电子科技大学:《数据分析与数据挖掘 Data Analysis and Data Mining》课程教学资源(课件讲稿)Lecture 03 Regression Analysis and Classification
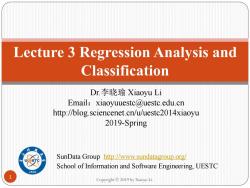
Lecture 3 Regression Analysis and Classification Dr.李晓瑜Xiaoyu Li Email:xiaoyuuestc@uestc.edu.cn http://blog.sciencenet.cn/u/uestc2014xiaoyu 2019-Spring SunData Group http://www.sundatagroup.org School of Information and Software Engineering,UESTC 1966 Copyright2019 by Xiaoyu Li
Dr.李晓瑜 Xiaoyu Li Email:xiaoyuuestc@uestc.edu.cn http://blog.sciencenet.cn/u/uestc2014xiaoyu 2019-Spring Lecture 3 Regression Analysis and Classification SunData Group http://www.sundatagroup.org/ School of Information and Software Engineering, UESTC Copyright © 2019 by Xiaoyu Li. 1
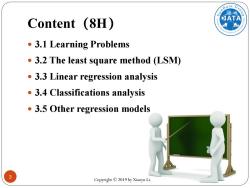
S3 Da t a G实o320 Content (8H) ATA 3.1 Learning Problems 3.2 The least square method (LSM) 3.3 Linear regression analysis .3.4 Classifications analysis 3.5 Other regression models 3 Copyright 2019 by Xiaoyu Li
Content(8H) 3.1 Learning Problems 3.2 The least square method (LSM) 3.3 Linear regression analysis 3.4 Classifications analysis 3.5 Other regression models Copyright © 2019 by Xiaoyu Li. 3
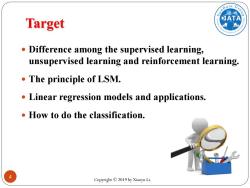
sunbata Groun Target Difference among the supervised learning, unsupervised learning and reinforcement learning. ·The principle of LSM. Linear regression models and applications. How to do the classification. 4 Copyright 2019 by Xiaoyu Li
Target Difference among the supervised learning, unsupervised learning and reinforcement learning. The principle of LSM. Linear regression models and applications. How to do the classification. Copyright © 2019 by Xiaoyu Li. 4
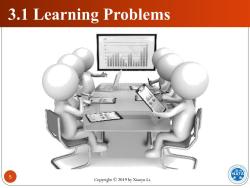
3.1 Learning Problems 5 DATA Copyright 2019 by Xiaoyu Li
Copyright © 2019 by Xiaoyu Li. 5 3.1 Learning Problems
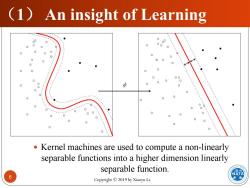
(1)An insight of Learning 0 00 0 0 0 0 ● Q 0 ● 0 0 0 0 Kernel machines are used to compute a non-linearly separable functions into a higher dimension linearly separable function. DATA 6 Copyright 2019 by Xiaoyu Li
Copyright © 2019 by Xiaoyu Li. 6 (1) An insight of Learning Kernel machines are used to compute a non-linearly separable functions into a higher dimension linearly separable function
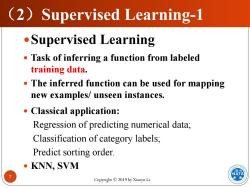
(2)Supervised Learning-1 ●Supervised Learning Task of inferring a function from labeled training data. The inferred function can be used for mapping new examples/unseen instances. Classical application: Regression of predicting numerical data; Classification of category labels; Predict sorting order. ·KNN,SVM DATA Copyright 2019 by Xiaoyu Li
Copyright © 2019 by Xiaoyu Li. 7 (2)Supervised Learning-1 Supervised Learning Task of inferring a function from labeled training data. The inferred function can be used for mapping new examples/ unseen instances. Classical application: Regression of predicting numerical data; Classification of category labels; Predict sorting order. KNN, SVM
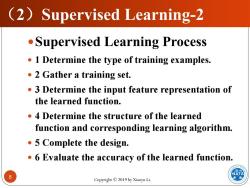
(2)Supervised Learning-2 .Supervised Learning Process 1 Determine the type of training examples. .2 Gather a training set. 3 Determine the input feature representation of the learned function. .4 Determine the structure of the learned function and corresponding learning algorithm .5 Complete the design. 6 Evaluate the accuracy of the learned function. ATA 8 Copyright 2019 by Xiaoyu Li
Copyright © 2019 by Xiaoyu Li. 8 (2)Supervised Learning-2 Supervised Learning Process 1 Determine the type of training examples. 2 Gather a training set. 3 Determine the input feature representation of the learned function. 4 Determine the structure of the learned function and corresponding learning algorithm. 5 Complete the design. 6 Evaluate the accuracy of the learned function
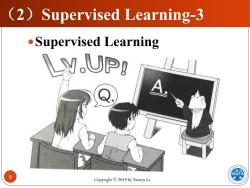
(2)Supervised Learning-3 ●Supervised Learning YUPA DATA 9 Copyright 2019 by Xiaoyu Li
Copyright © 2019 by Xiaoyu Li. 9 (2)Supervised Learning-3 Supervised Learning
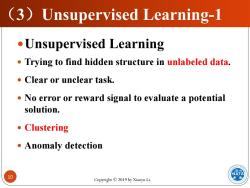
(3)Unsupervised Learning-1 Unsupervised Learning Trying to find hidden structure in unlabeled data. Clear or unclear task. No error or reward signal to evaluate a potential solution. ·Clustering .Anomaly detection 10 DATA Copyright 2019 by Xiaoyu Li
Copyright © 2019 by Xiaoyu Li. 10 (3)Unsupervised Learning-1 Unsupervised Learning Trying to find hidden structure in unlabeled data. Clear or unclear task. No error or reward signal to evaluate a potential solution. Clustering Anomaly detection
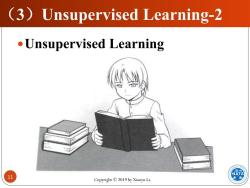
(3)Unsupervised Learning-2 Unsupervised Learning 11 DATA Copyright 2019 by Xiaoyu Li
Copyright © 2019 by Xiaoyu Li. 11 Unsupervised Learning (3)Unsupervised Learning-2
按次数下载不扣除下载券;
注册用户24小时内重复下载只扣除一次;
顺序:VIP每日次数-->可用次数-->下载券;
- 电子科技大学:《数据分析与数据挖掘 Data Analysis and Data Mining》课程教学资源(课件讲稿)Lecture 03 Regression Analysis(Logistic Regression).pdf
- 电子科技大学:《数据分析与数据挖掘 Data Analysis and Data Mining》课程教学资源(课件讲稿)Lecture 02 Raw Data Analysis and Pre-processing(2.1-2.4).pdf
- 电子科技大学:《数据分析与数据挖掘 Data Analysis and Data Mining》课程教学资源(课件讲稿)Lecture 02 Raw Data Analysis and Pre-processing(2.5-2.7).pdf
- 电子科技大学:《数据分析与数据挖掘 Data Analysis and Data Mining》课程教学资源(课件讲稿)Lecture 01 Overview Data Analysis and Data Mining(李晓瑜).pdf
- 电子科技大学:《数据分析与数据挖掘 Data Analysis and Data Mining》课程教学资源(课件讲稿)量子降维算法.pdf
- 电子科技大学:《数据分析与数据挖掘 Data Analysis and Data Mining》课程教学资源(课件讲稿)量子神经网络(Neural Network,NN).pdf
- 电子科技大学:《数据分析与数据挖掘 Data Analysis and Data Mining》课程教学资源(课件讲稿)量子支持向量机(support vector machine, SVM).pdf
- 电子科技大学:《数据分析与数据挖掘 Data Analysis and Data Mining》课程教学资源(课件讲稿)量子机器学习(量子K-means算法).pdf
- 电子科技大学:《数据分析与数据挖掘 Data Analysis and Data Mining》课程教学资源(课件讲稿)隐马尔科夫算法.pdf
- 电子科技大学:《数据分析与数据挖掘 Data Analysis and Data Mining》课程教学资源(课件讲稿)降维算法.pdf
- 电子科技大学:《数据分析与数据挖掘 Data Analysis and Data Mining》课程教学资源(课件讲稿)分类算法(朱钦圣).pdf
- 电子科技大学:《数据分析与数据挖掘 Data Analysis and Data Mining》课程教学资源(课件讲稿)聚类算法.pdf
- 电子科技大学:《数据分析与数据挖掘 Data Analysis and Data Mining》课程教学资源(课件讲稿)量子力学.pdf
- 电子科技大学:《数据分析与数据挖掘 Data Analysis and Data Mining》课程教学资源(课件讲稿)决策树.pdf
- 电子科技大学:《数据分析与数据挖掘 Data Analysis and Data Mining》课程教学资源(课件讲稿)线性模型.pdf
- 电子科技大学:《数据分析与数据挖掘 Data Analysis and Data Mining》课程教学资源(课件讲稿)模型评估与选择.pdf
- 电子科技大学:《数据分析与数据挖掘 Data Analysis and Data Mining》课程教学资源(课件讲稿)绪论.pdf
- 南京大学:《软件工程 Software Engineering》课程教学资源(PPT课件讲稿)Part 25 软件开发的新方法 New Methodology(Agile方法).ppt
- 南京大学:《软件工程 Software Engineering》课程教学资源(PPT课件讲稿)Part 24 软件工程中的高级课题 Advanced Topics in Software Engineering.ppt
- 南京大学:《软件工程 Software Engineering》课程教学资源(PPT课件讲稿)Part 23 软件过程、管理与质量 Software Process, Management, and Quality.ppt
- 电子科技大学:《数据分析与数据挖掘 Data Analysis and Data Mining》课程教学资源(课件讲稿)Lecture 05 Clustering Analysis.pdf
- 电子科技大学:《数据分析与数据挖掘 Data Analysis and Data Mining》课程教学资源(课件讲稿)Lecture 04 Association Rules of Data Reasoning(Apriori Algorithm、Improve of Apriori Algorithm).pdf
- 电子科技大学:《数据分析与数据挖掘 Data Analysis and Data Mining》课程教学资源(课件讲稿)Lecture 04 Association Rules of Data Reasoning(FP-growth Algorithm).pdf
- 电子科技大学:《数据分析与数据挖掘 Data Analysis and Data Mining》课程教学资源(课件讲稿)Lecture 04 Association Rules of Data Reasoning.pdf
- 电子科技大学:《数据分析与数据挖掘 Data Analysis and Data Mining》课程教学资源(课件讲稿)Lecture 06 Classification.pdf
- 电子科技大学:《算法设计与分析 Algorithms Design and Analysis》课程教学资源(课件讲稿)第一章 算法概述 Algorithm Introduction(刘瑶、陈佳).pdf
- 电子科技大学:《算法设计与分析 Algorithms Design and Analysis》课程教学资源(课件讲稿)第二章 递归与分治策略.pdf
- 电子科技大学:《算法设计与分析 Algorithms Design and Analysis》课程教学资源(课件讲稿)第三章 动态规划 Dynamic Programming.pdf
- 电子科技大学:《算法设计与分析 Algorithms Design and Analysis》课程教学资源(课件讲稿)第四章 贪心算法(Greedy Algorithm).pdf
- 电子科技大学:《算法设计与分析 Algorithms Design and Analysis》课程教学资源(课件讲稿)第五章 回朔法(Backtracking Algorithm).pdf
- 电子科技大学:《算法设计与分析 Algorithms Design and Analysis》课程教学资源(课件讲稿)第六章 分支限界法(Branch and Bound Method).pdf
- 上饶师范学院:《数据库系统原理 An Introduction to Database System》课程教学资源(电子教案,颜清).doc
- 电子科技大学:《算法设计与分析 Design and Analysis of Algorithms》研究生课程教学资源(课件讲稿,英文版)01 Introduction(肖鸣宇).pdf
- 电子科技大学:《算法设计与分析 Design and Analysis of Algorithms》研究生课程教学资源(课件讲稿,英文版)Stable Matching.pdf
- 电子科技大学:《算法设计与分析 Design and Analysis of Algorithms》研究生课程教学资源(课件讲稿,英文版)02 Basics of algorithm design & analysis.pdf
- 电子科技大学:《算法设计与分析 Design and Analysis of Algorithms》研究生课程教学资源(课件讲稿,英文版)03 Maximum Flow.pdf
- 电子科技大学:《算法设计与分析 Design and Analysis of Algorithms》研究生课程教学资源(课件讲稿,英文版)04 NP and Computational Intractability.pdf
- 电子科技大学:《算法设计与分析 Design and Analysis of Algorithms》研究生课程教学资源(课件讲稿,英文版)05 Approximation Algorithms.pdf
- 电子科技大学:《现代密码理论 Modern Cryptographic Theory》课程教学资源(课件讲稿)第1章 概述(李发根).pdf
- 电子科技大学:《现代密码理论 Modern Cryptographic Theory》课程教学资源(课件讲稿)第2章 古典密码.pdf