《数理统计》课程教学资源(参考资料)Large sample properties of MLE 03

46 Section 8.3.Efficiency Suppose we want to estimate a real-valued function of 0,say g(0), g():RR.Assume that g has continuous partial derivatives. Consider estimating g(0o)by g((Xn)),where (Xn)is the MLE. Assuming that the 8 regularity conditions hold,we know that √元((Xn)-do)巳N(0,I-l(do).By the multivariate delta method,we know that √m(g((xn)-g(0o)2N(0,a(0o)'I-1(0o)a(0o) where a(0o)is the gradient of g(0)at 00
46 Section 8.3. Efficiency Suppose we want to estimate a real-valued function of θ, say g(θ), g(·) : Rk → R. Assume that g has continuous partial derivatives. Consider estimating g(θ0) by g( ˆ θ(Xn)), where ˆ θ(Xn) is the MLE. Assuming that the 8 regularity conditions hold, we know that √n( ˆ θ(Xn) − θ0) D→ N(0, I−1(θ0)). By the multivariate delta method, we know that √n(g(ˆθ(Xn)) − g(θ0)) D→ N(0, a(θ0)I−1(θ0)a(θ0)) where a(θ0) is the gradient of g(θ) at θ0
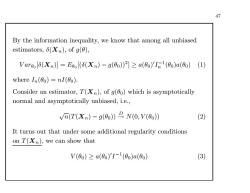
47 By the information inequality,we know that among all unbiased estimators,6(Xn),of g(0), Varoo [6(Xn)]=Eoo[(8(Xn)-g(0o))2]>a(00)'In (0o)a(0o)(1) where In(00)=nI(00). Consider an estimator,T(Xn),of g(0o)which is asymptotically normal and asymptotically unbiased,i.e., n(T(Xn)-g(0o))2 N(0,V(0o)) (2) It turns out that under some additional regularity conditions on T(Xn),we can show that V(0o)≥a(0o)'I-1(0o)a(0o) (3)
47 By the information inequality, we know that among all unbiased estimators, δ(Xn), of g(θ), V arθ0 [δ(Xn)] = Eθ0 [(δ(Xn) − g(θ0))2] ≥ a(θ0)I−1 n (θ0)a(θ0) (1) where In(θ0) = nI(θ0). Consider an estimator, T(Xn), of g(θ0) which is asymptotically normal and asymptotically unbiased, i.e., √n(T(Xn) − g(θ0)) D→ N(0, V (θ0)) (2) It turns out that under some additional regularity conditions on T(Xn), we can show that V (θ0) ≥ a(θ0)I−1(θ0)a(θ0) (3)

48 Definition:A regular estimator T(Xn)of g(0o)which satisfies (2) with V(0o)=a(0o)'I1(00)a(0o)is said to be asymptotically efficient. If g((X))is regular,then we know that it is asymptotically efficient. Remarks about Lower Bounds (1)and (3) .(1)is attained only under exceptional circumstances (i.e., usually need completeness),while (3)is obtained under quite general regularity conditions. The UMVUE tends to be unique,while asymptotically efficient estimators are not.If T(Xn)is asymptotically efficient,then so is T(Xn)+Rn;provided nRn 0. In (1),the estimator must be unbiased,whereas in(3),the estimator must be consistent and asymptotically unbiased
48 Definition: A regular estimator T(Xn) of g(θ0) which satisfies (2) with V (θ0) = a(θ0)I−1(θ0)a(θ0) is said to be asymptotically efficient. If g( ˆ θ(Xn)) is regular, then we know that it is asymptotically efficient. Remarks about Lower Bounds (1) and (3) • (1) is attained only under exceptional circumstances (i.e., usually need completeness), while (3) is obtained under quite general regularity conditions. • The UMVUE tends to be unique, while asymptotically efficient estimators are not. If T(Xn) is asymptotically efficient, then so is T(Xn) + Rn, provided √nRn P→ 0. • In (1), the estimator must be unbiased, whereas in (3), the estimator must be consistent and asymptotically unbiased
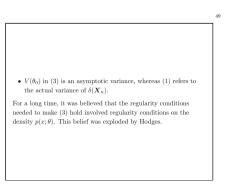
49 .V(0o)in (3)is an asymptotic variance,whereas (1)refers to the actual variance of 6(Xn). For a long time,it was believed that the regularity conditions needed to make (3)hold involved regularity conditions on the density p(x;0).This belief was exploded by Hodges
49 • V (θ0) in (3) is an asymptotic variance, whereas (1) refers to the actual variance of δ(Xn). For a long time, it was believed that the regularity conditions needed to make (3) hold involved regularity conditions on the density p(x; θ). This belief was exploded by Hodges
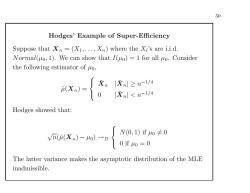
50 Hodges'Example of Super-Efficiency Suppose thatXn=(X1,...,Xn)where the Xi's are i.i.d. Normal(uo,1).We can show that I(uo)=1 for all uo.Consider the following estimator of uo, n|xn≥n-1/4 )|xml<n-1/4 Hodges showed that: VHECN. N(0,1)ifo≠0 The latter variance makes the asymptotic distribution of the MLE inadmissible
50 Hodges’ Example of Super-Efficiency Suppose that Xn = (X1,...,Xn) where the Xi’s are i.i.d. Normal(µ0, 1). We can show that I(µ0) = 1 for all µ0. Consider the following estimator of µ0, µ ˆ(Xn) = ⎧⎨⎩ X¯ n |X¯ n| ≥ n−1/4 0 |X¯ n| < n−1/4 Hodges showed that: √n(ˆµ(Xn) − µ0) →D ⎧⎨⎩ N(0, 1) if µ0 = 0 0 if µ0 = 0 The latter variance makes the asymptotic distribution of the MLE inadmissible
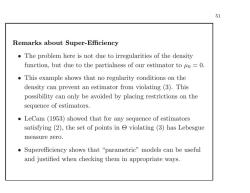
51 Remarks about Super-Efficiency The problem here is not due to irregularities of the density function,but due to the partialness of our estimator to uo =0. This example shows that no regularity conditions on the density can prevent an estimator from violating (3).This possibility can only be avoided by placing restrictions on the sequence of estimators. LeCam (1953)showed that for any sequence of estimators satisfying (2),the set of points in violating (3)has Lebesgue measure zero. ●Superefficiency shows that“parametric”models can be useful and justified when checking them in appropriate ways
51 Remarks about Super-Efficiency • The problem here is not due to irregularities of the density function, but due to the partialness of our estimator to µ0 = 0. • This example shows that no regularity conditions on the density can prevent an estimator from violating (3). This possibility can only be avoided by placing restrictions on the sequence of estimators. • LeCam (1953) showed that for any sequence of estimators satisfying (2), the set of points in Θ violating (3) has Lebesgue measure zero. • Superefficiency shows that “parametric” models can be useful and justified when checking them in appropriate ways
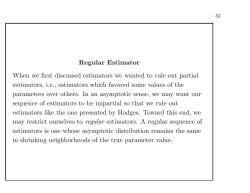
52 Regular Estimator When we first discussed estimators we wanted to rule out partial estimators,i.e.,estimators which favored some values of the parameters over others.In an asymptotic sense,we may want our sequence of estimators to be impartial so that we rule out estimators like the one presented by Hodges.Toward this end,we may restrict ourselves to regular estimators.A regular sequence of estimators is one whose asymptotic distribution remains the same in shrinking neighborhoods of the true parameter value
52 Regular Estimator When we first discussed estimators we wanted to rule out partial estimators, i.e., estimators which favored some values of the parameters over others. In an asymptotic sense, we may want our sequence of estimators to be impartial so that we rule out estimators like the one presented by Hodges. Toward this end, we may restrict ourselves to regular estimators. A regular sequence of estimators is one whose asymptotic distribution remains the same in shrinking neighborhoods of the true parameter value
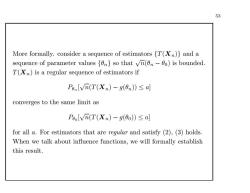
53 More formally.consider a sequence of estimators {T(Xn)}and a sequence of parameter values {on}so that vn(n-00)is bounded. T(Xn)is a regular sequence of estimators if Pan[v元(T(Xn)-g(0n))≤ad converges to the same limit as Pa[v元(T(Xn)-g(0o)≤ad for all a.For estimators that are regular and satisfy (2),(3)holds. When we talk about influence functions,we will formally establish this result
53 More formally. consider a sequence of estimators {T(Xn)} and a sequence of parameter values {θn} so that √n(θn − θ0) is bounded. T(Xn) is a regular sequence of estimators if Pθn [√n(T(Xn) − g(θn)) ≤ a] converges to the same limit as Pθ0 [√n(T(Xn) − g(θ0)) ≤ a] for all a. For estimators that are regular and satisfy (2), (3) holds. When we talk about influence functions, we will formally establish this result
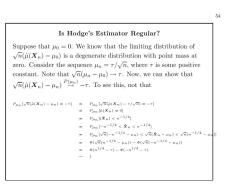
54 Is Hodge's Estimator Regular? Suppose that uo =0.We know that the limiting distribution of vn((n)-Lo)is a degenerate distribution with point mass at zero.Consider the sequence un=T/√元,where r is some positive constant.Note that vn(un-uo)T.Now,we can show that ()u).To see this,not that Pμn【元(a(xn)-hn)=-]=Pμn【v元(n(Xn)-T/√元)=-T] Pμn[m(Xn)=o] PunllXnl<n-1/4] = Pun【-n-1/4<xn<n-1/4] Pun元(-n-1/4-un)<V元(xn-un)<V元(n-1/4-u】 = Φ(W元(n-1/4-n)》-Φ(元(-n-1/4-un) Φ(n1/4-r)-Φ(-n1/4-r) 1
54 Is Hodge’s Estimator Regular? Suppose that µ0 = 0. We know that the limiting distribution of √n(ˆµ(Xn) − µ0) is a degenerate distribution with point mass at zero. Consider the sequence µn = τ /√n, where τ is some positive constant. Note that √n(µn − µ0) → τ. Now, we can show that √n(ˆµ(Xn) − µn) P (µn) → −τ. To see this, not that Pµn [√n(ˆµ(Xn) − µn) = −τ] = Pµn [√n(ˆµ(Xn) − τ/√n) = −τ] = Pµn [ˆµ(Xn) = 0] = Pµn [|X ¯ n| < n−1/4] = Pµn [−n−1/4 < X¯ n < n−1/4] = Pµn [√n(−n−1/4 − µn) < √n(X¯ n − µn) < √n(n−1/4 − µn)] = Φ(√n(n−1/4 − µn)) − Φ(√n(−n−1/4 − µn)) = Φ(n1/4 − τ) − Φ(−n1/4 − τ) → 1
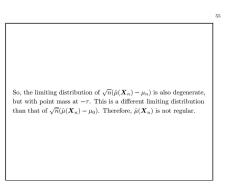
55 So,the limiting distribution of vn((Xn)-un)is also degenerate, but with point mass at -7.This is a different limiting distribution than that of vn((Xn)-uo).Therefore,(Xn)is not regular
55 So, the limiting distribution of √n(ˆµ(Xn) − µn) is also degenerate, but with point mass at −τ. This is a different limiting distribution than that of √n(ˆµ(Xn) − µ0). Therefore, ˆµ(Xn) is not regular
按次数下载不扣除下载券;
注册用户24小时内重复下载只扣除一次;
顺序:VIP每日次数-->可用次数-->下载券;
- 《数理统计》课程教学资源(参考资料)Large sample properties of MLE 02.pdf
- 《数理统计》课程教学资源(参考资料)Large sample properties of MLE 01.pdf
- 《数理统计》课程教学资源(参考资料)An Inconsistent maximum likelihood estimate.pdf
- 《数理统计》课程教学资源(参考资料)Maximum Likelihood - An Introduction.pdf
- 中国科学技术大学:《数理统计》课程教学资源(课件讲义)第五讲 点估计方法(二)极大似然估计方法.pdf
- 中国科学技术大学:《数理统计》课程教学资源(课件讲义)第四讲 点估计方法(一)矩估计方法.pdf
- 中国科学技术大学:《数理统计》课程教学资源(课件讲义)第三讲 指数族与充分完备统计量.pdf
- 中国科学技术大学:《数理统计》课程教学资源(课件讲义)第二讲 统计量的分布(抽样分布).pdf
- 《数理统计》课程教学资源(参考资料)Hoeffding's Indequality 证明中的一个不等式的证明 complementary.pdf
- 《数理统计》课程教学资源(参考资料)Glivenko-Cantelli 定理的证明.pdf
- 中国科学技术大学:《数理统计》课程教学资源(PPT课件讲稿)统计学——统计数据的描述.ppt
- 中国科学技术大学:《数理统计》课程教学资源(课件讲义)第一讲 总体与样本 What Statistics can do(主讲:张伟平).ppt
- 佛山科学技术学院:《生物统计附试验设计》课程电子教案(PPT课件讲稿)第六章 方差分析(二)第二节 单因素试验资料的方差分析、第三节 两因素试验资料的方差分析.ppt
- 佛山科学技术学院:《生物统计附试验设计》课程电子教案(PPT课件讲稿)第六章 方差分析(三)第四节 方差分析的数学模型与期望均方、第五节 数据转换.ppt
- 佛山科学技术学院:《生物统计附试验设计》课程电子教案(PPT课件讲稿)第六章 方差分析 Analysis of Variance(一)第一节 方差分析的基本原理与步骤.ppt
- 佛山科学技术学院:《生物统计附试验设计》课程电子教案(PPT课件讲稿)第七章 次数资料分析——X2检验.ppt
- 佛山科学技术学院:《生物统计附试验设计》课程电子教案(PPT课件讲稿)第四章 常用概率分布.ppt
- 佛山科学技术学院:《生物统计附试验设计》课程电子教案(PPT课件讲稿)第五章 假设检验(二).ppt
- 佛山科学技术学院:《生物统计附试验设计》课程电子教案(PPT课件讲稿)第五章 假设检验(一)均数差异显著性检验——t检验.ppt
- 佛山科学技术学院:《生物统计附试验设计》课程电子教案(PPT课件讲稿)第二章 资料的整理.ppt
- 《数理统计》课程教学资源(参考资料)Large sample properties of MLE 04.pdf
- 《数理统计》课程教学资源(参考资料)Large sample properties of MLE 05.pdf
- 《数理统计》课程教学资源(参考资料)THE MM, ME, ML, EL, EF AND GMM APPROACHES TO ESTIMATION - A SYNTHESIS.pdf
- 《数理统计》课程教学资源(参考资料)Another scratch proof of consistency and asymptotical normal of MLE.pdf
- 中国科学技术大学:《数理统计》课程教学资源(课件讲义)第六讲 点估计方法(三)一致最小方差无偏估计.pdf
- 中国科学技术大学:《数理统计》课程教学资源(课件讲义)第七讲 区间估计(一)置信区间.pdf
- 中国科学技术大学:《数理统计》课程教学资源(课件讲义)第八讲 区间估计(二)容忍区间.pdf
- 中国科学技术大学:《数理统计》课程教学资源(课件讲义)第九讲 参数假设检验(一).pdf
- 《数理统计》课程教学资源(参考资料)How do we do hypothesis testing.pdf
- 中国科学技术大学:《数理统计》课程教学资源(课件讲义)第十讲 参数假设检验(二).pdf
- 中国科学技术大学:《数理统计》课程教学资源(课件讲义)第十一讲 参数假设检验(三).pdf
- 《数理统计》课程教学资源(参考资料)Likelihood Ratio, Wald, and(Rao)Score Tests.pdf
- 中国科学技术大学:《数理统计》课程教学资源(课件讲义)第十二讲 非参数检验(一).pdf
- 中国科学技术大学:《数理统计》课程教学资源(课件讲义)第十二讲 非参数检验(二).pdf
- 中国科学技术大学:《数理统计》课程教学资源(课件讲义)第十三讲 Bayes统计初步(Bayes方法和统计决策理论).pdf
- 《数理统计》课程教学资源(参考资料)THE FORMAL DEFINITION OF REFERENCE PRIORS, ANNALS, 2009.pdf
- 《数理统计》课程教学资源(参考资料)Bayes Factor - What They Are and What They Are Not.pdf
- 中国科学技术大学:《数理统计》课程教学资源(课件讲义)第十四讲 回归分析(线性回归模型).pdf
- 中国科学技术大学:《实用统计软件》课程课件讲义(统计计算与软件)第一讲 R语言基础(一).pdf
- 《实用统计软件》课程教学资源(阅读材料)R for beginner(中文第二版,共七章).pdf