《大数据 Big Data》课程教学资源(参考文献)Learning to Hash for Big Data - A Tutorial
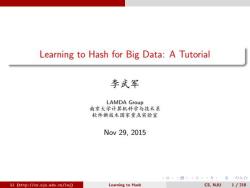
Learning to Hash for Big Data:A Tutorial 李武军 LAMDA Group 南京大学计算机科学与技术系 软件新技术国家重,点实验室 Nov29,2015 日卡*2元至Q0 Li (http://cs.nju.edu.cn/lvj) Learning to Hash CS.NJU 1/210
Learning to Hash for Big Data: A Tutorial o… LAMDA Group HÆåÆOéÅâÆÜE‚X ^á#E‚I[:¢ø Nov 29, 2015 Li (http://cs.nju.edu.cn/lwj) Learning to Hash CS, NJU 1 / 210
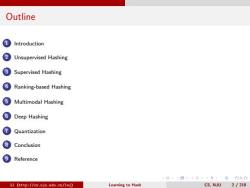
Outline Introduction ② Unsupervised Hashing Supervised Hashing Ranking-based Hashing Multimodal Hashing 6 Deep Hashing Quantization Conclusion ⑨ Reference 日卡回24元,互Q0 Li (http://cs.nju.edu.cn/lwj) Learning to Hash CS.NJU 2 /210
Outline 1 Introduction 2 Unsupervised Hashing 3 Supervised Hashing 4 Ranking-based Hashing 5 Multimodal Hashing 6 Deep Hashing 7 Quantization 8 Conclusion 9 Reference Li (http://cs.nju.edu.cn/lwj) Learning to Hash CS, NJU 2 / 210
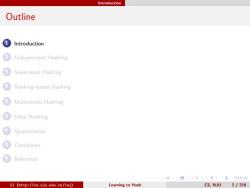
Introduction Outline Introduction Unsupervised Hashing Supervised Hashing Ranking-based Hashing Multimodal Hashing Deep Hashing Quantization Conclusion Reference 日卡三4元,互Q0 Li (http://cs.nju.edu.cn/lwj) Learning to Hash CS.NJU 3/210
Introduction Outline 1 Introduction 2 Unsupervised Hashing 3 Supervised Hashing 4 Ranking-based Hashing 5 Multimodal Hashing 6 Deep Hashing 7 Quantization 8 Conclusion 9 Reference Li (http://cs.nju.edu.cn/lwj) Learning to Hash CS, NJU 3 / 210
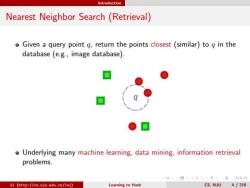
Introduction Nearest Neighbor Search (Retrieval) Given a query point g,return the points closest(similar)to g in the database (e.g.,image database). oUnderlying many machine learning,data mining,information retrieval problems. 口卡+得,二4元互)Q0 Li (http://cs.nju.edu.cn/lwj) Learning to Hash CS.NJU 4 /210
Introduction Nearest Neighbor Search (Retrieval) Given a query point q, return the points closest (similar) to q in the database (e.g., image database). Underlying many machine learning, data mining, information retrieval problems. Li (http://cs.nju.edu.cn/lwj) Learning to Hash CS, NJU 4 / 210
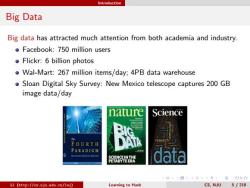
Introduction Big Data Big data has attracted much attention from both academia and industry. Facebook:750 million users Flickr:6 billion photos Wal-Mart:267 million items/day;4PB data warehouse oSloan Digital Sky Survey:New Mexico telescope captures 200 GB image data/day Science FOURTH PARADIGM data Li (http://cs.nju.edu.cn/lwj) Learning to Hash CS.NJU 5 /210
Introduction Big Data Big data has attracted much attention from both academia and industry. Facebook: 750 million users Flickr: 6 billion photos Wal-Mart: 267 million items/day; 4PB data warehouse Sloan Digital Sky Survey: New Mexico telescope captures 200 GB image data/day Li (http://cs.nju.edu.cn/lwj) Learning to Hash CS, NJU 5 / 210
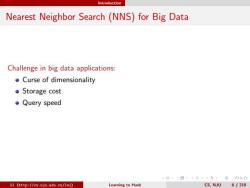
Introduction Nearest Neighbor Search(NNS)for Big Data Challenge in big data applications: o Curse of dimensionality ●Storage cost ●Query speed 日卡三4元,互Q0 Li (http://cs.nju.edu.cn/lwj) Learning to Hash CS.NJU 6 /210
Introduction Nearest Neighbor Search (NNS) for Big Data Challenge in big data applications: Curse of dimensionality Storage cost Query speed Li (http://cs.nju.edu.cn/lwj) Learning to Hash CS, NJU 6 / 210
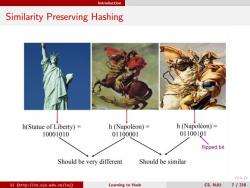
Introduction Similarity Preserving Hashing h(Statue of Liberty)= h(Napoleon)= h (Napoleon)= 10001010 01100001 011001Q1 flipped bit Should be very different Should be similar 0Q0 Li (http://cs.nju.edu.cn/lvj) Learning to Hash CS.NJU 7/210
Introduction Similarity Preserving Hashing Li (http://cs.nju.edu.cn/lwj) Learning to Hash CS, NJU 7 / 210
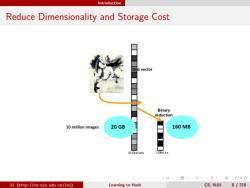
Introduction Reduce Dimensionality and Storage Cost Gist vector Binary reduction 10 million images 20 GB 160MB 口卡+得二4元互)Q0 Li (http://cs.nju.edu.cn/lwj) Learning to Hash CS.NJU 8/210
Introduction Reduce Dimensionality and Storage Cost Li (http://cs.nju.edu.cn/lwj) Learning to Hash CS, NJU 8 / 210
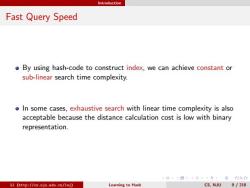
Introduction Fast Query Speed o By using hash-code to construct index,we can achieve constant or sub-linear search time complexity. o In some cases,exhaustive search with linear time complexity is also acceptable because the distance calculation cost is low with binary representation. 日卡三4元,互Q0 Li (http://cs.nju.edu.cn/lwj) Learning to Hash CS.NJU 9/210
Introduction Fast Query Speed By using hash-code to construct index, we can achieve constant or sub-linear search time complexity. In some cases, exhaustive search with linear time complexity is also acceptable because the distance calculation cost is low with binary representation. Li (http://cs.nju.edu.cn/lwj) Learning to Hash CS, NJU 9 / 210
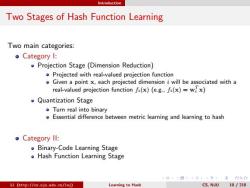
Introduction Two Stages of Hash Function Learning Two main categories: oCategory I: Projection Stage (Dimension Reduction) Projected with real-valued projection function o Given a point x,each projected dimension i will be associated with a real-valued projection function fi(x)(e.g.,fi(x)=wx) Quantization Stage Turn real into binary o Essential difference between metric learning and learning to hash Category Il: Binary-Code Learning Stage Hash Function Learning Stage 日卡三4元,互Q0 Li (http://cs.nju.edu.cn/lvj) Learning to Hash CS.NJU 10 /210
Introduction Two Stages of Hash Function Learning Two main categories: Category I: Projection Stage (Dimension Reduction) Projected with real-valued projection function Given a point x, each projected dimension i will be associated with a real-valued projection function fi(x) (e.g., fi(x) = wT i x) Quantization Stage Turn real into binary Essential difference between metric learning and learning to hash Category II: Binary-Code Learning Stage Hash Function Learning Stage Li (http://cs.nju.edu.cn/lwj) Learning to Hash CS, NJU 10 / 210
按次数下载不扣除下载券;
注册用户24小时内重复下载只扣除一次;
顺序:VIP每日次数-->可用次数-->下载券;
- 《大数据 Big Data》课程教学资源(参考文献)大数据机器学习 Big Data Machine Learning.pdf
- 《大数据 Big Data》课程教学资源(参考文献)Learning to Hash for Big Data.pdf
- 《大数据 Big Data》课程教学资源(参考文献)Learning to Hash for Big Data.pdf
- 《大数据 Big Data》课程教学资源(参考文献)大数据机器学习 Big Data Machine Learning.pdf
- 《大数据 Big Data》课程教学资源(参考文献)Learning to Hash for Big Data Retrieval and Mining(南京大学:李武军).pdf
- 《大数据 Big Data》课程教学资源(参考文献)Learning to Hash for Big Data Retrieval and Mining(南京大学:李武军).pdf
- 南京大学:《形式语言与自动机 Formal Languages and Automata》课程教学资源(PPT课件讲稿)Decidability, Complexity(P, NP, NPC and related).pptx
- 南京大学:《形式语言与自动机 Formal Languages and Automata》课程教学资源(PPT课件讲稿)Timed Automata.ppt
- 南京大学:《形式语言与自动机 Formal Languages and Automata》课程教学资源(PPT课件讲稿)Petri Net.pptx
- 南京大学:《形式语言与自动机 Formal Languages and Automata》课程教学资源(PPT课件讲稿)Transition System.pptx
- 南京大学:《形式语言与自动机 Formal Languages and Automata》课程教学资源(PPT课件讲稿)Turing Machine.pptx
- 南京大学:《形式语言与自动机 Formal Languages and Automata》课程教学资源(PPT课件讲稿)Properties of CFL(The Pumping Lemma for CFL’s).pptx
- 南京大学:《形式语言与自动机 Formal Languages and Automata》课程教学资源(PPT课件讲稿)Pushdown Automata.pptx
- 南京大学:《形式语言与自动机 Formal Languages and Automata》课程教学资源(PPT课件讲稿)Regular Expression.pptx
- 南京大学:《形式语言与自动机 Formal Languages and Automata》课程教学资源(PPT课件讲稿)Context Free Grammar.pptx
- 南京大学:《形式语言与自动机 Formal Languages and Automata》课程教学资源(PPT课件讲稿)Finite Automata.pptx
- 南京大学:《软件安全 Software Security》课程教学资源(PPT课件讲稿)Byzantine Generals Problem.ppt
- 南京大学:《软件安全 Software Security》课程教学资源(PPT课件讲稿)Use-after-free.pptx
- 南京大学:《软件安全 Software Security》课程教学资源(PPT课件讲稿)Taint Analysis.pptx
- 南京大学:《软件安全 Software Security》课程教学资源(PPT课件讲稿)Program Analysis - Data Flow Analysis.pptx
- 《大数据 Big Data》课程教学资源(参考文献)Parallel and Distributed Stochastic Learning - Towards Scalable Learning for Big Data Intelligence(南京大学:李武军).pdf
- 《人工智能、机器学习与大数据》课程教学资源(参考文献)Coherence functions for multicategory margin-based classification methods.pdf
- 《人工智能、机器学习与大数据》课程教学资源(参考文献)Latent Wishart processes for relational kernel learning.pdf
- 《人工智能、机器学习与大数据》课程教学资源(参考文献)Latent Wishart processes for relational kernel learning(讲稿).pdf
- 《人工智能、机器学习与大数据》课程教学资源(参考文献)agiCoFi - Tag informed collaborative filtering.pdf
- 《人工智能、机器学习与大数据》课程教学资源(参考文献)Localized content-based image retrieval through evidence region identification.pdf
- 《人工智能、机器学习与大数据》课程教学资源(参考文献)Relation regularized matrix factorization.pdf
- 《人工智能、机器学习与大数据》课程教学资源(参考文献)Relation regularized matrix factorization(讲稿).pdf
- 《人工智能、机器学习与大数据》课程教学资源(参考文献)Probabilistic relational PCA.pdf
- 《人工智能、机器学习与大数据》课程教学资源(参考文献)Gaussian process latent random field.pdf
- 《人工智能、机器学习与大数据》课程教学资源(参考文献)Multiple-instance learning via disambiguation.pdf
- 《人工智能、机器学习与大数据》课程教学资源(参考文献)Generalized latent factor models for social network analysis.pdf
- 《人工智能、机器学习与大数据》课程教学资源(参考文献)Social relations model for collaborative filtering.pdf
- 《人工智能、机器学习与大数据》课程教学资源(参考文献)Sparse probabilistic relational projection.pdf
- 《人工智能、机器学习与大数据》课程教学资源(参考文献)Emoticon smoothed language models for Twitter sentiment analysis.pdf
- 《人工智能、机器学习与大数据》课程教学资源(参考文献)Double-bit quantization for hashing.pdf
- 《人工智能、机器学习与大数据》课程教学资源(参考文献)Manhattan hashing for large-scale image retrieval.pdf
- 《人工智能、机器学习与大数据》课程教学资源(参考文献)Isotropic hashing.pdf
- 《人工智能、机器学习与大数据》课程教学资源(参考文献)Collaborative topic regression with social regularization for tag recommendation.pdf
- 《人工智能、机器学习与大数据》课程教学资源(参考文献)Online Egocentric models for citation networks.pdf