南京大学:《随机算法 Randomized Algorithms》课程教学资源(课件讲稿)Concentration
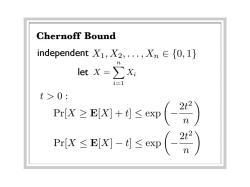
Chernoff Bound independent X1,X2,...,XnE 10,1} IetX=∑X i=1 t>0: PX≥町N8s即() PrX≤EX]-t≤exp
X = X n i=1 Xi independent X1, X2,...,Xn 2 {0, 1} Chernoff Bound t > 0 : Pr[X E[X] + t] exp ✓ 2t 2 n ◆ Pr[X E[X] t] exp ✓ 2t 2 n ◆ let
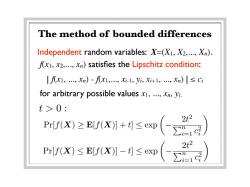
The method of bounded differences Independent random variables:X-(X1,X2,...,X). 1,x2..,)satisfies the Lipschitz condition: |jx1,,n)-fx1,,xi1,yi,Xi+1,,xn)|≤Ci for arbitrary possible valuesx1,...,xn,yi. t>0: x>grxyfse( 2t2 Pr[f(X)≤E[f(X)】-t)≤exp
Independent random variables: X=(X1, X2,..., Xn). The method of bounded differences f(x1, x2,..., xn) satisfies the Lipschitz condition: | f(x1, ..., xn) - f(x1,..., xi-1, yi, xi+1, ..., xn) | ≤ ci for arbitrary possible values x1, ..., xn, yi. t > 0 : Pr[f(X) E[f(X)] t] exp ✓ 2t 2 Pn i=1 c2 i ◆ Pr[f(X) E[f(X)] + t] exp ✓ 2t 2 Pn i=1 c2 i ◆
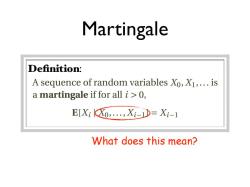
Martingale Definition: A sequence of random variables Xo,X1,...is a martingale if for all i>0, E[Xi KXo,...,Xi=Xi-1 What does this mean?
E[Xi | X0,...,Xi⇥1] = Xi⇥1 Definition: A sequence of random variables X0,X1,... is a martingale if for all i > 0, Martingale What does this mean?
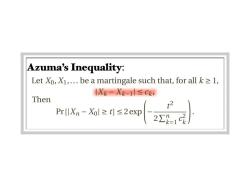
Azuma's Inequality: Let Xo,X1,...be a martingale such that,for all k =1, IXk-Xk-1≤Ck, Then 12 Pr[lXn-Xol≥t]≤2exp 2∑K=1c呢
Azuma’s Inequality: Let X0,X1,... be a martingale such that, for all k 1, |Xk ⇥ Xk⇥1| ⇤ ck , Then Pr[|Xn ⇥ X0| ⌅ t] ⇤ 2 exp⇤ ⇥ t 2 2 ⇥n k=1 c2 k
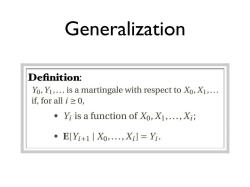
Generalization Definition: Yo,Y1,...is a martingale with respect to Xo,X1,... if,for all i≥0, Yi is a function of Xo,X1,...,Xi; 。E[Yi+1|X0,.,Xi]=Yi
Generalization Definition: Y0,Y1,... is a martingale with respect to X0,X1,... if, for all i 0, • Yi is a function of X0,X1,...,Xi ; • E[Yi+1 | X0,...,Xi] = Yi
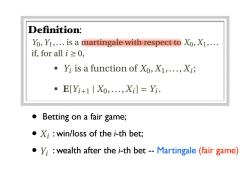
Definition: Yo,Y1,...is a martingale with respect to Xo,X1,... if,for all i≥0, .Yi is a function of Xo,X1,...,Xi; .E[Yi+1 Xo,...,Xi]Yi. Betting on a fair game; Xi:win/loss of the i-th bet; Yi wealth after the i-th bet--Martingale(fair game)
Definition: Y0,Y1,... is a martingale with respect to X0,X1,... if, for all i 0, • Yi is a function of X0,X1,...,Xi ; • E[Yi+1 | X0,...,Xi] = Yi . • Betting on a fair game; • : win/loss of the i-th bet; • : wealth after the i-th bet -- Martingale (fair game) Xi Yi
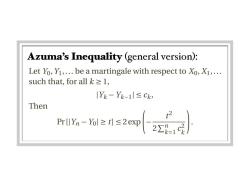
Azuma's Inequality (general version): Let Yo,Y1,...be a martingale with respect to Xo,X1,... such that,,for all k≥l, IYk-Yk-1≤Ck, Then Pr[lYn-Yol≥t]≤2exp 2∑1c
Azuma’s Inequality (general version): Then Let Y0,Y1,... be a martingale with respect to X0,X1,... such that, for all k 1, |Yk ⇥Yk⇥1| ⇤ ck , Pr[|Yn ⇥Y0| ⌅ t] ⇤ 2 exp⇤ ⇥ t 2 2 ⇥n k=1 c2 k
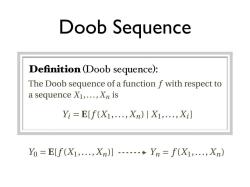
Doob Sequence Definition (Doob sequence): The Doob sequence of a function f with respect to a sequence X1,...,Xn is Yi=E[f(X1,...,Xn)X1,...,Xi] Yo=E[f(X1,.…,Xn)】->Yn=f(X1,.,Xn)
Definition (Doob sequence): Yi = E[f (X1,...,Xn) | X1,...,Xi] The Doob sequence of a function f with respect to a sequence X1,...,Xn is Y0 = E[f (X1,...,Xn)] Yn = f (X1,...,Xn) Doob Sequence
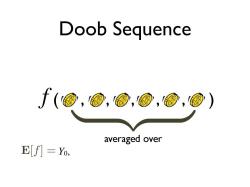
Doob Sequence @, @,@, averaged over E f=Yo
f ( , , , , , ) averaged over Doob Sequence E[f] = Y0
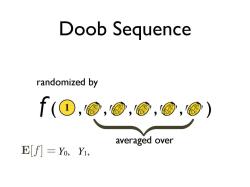
Doob Sequence randomized by f(①,@, @, averaged over E[f月=Yo,Y
1 f ( , , , , , ) randomized by averaged over Doob Sequence E[f] = Y0, Y1
按次数下载不扣除下载券;
注册用户24小时内重复下载只扣除一次;
顺序:VIP每日次数-->可用次数-->下载券;
- 南京大学:《随机算法 Randomized Algorithms》课程教学资源(课件讲稿)Chernoff.pdf
- 南京大学:《随机算法 Randomized Algorithms》课程教学资源(课件讲稿)Balls and Bins.pdf
- 南京大学:《组合数学 Combinatorics》课程教学资源(课件讲稿)Ramsey Theory.pdf
- 南京大学:《组合数学 Combinatorics》课程教学资源(课件讲稿)The Probabilistic Method.pdf
- 南京大学:《组合数学 Combinatorics》课程教学资源(课件讲稿)Principle of Inclusion-Exclusion(PIE).pdf
- 南京大学:《组合数学 Combinatorics》课程教学资源(课件讲稿)Polya.pdf
- 南京大学:《组合数学 Combinatorics》课程教学资源(课件讲稿)Matching Theory.pdf
- 南京大学:《组合数学 Combinatorics》课程教学资源(课件讲稿)Generating Function.pdf
- 南京大学:《组合数学 Combinatorics》课程教学资源(课件讲稿)Extremal Sets.pdf
- 南京大学:《组合数学 Combinatorics》课程教学资源(课件讲稿)Extremal Combinatorics.pdf
- 南京大学:《组合数学 Combinatorics》课程教学资源(课件讲稿)Existence.pdf
- 南京大学:《组合数学 Combinatorics》课程教学资源(课件讲稿)Cayley.pdf
- 南京大学:《组合数学 Combinatorics》课程教学资源(课件讲稿)Basic Enumeration(主讲:尹一通).pdf
- 南京大学:《高级算法 Advanced Algorithms》课程教学资源(课件讲稿)Exercise Lecture For Advanced Algorithms(2022 Fall).pdf
- 南京大学:《高级算法 Advanced Algorithms》课程教学资源(课件讲稿)SDP-Based Algorithms.pdf
- 南京大学:《高级算法 Advanced Algorithms》课程教学资源(课件讲稿)Rounding Linear Program.pdf
- 南京大学:《高级算法 Advanced Algorithms》课程教学资源(课件讲稿)LP Duality.pdf
- 南京大学:《高级算法 Advanced Algorithms》课程教学资源(课件讲稿)Dimension Reduction.pdf
- 南京大学:《高级算法 Advanced Algorithms》课程教学资源(课件讲稿)Rounding Data.pdf
- 南京大学:《高级算法 Advanced Algorithms》课程教学资源(课件讲稿)Lovász Local Lemma.pdf
- 南京大学:《随机算法 Randomized Algorithms》课程教学资源(课件讲稿)Coupling.pdf
- 南京大学:《随机算法 Randomized Algorithms》课程教学资源(课件讲稿)Finger printing.pdf
- 南京大学:《随机算法 Randomized Algorithms》课程教学资源(课件讲稿)Identity Testing.pdf
- 南京大学:《随机算法 Randomized Algorithms》课程教学资源(课件讲稿)Lovász Local Lemma.pdf
- 南京大学:《随机算法 Randomized Algorithms》课程教学资源(课件讲稿)Markov Chain.pdf
- 南京大学:《随机算法 Randomized Algorithms》课程教学资源(课件讲稿)Min-Cut.pdf
- 南京大学:《随机算法 Randomized Algorithms》课程教学资源(课件讲稿)Mixing.pdf
- 南京大学:《随机算法 Randomized Algorithms》课程教学资源(课件讲稿)Moments.pdf
- 南京大学:《随机算法 Randomized Algorithms》课程教学资源(课件讲稿)Random Rounding.pdf
- 南京大学:《随机算法 Randomized Algorithms》课程教学资源(课件讲稿)Universal Hashing.pdf
- 电子科技大学:《嵌入式系统设计 Embedded Systems Design》课程教学资源(课件讲稿)Chapter 1 Overview(廖勇).pdf
- 电子科技大学:《嵌入式系统设计 Embedded Systems Design》课程教学资源(课件讲稿)Chapter 2 Hardware System.pdf
- 电子科技大学:《嵌入式系统设计 Embedded Systems Design》课程教学资源(课件讲稿)Chapter 3 Software System.pdf
- 电子科技大学:《嵌入式系统设计 Embedded Systems Design》课程教学资源(课件讲稿)Chapter 4 Task Management.pdf
- 电子科技大学:《嵌入式系统设计 Embedded Systems Design》课程教学资源(课件讲稿)Chapter 5 ask Management.pdf
- 电子科技大学:《嵌入式系统设计 Embedded Systems Design》课程教学资源(课件讲稿)Case Analysis - Use DARTS to Design a S/W System of Robot Controller.pdf
- 电子科技大学:《嵌入式系统设计 Embedded Systems Design》课程教学资源(课件讲稿)Case 4.pdf
- 电子科技大学:《嵌入式系统设计 Embedded Systems Design》课程教学资源(课件讲稿)Chapter 3 Hot topics in ES.pdf
- 中国计算机学会学术著作丛书:《对等网络——结构、应用与设计 Peer-to-Peer Network Structure, Application and Design》PDF电子书(正文,共九章).pdf
- 《计算机科学》相关教学资源(参考文献)Dynamic inference in probabilistic graphical models.pdf