《数理统计》课程教学资源(参考资料)Glivenko-Cantelli 定理的证明
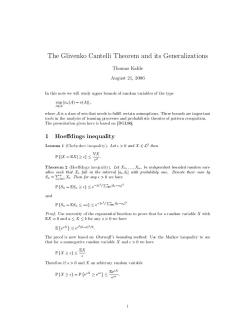
The Glivenko Cantelli Theorem and its Generalizations Thomas Kahle August 21,2006 In this note we will study upper bounds of random variables of the type sup vn(A)-v(A)川: AEA where A is a class of sets that needs to fulfill certain assumptions.These bounds are important tools in the analysis of learning processes and probabilistic theories of pattern recognition. The presentation given here is based on [DGL96]. 1 Hoeffdings inequality Lemma 1 (Cheby shev inequality).Lete>0 and X E C2 then VX PIX-EXI≥≤ Theorem 2 (Hoeffdings inequality).Let X1,...,Xn,be independent bounded random vari- ables such that Xi fall in the interval ai,bi]with probability one.Denote their sum by Sn=Xi.Then for any e>0 we have P{S-E5n≥≤e-21∑,6-a)2 d P{Sn-ESn≤-e}≤e-2E1∑6-a)P Proof.Use convexity of the exponential function to prove that for a random variable X with EX=0anda≤X≤b for any s>0 we have E{ex}≤e2b-a2/s The proof is now based on Chernoff's bounding method:Use the Markov inequality to see that for a nonnegative random variable X and e>0 we have P(X≥sX Therefore if s>0 and X an arbitrary random variable P{X≥e}=P{ex≥ese}≤ Eesx ese 1
The Glivenko Cantelli Theorem and its Generalizations Thomas Kahle August 21, 2006 In this note we will study upper bounds of random variables of the type sup A∈A |νn(A) − ν(A)| , where A is a class of sets that needs to fulll certain assumptions. These bounds are important tools in the analysis of learning processes and probabilistic theories of pattern recognition. The presentation given here is based on [DGL96]. 1 Hoedings inequality Lemma 1 (Chebyshev inequality). Let ² > 0 and X ∈ L2 then P {|X − EX| ≥ ²} ≤ VX ² 2 . Theorem 2 (Hoedings inequality). Let X1, . . . , Xn, be independent bounded random variables such that Xi fall in the interval [ai , bi ] with probability one. Denote their sum by Sn = Pn i=1 Xi . Then for any ² > 0 we have P {Sn − ESn ≥ ²} ≤ e −2² 2/ Pn i=1(bi−ai) 2 and P {Sn − ESn ≤ −²} ≤ e −2² 2/ Pn i=1(bi−ai) 2 Proof. Use convexity of the exponential function to prove that for a random variable X with EX = 0 and a ≤ X ≤ b for any s > 0 we have E © e sXª ≤ e s 2 (b−a) 2/8 . The proof is now based on Cherno 's bounding method: Use the Markov inequality to see that for a nonnegative random variable X and ² > 0 we have P {X ≥ ²} ≤ EX ² Therefore if s > 0 and X an arbitrary random variable P {X ≥ ²} = P © e sX ≥ e s²ª ≤ Ee sX e s² . 1

Chernoff's method now is to find an s>0 that minimizes the upper bound or at least makes it small.In our case we have =eⅡE{e-w} by independence 1 ≤e-eⅡea-aP/sby first line of proof =e-sees2∑-1(b:-a4)2/8 =e-2e2/∑-16-a4)2 chooses=4e/∑-a)2 i=l The second inequality is proved analogously. ▣ 2 The Glivenko Cantelli Theorem As a fir st step we study an alternative proof of the well known theorem Theorem 3 (Glivenko-Cantelli).Let Z1,...:Zn be i.i.d.real valued random variables with distribution function F(z)=P(Z1 2).Let 5▣1 be the standard empirical distribution function.Then P{supF(e)-Fn(a训>e}≤8(n+1)e-n/32 z∈R and in particular by the Borel-Cantelli lemma lim supF(z)-Fn(z川=0 with probability one n→0∞ze2 The proof we will give is not the simplest one possible,but it contains the ideas of the generalization we will consider later.The argument given here is due to symmetrization ideas of Dudley [Dud78]and Pollard Pol84]. Proof.Assume ne2>2,otherwise the bound is trivial.Introduce the following notation v(A=P{☑∈A}anda(A)=是∑=llZ,eA}where AR is a measurable st..Denote by A the class of sets of the form (-oo,2],zER.Then we have sup lF(2)-Fn(2)=sup Ivn(A)-v(A)I. zER AEA STEP 1.FIRST SYMMETRIZATION BY A GHOST SAMPLE Define random variables Zi,...,ZER such that Z1,...,Zn,Z1,...,Zn are all i.i.d.Denote by the empirical measure of the primed variables.For ne2 2 we have P{腮.(-(4训>s2P{腰.(-(A训≥引 2
Cherno's method now is to nd an s > 0 that minimizes the upper bound or at least makes it small. In our case we have P {Sn − ESn ≥ ²} ≤ e −s²E ( exp à s Xn i=1 (Xi − EXi) !) = e−s² Yn i=1 E n e s(Xi−EXi) o by independence ≤ e −s² Yn i=1 e s 2 (bi−ai) 2/8 by rst line of proof = e−s²e s 2 Pn i=1(bi−ai) 2/8 = e−2² 2/ Pn i=1(bi−ai) 2 choose s = 4²/Xn i=1 (bi − ai) 2 The second inequality is proved analogously. 2 The Glivenko Cantelli Theorem As a rst step we study an alternative proof of the well known theorem Theorem 3 (Glivenko-Cantelli). Let Z1, . . . , Zn be i.i.d. real valued random variables with distribution function F(z) = P(Z1 ≤ z). Let Fn(z) = 1 n Xn i=1 1{Zi≤z} be the standard empirical distribution function. Then P ½ sup z∈R |F(z) − Fn(z)| > ²¾ ≤ 8 (n + 1) e−n²2/32 , and in particular by the Borel-Cantelli lemma limn→∞ sup z∈R |F(z) − Fn(z)| = 0 with probability one. The proof we will give is not the simplest one possible, but it contains the ideas of the generalization we will consider later. The argument given here is due to symmetrization ideas of Dudley [Dud78] and Pollard [Pol84]. Proof. Assume n²2 > 2, otherwise the bound is trivial. Introduce the following notation ν(A) = P {Z1 ∈ A} and νn(A) = 1 n Pn j=1 1{Zj∈A} where A ⊆ R is a measurable set. Denote by A the class of sets of the form (−∞, z], z ∈ R. Then we have sup z∈R |F(z) − Fn(z)| = sup A∈A |νn(A) − ν(A)| . Step 1. First symmetrization by a ghost sample Dene random variables Z 0 1 , . . . , Z0 n ∈ R such that Z1, . . . , Zn, Z0 1 , . . . , Z0 n are all i.i.d. Denote by ν 0 n the empirical measure of the primed variables. For n²2 ≥ 2 we have P ½ sup A∈A |νn(A) − ν(A)| > ²¾ ≤ 2P ½ sup A∈A |νn(A) − ν 0 n(A)| ≥ ² 2 ¾ 2

2 THE GLIVENKO CANTELLI THEOREM 3 To see this let A*be a set for which vn(A*)-v(A*)I>e if existent or a fixed set in A otherwise.The measur ability of such a choice needs to be checked,using the technique of step 3 of the proof,showing that its actually a choice from finitely many sets only.Then P{.4-4训>分身≥P{(4)-(4I>} ≥P{(4)-4川>e,以4-4*I分}≥.(a)-4训> 1 sup lun(A)-v(A)I>e ≥2P1 STEP 2.SECOND SYMMETRIZATION BY RANDOM SIGNS Let 01,...,on be i.i.d.sign variables that are also independent of all Zi,Z and satisfy P{o 1)=P{o =-1)=j.Then using step 1 (aw2-w2小>} 11 =2P E.a-2列>} We now apply the union bound to remove the auxiliary random variables Z1,...,Zn {2aa->引 s{☏容wa小}+{空网 STEP 3.CONDITIONING To find a bound on the probability r{空wa小}-假2小 we will condition on Z1,...,Zn.The conditional probability can be bounded and afterwards we will remove the conditioning by just taking the expectation value
2 THE GLIVENKO CANTELLI THEOREM 3 To see this let A∗ be a set for which |νn(A∗ ) − ν(A∗ )| > ² if existent or a xed set in A otherwise. The measurability of such a choice needs to be checked, using the technique of step 3 of the proof, showing that its actually a choice from nitely many sets only. Then P ½ sup A∈A |νn(A) − ν 0 n(A)| > ² 2 ¾ ≥ P n |νn(A ∗ ) − ν 0 n(A ∗ )| > ² 2 o ≥ P n |νn(A ∗ ) − ν(A ∗ )| > ², |ν 0 n(A ∗ ) − ν(A ∗ )| ²}P n |ν 0 n(A ∗ ) − ν(A ∗ )| ² 2 ¾ ≥ 1 2 P {|νn(A ∗ ) − ν(A ∗ )| > ²} ≥ 1 2 P ½ sup A∈A |νn(A) − ν(A)| > ²¾ . Step 2. Second Symmetrization by random signs Let σ1, . . . , σn be i.i.d. sign variables that are also independent of all Zi , Z0 i and satisfy P {σ = 1} = P {σ = −1} = 1 2 . Then using step 1 P ½ sup A∈A |νn(A) − ν(A)| > ²¾ ≤ 2P ( sup A∈A 1 n ¯ ¯ ¯ ¯ ¯ Xn i=1 ¡ 1{A}(Zi) − 1{A}(Z 0 i ) ¢ ¯ ¯ ¯ ¯ ¯ > ² 2 ) = 2P ( sup A∈A 1 n ¯ ¯ ¯ ¯ ¯ Xn i=1 σi ¡ 1{A}(Zi) − 1{A}(Z 0 i ) ¢ ¯ ¯ ¯ ¯ ¯ > ² 2 ) . We now apply the union bound to remove the auxiliary random variables Z 0 1 , . . . , Z0 n. P ( sup A∈A 1 n ¯ ¯ ¯ ¯ ¯ Xn i=1 σi ¡ 1{A}(Zi) − 1{A}(Z 0 i ) ¢ ¯ ¯ ¯ ¯ ¯ > ² 2 ) ≤ P ( sup A∈A 1 n ¯ ¯ ¯ ¯ ¯ Xn i=1 σi1{A}(Zi) ¯ ¯ ¯ ¯ ¯ > ² 4 ) + P ( sup A∈A 1 n ¯ ¯ ¯ ¯ ¯ Xn i=1 σi1{A}(Z 0 i ) ¯ ¯ ¯ ¯ ¯ > ² 4 ) = 2P ( sup A∈A 1 n ¯ ¯ ¯ ¯ ¯ Xn i=1 σi1{A}(Zi) ¯ ¯ ¯ ¯ ¯ > ² 4 ) . Step 3. Conditioning To nd a bound on the probability P ( sup A∈A 1 n ¯ ¯ ¯ ¯ ¯ Xn i=1 σi1{A}(Zi) ¯ ¯ ¯ ¯ ¯ > ² 4 ) = P ( sup z∈R 1 n ¯ ¯ ¯ ¯ ¯ Xn i=1 σi1{Zi≤z} ¯ ¯ ¯ ¯ ¯ > ² 4 ) we will condition on Z1, . . . , Zn. The conditional probability can be bounded and afterwards we will remove the conditioning by just taking the expectation value

We observe that,having 21,...,2nER fixed,as z ranges over R the number of different vectors(lz≤},,lzn≤e})is at most n+1.Therefore conditioned on Z1,,Zn the supremum in the probability above is just a maximum taken over the at most n+1 random variables.We apply the union bound to find sup aa(>.n A∈A ≤(m+1)sup Having the supremum outside the probability we are left with finding an exponential bound on the probability 店w12} STEP 4.HOEFFDINGS INEQUALITY As we have conditioned on the values of the Z1,...,Zn we can regard 21.....as fixed.Then )is the sum of n independent zero mean random variables between-1 and 1.We can therefore apply Hoeffdings inequality: 店a4>么2}s Thus we find ≤2(n+1)e-ne2/32 Take the expectation value on both sides to find ≤2(n+1)e-ne2/32 Altogether we have P{.④-以>s8u+ecm which finishes the proof. 口 3 Generalizations Next we want to prove the Vapnik Chervonenkis inequality,which is a mighty generalization of the Glivenko Cantelli Theorem.The proof we just studied is after a slight adjustment already proof of the stronger theorem. The aim of the generalization is to give the statement for arbitrary classes of measurable sets A.We then need to refine the argument in the proof were the sup is identified as a maximum. Definition 4.Let A be a collection of measurable sets in Rd.For z1,...,ERd we call NA(z1,...,zn):=Ifz1,...,2n}nA:AEA} 4
We observe that, having z1, . . . , zn ∈ R xed, as z ranges over R the number of dierent vectors ¡ 1{z1≤z}, . . . , 1{zn≤z} ¢ is at most n + 1. Therefore conditioned on Z1, . . . , Zn the supremum in the probability above is just a maximum taken over the at most n + 1 random variables. We apply the union bound to nd P ( sup A∈A 1 n ¯ ¯ ¯ ¯ ¯ Xn i=1 σi1{A}(Zi) ¯ ¯ ¯ ¯ ¯ > ² 4 | Z1, . . . , Zn ) ≤ (n + 1) sup A∈A P ( 1 n ¯ ¯ ¯ ¯ ¯ Xn i=1 σi1{A}(Zi) ¯ ¯ ¯ ¯ ¯ > ² 4 | Z1, . . . , Zn ) . Having the supremum outside the probability we are left with nding an exponential bound on the probability P ( 1 n ¯ ¯ ¯ ¯ ¯ Xn i=1 σi1{A}(Zi) ¯ ¯ ¯ ¯ ¯ > ² 4 | Z1, . . . , Zn ) . Step 4. Hoeffdings inequality As we have conditioned on the values of the Z1, . . . , Zn we can regard z1, . . . , zn as xed. Then Pn i=1 σi1{A}(zi) is the sum of n independent zero mean random variables between −1 and 1. We can therefore apply Hoedings inequality: P ( 1 n ¯ ¯ ¯ ¯ ¯ Xn i=1 σi1{A}(Zi) ¯ ¯ ¯ ¯ ¯ > ² 4 | Z1, . . . , Zn ) ≤ 2e−n²2/32 . Thus we nd P ( sup A∈A 1 n ¯ ¯ ¯ ¯ ¯ Xn i=1 σi1{A}(Zi) ¯ ¯ ¯ ¯ ¯ > ² 4 | Z1, . . . , Zn ) ≤ 2(n + 1)e−n²2/32 . Take the expectation value on both sides to nd P ( sup A∈A 1 n ¯ ¯ ¯ ¯ ¯ Xn i=1 σi1{A}(Zi) ¯ ¯ ¯ ¯ ¯ > ² 4 ) ≤ 2(n + 1)e−n²2/32 . Altogether we have P ½ sup A∈A |νn(A) − ν(A)| > ²¾ ≤ 8(n + 1)e−n²2/32 . which nishes the proof. 3 Generalizations Next we want to prove the Vapnik Chervonenkis inequality, which is a mighty generalization of the Glivenko Cantelli Theorem. The proof we just studied is after a slight adjustment already proof of the stronger theorem. The aim of the generalization is to give the statement for arbitrary classes of measurable sets A. We then need to rene the argument in the proof were the sup is identied as a maximum. Denition 4. Let A be a collection of measurable sets in R d . For z1, . . . , zn ∈ R d we call NA(z1, . . . , zn) := |{{z1, . . . , zn} ∩ A : A ∈ A}| 4
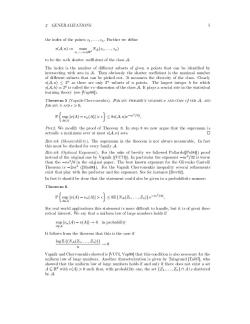
3 GENERALIZATIONS 5 the index of the points 21,...,2n.Further we define sAm)=me N) to be the n-th shatter coefficient of the class A. The index is the number of different subsets of given n points that can be identified by intersecting with sets in A.Then obviously the shatter coefficient is the maximal number of different subsets that can be picked out.It measures the diversity of the class.Clearly s(A,n)0, P sup v(A)-vn(A)I>ee 8ENA(Z....Zn)}e-ne/2 、AA For real world applications this statement is more difficult to hande,but it is of great theo- retical interest.We say that a uniform law of large numbers holds if sup vn(A)-v(A)0 in probability AEA It follows from the theorem that this is the case if 1og{(N4(Z,,Zn)》一0 Vapnik and Chervonenkis showed is [VC71 Vap98 that this condition is also necessary for the uniform law of large numbers.Another characterization is given by Talagrand [Tal87,who showed that the uniform law of large numbers holds if and only if there does not exist a set A CRd with v(A)>0 such that,with probability one,the set {21,...,Zn}nA is shattered by A
3 GENERALIZATIONS 5 the index of the points z1, . . . , zn. Further we dene s(A, n) := max z1,...,zn∈Rd NA(z1, . . . , zn) to be the n-th shatter coecient of the class A. The index is the number of dierent subsets of given n points that can be identied by intersecting with sets in A. Then obviously the shatter coecient is the maximal number of dierent subsets that can be picked out. It measures the diversity of the class. Clearly s(A, n) ≤ 2 n as there are only 2 n subsets of n points. The largest integer h for which s(A, h) = 2h is called the vc-dimension of the class A. It plays a crucial role in the statistical learning theory (see [Vap00]). Theorem 5 (Vapnik-Chervonenkis). For any probability measure ν and class of sets A, and for any n and ² > 0, P ½ sup A∈A |ν(A) − νn(A)| > ²¾ ≤ 8s(A, n)e−n²2/32 , Proof. We modify the proof of Theorem 3: In step 3 we now argue that the supremum is actually a maximum over at most s(A, n) sets. Remark (Measurability). The supremum in the theorem is not always measurable. In fact this must be checked for every family A. Remark (Optimal Exponent). For the sake of brevity we followed Pollards([Pol84]) proof instead of the original one by Vapnik ([VC71]). In particular the exponent −n²2/32 is worse than the −n²2/8 in the original paper. The best known exponent for the Glivenko Cantelli Theorem is −2n²2 ([Mas90]). For the Vapnik Chervonenkis inequality several renements exist that play with the prefactor and the exponent. See for instance [Dev82]. In fact it should be clear that the statement could also be given in a probabilistic manner: Theorem 6. P ½ sup A∈A |ν(A) − νn(A)| > ²¾ ≤ 8E {NA(Z1, . . . , Zn)} e −n²2/32 , For real world applications this statement is more dicult to handle, but it is of great theoretical interest. We say that a uniform law of large numbers holds if sup A∈A |νn(A) − ν(A)| → 0 in probability It follows from the theorem that this is the case if log E {(NA(Z1, . . . , Zn))} n → 0 Vapnik and Chervonenkis showed is [VC71, Vap98] that this condition is also necessary for the uniform law of large numbers. Another characterization is given by Talagrand [Tal87], who showed that the uniform law of large numbers holds if and only if there does not exist a set A ⊆ R d with ν(A) > 0 such that, with probability one, the set {Z1, . . . , Zn} ∩ A is shattered by A
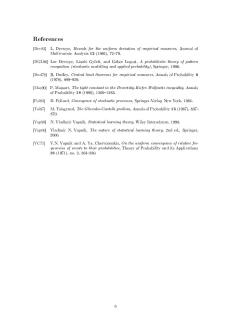
References [Dev82]L.Devroye,Bounds for the uniform deviation of empirical measures,Journal of Multivariate Analysis 12(1982),72-79. [DGL96]Luc Devroye,Laszlo Gyorfi,and Gabor Lugosi,A probabilistic theory of pattern recognition (stochastic modelling and applied probability),Springer,1996. [Dud78 R.Dudley,Central limit theorems for empirical measures,Annals of Probability 6 (1978),899-929. [Mas90 P.Massart,The tight constant in the Dvoretzky-Kiefer-Wolfowitz inequaltiy,Annals of Probability 18(1990),1269-1283. [Pol84]D.Pollard,Convegence of stochastic processes,Springer-Verlag New York,1984. [Tal87]M.Talagrand,The Glivenko-Cantelli problem,Annals of Probability 15(1987),837- 870. [Vap98 N.Vladimir Vapnik,Statistical learning theory,Wiley Interscience,1998. [Vap00 Vladimir N.Vapnik,The nature of statistical learning theory,2nd ed.,Springer, 2000. [VC71]V.N.Vapnik and A.Ya.Chervonenkis,On the uniform convergence of relative fre quencies of events to their probabilities,Theory of Probability and its Applications 16(1971),n0.2,264-280. 6
References [Dev82] L. Devroye, Bounds for the uniform deviation of empirical measures, Journal of Multivariate Analysis 12 (1982), 7279. [DGL96] Luc Devroye, László Györ, and Gábor Lugosi, A probabilistic theory of pattern recognition (stochastic modelling and applied probability), Springer, 1996. [Dud78] R. Dudley, Central limit theorems for empirical measures, Annals of Probability 6 (1978), 899929. [Mas90] P. Massart, The tight constant in the Dvoretzky-Kiefer-Wolfowitz inequaltiy, Annals of Probability 18 (1990), 12691283. [Pol84] D. Pollard, Convegence of stochastic processes, Springer-Verlag New York, 1984. [Tal87] M. Talagrand, The Glivenko-Cantelli problem, Annals of Probability 15 (1987), 837 870. [Vap98] N. Vladimir Vapnik, Statistical learning theory, Wiley Interscience, 1998. [Vap00] Vladimir N. Vapnik, The nature of statistical learning theory, 2nd ed., Springer, 2000. [VC71] V.N. Vapnik and A. Ya. Chervonenkis, On the uniform convergence of relative frequencies of events to their probabilities, Theory of Probability and its Applications 16 (1971), no. 2, 264280. 6
按次数下载不扣除下载券;
注册用户24小时内重复下载只扣除一次;
顺序:VIP每日次数-->可用次数-->下载券;
- 中国科学技术大学:《数理统计》课程教学资源(PPT课件讲稿)统计学——统计数据的描述.ppt
- 中国科学技术大学:《数理统计》课程教学资源(课件讲义)第一讲 总体与样本 What Statistics can do(主讲:张伟平).ppt
- 佛山科学技术学院:《生物统计附试验设计》课程电子教案(PPT课件讲稿)第六章 方差分析(二)第二节 单因素试验资料的方差分析、第三节 两因素试验资料的方差分析.ppt
- 佛山科学技术学院:《生物统计附试验设计》课程电子教案(PPT课件讲稿)第六章 方差分析(三)第四节 方差分析的数学模型与期望均方、第五节 数据转换.ppt
- 佛山科学技术学院:《生物统计附试验设计》课程电子教案(PPT课件讲稿)第六章 方差分析 Analysis of Variance(一)第一节 方差分析的基本原理与步骤.ppt
- 佛山科学技术学院:《生物统计附试验设计》课程电子教案(PPT课件讲稿)第七章 次数资料分析——X2检验.ppt
- 佛山科学技术学院:《生物统计附试验设计》课程电子教案(PPT课件讲稿)第四章 常用概率分布.ppt
- 佛山科学技术学院:《生物统计附试验设计》课程电子教案(PPT课件讲稿)第五章 假设检验(二).ppt
- 佛山科学技术学院:《生物统计附试验设计》课程电子教案(PPT课件讲稿)第五章 假设检验(一)均数差异显著性检验——t检验.ppt
- 佛山科学技术学院:《生物统计附试验设计》课程电子教案(PPT课件讲稿)第二章 资料的整理.ppt
- 佛山科学技术学院:《生物统计附试验设计》课程电子教案(PPT课件讲稿)第三章 资料的统计描述.ppt
- 佛山科学技术学院:《生物统计附试验设计》课程电子教案(PPT课件讲稿)第一章 绪论 Biometrics & Experimental Design(主讲:吕敏芝).ppt
- 佛山科学技术学院:《生物统计附试验设计》课程电子教案(PPT课件讲稿)第十章 协方差分析.ppt
- 佛山科学技术学院:《生物统计附试验设计》课程电子教案(PPT课件讲稿)第八章 直线回归与相关.ppt
- 江西农业大学学报:奶牛产奶量与乳成分的多元回归分析(宁夏大学:张巧娥、吴学荣、马水鱼、邢燕).pdf
- 山东大学:《SAS软件应用基础》课程电子教案(PPT课件)第九章 t检验和方差分析(2/2)、第十章 相关与回归.ppt
- 山东大学:《SAS软件应用基础》课程电子教案(PPT课件)第四章 数据步程序设计.ppt
- 山东大学:《SAS软件应用基础》课程电子教案(PPT课件)第八章 基础统计过程(2/2)、第九章 t检验和方差分析(1/2).ppt
- 山东大学:《SAS软件应用基础》课程电子教案(PPT课件)第六章 SAS过程中常用语句、第七章 SAS服务过程、第八章 基础统计过程(1/2).ppt
- 山东大学:《SAS软件应用基础》课程电子教案(PPT课件)第四章 数据步程序设计、第五章 SAS文件操作.ppt
- 《数理统计》课程教学资源(参考资料)Hoeffding's Indequality 证明中的一个不等式的证明 complementary.pdf
- 中国科学技术大学:《数理统计》课程教学资源(课件讲义)第二讲 统计量的分布(抽样分布).pdf
- 中国科学技术大学:《数理统计》课程教学资源(课件讲义)第三讲 指数族与充分完备统计量.pdf
- 中国科学技术大学:《数理统计》课程教学资源(课件讲义)第四讲 点估计方法(一)矩估计方法.pdf
- 中国科学技术大学:《数理统计》课程教学资源(课件讲义)第五讲 点估计方法(二)极大似然估计方法.pdf
- 《数理统计》课程教学资源(参考资料)Maximum Likelihood - An Introduction.pdf
- 《数理统计》课程教学资源(参考资料)An Inconsistent maximum likelihood estimate.pdf
- 《数理统计》课程教学资源(参考资料)Large sample properties of MLE 01.pdf
- 《数理统计》课程教学资源(参考资料)Large sample properties of MLE 02.pdf
- 《数理统计》课程教学资源(参考资料)Large sample properties of MLE 03.pdf
- 《数理统计》课程教学资源(参考资料)Large sample properties of MLE 04.pdf
- 《数理统计》课程教学资源(参考资料)Large sample properties of MLE 05.pdf
- 《数理统计》课程教学资源(参考资料)THE MM, ME, ML, EL, EF AND GMM APPROACHES TO ESTIMATION - A SYNTHESIS.pdf
- 《数理统计》课程教学资源(参考资料)Another scratch proof of consistency and asymptotical normal of MLE.pdf
- 中国科学技术大学:《数理统计》课程教学资源(课件讲义)第六讲 点估计方法(三)一致最小方差无偏估计.pdf
- 中国科学技术大学:《数理统计》课程教学资源(课件讲义)第七讲 区间估计(一)置信区间.pdf
- 中国科学技术大学:《数理统计》课程教学资源(课件讲义)第八讲 区间估计(二)容忍区间.pdf
- 中国科学技术大学:《数理统计》课程教学资源(课件讲义)第九讲 参数假设检验(一).pdf
- 《数理统计》课程教学资源(参考资料)How do we do hypothesis testing.pdf
- 中国科学技术大学:《数理统计》课程教学资源(课件讲义)第十讲 参数假设检验(二).pdf