电子科技大学:《数据分析与数据挖掘 Data Analysis and Data Mining》课程教学资源(课件讲稿)Lecture 02 Raw Data Analysis and Pre-processing(2.5-2.7)
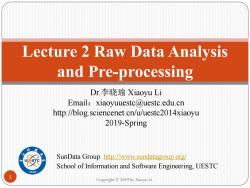
Lecture 2 Raw Data Analysis and Pre-processing Dr.李晓瑜Xiaoyu Li Email:xiaoyuuestc@uestc.edu.cn http://blog.sciencenet.cn/u/uestc2014xiaoyu 2019-Spring SunData Group http://www.sundatagroup.org School of Information and Software Engineering,UESTC 1966 Copyright2019 by Xiaoyu Li
Dr.李晓瑜 Xiaoyu Li Email:xiaoyuuestc@uestc.edu.cn http://blog.sciencenet.cn/u/uestc2014xiaoyu 2019-Spring Lecture 2 Raw Data Analysis and Pre-processing SunData Group http://www.sundatagroup.org/ School of Information and Software Engineering, UESTC Copyright © 2019 by Xiaoyu Li. 1
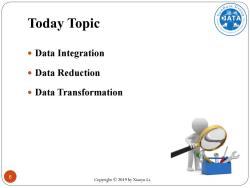
飞黄多2t3美爱爱) Today Topic DATA Data Integration ●Data reduction ●Data Transformation 6 Copyright 2019 by Xiaoyu Li
Today Topic Data Integration Data Reduction Data Transformation Copyright © 2019 by Xiaoyu Li. 6
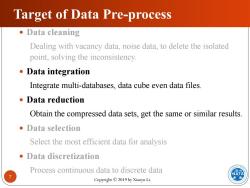
Target of Data Pre-process ·Data cleaning Dealing with vacancy data,noise data,to delete the isolated point,solving the inconsistency. Data integration Integrate multi-databases,data cube even data files. Data reduction Obtain the compressed data sets,get the same or similar results. ●Data selection Select the most efficient data for analysis ●Data discretization Process continuous data to discrete data 7 DATA Copyright 2019 by Xiaoyu Li
Copyright © 2019 by Xiaoyu Li. 7 Target of Data Pre-process Data cleaning Dealing with vacancy data, noise data, to delete the isolated point, solving the inconsistency. Data integration Integrate multi-databases, data cube even data files. Data reduction Obtain the compressed data sets, get the same or similar results. Data selection Select the most efficient data for analysis Data discretization Process continuous data to discrete data
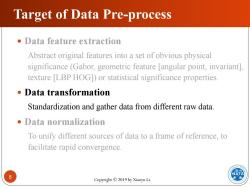
Target of Data Pre-process Data feature extraction Abstract original features into a set of obvious physical significance (Gabor,geometric feature [angular point,invariant]. texture [LBP HOG])or statistical significance properties. 。Data transformation Standardization and gather data from different raw data. ●Data normalization To unify different sources of data to a frame of reference,to facilitate rapid convergence. DATA 8 Copyright 2019 by Xiaoyu Li
Copyright © 2019 by Xiaoyu Li. 8 Target of Data Pre-process Data feature extraction Abstract original features into a set of obvious physical significance (Gabor, geometric feature [angular point, invariant], texture [LBP HOG]) or statistical significance properties. Data transformation Standardization and gather data from different raw data. Data normalization To unify different sources of data to a frame of reference, to facilitate rapid convergence
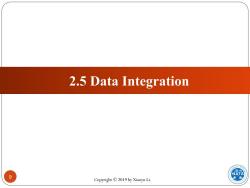
2.5 Data Integration DATA 9 Copyright 2019 by Xiaoyu Li
Copyright © 2019 by Xiaoyu Li. 9 2.5 Data Integration
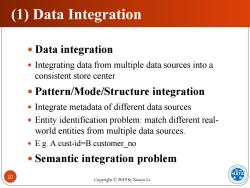
(1)Data Integration ●Data integration Integrating data from multiple data sources into a consistent store center Pattern/Mode/Structure integration Integrate metadata of different data sources Entity identification problem:match different real- world entities from multiple data sources. .E.g.A.cust-id=B.customer no Semantic integration problem 10 DATA Copyright 2019 by Xiaoyu Li
Copyright © 2019 by Xiaoyu Li. 10 (1) Data Integration Data integration Integrating data from multiple data sources into a consistent store center Pattern/Mode/Structure integration Integrate metadata of different data sources Entity identification problem: match different realworld entities from multiple data sources. E.g. A.cust-id=B.customer_no Semantic integration problem
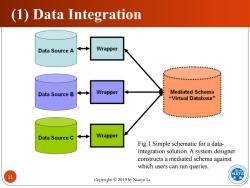
(1)Data Integration Data Source A Wrapper Data Source B Wrapper Mediated Schema “Virtual Database” Data Source C Wrapper Fig.1 Simple schematic for a data- integration solution.A system designer constructs a mediated schema against which users can run queries. 11 Copyright 2019 by Xiaoyu Li
Copyright © 2019 by Xiaoyu Li. 11 (1) Data Integration Fig.1 Simple schematic for a dataintegration solution. A system designer constructs a mediated schema against which users can run queries
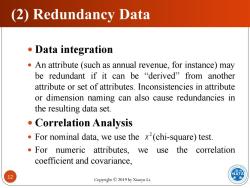
(2)Redundancy Data Data integration An attribute (such as annual revenue,for instance)may be redundant if it can be "derived"from another attribute or set of attributes.Inconsistencies in attribute or dimension naming can also cause redundancies in the resulting data set. Correlation Analysis For nominal data,we use the x(chi-square)test For numeric attributes,we use t the correlation coefficient and covariance, ATA 12 Copyright 2019 by Xiaoyu Li
Copyright © 2019 by Xiaoyu Li. 12 (2) Redundancy Data Data integration An attribute (such as annual revenue, for instance) may be redundant if it can be “derived” from another attribute or set of attributes. Inconsistencies in attribute or dimension naming can also cause redundancies in the resulting data set. Correlation Analysis For nominal data, we use the (chi-square) test. For numeric attributes, we use the correlation coefficient and covariance
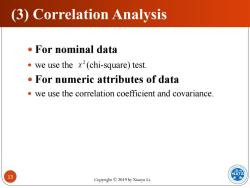
(3)Correlation Analysis For nominal data we use the x2(chi-square)test. For numeric attributes of data we use the correlation coefficient and covariance. 13 DATA Copyright 2019 by Xiaoyu Li
Copyright © 2019 by Xiaoyu Li. 13 (3) Correlation Analysis For nominal data we use the (chi-square) test. For numeric attributes of data we use the correlation coefficient and covariance
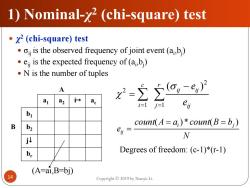
1)Nominal-x2 (chi-square)test .x2(chi-square)test .is the observed frequency of joint event (aib) eis the expected frequency of (ab) N is the number of tuples A x2-2a,e a1 a2 i c i=1 i=1 b1 B b2 count(A=a;)*couni(B=b) ji N br Degrees of freedom:(c-1)*(r-1) (A=ai,B=bj) 14 Copyright C 2019 by Xiaoyu Li
Copyright © 2019 by Xiaoyu Li. 14 χ 2 (chi-square) test σij is the observed frequency of joint event (ai ,bj ) eij is the expected frequency of (ai ,bj ) N is the number of tuples A a1 a2 i ac b1 B b2 j br (A=ai,B=bj) = = − = r j ij ij ij c i e e 1 2 1 2 ( ) N count A a count B b e i j ij ( = ) * ( = ) = Degrees of freedom: (c-1)*(r-1) 1) Nominal-χ 2 (chi-square) test
按次数下载不扣除下载券;
注册用户24小时内重复下载只扣除一次;
顺序:VIP每日次数-->可用次数-->下载券;
- 电子科技大学:《数据分析与数据挖掘 Data Analysis and Data Mining》课程教学资源(课件讲稿)Lecture 01 Overview Data Analysis and Data Mining(李晓瑜).pdf
- 电子科技大学:《数据分析与数据挖掘 Data Analysis and Data Mining》课程教学资源(课件讲稿)量子降维算法.pdf
- 电子科技大学:《数据分析与数据挖掘 Data Analysis and Data Mining》课程教学资源(课件讲稿)量子神经网络(Neural Network,NN).pdf
- 电子科技大学:《数据分析与数据挖掘 Data Analysis and Data Mining》课程教学资源(课件讲稿)量子支持向量机(support vector machine, SVM).pdf
- 电子科技大学:《数据分析与数据挖掘 Data Analysis and Data Mining》课程教学资源(课件讲稿)量子机器学习(量子K-means算法).pdf
- 电子科技大学:《数据分析与数据挖掘 Data Analysis and Data Mining》课程教学资源(课件讲稿)隐马尔科夫算法.pdf
- 电子科技大学:《数据分析与数据挖掘 Data Analysis and Data Mining》课程教学资源(课件讲稿)降维算法.pdf
- 电子科技大学:《数据分析与数据挖掘 Data Analysis and Data Mining》课程教学资源(课件讲稿)分类算法(朱钦圣).pdf
- 电子科技大学:《数据分析与数据挖掘 Data Analysis and Data Mining》课程教学资源(课件讲稿)聚类算法.pdf
- 电子科技大学:《数据分析与数据挖掘 Data Analysis and Data Mining》课程教学资源(课件讲稿)量子力学.pdf
- 电子科技大学:《数据分析与数据挖掘 Data Analysis and Data Mining》课程教学资源(课件讲稿)决策树.pdf
- 电子科技大学:《数据分析与数据挖掘 Data Analysis and Data Mining》课程教学资源(课件讲稿)线性模型.pdf
- 电子科技大学:《数据分析与数据挖掘 Data Analysis and Data Mining》课程教学资源(课件讲稿)模型评估与选择.pdf
- 电子科技大学:《数据分析与数据挖掘 Data Analysis and Data Mining》课程教学资源(课件讲稿)绪论.pdf
- 南京大学:《软件工程 Software Engineering》课程教学资源(PPT课件讲稿)Part 25 软件开发的新方法 New Methodology(Agile方法).ppt
- 南京大学:《软件工程 Software Engineering》课程教学资源(PPT课件讲稿)Part 24 软件工程中的高级课题 Advanced Topics in Software Engineering.ppt
- 南京大学:《软件工程 Software Engineering》课程教学资源(PPT课件讲稿)Part 23 软件过程、管理与质量 Software Process, Management, and Quality.ppt
- 南京大学:《软件工程 Software Engineering》课程教学资源(PPT课件讲稿)Part 22 面向对象软件工程 Object-Oriented Software Engineering(Unified Modeling Language, UML).ppt
- 南京大学:《软件工程 Software Engineering》课程教学资源(PPT课件讲稿)Part 21 传统软件工程方法 Conventional Methods for Software Engineering.ppt
- 《软件工程 Software Engineering》课程教学资源:软件文档编写指南.doc
- 电子科技大学:《数据分析与数据挖掘 Data Analysis and Data Mining》课程教学资源(课件讲稿)Lecture 02 Raw Data Analysis and Pre-processing(2.1-2.4).pdf
- 电子科技大学:《数据分析与数据挖掘 Data Analysis and Data Mining》课程教学资源(课件讲稿)Lecture 03 Regression Analysis(Logistic Regression).pdf
- 电子科技大学:《数据分析与数据挖掘 Data Analysis and Data Mining》课程教学资源(课件讲稿)Lecture 03 Regression Analysis and Classification.pdf
- 电子科技大学:《数据分析与数据挖掘 Data Analysis and Data Mining》课程教学资源(课件讲稿)Lecture 05 Clustering Analysis.pdf
- 电子科技大学:《数据分析与数据挖掘 Data Analysis and Data Mining》课程教学资源(课件讲稿)Lecture 04 Association Rules of Data Reasoning(Apriori Algorithm、Improve of Apriori Algorithm).pdf
- 电子科技大学:《数据分析与数据挖掘 Data Analysis and Data Mining》课程教学资源(课件讲稿)Lecture 04 Association Rules of Data Reasoning(FP-growth Algorithm).pdf
- 电子科技大学:《数据分析与数据挖掘 Data Analysis and Data Mining》课程教学资源(课件讲稿)Lecture 04 Association Rules of Data Reasoning.pdf
- 电子科技大学:《数据分析与数据挖掘 Data Analysis and Data Mining》课程教学资源(课件讲稿)Lecture 06 Classification.pdf
- 电子科技大学:《算法设计与分析 Algorithms Design and Analysis》课程教学资源(课件讲稿)第一章 算法概述 Algorithm Introduction(刘瑶、陈佳).pdf
- 电子科技大学:《算法设计与分析 Algorithms Design and Analysis》课程教学资源(课件讲稿)第二章 递归与分治策略.pdf
- 电子科技大学:《算法设计与分析 Algorithms Design and Analysis》课程教学资源(课件讲稿)第三章 动态规划 Dynamic Programming.pdf
- 电子科技大学:《算法设计与分析 Algorithms Design and Analysis》课程教学资源(课件讲稿)第四章 贪心算法(Greedy Algorithm).pdf
- 电子科技大学:《算法设计与分析 Algorithms Design and Analysis》课程教学资源(课件讲稿)第五章 回朔法(Backtracking Algorithm).pdf
- 电子科技大学:《算法设计与分析 Algorithms Design and Analysis》课程教学资源(课件讲稿)第六章 分支限界法(Branch and Bound Method).pdf
- 上饶师范学院:《数据库系统原理 An Introduction to Database System》课程教学资源(电子教案,颜清).doc
- 电子科技大学:《算法设计与分析 Design and Analysis of Algorithms》研究生课程教学资源(课件讲稿,英文版)01 Introduction(肖鸣宇).pdf
- 电子科技大学:《算法设计与分析 Design and Analysis of Algorithms》研究生课程教学资源(课件讲稿,英文版)Stable Matching.pdf
- 电子科技大学:《算法设计与分析 Design and Analysis of Algorithms》研究生课程教学资源(课件讲稿,英文版)02 Basics of algorithm design & analysis.pdf
- 电子科技大学:《算法设计与分析 Design and Analysis of Algorithms》研究生课程教学资源(课件讲稿,英文版)03 Maximum Flow.pdf
- 电子科技大学:《算法设计与分析 Design and Analysis of Algorithms》研究生课程教学资源(课件讲稿,英文版)04 NP and Computational Intractability.pdf