广东工业大学:《机器学习》课程教学资源(课件讲义)第19讲 ViT及注意力机制改进(各式各样的Attention)

各式各樣的Attention Hung-yi Lee李宏毅
各式各樣的 Attention Hung-yi Lee 李宏毅

Prerequisite https://youtu.be/hYdO9CscNes https://youtu.be/gmsMY5kc-zw 【機器學習2021】自注意力 【機器學習2021】自注意力 機制(Self-attention)(上) 機制(Self-attention)(下)
Prerequisite https://youtu.be/hYdO9CscNes https://youtu.be/gmsMY5kc-zw 【機器學習2021】自注意力 機制 (Self-attention) (上) 【機器學習2021】自注意力 機制 (Self-attention) (下)

To Learn More.… Big Bird Transformer Synthesizer Performer ○Linformer ●Reformer Sinkhorn 0 Linear Transformer Long Range Arena:A Benchmark for Efficient Local Attention ● Transformers https://arxiv.org/abs/2011.04006 100 150 200 250 300 350 Speed (examples per sec) Recurrence Pefome sarTeiore Low Rank/ Memory Kernels ETC Transformer Synthe Big Bird Leamable Fixed/Factorlzed/ Patterns Random Patterns Efficient Transformers:A Survey eoe https://arxiv.org/abs/2009.06732 Axia
To Learn More … https://arxiv.org/abs/2009.06732 Efficient Transformers: A Survey Long Range Arena: A Benchmark for Efficient Transformers https://arxiv.org/abs/2011.04006 3
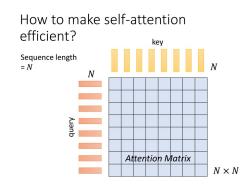
How to make self-attention efficient? key Sequence length =N anb 三三 Attention Matrix N×W
How to make self-attention efficient? Attention Matrix key query 𝑁 𝑁 𝑁 × 𝑁 Sequence length = 𝑁

Output Probabilities Notice Softmax Self-attention is only a Add Norm module in a larger Feed Forward network. Add Norm Add Norm ·Self-attention Multi-Head Feed Attention dominates computation Forward when N is large. Add Norm Add Norm Masked Multi-Head Multi-Head Usually developed for Attention Attention image processing Positional Positional Encoding Encoding N= Input Output 256 Embedding Embedding 256*256 Inputs Outputs 256 (shifted right)
Notice • Self-attention is only a module in a larger network. • Self-attention dominates computation when 𝑁 is large. • Usually developed for image processing 𝑁 = 256 ∗ 256 256 256

Skip Some Calculations with Human Knowledge Can we fill in some values with human knowledge?
Skip Some Calculations with Human Knowledge Can we fill in some values with human knowledge?

Local Attention Truncated Attention Set to 0 Similar with CNN Calculate attention key weight
Local Attention / Truncated Attention Calculate attention weight Set to 0 …… Similar with CNN key query
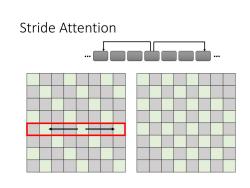
Stride Attention
Stride Attention … …
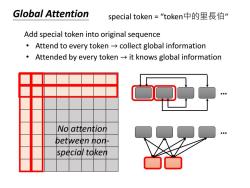
Global Attention special token="token中的里長伯" Add special token into original sequence Attend to every token-collect global information Attended by every token->it knows global information No attention between non- special token
… … Global Attention Add special token into original sequence • Attend to every token → collect global information • Attended by every token → it knows global information special token = “token中的里長伯“ No attention between nonspecial token
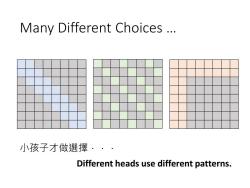
Many Different Choices .. 小孩子才做摆擇··。 Different heads use different patterns
Many Different Choices … Different heads use different patterns. 小孩子才做選擇...
按次数下载不扣除下载券;
注册用户24小时内重复下载只扣除一次;
顺序:VIP每日次数-->可用次数-->下载券;
- 广东工业大学:《机器学习》课程教学资源(课件讲义)第19讲 ViT及注意力机制改进(Vision Transformers ,ViTs).pdf
- 广东工业大学:《机器学习》课程教学资源(课件讲义)第18讲 变换器模型 Transformer.pdf
- 广东工业大学:《机器学习》课程教学资源(PPT讲稿)第18讲 变换器模型 Transformer.pptx
- 广东工业大学:《机器学习》课程教学资源(课件讲义)第17讲 注意力机制(自注意力).pdf
- 广东工业大学:《机器学习》课程教学资源(PPT讲稿)第17讲 注意力机制(概述).pptx
- 广东工业大学:《机器学习》课程教学资源(PPT讲稿)第16讲 现代循环神经网络(嵌入向量, 词嵌入, 子词嵌入, 全局向量的词嵌入).pptx
- 广东工业大学:《机器学习》课程教学资源(PPT讲稿)第16讲 现代循环神经网络(编码器解码器,Seq2seq模型,束搜索).pptx
- 广东工业大学:《机器学习》课程教学资源(PPT讲稿)第16讲 现代循环神经网络(高级循环神经网络).pptx
- 广东工业大学:《机器学习》课程教学资源(课件讲义)第15讲 无监督学习——降维深度学习可视化(Neighbor Embedding,LLE T-SNE).pdf
- 广东工业大学:《机器学习》课程教学资源(课件讲义)第15讲 无监督学习——降维深度学习可视化(PCA Kmeans).pdf
- 广东工业大学:《机器学习》课程教学资源(课件讲义)第14讲 循环神经网络(RNN).pdf
- 广东工业大学:《机器学习》课程教学资源(课件讲义)第13讲 卷积神经网络计算机视觉应用(目标检测,计算机视觉训练技巧).pdf
- 广东工业大学:《机器学习》课程教学资源(课件讲义)第13讲 卷积神经网络计算机视觉应用(Inception, 批量归一化和残差网络ResNet).pdf
- 广东工业大学:《机器学习》课程教学资源(课件讲义)第12讲 卷积神经网络(LeNet, AlexNet, VGG和NiN).pdf
- 广东工业大学:《机器学习》课程教学资源(课件讲义)第12讲 卷积神经网络(卷积和池化层).pdf
- 广东工业大学:《机器学习》课程教学资源(课件讲义)第11讲 感知机模型与多层感知机(前馈神经网络,DNN BP).pdf
- 广东工业大学:《机器学习》课程教学资源(课件讲义)第10讲 神经网络的优化(激活函数 dropout).pdf
- 广东工业大学:《机器学习》课程教学资源(课件讲义)第10讲 神经网络的优化(梯度消失和梯度爆炸BN).pdf
- 广东工业大学:《机器学习》课程教学资源(课件讲义)第10讲 神经网络的优化(自适应学习率 AdaGrad RMSProp).pdf
- 广东工业大学:《机器学习》课程教学资源(课件讲义)第10讲 神经网络的优化(batch和动量Momentum NAG).pdf
- 广东工业大学:《机器学习》课程教学资源(课件讲义)第20讲 预训练模型 Pre-training of Deep Bidirectional Transformers for Language Understanding(授课:周郭许).pdf
- 广东工业大学:《机器学习》课程教学资源(课件讲义)第21讲 生成式网络模型(自编码器 Deep Auto-encoder).pdf
- 广东工业大学:《机器学习》课程教学资源(课件讲义)第21讲 生成式网络模型(VAE Generation).pdf
- 广东工业大学:《机器学习》课程教学资源(课件讲义)第22讲 生成式网络模型(Diffusion Model).pdf
- 广东工业大学:《机器学习》课程教学资源(课件讲义)第22讲 生成式网络模型(Stable Diffusion).pdf
- 北京信息科技大学:计算机学院各专业课程教学大纲汇编.pdf
- 北京信息科技大学:计算中心及图书馆课程教学大纲汇编.pdf
- 新乡学院:数学与统计学院信息与计算科学专业《数学分析Ⅰ》课程教学大纲(2015).pdf
- 新乡学院:数学与统计学院信息与计算科学专业《数学分析Ⅱ》课程教学大纲(2015).pdf
- 新乡学院:数学与统计学院信息与计算科学专业《数学分析Ⅲ》课程教学大纲(2015).pdf
- 新乡学院:数学与统计学院信息与计算科学专业《高等代数Ⅰ》课程教学大纲(2015).pdf
- 新乡学院:数学与统计学院信息与计算科学专业《高等代数Ⅱ》课程教学大纲(2015).pdf
- 新乡学院:数学与统计学院信息与计算科学专业《解析几何》课程教学大纲(2015).pdf
- 新乡学院:数学与统计学院信息与计算科学专业《复变函数论》课程教学大纲(2015).pdf
- 新乡学院:数学与统计学院信息与计算科学专业《常微分方程》课程教学大纲(2015).pdf
- 新乡学院:数学与统计学院信息与计算科学专业《实变函数与泛函分析》课程教学大纲(2015).pdf
- 新乡学院:数学与统计学院信息与计算科学专业《概率论》课程教学大纲(2015).pdf
- 新乡学院:数学与统计学院信息与计算科学专业《数理统计》课程教学大纲(2015).pdf
- 新乡学院:数学与统计学院信息与计算科学专业《初等数论》课程教学大纲(2015).pdf
- 新乡学院:数学与统计学院信息与计算科学专业《数据结构》课程教学大纲(2015).pdf