《人工智能、机器学习与大数据》课程教学资源(参考文献)Relation regularized matrix factorization(讲稿)
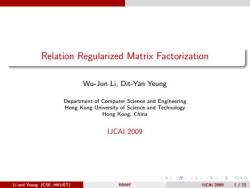
Relation Regularized Matrix Factorization Wu-Jun Li,Dit-Yan Yeung Department of Computer Science and Engineering Hong Kong University of Science and Technology Hong Kong,China IJCAI 2009 4口4日+立4至卡至及0 Li and Yeung (CSE.HKUST) RRMF UCA120091/23
Relation Regularized Matrix Factorization Wu-Jun Li, Dit-Yan Yeung Department of Computer Science and Engineering Hong Kong University of Science and Technology Hong Kong, China IJCAI 2009 Li and Yeung (CSE, HKUST) RRMF IJCAI 2009 1 / 23
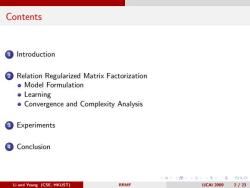
Contents Introduction Relation Regularized Matrix Factorization o Model Formulation ●Learning Convergence and Complexity Analysis Experiments Conclusion 4日4日+4立4至至只0 Li and Yeung (CSE.HKUST) RRMF UCA1200952/23
Contents 1 Introduction 2 Relation Regularized Matrix Factorization Model Formulation Learning Convergence and Complexity Analysis 3 Experiments 4 Conclusion Li and Yeung (CSE, HKUST) RRMF IJCAI 2009 2 / 23
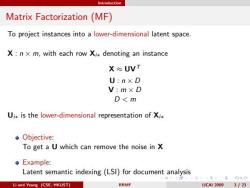
Introduction Matrix Factorization(MF) To project instances into a lower-dimensional latent space. X:n x m,with each row Xis denoting an instance X≈UVT U:n×D V:m×D D<m Ui*is the lower-dimensional representation of Xi 。Objective: To get a U which can remove the noise in X 。Example: Latent semantic indexing(LSI)for document analysis Li and Yeung (CSE.HKUST) RRMF UCA120093/23
Introduction Matrix Factorization (MF) To project instances into a lower-dimensional latent space. X : n × m, with each row Xi∗ denoting an instance X ≈ UVT U : n × D V : m × D D < m Ui∗ is the lower-dimensional representation of Xi∗ Objective: To get a U which can remove the noise in X Example: Latent semantic indexing (LSI) for document analysis Li and Yeung (CSE, HKUST) RRMF IJCAI 2009 3 / 23
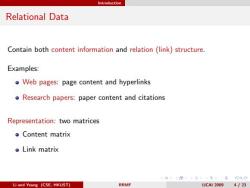
Introduction Relational Data Contain both content information and relation (link)structure. Examples: o Web pages:page content and hyperlinks o Research papers:paper content and citations Representation:two matrices o Content matrix o Link matrix 4口4日+1立4至卡三只0 Li and Yeung (CSE.HKUST) RRMF UCA120094/23
Introduction Relational Data Contain both content information and relation (link) structure. Examples: Web pages: page content and hyperlinks Research papers: paper content and citations Representation: two matrices Content matrix Link matrix Li and Yeung (CSE, HKUST) RRMF IJCAI 2009 4 / 23
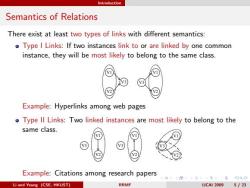
Introduction Semantics of Relations There exist at least two types of links with different semantics: o Type I Links:If two instances link to or are linked by one common instance,they will be most likely to belong to the same class. Example:Hyperlinks among web pages o Type ll Links:Two linked instances are most likely to belong to the same class. Example:Citations among research papers 4口4香+之卡要,三)Q0 Li and Yeung (CSE.HKUST) RRMF UCA120095/23
Introduction Semantics of Relations There exist at least two types of links with different semantics: Type I Links: If two instances link to or are linked by one common instance, they will be most likely to belong to the same class. V1 V2 V3 V1 V2 V3 Example: Hyperlinks among web pages Type II Links: Two linked instances are most likely to belong to the same class. V1 V2 V3 V1 V2 V3 V1 V2 V3 Example: Citations among research papers Li and Yeung (CSE, HKUST) RRMF IJCAI 2009 5 / 23
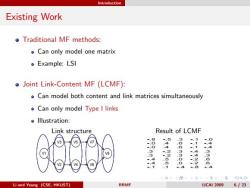
Introduction Existing Work o Traditional MF methods: Can only model one matrix ·Example:LSl Joint Link-Content MF (LCMF): Can model both content and link matrices simultaneously Can only model Type I links lllustration: Link structure Result of LCMF =.8 =.5 -3 =.1 =,0 .0 .4 -.1 -.4 =.0 .4 =.1 . .3 3 =.4 3 =.4 .0 -.1 =.4 -.8 .4 Li and Yeung (CSE.HKUST) RRMF UCA12009 6/23
Introduction Existing Work Traditional MF methods: Can only model one matrix Example: LSI Joint Link-Content MF (LCMF): Can model both content and link matrices simultaneously Can only model Type I links Illustration: Link structure V1 V8 V6 V7 V4 V5 V2 V3 Result of LCMF -.8 -.5 .3 -.1 -.0 -.0 .4 .6 -.1 -.4 -.0 .4 .6 -.1 -.4 .3 -.2 .3 -.4 .3 .3 -.2 .3 -.4 .3 -.4 .5 .0 -.2 .6 -.4 .5 .0 -.2 .6 -.1 .1 -.4 -.8 -.4 Li and Yeung (CSE, HKUST) RRMF IJCAI 2009 6 / 23
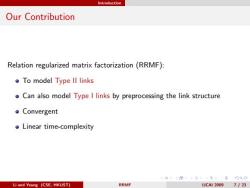
Introduction Our Contribution Relation regularized matrix factorization (RRMF): To model Type ll links Can also model Type I links by preprocessing the link structure ●Convergent o Linear time-complexity 日卡4日卡4三4至卡三80 Li and Yeung (CSE.HKUST) RRMF UCA120097/23
Introduction Our Contribution Relation regularized matrix factorization (RRMF): To model Type II links Can also model Type I links by preprocessing the link structure Convergent Linear time-complexity Li and Yeung (CSE, HKUST) RRMF IJCAI 2009 7 / 23
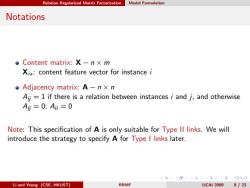
Relation Regularized Matrix Factorization Model Formulaton Notations Content matrix:X-n x m Xi:content feature vector for instance i o Adjacency matrix:A-n x n Aij =1 if there is a relation between instances i and j,and otherwise Aij=0;Aii=0 Note:This specification of A is only suitable for Type Il links.We will introduce the strategy to specify A for Type I links later. 4口4日+1艺4至卡三)Q0 Li and Yeung (CSE.HKUST) RRMF UCA120098/23
Relation Regularized Matrix Factorization Model Formulation Notations Content matrix: X − n × m Xi∗: content feature vector for instance i Adjacency matrix: A − n × n Aij = 1 if there is a relation between instances i and j, and otherwise Aij = 0; Aii = 0 Note: This specification of A is only suitable for Type II links. We will introduce the strategy to specify A for Type I links later. Li and Yeung (CSE, HKUST) RRMF IJCAI 2009 8 / 23
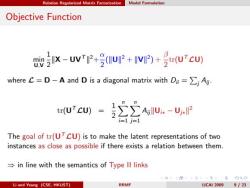
Relation Regularized Matrix Factodzation Mode Fomlton Objective Function IX-UVTIP+2(UIP+IVP)+(UTCU) where C=D-A and D is a diagonal matrix with Di=iAij. (UTLU)=2a∑∑Ayu.-U.P i=1i=1 The goal of tr(UCU)is to make the latent representations of two instances as close as possible if there exists a relation between them. in line with the semantics of Type ll links 口卡4日+4三4至卡三及0 Li and Yeung (CSE.HKUST) RRMF UCA120099/23
Relation Regularized Matrix Factorization Model Formulation Objective Function min U,V 1 2 kX − UVT k 2+ α 2 (kUk 2 + kVk 2 ) + β 2 tr(U TLU) where L = D − A and D is a diagonal matrix with Dii = P j Aij . tr(U TLU) = 1 2 Xn i=1 Xn j=1 AijkUi∗ − Uj∗k 2 The goal of tr(UTLU) is to make the latent representations of two instances as close as possible if there exists a relation between them. ⇒ in line with the semantics of Type II links Li and Yeung (CSE, HKUST) RRMF IJCAI 2009 9 / 23
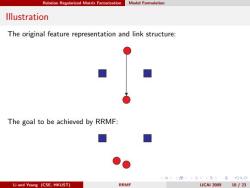
Relation Regularized Matrix Factorization Model Fommulaton lllustration The original feature representation and link structure: The goal to be achieved by RRMF: ■ 4口4日+1艺4至卡三)风0 Li and Yeung (CSE.HKUST) RRMF 1UCA1200910/23
Relation Regularized Matrix Factorization Model Formulation Illustration The original feature representation and link structure: The goal to be achieved by RRMF: Li and Yeung (CSE, HKUST) RRMF IJCAI 2009 10 / 23
按次数下载不扣除下载券;
注册用户24小时内重复下载只扣除一次;
顺序:VIP每日次数-->可用次数-->下载券;
- 《人工智能、机器学习与大数据》课程教学资源(参考文献)Relation regularized matrix factorization.pdf
- 《人工智能、机器学习与大数据》课程教学资源(参考文献)Localized content-based image retrieval through evidence region identification.pdf
- 《人工智能、机器学习与大数据》课程教学资源(参考文献)agiCoFi - Tag informed collaborative filtering.pdf
- 《人工智能、机器学习与大数据》课程教学资源(参考文献)Latent Wishart processes for relational kernel learning(讲稿).pdf
- 《人工智能、机器学习与大数据》课程教学资源(参考文献)Latent Wishart processes for relational kernel learning.pdf
- 《人工智能、机器学习与大数据》课程教学资源(参考文献)Coherence functions for multicategory margin-based classification methods.pdf
- 《大数据 Big Data》课程教学资源(参考文献)Parallel and Distributed Stochastic Learning - Towards Scalable Learning for Big Data Intelligence(南京大学:李武军).pdf
- 《大数据 Big Data》课程教学资源(参考文献)Learning to Hash for Big Data - A Tutorial.pdf
- 《大数据 Big Data》课程教学资源(参考文献)大数据机器学习 Big Data Machine Learning.pdf
- 《大数据 Big Data》课程教学资源(参考文献)Learning to Hash for Big Data.pdf
- 《大数据 Big Data》课程教学资源(参考文献)Learning to Hash for Big Data.pdf
- 《大数据 Big Data》课程教学资源(参考文献)大数据机器学习 Big Data Machine Learning.pdf
- 《大数据 Big Data》课程教学资源(参考文献)Learning to Hash for Big Data Retrieval and Mining(南京大学:李武军).pdf
- 《大数据 Big Data》课程教学资源(参考文献)Learning to Hash for Big Data Retrieval and Mining(南京大学:李武军).pdf
- 南京大学:《形式语言与自动机 Formal Languages and Automata》课程教学资源(PPT课件讲稿)Decidability, Complexity(P, NP, NPC and related).pptx
- 南京大学:《形式语言与自动机 Formal Languages and Automata》课程教学资源(PPT课件讲稿)Timed Automata.ppt
- 南京大学:《形式语言与自动机 Formal Languages and Automata》课程教学资源(PPT课件讲稿)Petri Net.pptx
- 南京大学:《形式语言与自动机 Formal Languages and Automata》课程教学资源(PPT课件讲稿)Transition System.pptx
- 南京大学:《形式语言与自动机 Formal Languages and Automata》课程教学资源(PPT课件讲稿)Turing Machine.pptx
- 南京大学:《形式语言与自动机 Formal Languages and Automata》课程教学资源(PPT课件讲稿)Properties of CFL(The Pumping Lemma for CFL’s).pptx
- 《人工智能、机器学习与大数据》课程教学资源(参考文献)Probabilistic relational PCA.pdf
- 《人工智能、机器学习与大数据》课程教学资源(参考文献)Gaussian process latent random field.pdf
- 《人工智能、机器学习与大数据》课程教学资源(参考文献)Multiple-instance learning via disambiguation.pdf
- 《人工智能、机器学习与大数据》课程教学资源(参考文献)Generalized latent factor models for social network analysis.pdf
- 《人工智能、机器学习与大数据》课程教学资源(参考文献)Social relations model for collaborative filtering.pdf
- 《人工智能、机器学习与大数据》课程教学资源(参考文献)Sparse probabilistic relational projection.pdf
- 《人工智能、机器学习与大数据》课程教学资源(参考文献)Emoticon smoothed language models for Twitter sentiment analysis.pdf
- 《人工智能、机器学习与大数据》课程教学资源(参考文献)Double-bit quantization for hashing.pdf
- 《人工智能、机器学习与大数据》课程教学资源(参考文献)Manhattan hashing for large-scale image retrieval.pdf
- 《人工智能、机器学习与大数据》课程教学资源(参考文献)Isotropic hashing.pdf
- 《人工智能、机器学习与大数据》课程教学资源(参考文献)Collaborative topic regression with social regularization for tag recommendation.pdf
- 《人工智能、机器学习与大数据》课程教学资源(参考文献)Online Egocentric models for citation networks.pdf
- 《人工智能、机器学习与大数据》课程教学资源(参考文献)Large-Scale Supervised Multimodal Hashing with Semantic Correlation Maximization.pdf
- 《人工智能、机器学习与大数据》课程教学资源(参考文献)Supervised Hashing with Latent Factor Models.pdf
- 《人工智能、机器学习与大数据》课程教学资源(参考文献)Coupled Group Lasso for Web-Scale CTR Prediction in Display Advertising.pdf
- 《人工智能、机器学习与大数据》课程教学资源(参考文献)Distributed Stochastic ADMM for Matrix Factorization.pdf
- 《人工智能、机器学习与大数据》课程教学资源(参考文献)Distributed Power-law Graph Computing - Theoretical and Empirical Analysis.pdf
- 《人工智能、机器学习与大数据》课程教学资源(参考文献)Multicategory large margin classification methods - hinge losses vs. coherence functions..pdf
- 《人工智能、机器学习与大数据》课程教学资源(参考文献)Relational collaborative topic regression for recommender systems.pdf
- 《人工智能、机器学习与大数据》课程教学资源(参考文献)Support Matrix Machines.pdf