广东工业大学:《机器学习》课程教学资源(课件讲义)第21讲 生成式网络模型(自编码器 Deep Auto-encoder)

Unsupervised Learning: Deep Auto-encoder
Unsupervised Learning: Deep Auto-encoder

Unsupervised Learning "We expect unsupervised learning to become far more important in the longer term.Human and animal learning is largely unsupervised:we discover the structure of the world by observing it,not by being told the name of every object." LeCun,Bengio,Hinton,Nature 2015 As I've said in previous statements:most of human and animal learning is unsupervised learning.If intelligence was a cake, unsupervised learning would be the cake,supervised learning would be the icing on the cake,and reinforcement learning would be the cherry on the cake.We know how to make the icing and the cherry,but we don't know how to make the cake. Yann LeCun,March 14,2016(Facebook)
Unsupervised Learning “We expect unsupervised learning to become far more important in the longer term. Human and animal learning is largely unsupervised: we discover the structure of the world by observing it, not by being told the name of every object.” – LeCun, Bengio, Hinton, Nature 2015 As I've said in previous statements: most of human and animal learning is unsupervised learning. If intelligence was a cake, unsupervised learning would be the cake, supervised learning would be the icing on the cake, and reinforcement learning would be the cherry on the cake. We know how to make the icing and the cherry, but we don't know how to make the cake. - Yann LeCun, March 14, 2016 (Facebook)
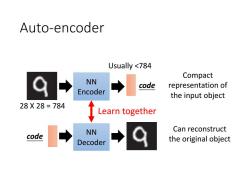
Auto-encoder Usually <784 Compact NN code representation of Encoder the input object 28X28=784 Learn together Can reconstruct code NN Decoder the original object
Auto-encoder NN Encoder NN Decoder code Compact representation of the input object code Can reconstruct the original object Learn together 28 X 28 = 784 Usually <784

Recap:PCA Minimize(x-龙)2 As close as possible encode decode W hidden layer Input layer (linear) output layer Bottleneck later Output of the hidden layer is the code
Recap: PCA 𝑥 Input layer 𝑊 𝑥 ො 𝑊𝑇 output layer hidden layer (linear) 𝑐 As close as possible Minimize 𝑥 − 𝑥 ො 2 Bottleneck later Output of the hidden layer is the code encode decode
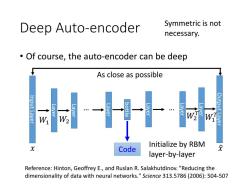
Deep Auto-encoder Symmetric is not necessary. Of course,the auto-encoder can be deep As close as possible W2 Initialize by RBM Code layer-by-layer Reference:Hinton,Geoffrey E.,and Ruslan R.Salakhutdinov."Reducing the dimensionality of data with neural networks."Science 313.5786(2006):504-507
Initialize by RBM layer-by-layer Reference: Hinton, Geoffrey E., and Ruslan R. Salakhutdinov. "Reducing the dimensionality of data with neural networks." Science 313.5786 (2006): 504-507 • Of course, the auto-encoder can be deep Deep Auto-encoder Input Layer Layer Layer bottle Output Layer Layer Layer Layer Layer … … Code As close as possible 𝑥 𝑥 ො 𝑊1 𝑊1 𝑇 𝑊2 𝑊2 𝑇 Symmetric is not necessary

Deep Auto-encoder Original /234 Image PCA /334 Deep Auto-encoder /234 1000 73A
Deep Auto-encoder Original Image PCA Deep Auto-encoder 784 784 784 1000 500 250 30 30 250 500 1000 784

空 函 1000
784 784 784 1000 500 250 2 2 250 500 1000 784
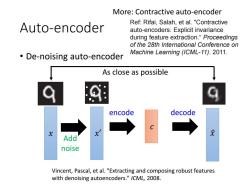
More:Contractive auto-encoder Ref:Rifai,Salah,et al."Contractive Auto-encoder auto-encoders:Explicit invariance during feature extraction."Proceedings of the 28th International Conference on De-noising auto-encoder Machine Learning(ICML-11).2011. As close as possible ◆ encode decode Add noise Vincent,Pascal,et al."Extracting and composing robust features with denoising autoencoders."/CML,2008
Auto-encoder • De-noising auto-encoder 𝑥 𝑥 ො 𝑐 encode decode Add noise 𝑥′ As close as possible More: Contractive auto-encoder Ref: Rifai, Salah, et al. "Contractive auto-encoders: Explicit invariance during feature extraction.“ Proceedings of the 28th International Conference on Machine Learning (ICML-11). 2011. Vincent, Pascal, et al. "Extracting and composing robust features with denoising autoencoders." ICML, 2008
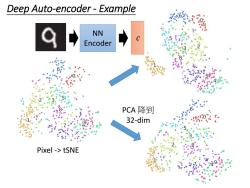
Deep Auto-encoder-Example NN C 5 Encoder 3 8 6 0,° 878 8e4 23 。 PCA降到 32-dim 5 .3 Pixel -tSNE 0 89
Deep Auto-encoder - Example Pixel -> tSNE 𝑐 NN Encoder PCA 降到 32-dim

Auto-encoder Text Retrieval Vector Space Model Bag-of-word this is 1 word string: query “This is an apple'”e 0 an 1 apple 1 pen 0 document Semantics are not considered
Auto-encoder – Text Retrieval word string: “This is an apple” … this is a an apple pen 1 1 0 1 1 0 Bag-of-word Semantics are not considered. Vector Space Model document query
按次数下载不扣除下载券;
注册用户24小时内重复下载只扣除一次;
顺序:VIP每日次数-->可用次数-->下载券;
- 广东工业大学:《机器学习》课程教学资源(课件讲义)第20讲 预训练模型 Pre-training of Deep Bidirectional Transformers for Language Understanding(授课:周郭许).pdf
- 广东工业大学:《机器学习》课程教学资源(课件讲义)第19讲 ViT及注意力机制改进(各式各样的Attention).pdf
- 广东工业大学:《机器学习》课程教学资源(课件讲义)第19讲 ViT及注意力机制改进(Vision Transformers ,ViTs).pdf
- 广东工业大学:《机器学习》课程教学资源(课件讲义)第18讲 变换器模型 Transformer.pdf
- 广东工业大学:《机器学习》课程教学资源(PPT讲稿)第18讲 变换器模型 Transformer.pptx
- 广东工业大学:《机器学习》课程教学资源(课件讲义)第17讲 注意力机制(自注意力).pdf
- 广东工业大学:《机器学习》课程教学资源(PPT讲稿)第17讲 注意力机制(概述).pptx
- 广东工业大学:《机器学习》课程教学资源(PPT讲稿)第16讲 现代循环神经网络(嵌入向量, 词嵌入, 子词嵌入, 全局向量的词嵌入).pptx
- 广东工业大学:《机器学习》课程教学资源(PPT讲稿)第16讲 现代循环神经网络(编码器解码器,Seq2seq模型,束搜索).pptx
- 广东工业大学:《机器学习》课程教学资源(PPT讲稿)第16讲 现代循环神经网络(高级循环神经网络).pptx
- 广东工业大学:《机器学习》课程教学资源(课件讲义)第15讲 无监督学习——降维深度学习可视化(Neighbor Embedding,LLE T-SNE).pdf
- 广东工业大学:《机器学习》课程教学资源(课件讲义)第15讲 无监督学习——降维深度学习可视化(PCA Kmeans).pdf
- 广东工业大学:《机器学习》课程教学资源(课件讲义)第14讲 循环神经网络(RNN).pdf
- 广东工业大学:《机器学习》课程教学资源(课件讲义)第13讲 卷积神经网络计算机视觉应用(目标检测,计算机视觉训练技巧).pdf
- 广东工业大学:《机器学习》课程教学资源(课件讲义)第13讲 卷积神经网络计算机视觉应用(Inception, 批量归一化和残差网络ResNet).pdf
- 广东工业大学:《机器学习》课程教学资源(课件讲义)第12讲 卷积神经网络(LeNet, AlexNet, VGG和NiN).pdf
- 广东工业大学:《机器学习》课程教学资源(课件讲义)第12讲 卷积神经网络(卷积和池化层).pdf
- 广东工业大学:《机器学习》课程教学资源(课件讲义)第11讲 感知机模型与多层感知机(前馈神经网络,DNN BP).pdf
- 广东工业大学:《机器学习》课程教学资源(课件讲义)第10讲 神经网络的优化(激活函数 dropout).pdf
- 广东工业大学:《机器学习》课程教学资源(课件讲义)第10讲 神经网络的优化(梯度消失和梯度爆炸BN).pdf
- 广东工业大学:《机器学习》课程教学资源(课件讲义)第21讲 生成式网络模型(VAE Generation).pdf
- 广东工业大学:《机器学习》课程教学资源(课件讲义)第22讲 生成式网络模型(Diffusion Model).pdf
- 广东工业大学:《机器学习》课程教学资源(课件讲义)第22讲 生成式网络模型(Stable Diffusion).pdf
- 北京信息科技大学:计算机学院各专业课程教学大纲汇编.pdf
- 北京信息科技大学:计算中心及图书馆课程教学大纲汇编.pdf
- 新乡学院:数学与统计学院信息与计算科学专业《数学分析Ⅰ》课程教学大纲(2015).pdf
- 新乡学院:数学与统计学院信息与计算科学专业《数学分析Ⅱ》课程教学大纲(2015).pdf
- 新乡学院:数学与统计学院信息与计算科学专业《数学分析Ⅲ》课程教学大纲(2015).pdf
- 新乡学院:数学与统计学院信息与计算科学专业《高等代数Ⅰ》课程教学大纲(2015).pdf
- 新乡学院:数学与统计学院信息与计算科学专业《高等代数Ⅱ》课程教学大纲(2015).pdf
- 新乡学院:数学与统计学院信息与计算科学专业《解析几何》课程教学大纲(2015).pdf
- 新乡学院:数学与统计学院信息与计算科学专业《复变函数论》课程教学大纲(2015).pdf
- 新乡学院:数学与统计学院信息与计算科学专业《常微分方程》课程教学大纲(2015).pdf
- 新乡学院:数学与统计学院信息与计算科学专业《实变函数与泛函分析》课程教学大纲(2015).pdf
- 新乡学院:数学与统计学院信息与计算科学专业《概率论》课程教学大纲(2015).pdf
- 新乡学院:数学与统计学院信息与计算科学专业《数理统计》课程教学大纲(2015).pdf
- 新乡学院:数学与统计学院信息与计算科学专业《初等数论》课程教学大纲(2015).pdf
- 新乡学院:数学与统计学院信息与计算科学专业《数据结构》课程教学大纲(2015).pdf
- 新乡学院:数学与统计学院信息与计算科学专业《数据结构实验》课程教学大纲(2015).pdf
- 新乡学院:数学与统计学院信息与计算科学专业《数学物理方程》课程教学大纲(2015).pdf