《数理统计》课程教学资源(参考资料)Likelihood Ratio, Wald, and(Rao)Score Tests
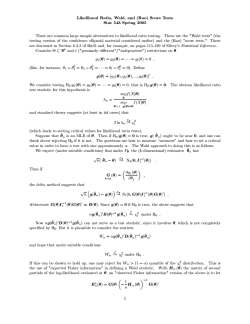
Likelihood Ratio,Wald,and (Rao)Score Tests Stat 543 Spring 2005 There are common large sample alternatives to likelihood ratio testing.These are the "Wald tests"(the testing version of the confidence ellipsoid material considered earlier)and the (Rao)"score tests.These are discussed in Section 6.3.2 of B&D and,for example,on pages 115-120 of Silvey's Statistical Inference. Consider cRand I("genuinely different"/"independent")restrictions on 0 g1(8)=92(8)=·=g(8)=0, (like,for instance,0=026=...=00=0).Define g0)÷(g1(0),92(0),9(0)'. We consider testing Ho:g(0)=g2(0)=...=g()=0,that is Ho:g()=0.The obvious likelihood ratio test statistic for this hypothesis is supf(X 0) 入n= sup f(X 0) 0s.t.g(0)=0 and standard theory suggests(at least in iid cases)that 2InAnx (which leads to setting critical values for likelihood ratio tests). Suppose that on is an MLE of 0.Then if Ho:g(0)=0 is true,g()ought to be near 0,and one can think about rejecting Ho if it is not.The questions are how to measure "nearness"and how to set a critical value in order to have a test with size approximately a.The Wald approach to doing this is as follows. We expect (under suitable conditions)that under Po the (k-dimensional)estimator On has √元(an-8)9Nk(0,I-1(8) Then if G(0)÷ 0g:(θ) kx ∂8, the delta method suggests that V元(g(an)-g(0))9N(0,G(8)I(0)G(a. Abbreviate G()I()G(0)'as B().Since g(0)=0 if Ho is true,the above suggests that ng(0)'B()-1g(0)x under Ho. Now ng(n)'B()-1g(n)can not serve as a test statistic,since it involves 0,which is not completely specified by Ho.But it is plausible to consider the statistic Wm÷ng(an)'B(0n)-1g(an)) and hope that under suitable conditions WnSX好under Ho· If this can be shown to hold up,one may reject for Wn >(1-a)quantile of the x?distribution.This is the use of "expected Fisher information"in defining a Wald statistic.With Hn()the matrix of second partials of the log-likelihood evaluated at 0,an "observed Fisher information"version of the above is to let -1 B(0)=c(0)-=Hn() G()
Likelihood Ratio, Wald, and (Rao) Score Tests Stat 543 Spring 2005 There are common large sample alternatives to likelihood ratio testing. These are the "Wald tests" (the testing version of the confidence ellipsoid material considered earlier) and the (Rao) "score tests." These are discussed in Section 6.3.2 of B&D and, for example, on pages 115-120 of Silvey’s Statistical Inference. Consider Θ ⊂ (1 − α) quantile of the χ2 l distribution. This is the use of "expected Fisher information" in defining a Wald statistic. With Hn (θ) the matrix of second partials of the log-likelihood evaluated at θ, an "observed Fisher information" version of the above is to let B∗ n(θ) = G(θ) µ − 1 n Hn (θ) ¶−1 G(θ) 0 1
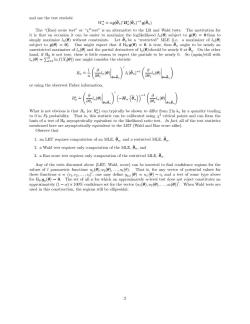
and use the test statistic Wi =ng(on)'Bi(on)-1g(on) The "(Rao)score test"or "x2test"is an alternative to the LR and Wald tests.The motivation for it is that on occasion it can be easier to maximize the loglikelihood In()subject to g(0)=0than to simply maximize In(0)without constraints.Let On be a "restricted"MLE (i.e.a maximizer of In(0) subject to g()=0).One might expect that if Ho:g()=0 is true,then On ought to be nearly an unrestricted maximizer of l(0)and the partial derivatives of In()should be nearly 0 at en.On the other hand,if Ho is not true,there is little reason to expect the partials to be nearly 0.So (again/still with ()In f())one might consider the statistic or using the observed Fisher information. Rn= (品aol)(a》'(0la) What is not obvious is that Rn (or R)can typically be shown to differ from 2In An by a quantity tending to 0 in Po probability.That is,this statistic can be calibrated using x2 critical points and can form the basis of a test of Ho asymptotically equivalent to the likelihood ratio test.In fact,all of the test statistics mentioned here are asymptotically equivalent to the LRT (Wald and Rao score alike). Observe that 1.an LRT requires computation of an MLE,0n,and a restricted MLE,, 2.a Wald test requires only computation of the MLE,On,and 3.a Rao score test requires only computation of the restricted MLE,On. Any of the tests discussed above (LRT,Wald,score)can be inverted to find confidence regions for the values of l parametric functions u(),u2(),...,u().That is,for any vector of potential values for these functions c=(c1,c2,...,c),one may define ge.i()=ui(0)-ci and a test of some type above for Ho:g(0)=0.The set of all c for which an approximately a-level test does not reject constitutes an approximately (1-a)x 100%confidence set for the vector (u1(0),u2(0),...,u())'.When Wald tests are used in this construction,the regions will be ellipsoidal. 2
and use the test statistic W∗ n . = ng(θ ˆn) 0 B∗ n(θ ˆn) −1g(θ ˆn) The “(Rao) score test” or “χ2 test” is an alternative to the LR and Wald tests. The motivation for it is that on occasion it can be easier to maximize the loglikelihood ln(θ) subject to g(θ) = 0 than to simply maximize ln(θ) without constraints. Let θ ˜n be a “restricted” MLE (i.e. a maximizer of ln(θ) subject to g(θ) = 0). One might expect that if H0:g(θ) = 0 is true, then θ ˜n ought to be nearly an unrestricted maximizer of ln(θ) and the partial derivatives of ln(θ)should be nearly 0 at θ ˜n. On the other hand, if H0 is not true, there is little reason to expect the partials to be nearly 0. So (again/still with ln(θ) = Pn i=1 ln f(Xi|θ)) one might consider the statistic Rn . = 1 n à ∂ ∂θi ln(θ) ¯ ¯ ¯ ¯ θ=θ ˜n !0 I1(θ ˜n) −1 à ∂ ∂θi ln(θ) ¯ ¯ ¯ ¯ θ=θ ˜n ! or using the observed Fisher information, R∗ n . = à ∂ ∂θi ln(θ) ¯ ¯ ¯ ¯ θ=θ ˜n !0 ³ −Hn ³ θ ˜n ´´−1 à ∂ ∂θi ln(θ) ¯ ¯ ¯ ¯ θ=θ ˜n ! What is not obvious is that Rn (or R∗ n) can typically be shown to differ from 2 ln λn by a quantity tending to 0 in Pθ probability. That is, this statistic can be calibrated using χ2 critical points and can form the basis of a test of H0 asymptotically equivalent to the likelihood ratio test. In fact, all of the test statistics mentioned here are asymptotically equivalent to the LRT (Wald and Rao score alike). Observe that 1. an LRT requires computation of an MLE, θ ˆn, and a restricted MLE, θ ˜n, 2. a Wald test requires only computation of the MLE, θ ˆn, and 3. a Rao score test requires only computation of the restricted MLE, θ ˜n. Any of the tests discussed above (LRT, Wald, score) can be inverted to find confidence regions for the values of l parametric functions u1(θ), u2(θ),...,ul(θ). That is, for any vector of potential values for these functions c = (c1, c2,...,cl) 0 , one may define gc,i (θ) = ui (θ) − ci and a test of some type above for H0:gc(θ) = 0. The set of all c for which an approximately α-level test does not reject constitutes an approximately (1 − α) × 100% confidence set for the vector (u1(θ), u2(θ),...,ul(θ))0 . When Wald tests are used in this construction, the regions will be ellipsoidal. 2
按次数下载不扣除下载券;
注册用户24小时内重复下载只扣除一次;
顺序:VIP每日次数-->可用次数-->下载券;
- 中国科学技术大学:《数理统计》课程教学资源(课件讲义)第十一讲 参数假设检验(三).pdf
- 中国科学技术大学:《数理统计》课程教学资源(课件讲义)第十讲 参数假设检验(二).pdf
- 《数理统计》课程教学资源(参考资料)How do we do hypothesis testing.pdf
- 中国科学技术大学:《数理统计》课程教学资源(课件讲义)第九讲 参数假设检验(一).pdf
- 中国科学技术大学:《数理统计》课程教学资源(课件讲义)第八讲 区间估计(二)容忍区间.pdf
- 中国科学技术大学:《数理统计》课程教学资源(课件讲义)第七讲 区间估计(一)置信区间.pdf
- 中国科学技术大学:《数理统计》课程教学资源(课件讲义)第六讲 点估计方法(三)一致最小方差无偏估计.pdf
- 《数理统计》课程教学资源(参考资料)Another scratch proof of consistency and asymptotical normal of MLE.pdf
- 《数理统计》课程教学资源(参考资料)THE MM, ME, ML, EL, EF AND GMM APPROACHES TO ESTIMATION - A SYNTHESIS.pdf
- 《数理统计》课程教学资源(参考资料)Large sample properties of MLE 05.pdf
- 《数理统计》课程教学资源(参考资料)Large sample properties of MLE 04.pdf
- 《数理统计》课程教学资源(参考资料)Large sample properties of MLE 03.pdf
- 《数理统计》课程教学资源(参考资料)Large sample properties of MLE 02.pdf
- 《数理统计》课程教学资源(参考资料)Large sample properties of MLE 01.pdf
- 《数理统计》课程教学资源(参考资料)An Inconsistent maximum likelihood estimate.pdf
- 《数理统计》课程教学资源(参考资料)Maximum Likelihood - An Introduction.pdf
- 中国科学技术大学:《数理统计》课程教学资源(课件讲义)第五讲 点估计方法(二)极大似然估计方法.pdf
- 中国科学技术大学:《数理统计》课程教学资源(课件讲义)第四讲 点估计方法(一)矩估计方法.pdf
- 中国科学技术大学:《数理统计》课程教学资源(课件讲义)第三讲 指数族与充分完备统计量.pdf
- 中国科学技术大学:《数理统计》课程教学资源(课件讲义)第二讲 统计量的分布(抽样分布).pdf
- 中国科学技术大学:《数理统计》课程教学资源(课件讲义)第十二讲 非参数检验(一).pdf
- 中国科学技术大学:《数理统计》课程教学资源(课件讲义)第十二讲 非参数检验(二).pdf
- 中国科学技术大学:《数理统计》课程教学资源(课件讲义)第十三讲 Bayes统计初步(Bayes方法和统计决策理论).pdf
- 《数理统计》课程教学资源(参考资料)THE FORMAL DEFINITION OF REFERENCE PRIORS, ANNALS, 2009.pdf
- 《数理统计》课程教学资源(参考资料)Bayes Factor - What They Are and What They Are Not.pdf
- 中国科学技术大学:《数理统计》课程教学资源(课件讲义)第十四讲 回归分析(线性回归模型).pdf
- 中国科学技术大学:《实用统计软件》课程课件讲义(统计计算与软件)第一讲 R语言基础(一).pdf
- 《实用统计软件》课程教学资源(阅读材料)R for beginner(中文第二版,共七章).pdf
- 中国科学技术大学:《实用统计软件》课程课件讲义(统计计算与软件)第二讲 R语言基础(二).pdf
- 中国科学技术大学:《实用统计软件》课程课件讲义(统计计算与软件)第三讲 LaTeX科技论文排版系统.pdf
- 《实用统计软件》课程教学资源(阅读材料)一份不太简短的LATEX 2ε介绍.pdf
- 中国科学技术大学:《实用统计软件》课程课件讲义(统计计算与软件)第四讲 随机数产生方法.pdf
- 中国科学技术大学:《实用统计软件》课程课件讲义(统计计算与软件)第五讲 Monte Carlo积分和方差减少技术.pdf
- 《实用统计软件》课程教学资源(阅读材料)多元分类问题中的应用 Variance Reduction with Monte Carlo Estimates of Error Rates in Multivariate Classication.pdf
- 《实用统计软件》课程教学资源(阅读材料)图像合成方面应用的一个介绍 Monte Carlo Integration.ppt
- 中国科学技术大学:《实用统计软件》课程课件讲义(统计计算与软件)第六讲 Monte Carlo方法在统计推断中的应用.pdf
- 中国科学技术大学:《实用统计软件》课程课件讲义(统计计算与软件)第七讲 Boostrap方法和Jackknife方法(自助和刀切).pdf
- 《实用统计软件》课程教学资源(阅读材料)T. DiCiccio and B.Efron(1996), Bootstrap Confidence Intervals, Statistical Science, 3,189-228.pdf
- 中国科学技术大学:《实用统计软件》课程课件讲义(统计计算与软件)第八讲 Markov Chain Monte Carlo(一)马尔科夫蒙特卡罗方法.pdf
- 《实用统计软件》课程教学资源(阅读材料)A History of Markov Chain Monte Carlo——Subjective Recollections from Incomplete Data.pdf