广东工业大学:《机器学习》课程教学资源(课件讲义)第19讲 ViT及注意力机制改进(Vision Transformers ,ViTs)
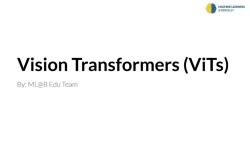
MACHINE LEARNING BERKELEY Vision Transformers (ViTs) By:ML@B Edu Team
Vision Transformers (ViTs) By: ML@B Edu Team
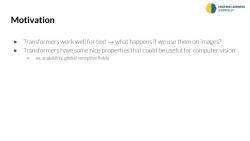
MACHINE LEARNING BERKELEY Motivation Transformers work well for text-what happens if we use them on images? Transformers have some nice properties that could be useful for computer vision o ex.scalability.global receptive fields
Motivation ● Transformers work well for text → what happens if we use them on images? ● Transformers have some nice properties that could be useful for computer vision ○ ex. scalability, global receptive fields
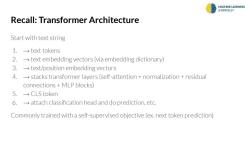
MACHINE LEARNING BERKELEY Recall:Transformer Architecture Start with text string 1.→text tokens 2.-text embedding vectors(via embedding dictionary) 3.text/position embedding vectors 4.stacks transformer layers(self-attention normalization residual connections MLP blocks) 5.→CLS token 6.-attach classification head and do prediction,etc. Commonly trained with a self-supervised objective(ex.next token prediction)
Recall: Transformer Architecture Start with text string 1. → text tokens 2. → text embedding vectors (via embedding dictionary) 3. → text/position embedding vectors 4. → stacks transformer layers (self-attention + normalization + residual connections + MLP blocks) 5. → CLS token 6. → attach classification head and do prediction, etc. Commonly trained with a self-supervised objective (ex. next token prediction)
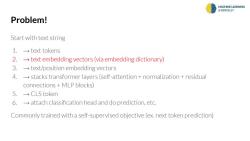
MACHINE LEARNING BERKELEY Problem! Start with text string 1.→text tokens 2.-text embedding vectors(via embedding dictionary) 3.text/position embedding vectors 4.stacks transformer layers(self-attention normalization residual connections MLP blocks) 5.→CLS token 6.attach classification head and do prediction,etc. Commonly trained with a self-supervised objective(ex.next token prediction)
Problem! Start with text string 1. → text tokens 2. → text embedding vectors (via embedding dictionary) 3. → text/position embedding vectors 4. → stacks transformer layers (self-attention + normalization + residual connections + MLP blocks) 5. → CLS token 6. → attach classification head and do prediction, etc. Commonly trained with a self-supervised objective (ex. next token prediction)
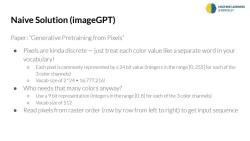
MACHINE LEARNING BERKELEY Naive Solution(imageGPT) Paper:"Generative Pretraining from Pixels" Pixels are kinda discrete-just treat each color value like a separate word in your vocabulary! o Each pixel is commonly represented by a 24 bit value(integers in the range [O,255]for each of the 3 color channels) o Vocab size of 2^24=16,777,216! Who needs that many colors anyway? o Use a 9 bit representation (integers in the range [0,8]for each of the 3 color channels) o Vocab size of 512 Read pixels from raster order(row by row from left to right)to get input sequence
Naive Solution (imageGPT) Paper: “Generative Pretraining from Pixels” ● Pixels are kinda discrete — just treat each color value like a separate word in your vocabulary! ○ Each pixel is commonly represented by a 24 bit value (integers in the range [0, 255] for each of the 3 color channels) ○ Vocab size of 2^24 = 16,777,216! ● Who needs that many colors anyway? ○ Use a 9 bit representation (integers in the range [0, 8] for each of the 3 color channels) ○ Vocab size of 512 ● Read pixels from raster order (row by row from left to right) to get input sequence
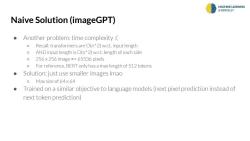
MACHINE LEARNING BERKELEY Naive Solution (imageGPT) Another problem:time complexity o Recall:transformers are O(n^2)w.r.t.input length o AND input length is O(n^2)w.r.t.length of each side o 256x 256 image=>65536 pixels o For reference,BERT only has a max length of 512 tokens ● Solution:just use smaller images Imao o Max size of 64 x 64 Trained on a similar objective to language models(next pixel prediction instead of next token prediction)
Naive Solution (imageGPT) ● Another problem: time complexity :( ○ Recall: transformers are O(n^2) w.r.t. input length ○ AND input length is O(n^2) w.r.t. length of each side ○ 256 x 256 image => 65536 pixels ○ For reference, BERT only has a max length of 512 tokens ● Solution: just use smaller images lmao ○ Max size of 64 x 64 ● Trained on a similar objective to language models (next pixel prediction instead of next token prediction)
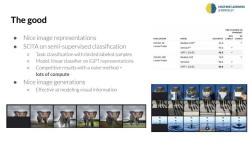
MACHINE LEARNING BERKELEY The good PRE-TRAINED ON ●' Nice image representations EVALUATION MODEL ACCURACY LARSLAE CIFAR-10 ResNet-15210 94.0 ● SOTA on semi-supervised classification Linear Probe SimCLR12 95.3 o Task:classification with limited labeled samples iGPT-L 32x32 96.3 CIFAR-100 ResNet-152 78.0 0 Model:linear classifer on iGPT representations Linear Probe SimCLR 80.2 o Competitive results with a naive method iGPT-L32x32 82.8 lots of compute ● Nice image generations o Effective at modeling visual information
The good ● Nice image representations ● SOTA on semi-supervised classification ○ Task: classification with limited labeled samples ○ Model: linear classifier on iGPT representations ○ Competitive results with a naive method + lots of compute ● Nice image generations ○ Effective at modeling visual information
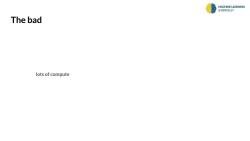
MACHINE LEARNING BERKELEY The bad lots of compute
The bad lots of compute
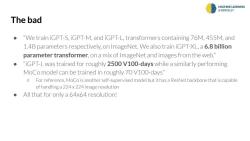
MACHINE LEARNING BERKELEY The bad ●' "We train iGPT-S,iGPT-M,and iGPT-L,transformers containing 76M,455M,and 1.4B parameters respectively,on ImageNet.We also train iGPT-XL,a 6.8 billion parameter transformer,on a mix of ImageNet and images from the web." ● "iGPT-L was trained for roughly 2500 V100-days while a similarly performing MoCo model can be trained in roughly 70 V100-days" o For reference,MoCo is another self-supervised model but it has a ResNet backbone that is capable of handling a 224 x 224 image resolution All that for only a 64x64 resolution!
The bad ● “We train iGPT-S, iGPT-M, and iGPT-L, transformers containing 76M, 455M, and 1.4B parameters respectively, on ImageNet. We also train iGPT-XL, a 6.8 billion parameter transformer, on a mix of ImageNet and images from the web.” ● “iGPT-L was trained for roughly 2500 V100-days while a similarly performing MoCo model can be trained in roughly 70 V100-days” ○ For reference, MoCo is another self-supervised model but it has a ResNet backbone that is capable of handling a 224 x 224 image resolution ● All that for only a 64x64 resolution!
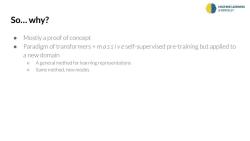
MACHINE LEARNING BERKELEY So...why? ● Mostly a proof of concept Paradigm of transformers +m a ss i ve self-supervised pre-training but applied to a new domain o A general method for learning representations o Same method,new modes
So… why? ● Mostly a proof of concept ● Paradigm of transformers + m a s s i v e self-supervised pre-training but applied to a new domain ○ A general method for learning representations ○ Same method, new modes
按次数下载不扣除下载券;
注册用户24小时内重复下载只扣除一次;
顺序:VIP每日次数-->可用次数-->下载券;
- 广东工业大学:《机器学习》课程教学资源(课件讲义)第18讲 变换器模型 Transformer.pdf
- 广东工业大学:《机器学习》课程教学资源(PPT讲稿)第18讲 变换器模型 Transformer.pptx
- 广东工业大学:《机器学习》课程教学资源(课件讲义)第17讲 注意力机制(自注意力).pdf
- 广东工业大学:《机器学习》课程教学资源(PPT讲稿)第17讲 注意力机制(概述).pptx
- 广东工业大学:《机器学习》课程教学资源(PPT讲稿)第16讲 现代循环神经网络(嵌入向量, 词嵌入, 子词嵌入, 全局向量的词嵌入).pptx
- 广东工业大学:《机器学习》课程教学资源(PPT讲稿)第16讲 现代循环神经网络(编码器解码器,Seq2seq模型,束搜索).pptx
- 广东工业大学:《机器学习》课程教学资源(PPT讲稿)第16讲 现代循环神经网络(高级循环神经网络).pptx
- 广东工业大学:《机器学习》课程教学资源(课件讲义)第15讲 无监督学习——降维深度学习可视化(Neighbor Embedding,LLE T-SNE).pdf
- 广东工业大学:《机器学习》课程教学资源(课件讲义)第15讲 无监督学习——降维深度学习可视化(PCA Kmeans).pdf
- 广东工业大学:《机器学习》课程教学资源(课件讲义)第14讲 循环神经网络(RNN).pdf
- 广东工业大学:《机器学习》课程教学资源(课件讲义)第13讲 卷积神经网络计算机视觉应用(目标检测,计算机视觉训练技巧).pdf
- 广东工业大学:《机器学习》课程教学资源(课件讲义)第13讲 卷积神经网络计算机视觉应用(Inception, 批量归一化和残差网络ResNet).pdf
- 广东工业大学:《机器学习》课程教学资源(课件讲义)第12讲 卷积神经网络(LeNet, AlexNet, VGG和NiN).pdf
- 广东工业大学:《机器学习》课程教学资源(课件讲义)第12讲 卷积神经网络(卷积和池化层).pdf
- 广东工业大学:《机器学习》课程教学资源(课件讲义)第11讲 感知机模型与多层感知机(前馈神经网络,DNN BP).pdf
- 广东工业大学:《机器学习》课程教学资源(课件讲义)第10讲 神经网络的优化(激活函数 dropout).pdf
- 广东工业大学:《机器学习》课程教学资源(课件讲义)第10讲 神经网络的优化(梯度消失和梯度爆炸BN).pdf
- 广东工业大学:《机器学习》课程教学资源(课件讲义)第10讲 神经网络的优化(自适应学习率 AdaGrad RMSProp).pdf
- 广东工业大学:《机器学习》课程教学资源(课件讲义)第10讲 神经网络的优化(batch和动量Momentum NAG).pdf
- 广东工业大学:《机器学习》课程教学资源(课件讲义)第9讲 神经网络的优化(梯度下降、学习率adagrad adam、随机梯度下降、特征缩放).pdf
- 广东工业大学:《机器学习》课程教学资源(课件讲义)第19讲 ViT及注意力机制改进(各式各样的Attention).pdf
- 广东工业大学:《机器学习》课程教学资源(课件讲义)第20讲 预训练模型 Pre-training of Deep Bidirectional Transformers for Language Understanding(授课:周郭许).pdf
- 广东工业大学:《机器学习》课程教学资源(课件讲义)第21讲 生成式网络模型(自编码器 Deep Auto-encoder).pdf
- 广东工业大学:《机器学习》课程教学资源(课件讲义)第21讲 生成式网络模型(VAE Generation).pdf
- 广东工业大学:《机器学习》课程教学资源(课件讲义)第22讲 生成式网络模型(Diffusion Model).pdf
- 广东工业大学:《机器学习》课程教学资源(课件讲义)第22讲 生成式网络模型(Stable Diffusion).pdf
- 北京信息科技大学:计算机学院各专业课程教学大纲汇编.pdf
- 北京信息科技大学:计算中心及图书馆课程教学大纲汇编.pdf
- 新乡学院:数学与统计学院信息与计算科学专业《数学分析Ⅰ》课程教学大纲(2015).pdf
- 新乡学院:数学与统计学院信息与计算科学专业《数学分析Ⅱ》课程教学大纲(2015).pdf
- 新乡学院:数学与统计学院信息与计算科学专业《数学分析Ⅲ》课程教学大纲(2015).pdf
- 新乡学院:数学与统计学院信息与计算科学专业《高等代数Ⅰ》课程教学大纲(2015).pdf
- 新乡学院:数学与统计学院信息与计算科学专业《高等代数Ⅱ》课程教学大纲(2015).pdf
- 新乡学院:数学与统计学院信息与计算科学专业《解析几何》课程教学大纲(2015).pdf
- 新乡学院:数学与统计学院信息与计算科学专业《复变函数论》课程教学大纲(2015).pdf
- 新乡学院:数学与统计学院信息与计算科学专业《常微分方程》课程教学大纲(2015).pdf
- 新乡学院:数学与统计学院信息与计算科学专业《实变函数与泛函分析》课程教学大纲(2015).pdf
- 新乡学院:数学与统计学院信息与计算科学专业《概率论》课程教学大纲(2015).pdf
- 新乡学院:数学与统计学院信息与计算科学专业《数理统计》课程教学大纲(2015).pdf
- 新乡学院:数学与统计学院信息与计算科学专业《初等数论》课程教学大纲(2015).pdf