calls to Fix(C) (including recursive calls): t φ : k-CNF of max degree d with m clauses on n variables
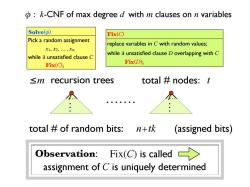
k-CNF of max degree d with m clauses on n variables Solve() Fix(C) Pick a random assignment replace variables in C with random values; X1,X2,…,X while 3 unsatisfied clause D overlapping with C while 3 unsatisfied clause C Fix(C); Fix(D); ≤n recursion trees total nodes:t total of random bits: n+tk (assigned bits) Observation: Fix(C)is called assignment of C is uniquely determined
≤m total # nodes: t Solve(φ) Pick a random assignment x1, x2, ... , xn; while ∃ unsatisfied clause C Fix(C); Fix(C) replace variables in C with random values; while ∃ unsatisfied clause D overlapping with C Fix(D); recursion trees total # of random bits: n+tk (assigned bits) Observation: Fix(C) is called assignment of C is uniquely determined φ : k-CNF of max degree d with m clauses on n variables
按次数下载不扣除下载券;
注册用户24小时内重复下载只扣除一次;
顺序:VIP每日次数-->可用次数-->下载券;
- 南京大学:《随机算法 Randomized Algorithms》课程教学资源(课件讲稿)Identity Testing.pdf
- 南京大学:《随机算法 Randomized Algorithms》课程教学资源(课件讲稿)Finger printing.pdf
- 南京大学:《随机算法 Randomized Algorithms》课程教学资源(课件讲稿)Coupling.pdf
- 南京大学:《随机算法 Randomized Algorithms》课程教学资源(课件讲稿)Concentration.pdf
- 南京大学:《随机算法 Randomized Algorithms》课程教学资源(课件讲稿)Chernoff.pdf
- 南京大学:《随机算法 Randomized Algorithms》课程教学资源(课件讲稿)Balls and Bins.pdf
- 南京大学:《组合数学 Combinatorics》课程教学资源(课件讲稿)Ramsey Theory.pdf
- 南京大学:《组合数学 Combinatorics》课程教学资源(课件讲稿)The Probabilistic Method.pdf
- 南京大学:《组合数学 Combinatorics》课程教学资源(课件讲稿)Principle of Inclusion-Exclusion(PIE).pdf
- 南京大学:《组合数学 Combinatorics》课程教学资源(课件讲稿)Polya.pdf
- 南京大学:《组合数学 Combinatorics》课程教学资源(课件讲稿)Matching Theory.pdf
- 南京大学:《组合数学 Combinatorics》课程教学资源(课件讲稿)Generating Function.pdf
- 南京大学:《组合数学 Combinatorics》课程教学资源(课件讲稿)Extremal Sets.pdf
- 南京大学:《组合数学 Combinatorics》课程教学资源(课件讲稿)Extremal Combinatorics.pdf
- 南京大学:《组合数学 Combinatorics》课程教学资源(课件讲稿)Existence.pdf
- 南京大学:《组合数学 Combinatorics》课程教学资源(课件讲稿)Cayley.pdf
- 南京大学:《组合数学 Combinatorics》课程教学资源(课件讲稿)Basic Enumeration(主讲:尹一通).pdf
- 南京大学:《高级算法 Advanced Algorithms》课程教学资源(课件讲稿)Exercise Lecture For Advanced Algorithms(2022 Fall).pdf
- 南京大学:《高级算法 Advanced Algorithms》课程教学资源(课件讲稿)SDP-Based Algorithms.pdf
- 南京大学:《高级算法 Advanced Algorithms》课程教学资源(课件讲稿)Rounding Linear Program.pdf
- 南京大学:《随机算法 Randomized Algorithms》课程教学资源(课件讲稿)Markov Chain.pdf
- 南京大学:《随机算法 Randomized Algorithms》课程教学资源(课件讲稿)Min-Cut.pdf
- 南京大学:《随机算法 Randomized Algorithms》课程教学资源(课件讲稿)Mixing.pdf
- 南京大学:《随机算法 Randomized Algorithms》课程教学资源(课件讲稿)Moments.pdf
- 南京大学:《随机算法 Randomized Algorithms》课程教学资源(课件讲稿)Random Rounding.pdf
- 南京大学:《随机算法 Randomized Algorithms》课程教学资源(课件讲稿)Universal Hashing.pdf
- 电子科技大学:《嵌入式系统设计 Embedded Systems Design》课程教学资源(课件讲稿)Chapter 1 Overview(廖勇).pdf
- 电子科技大学:《嵌入式系统设计 Embedded Systems Design》课程教学资源(课件讲稿)Chapter 2 Hardware System.pdf
- 电子科技大学:《嵌入式系统设计 Embedded Systems Design》课程教学资源(课件讲稿)Chapter 3 Software System.pdf
- 电子科技大学:《嵌入式系统设计 Embedded Systems Design》课程教学资源(课件讲稿)Chapter 4 Task Management.pdf
- 电子科技大学:《嵌入式系统设计 Embedded Systems Design》课程教学资源(课件讲稿)Chapter 5 ask Management.pdf
- 电子科技大学:《嵌入式系统设计 Embedded Systems Design》课程教学资源(课件讲稿)Case Analysis - Use DARTS to Design a S/W System of Robot Controller.pdf
- 电子科技大学:《嵌入式系统设计 Embedded Systems Design》课程教学资源(课件讲稿)Case 4.pdf
- 电子科技大学:《嵌入式系统设计 Embedded Systems Design》课程教学资源(课件讲稿)Chapter 3 Hot topics in ES.pdf
- 中国计算机学会学术著作丛书:《对等网络——结构、应用与设计 Peer-to-Peer Network Structure, Application and Design》PDF电子书(正文,共九章).pdf
- 《计算机科学》相关教学资源(参考文献)Dynamic inference in probabilistic graphical models.pdf
- 《计算机科学》相关教学资源(参考文献)Dynamic Sampling from Graphical Models.pdf
- 《计算机科学》相关教学资源(参考文献)On Local Distributed Sampling and Counting.pdf
- 《计算机科学》相关教学资源(参考文献)What can be sampled locally?.pdf
- 《计算机科学》相关教学资源(参考文献)Convergence of MCMC and Loopy BP in the Tree Uniqueness Region for the Hard-Core Model.pdf