广东工业大学:《机器学习》课程教学资源(课件讲义)第15讲 无监督学习——降维深度学习可视化(PCA Kmeans)

Unsupervised Learning: Principle Component Analysis
Unsupervised Learning: Principle Component Analysis
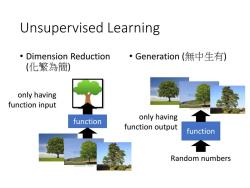
Unsupervised Learning ● Dimension Reduction ·Generation(無中生有) (化繁為簡) only having function input function only having function output function Random numbers
Unsupervised Learning • Dimension Reduction (化繁為簡) • Generation (無中生有) function function Random numbers only having function input only having function output
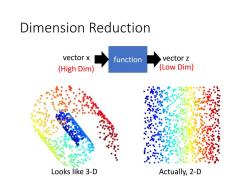
Dimension Reduction vector x function vector z (High Dim) (Low Dim) Looks like 3-D Actually,2-D
Dimension Reduction Looks like 3-D Actually, 2-D function vector x vector z (High Dim) (Low Dim)
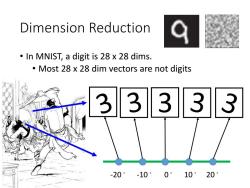
Dimension Reduction In MNIST,a digit is 28 x 28 dims. Most 28 x 28 dim vectors are not digits 3 333 -20°-10° 0°10°20°
Dimension Reduction • In MNIST, a digit is 28 x 28 dims. • Most 28 x 28 dim vectors are not digits 3 -20。 -10。 0。 10。 20
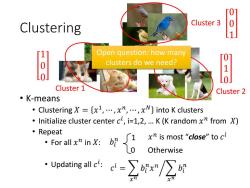
0 Clustering Cluster 3 0 1 Open question:how many 8 clusters do we need? Cluster 1 Cluster 2 。K-means ·Clustering X={xl,…,xn,,xN}into K clusters Initialize cluster center cl,i=1,2,...K (K random x from X) 。Repeat nX好0 x is most "close"to ci Otherwise ·Updating4c=∑oix/∑时
Clustering • K-means • Clustering 𝑋 = 𝑥 1 , ⋯ , 𝑥 𝑛 , ⋯ , 𝑥 𝑁 into K clusters • Initialize cluster center 𝑐 𝑖 , i=1,2, … K (K random 𝑥 𝑛 from 𝑋) • Repeat • For all 𝑥 𝑛 in 𝑋: • Updating all 𝑐 𝑖 : Cluster 1 Cluster 2 Cluster 3 Open question: how many clusters do we need? 𝑏𝑖 𝑛 0 1 𝑐 𝑖 = ൘ 𝑥 𝑛 𝑏𝑖 𝑛 𝑥 𝑛 𝑥 𝑛 𝑏𝑖 𝑛 Otherwise 𝑥 𝑛 is most “close” to 𝑐 𝑖 1 0 0 0 1 0 0 0 1
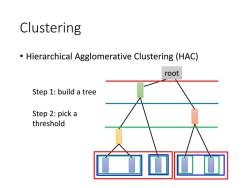
Clustering Hierarchical Agglomerative Clustering (HAC) root Step 1:build a tree Step 2:pick a threshold
Clustering • Hierarchical Agglomerative Clustering (HAC) Step 1: build a tree Step 2: pick a threshold root

Distributed Representation 強化系 Clustering:an object must 放出系 belong to one cluster 贵化系 發 小傑是強化系 具現化系 Distributed representation 操作系 特質系 強化系 0.70 放出系 0.25 變化系 強 0.05 者強自 他 小傑是 化系能力 操作系 0.00 具現化系 0.00 特質系 0.00
Distributed Representation • Clustering: an object must belong to one cluster • Distributed representation 小傑是強化系 小傑是 強化系 0.70 放出系 0.25 變化系 0.05 操作系 0.00 具現化系 0.00 特質系 0.00
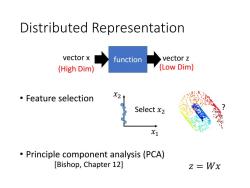
Distributed Representation vector x function vector z (High Dim) (Low Dim) 。Feature selection X2 Select x2 X1 Principle component analysis (PCA) [Bishop,Chapter 12] z=Wx
Distributed Representation • Feature selection • Principle component analysis (PCA) ? 𝑥1 𝑥2 Select 𝑥2 [Bishop, Chapter 12] 𝑧 = 𝑊𝑥 function vector x vector z (High Dim) (Low Dim)
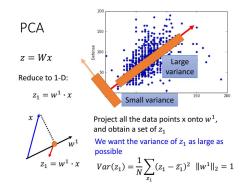
200 PCA 150 100 z=Wx Large So variance Reduce to 1-D: 1=w1·x 150 200 Small variance Project all the data points x onto w1, and obtain a set of Z1 We want the variance of z1 as large as possible =w1·x are)-∑a-we=1
PCA 𝑤1 𝑥 Project all the data points x onto 𝑤1 , and obtain a set of 𝑧1 We want the variance of 𝑧1 as large as possible Large variance Small variance 𝑉𝑎𝑟 𝑧1 = 1 𝑁 𝑧1 𝑧1 − 𝑧ഥ1 2 𝑧 = 𝑊𝑥 𝑧1 = 𝑤1 ∙ 𝑥 𝑧1 = 𝑤1 ∙ 𝑥 Reduce to 1-D: 𝑤1 2 = 1

PCA Project all the data points x onto w1, and obtain a set of z1 z=Wx We want the variance of z1 as large as possible Reduce to 1-D: Vare)=∑a-a2w2=1 Z1=w1·x Z1 Z2 w2.x We want the variance of z2 as large as [(w1)T possible W = (w2)T var(z)=∑a,-a2w2l2=1 w1w2=0 Orthogonal matrix
PCA We want the variance of 𝑧2 as large as possible 𝑉𝑎𝑟 𝑧2 = 1 𝑁 𝑧2 𝑧2 − 𝑧ഥ2 2 𝑤2 2 = 1 𝑤1 ∙ 𝑤2 = 0 𝑧 = 𝑊𝑥 𝑧1 = 𝑤1 ∙ 𝑥 Reduce to 1-D: Project all the data points x onto 𝑤1 , and obtain a set of 𝑧1 We want the variance of 𝑧1 as large as possible 𝑉𝑎𝑟 𝑧1 = 1 𝑁 𝑧1 𝑧1 − 𝑧ഥ1 2 𝑤1 2 = 1 𝑧2 = 𝑤2 ∙ 𝑥 𝑊 = 𝑤1 𝑇 𝑤2 𝑇 ⋮ Orthogonal matrix
按次数下载不扣除下载券;
注册用户24小时内重复下载只扣除一次;
顺序:VIP每日次数-->可用次数-->下载券;
- 广东工业大学:《机器学习》课程教学资源(课件讲义)第14讲 循环神经网络(RNN).pdf
- 广东工业大学:《机器学习》课程教学资源(课件讲义)第13讲 卷积神经网络计算机视觉应用(目标检测,计算机视觉训练技巧).pdf
- 广东工业大学:《机器学习》课程教学资源(课件讲义)第13讲 卷积神经网络计算机视觉应用(Inception, 批量归一化和残差网络ResNet).pdf
- 广东工业大学:《机器学习》课程教学资源(课件讲义)第12讲 卷积神经网络(LeNet, AlexNet, VGG和NiN).pdf
- 广东工业大学:《机器学习》课程教学资源(课件讲义)第12讲 卷积神经网络(卷积和池化层).pdf
- 广东工业大学:《机器学习》课程教学资源(课件讲义)第11讲 感知机模型与多层感知机(前馈神经网络,DNN BP).pdf
- 广东工业大学:《机器学习》课程教学资源(课件讲义)第10讲 神经网络的优化(激活函数 dropout).pdf
- 广东工业大学:《机器学习》课程教学资源(课件讲义)第10讲 神经网络的优化(梯度消失和梯度爆炸BN).pdf
- 广东工业大学:《机器学习》课程教学资源(课件讲义)第10讲 神经网络的优化(自适应学习率 AdaGrad RMSProp).pdf
- 广东工业大学:《机器学习》课程教学资源(课件讲义)第10讲 神经网络的优化(batch和动量Momentum NAG).pdf
- 广东工业大学:《机器学习》课程教学资源(课件讲义)第9讲 神经网络的优化(梯度下降、学习率adagrad adam、随机梯度下降、特征缩放).pdf
- 广东工业大学:《机器学习》课程教学资源(课件讲义)第9讲 神经网络的优化(损失函数).pdf
- 广东工业大学:《机器学习》课程教学资源(课件讲义)第8讲 集成学习(决策树的演化).pdf
- 广东工业大学:《机器学习》课程教学资源(课件讲义)第7讲 集成学习(决策树).pdf
- 广东工业大学:《机器学习》课程教学资源(课件讲义)第6讲 线性回归模型及其求解方法 Linear Regression Model and Its Solution.pdf
- 广东工业大学:《机器学习》课程教学资源(课件讲义)第5讲 分类问题(4.4 朴素?叶斯分类器).pdf
- 广东工业大学:《机器学习》课程教学资源(课件讲义)第5讲 分类问题(4.3 ?持向量机 SVM).pdf
- 广东工业大学:《机器学习》课程教学资源(课件讲义)第4讲 分类问题(4.1 分类与回归问题概述 4.2 分类性能度量?法).pdf
- 广东工业大学:《机器学习》课程教学资源(课件讲义)第3讲 特征工程 Feature Engineering.pdf
- 广东工业大学:《机器学习》课程教学资源(课件讲义)第2讲 模型评估与选择.pdf
- 广东工业大学:《机器学习》课程教学资源(课件讲义)第15讲 无监督学习——降维深度学习可视化(Neighbor Embedding,LLE T-SNE).pdf
- 广东工业大学:《机器学习》课程教学资源(PPT讲稿)第16讲 现代循环神经网络(高级循环神经网络).pptx
- 广东工业大学:《机器学习》课程教学资源(PPT讲稿)第16讲 现代循环神经网络(编码器解码器,Seq2seq模型,束搜索).pptx
- 广东工业大学:《机器学习》课程教学资源(PPT讲稿)第16讲 现代循环神经网络(嵌入向量, 词嵌入, 子词嵌入, 全局向量的词嵌入).pptx
- 广东工业大学:《机器学习》课程教学资源(PPT讲稿)第17讲 注意力机制(概述).pptx
- 广东工业大学:《机器学习》课程教学资源(课件讲义)第17讲 注意力机制(自注意力).pdf
- 广东工业大学:《机器学习》课程教学资源(PPT讲稿)第18讲 变换器模型 Transformer.pptx
- 广东工业大学:《机器学习》课程教学资源(课件讲义)第18讲 变换器模型 Transformer.pdf
- 广东工业大学:《机器学习》课程教学资源(课件讲义)第19讲 ViT及注意力机制改进(Vision Transformers ,ViTs).pdf
- 广东工业大学:《机器学习》课程教学资源(课件讲义)第19讲 ViT及注意力机制改进(各式各样的Attention).pdf
- 广东工业大学:《机器学习》课程教学资源(课件讲义)第20讲 预训练模型 Pre-training of Deep Bidirectional Transformers for Language Understanding(授课:周郭许).pdf
- 广东工业大学:《机器学习》课程教学资源(课件讲义)第21讲 生成式网络模型(自编码器 Deep Auto-encoder).pdf
- 广东工业大学:《机器学习》课程教学资源(课件讲义)第21讲 生成式网络模型(VAE Generation).pdf
- 广东工业大学:《机器学习》课程教学资源(课件讲义)第22讲 生成式网络模型(Diffusion Model).pdf
- 广东工业大学:《机器学习》课程教学资源(课件讲义)第22讲 生成式网络模型(Stable Diffusion).pdf
- 北京信息科技大学:计算机学院各专业课程教学大纲汇编.pdf
- 北京信息科技大学:计算中心及图书馆课程教学大纲汇编.pdf
- 新乡学院:数学与统计学院信息与计算科学专业《数学分析Ⅰ》课程教学大纲(2015).pdf
- 新乡学院:数学与统计学院信息与计算科学专业《数学分析Ⅱ》课程教学大纲(2015).pdf
- 新乡学院:数学与统计学院信息与计算科学专业《数学分析Ⅲ》课程教学大纲(2015).pdf