《数理统计》课程教学资源(参考资料)Large sample properties of MLE 01
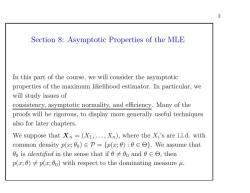
2 Section 8:Asymptotic Properties of the MLE In this part of the course,we will consider the asymptotic properties of the maximum likelihood estimator.In particular,we will study issues of consistency,asymptotic normality,and efficiency.Many of the proofs will be rigorous,to display more generally useful techniques also for later chapters. We suppose that Xn=(X1,...,Xn),where the Xi's are i.i.d.with common density p(x;fo)∈P={p(x;O):0∈Θ}.We assume that 0 o is identified in the sense that if0≠0oand0∈Θ,then p(x;0)p(x;00)with respect to the dominating measure u
2 Section 8: Asymptotic Properties of the MLE In this part of the course, we will consider the asymptotic properties of the maximum likelihood estimator. In particular, we will study issues of consistency, asymptotic normality, and efficiency. Many of the proofs will be rigorous, to display more generally useful techniques also for later chapters. We suppose that Xn = (X1,...,Xn), where the Xi’s are i.i.d. with common density p(x; θ0) ∈ P = {p(x; θ) : θ ∈ Θ}. We assume that θ0 is identified in the sense that if θ = θ0 and θ ∈ Θ, then p(x; θ) = p(x; θ0) with respect to the dominating measure µ.
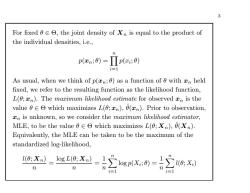
3 For fixed aee,the joint density of Xn is equal to the product of the individual densities,i.e., p(cn;0)=Πp(c;8) i=1 As usual,when we think of p(n;0)as a function of 0 with n held fixed,we refer to the resulting function as the likelihood function, L(0;n).The maximum likelihood estimate for observed xn is the value 0 which maximizes L(;),0(n).Prior to observation, xn is unknown,so we consider the marimum likelihood estimator, MLE,to be the value 0e which maximizes L(;Xn),0(Xn). Equivalently,the MLE can be taken to be the maximum of the standardized log-likelihood, 1(0;Xn)log L(0;Xn) 2 -2∑1ogp(x:0)=∑ (0:X) n n m m i=1 i=1
3 For fixed θ ∈ Θ, the joint density of Xn is equal to the product of the individual densities, i.e., p(xn; θ) = n i=1 p(xi; θ) As usual, when we think of p(xn; θ) as a function of θ with xn held fixed, we refer to the resulting function as the likelihood function, L(θ; xn). The maximum likelihood estimate for observed xn is the value θ ∈ Θ which maximizes L(θ; xn), ˆ θ(xn). Prior to observation, xn is unknown, so we consider the maximum likelihood estimator, MLE, to be the value θ ∈ Θ which maximizes L(θ; Xn), ˆ θ(Xn). Equivalently, the MLE can be taken to be the maximum of the standardized log-likelihood, l(θ; Xn) n = log L(θ; Xn) n = 1 n n i=1 log p(Xi; θ) = 1n n i=1 l(θ; Xi)
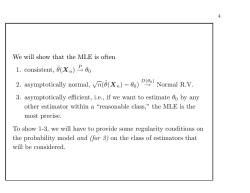
We will show that the MLE is often 1.consistent,0(n)0 2.asymptotically normal,())(Normal R.V. 3. asymptotically efficient,i.e.,if we want to estimate 0o by any other estimator within a "reasonable class,"the MLE is the most precise. To show 1-3,we will have to provide some regularity conditions on the probability model and (for 3)on the class of estimators that will be considered
4 We will show that the MLE is often 1. consistent, ˆ θ(Xn) P→ θ0 2. asymptotically normal, √n( ˆ θ(Xn) − θ0) D(θ0) → Normal R.V. 3. asymptotically efficient, i.e., if we want to estimate θ0 by any other estimator within a “reasonable class,” the MLE is the most precise. To show 1-3, we will have to provide some regularity conditions on the probability model and (for 3) on the class of estimators that will be considered
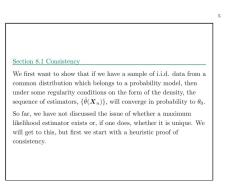
5 Section 8.1 Consistency We first want to show that if we have a sample of i.i.d.data from a common distribution which belongs to a probability model,then under some regularity conditions on the form of the density,the sequence of estimators,{(Xn)},will converge in probability to 00. So far,we have not discussed the issue of whether a maximum likelihood estimator exists or,if one does,whether it is unique.We will get to this,but first we start with a heuristic proof of consistency
5 Section 8.1 Consistency We first want to show that if we have a sample of i.i.d. data from a common distribution which belongs to a probability model, then under some regularity conditions on the form of the density, the sequence of estimators, { ˆ θ(Xn)}, will converge in probability to θ0. So far, we have not discussed the issue of whether a maximum likelihood estimator exists or, if one does, whether it is unique. We will get to this, but first we start with a heuristic proof of consistency
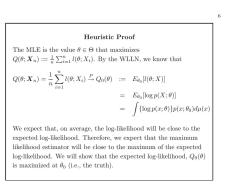
6 Heuristic Proof The MLE is the value 0e that maximizes Q(e:Xn):=是∑1l(0:X).By the WLLN,we know that Q(0:)=>1(0:X:)Qo(0):Eoo[l(0;X)] 2=1 Eoo[logp(X;0)] {logp(x;0)}p(x;0o)du(x) We expect that,on average,the log-likelihood will be close to the expected log-likelihood.Therefore,we expect that the maximum likelihood estimator will be close to the maximum of the expected log-likelihood.We will show that the expected log-likelihood,Qo(0) is maximized at 0o (i.e.,the truth)
6 Heuristic Proof The MLE is the value θ ∈ Θ that maximizes Q(θ; Xn) := 1n ni=1 l(θ; Xi). By the WLLN, we know that Q(θ; Xn) = 1 n n i=1 l(θ; Xi) P→ Q0(θ) := Eθ0 [l(θ; X)] = Eθ0 [log p(X; θ)] = {log p(x; θ)}p(x; θ0)dµ(x) We expect that, on average, the log-likelihood will be close to the expected log-likelihood. Therefore, we expect that the maximum likelihood estimator will be close to the maximum of the expected log-likelihood. We will show that the expected log-likelihood, Q0(θ) is maximized at θ0 (i.e., the truth).
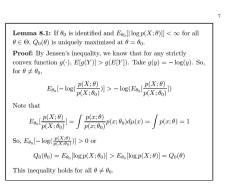
7 Lemma 8.1:If 0o is identified and Eo[l logp(X;0)]g(E[Y]).Take g(y)=-log(y).So, for0卡0o, Eoo[-log( >-品》 Note that 厂密-r= So,Ea[-log(〗>0or Qo(0o)=Eoo[logp(X;00)]>E0o [logp(X;0)]=Qo(0) This inequality holds for all 000
7 Lemma 8.1: If θ0 is identified and Eθ0 [| log p(X; θ)|] g(E[Y ]). Take g(y) = − log(y). So, for θ = θ0, Eθ0 [− log( p(X; θ) p(X; θ0))] > − log(Eθ0 [ p(X; θ) p(X; θ0)]) Note that Eθ0 [ p(X; θ) p(X; θ0)] = p(x; θ) p(x; θ0)p(x; θ0)dµ(x) = p(x; θ)=1 So, Eθ0 [− log( p(X;θ) p(X;θ0) )] > 0 or Q0(θ0) = Eθ0 [log p(X; θ0)] > Eθ0 [log p(X; θ)] = Q0(θ) This inequality holds for all θ = θ0.
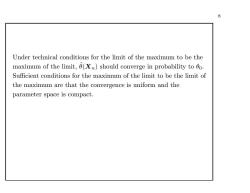
8 Under technical conditions for the limit of the maximum to be the maximum of the limit,0(Xn)should converge in probability to 00. Sufficient conditions for the maximum of the limit to be the limit of the maximum are that the convergence is uniform and the parameter space is compact
8 Under technical conditions for the limit of the maximum to be the maximum of the limit, ˆ θ(Xn) should converge in probability to θ0. Sufficient conditions for the maximum of the limit to be the limit of the maximum are that the convergence is uniform and the parameter space is compact
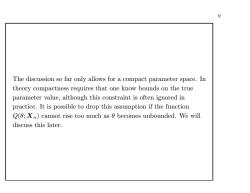
9 The discussion so far only allows for a compact parameter space.In theory compactness requires that one know bounds on the true parameter value,although this constraint is often ignored in practice.It is possible to drop this assumption if the function Q(0;Xn)cannot rise too much as 0 becomes unbounded.We will discuss this later
9 The discussion so far only allows for a compact parameter space. In theory compactness requires that one know bounds on the true parameter value, although this constraint is often ignored in practice. It is possible to drop this assumption if the function Q(θ; Xn) cannot rise too much as θ becomes unbounded. We will discuss this later
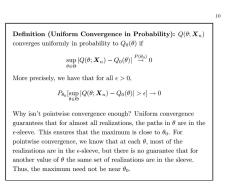
10 Definition (Uniform Convergence in Probability):Q(0;Xn) converges uniformly in probability to Qo(0)if sup(;)-Qo()P() 0∈Θ More precisely,we have that for all e>0, Poo[sup Q(0;Xn)-Qo(0)I>e]0 0∈⊙ Why isn't pointwise convergence enough?Uniform convergence guarantees that for almost all realizations,the paths in 0 are in the e-sleeve.This ensures that the maximum is close to 00.For pointwise convergence,we know that at each 0,most of the realizations are in the e-sleeve,but there is no guarantee that for another value of 0 the same set of realizations are in the sleeve. Thus,the maximum need not be near 00
10 Definition (Uniform Convergence in Probability): Q(θ; Xn) converges uniformly in probability to Q0(θ) if sup θ∈Θ |Q(θ; Xn) − Q0(θ)| P (θ0) → 0 More precisely, we have that for all > 0, Pθ0 [sup θ∈Θ |Q(θ; Xn) − Q0(θ)| > ] → 0 Why isn’t pointwise convergence enough? Uniform convergence guarantees that for almost all realizations, the paths in θ are in the -sleeve. This ensures that the maximum is close to θ0. For pointwise convergence, we know that at each θ, most of the realizations are in the -sleeve, but there is no guarantee that for another value of θ the same set of realizations are in the sleeve. Thus, the maximum need not be near θ0.
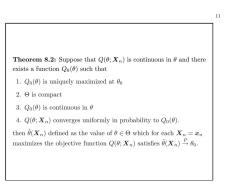
11 Theorem 8.2:Suppose that Q(0;Xn)is continuous in 0 and there exists a function Qo(0)such that 1.Qo(0)is uniquely maximized at 0o 2.Θis compact 3.Qo(0)is continuous in 0 4.Q(0;Xn)converges uniformly in probability to Qo(0). then (Xn)defined as the value of aee which for each Xn=n maximizes the objective function Q(;Xn)satisfies 0(Xn)00
11 Theorem 8.2: Suppose that Q(θ; Xn) is continuous in θ and there exists a function Q0(θ) such that 1. Q0(θ) is uniquely maximized at θ0 2. Θ is compact 3. Q0(θ) is continuous in θ 4. Q(θ; Xn) converges uniformly in probability to Q0(θ). then ˆ θ(Xn) defined as the value of θ ∈ Θ which for each Xn = xn maximizes the objective function Q(θ; Xn) satisfies ˆ θ(Xn) P→ θ0
按次数下载不扣除下载券;
注册用户24小时内重复下载只扣除一次;
顺序:VIP每日次数-->可用次数-->下载券;
- 《数理统计》课程教学资源(参考资料)An Inconsistent maximum likelihood estimate.pdf
- 《数理统计》课程教学资源(参考资料)Maximum Likelihood - An Introduction.pdf
- 中国科学技术大学:《数理统计》课程教学资源(课件讲义)第五讲 点估计方法(二)极大似然估计方法.pdf
- 中国科学技术大学:《数理统计》课程教学资源(课件讲义)第四讲 点估计方法(一)矩估计方法.pdf
- 中国科学技术大学:《数理统计》课程教学资源(课件讲义)第三讲 指数族与充分完备统计量.pdf
- 中国科学技术大学:《数理统计》课程教学资源(课件讲义)第二讲 统计量的分布(抽样分布).pdf
- 《数理统计》课程教学资源(参考资料)Hoeffding's Indequality 证明中的一个不等式的证明 complementary.pdf
- 《数理统计》课程教学资源(参考资料)Glivenko-Cantelli 定理的证明.pdf
- 中国科学技术大学:《数理统计》课程教学资源(PPT课件讲稿)统计学——统计数据的描述.ppt
- 中国科学技术大学:《数理统计》课程教学资源(课件讲义)第一讲 总体与样本 What Statistics can do(主讲:张伟平).ppt
- 佛山科学技术学院:《生物统计附试验设计》课程电子教案(PPT课件讲稿)第六章 方差分析(二)第二节 单因素试验资料的方差分析、第三节 两因素试验资料的方差分析.ppt
- 佛山科学技术学院:《生物统计附试验设计》课程电子教案(PPT课件讲稿)第六章 方差分析(三)第四节 方差分析的数学模型与期望均方、第五节 数据转换.ppt
- 佛山科学技术学院:《生物统计附试验设计》课程电子教案(PPT课件讲稿)第六章 方差分析 Analysis of Variance(一)第一节 方差分析的基本原理与步骤.ppt
- 佛山科学技术学院:《生物统计附试验设计》课程电子教案(PPT课件讲稿)第七章 次数资料分析——X2检验.ppt
- 佛山科学技术学院:《生物统计附试验设计》课程电子教案(PPT课件讲稿)第四章 常用概率分布.ppt
- 佛山科学技术学院:《生物统计附试验设计》课程电子教案(PPT课件讲稿)第五章 假设检验(二).ppt
- 佛山科学技术学院:《生物统计附试验设计》课程电子教案(PPT课件讲稿)第五章 假设检验(一)均数差异显著性检验——t检验.ppt
- 佛山科学技术学院:《生物统计附试验设计》课程电子教案(PPT课件讲稿)第二章 资料的整理.ppt
- 佛山科学技术学院:《生物统计附试验设计》课程电子教案(PPT课件讲稿)第三章 资料的统计描述.ppt
- 佛山科学技术学院:《生物统计附试验设计》课程电子教案(PPT课件讲稿)第一章 绪论 Biometrics & Experimental Design(主讲:吕敏芝).ppt
- 《数理统计》课程教学资源(参考资料)Large sample properties of MLE 02.pdf
- 《数理统计》课程教学资源(参考资料)Large sample properties of MLE 03.pdf
- 《数理统计》课程教学资源(参考资料)Large sample properties of MLE 04.pdf
- 《数理统计》课程教学资源(参考资料)Large sample properties of MLE 05.pdf
- 《数理统计》课程教学资源(参考资料)THE MM, ME, ML, EL, EF AND GMM APPROACHES TO ESTIMATION - A SYNTHESIS.pdf
- 《数理统计》课程教学资源(参考资料)Another scratch proof of consistency and asymptotical normal of MLE.pdf
- 中国科学技术大学:《数理统计》课程教学资源(课件讲义)第六讲 点估计方法(三)一致最小方差无偏估计.pdf
- 中国科学技术大学:《数理统计》课程教学资源(课件讲义)第七讲 区间估计(一)置信区间.pdf
- 中国科学技术大学:《数理统计》课程教学资源(课件讲义)第八讲 区间估计(二)容忍区间.pdf
- 中国科学技术大学:《数理统计》课程教学资源(课件讲义)第九讲 参数假设检验(一).pdf
- 《数理统计》课程教学资源(参考资料)How do we do hypothesis testing.pdf
- 中国科学技术大学:《数理统计》课程教学资源(课件讲义)第十讲 参数假设检验(二).pdf
- 中国科学技术大学:《数理统计》课程教学资源(课件讲义)第十一讲 参数假设检验(三).pdf
- 《数理统计》课程教学资源(参考资料)Likelihood Ratio, Wald, and(Rao)Score Tests.pdf
- 中国科学技术大学:《数理统计》课程教学资源(课件讲义)第十二讲 非参数检验(一).pdf
- 中国科学技术大学:《数理统计》课程教学资源(课件讲义)第十二讲 非参数检验(二).pdf
- 中国科学技术大学:《数理统计》课程教学资源(课件讲义)第十三讲 Bayes统计初步(Bayes方法和统计决策理论).pdf
- 《数理统计》课程教学资源(参考资料)THE FORMAL DEFINITION OF REFERENCE PRIORS, ANNALS, 2009.pdf
- 《数理统计》课程教学资源(参考资料)Bayes Factor - What They Are and What They Are Not.pdf
- 中国科学技术大学:《数理统计》课程教学资源(课件讲义)第十四讲 回归分析(线性回归模型).pdf