电子科技大学:《贝叶斯学习与随机矩阵及在无线通信中的应用 BI-RM-AWC》课程教学资源(学习资料)随机矩阵补充材料 Analysis of neural networks - a random matrix approach

7.Analysis of neural networks: a random matrix approach 1
1 7. Analysis of neural networks: a random matrix approach

7 Analysis of neural networks: a random matrix approach The inherent intractability of neural network performances,which mainly originates from the non linearity of the neural activations (as well as from learning by back-propagation of the error). With this observation in mind,we propose here a theoretical study of the performance of large dimensional neural networks (in the sense of large datasets and number of neurons)
The inherent intractability of neural network performances, which mainly originates from the non linearity of the neural activations (as well as from learning by back-propagation of the error). 7 Analysis of neural networks: a random matrix approach With this observation in mind, we propose here a theoretical study of the performance of large dimensional neural networks (in the sense of large datasets and number of neurons)
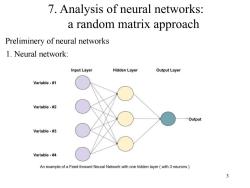
7.Analysis of neural networks: a random matrix approach Preliminery of neural networks 1.Neural network: Input Layer Hidden Layer Output Layer Variable-#1 Variable-#2 Output Variable-#3 Variable-#4 An example of a Feed-forward Neural Network with one hidden layer(with 3 neurons 3
3 7. Analysis of neural networks: a random matrix approach Preliminery of neural networks 1. Neural network:
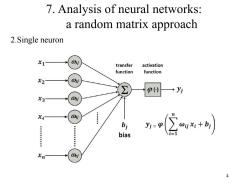
7.Analysis of neural networks: a random matrix approach 2.Single neuron x1 j transfer activation function function X2 02j P() X3 03j XA 04j bi bias y公+ Xn Onj 4
4 7. Analysis of neural networks: a random matrix approach 2.Single neuron
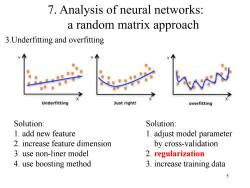
7.Analysis of neural networks: a random matrix approach 3.Underfitting and overfitting Underfitting Just right! overfitting Solution: Solution: 1.add new feature 1.adjust model parameter 2.increase feature dimension by cross-validation 3.use non-liner model 2.regularization 4.use boosting method 3.increase training data 5
5 7. Analysis of neural networks: a random matrix approach 3.Underfitting and overfitting Solution: 1. add new feature 2. increase feature dimension 3. use non-liner model 4. use boosting method Solution: 1. adjust model parameter by cross-validation 2. regularization 3. increase training data

7.Analysis of neural networks: a random matrix approach 4.Regularization Regularization is a main method to avoid overfitting,by introducing regularization term into loss model: w*=argmin L(y,f(w,x))+XR(w) 个 regularization term where A is the regularization parameter,and (w)=w N=15 6 0
6 7. Analysis of neural networks: a random matrix approach 4.Regularization Regularization is a main method to avoid overfitting, by introducing regularization term into loss model: ↑ regularization term where is the regularization parameter, and

7 Analysis of neural networks: a random matrix approach System Model The network is fed by a set of T input data vectors x [...rE RPxT and is trained to map corresponding vectors Y =,...RxT hidden layer of n neurons with non-linear activation function.The training phase is particular in that only the hidden layer-to-sink connectivity matrix Be RTx is learnt while the input-to-hidden layer connectivity matrix W E RpxT is static but randomly selected n neurons Y=[1,,r] X=[x1,,xT] 7 o(Wxt) Y≈Y?
7 7 Analysis of neural networks: a random matrix approach System Model The network is fed by a set of T input data vectors and is trained to map corresponding vectors hidden layer of n neurons with non-linear activation function . The training phase is particular in that only the hidden layer-to-sink connectivity matrix is learnt while the input-to-hidden layer connectivity matrix is static but randomly selected
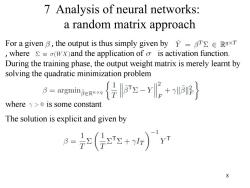
7 Analysis of neural networks: a random matrix approach For a given B,the output is thus simply given by y =BT>E RaxT where >=(Wx)and the application of o is activation function During the training phase,the output weight matrix is merely learnt by solving the quadratic minimization problem B=argmingeRnxa 行y-g+呢} where y>o is some constant The solution is explicit and given by g=(宁四+)'r 8
8 For a given , the output is thus simply given by , where , and , and the application of is activation function. During the training phase, the output weight matrix is merely learnt by solving the quadratic minimization problem where is some constant The solution is explicit and given by 7 Analysis of neural networks: a random matrix approach
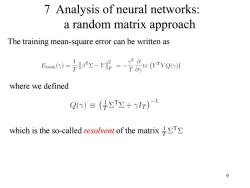
7 Analysis of neural networks: a random matrix approach The training mean-square error can be written as Fuo()( where we defined Q()=(号T+ylr)- which is the so-called resolvent of the matrix> 9
9 7 Analysis of neural networks: a random matrix approach The training mean-square error can be written as where we defined which is the so-called resolvent of the matrix

7 Analysis of neural networks: a random matrix approach We define A=YTY,recall that our objective is to retrieve a matrix Q() such that.Ttr A(Q()-Q())0.Let us write Q()=(F+yIr)-for some deterministic FeRTxT to be identified.Then,we have tA(Q()-Q()》 -7A0)(F-7)o) -7rAQ)ro)-7∑7.Q)A912 where the first equality uses A-1-B-1=A-(B-A)B-1.while the second equality uses T∑=∑=1以卫, with >i..being the row of 10
10 7 Analysis of neural networks: a random matrix approach We define recall that our objective is to retrieve a matrix such that, . Let us write some deterministic for to be identified. Then, we have where the first equality uses equality uses while the second with being the row of
按次数下载不扣除下载券;
注册用户24小时内重复下载只扣除一次;
顺序:VIP每日次数-->可用次数-->下载券;
- 电子科技大学:《贝叶斯学习与随机矩阵及在无线通信中的应用 BI-RM-AWC》课程教学资源(学习资料)贝叶斯学习补充材料.pdf
- 电子科技大学:《贝叶斯学习与随机矩阵及在无线通信中的应用 BI-RM-AWC》课程教学资源(课件讲稿)09 Sparse Signal Recovery.pdf
- 电子科技大学:《贝叶斯学习与随机矩阵及在无线通信中的应用 BI-RM-AWC》课程教学资源(课件讲稿)08 Linear Regression.pdf
- 《贝叶斯学习与随机矩阵及在无线通信中的应用 BI-RM-AWC》课程教学资源(学习资料)Random Matrix Theory and Wireless Communications.pdf
- 电子科技大学:《贝叶斯学习与随机矩阵及在无线通信中的应用 BI-RM-AWC》课程教学资源(课件讲稿)05 Free Probability.pdf
- 电子科技大学:《贝叶斯学习与随机矩阵及在无线通信中的应用 BI-RM-AWC》课程教学资源(课件讲稿)07 Analysis of neural networks - a random matrix approach.pdf
- 电子科技大学:《贝叶斯学习与随机矩阵及在无线通信中的应用 BI-RM-AWC》课程教学资源(课件讲稿)06 Non-asymptotic Analysis for Large Random Matrix.pdf
- 电子科技大学:《贝叶斯学习与随机矩阵及在无线通信中的应用 BI-RM-AWC》课程教学资源(课件讲稿)04 Asymptotic Spectrum Theorems(4/4).pdf
- 电子科技大学:《贝叶斯学习与随机矩阵及在无线通信中的应用 BI-RM-AWC》课程教学资源(课件讲稿)04 Asymptotic Spectrum Theorems(3/4).pdf
- 电子科技大学:《贝叶斯学习与随机矩阵及在无线通信中的应用 BI-RM-AWC》课程教学资源(课件讲稿)04 Asymptotic Spectrum Theorems(2/4).pdf
- 《贝叶斯学习与随机矩阵及在无线通信中的应用 BI-RM-AWC》课程教学资源(文献书籍)An Introduction to Random Matrices(Greg W. Anderson、Alice Guionnet).pdf
- 电子科技大学:《贝叶斯学习与随机矩阵及在无线通信中的应用 BI-RM-AWC》课程教学资源(课件讲稿)04 Asymptotic Spectrum Theorems(1/4).pdf
- 电子科技大学:《贝叶斯学习与随机矩阵及在无线通信中的应用 BI-RM-AWC》课程教学资源(课件讲稿)03 Transforms.pdf
- 电子科技大学:《贝叶斯学习与随机矩阵及在无线通信中的应用 BI-RM-AWC》课程教学资源(课件讲稿)02 Types of Matrices and Local Non-Asymptotic Results.pdf
- 电子科技大学:《贝叶斯学习与随机矩阵及在无线通信中的应用 BI-RM-AWC》课程教学资源(课件讲稿)01 Introduction of Wireless Channel and Random Matrices(陈智).pdf
- 《贝叶斯学习与随机矩阵及在无线通信中的应用 BI-RM-AWC》课程教学资源(文献书籍)Random Matrix Theory and Wireless Communications.pdf
- 《贝叶斯学习与随机矩阵及在无线通信中的应用 BI-RM-AWC》课程教学资源(文献书籍)PRML中文版——模式识别与机器学习.pdf
- 《贝叶斯学习与随机矩阵及在无线通信中的应用 BI-RM-AWC》课程教学资源(文献书籍)Pattern Recognition and Machine Learning.pdf
- 电子科技大学:《时域测试技术综合实验 Comprehensive Experiment of Time Domain Testing Technology》课程教学资源(课件讲稿)实验十四 虚拟数字示波器实验.pdf
- 电子科技大学:《时域测试技术综合实验 Comprehensive Experiment of Time Domain Testing Technology》课程教学资源(课件讲稿)实验十三 基于FPGA的地址译码实验.pdf
- 广东海洋大学:《数字信号处理 Digital Signal Processing》课程教学资源(电子教案).doc
- 电子科技大学:《ASIC设计 Application Specific Integrated Circuit Design(ASIC)》课程教学资源(课件讲稿)Topic 1 Introduction(About IC technology).pdf
- 电子科技大学:《ASIC设计 Application Specific Integrated Circuit Design(ASIC)》课程教学资源(课件讲稿)Topic 1 Introduction(About ASIC Design).pdf
- 电子科技大学:《ASIC设计 Application Specific Integrated Circuit Design(ASIC)》课程教学资源(课件讲稿)Topic1 Introduction(About Our Course).pdf
- 电子科技大学:《ASIC设计 Application Specific Integrated Circuit Design(ASIC)》课程教学资源(课件讲稿)Topic 2 FPGA Design with Verilog(FPGA Design Method、Design Examples).pdf
- 电子科技大学:《ASIC设计 Application Specific Integrated Circuit Design(ASIC)》课程教学资源(课件讲稿)Topic 2 FPGA Design with Verilog(Supplementary).pdf
- 电子科技大学:《ASIC设计 Application Specific Integrated Circuit Design(ASIC)》课程教学资源(课件讲稿)Topic 3 Verification and Test.pdf
- 电子科技大学:《ASIC设计 Application Specific Integrated Circuit Design(ASIC)》课程教学资源(课件讲稿)Topic 4 VLSI for DSP.pdf
- 电子科技大学:《高等数字集成电路设计 Advanced Digital Integrated Circuits Design》课程教学资源(教学大纲,负责人:贺雅娟).pdf
- 电子科技大学:《高等数字集成电路设计 Advanced Digital Integrated Circuits Design》课程教学资源(课件讲稿)Lecture 1 Introduction & The Fabrics.pdf
- 电子科技大学:《模拟集成电路分析与设计 Analysis and Design of Analog Integrated Circuit》课程教学资源(教学大纲,负责人:罗萍).pdf
- 电子科技大学:《模拟集成电路分析与设计 Analysis and Design of Analog Integrated Circuit》课程教学资源(课件讲稿)Chapter 01 Introduction、Models and comparison of integrated-circuit active devices.pdf
- 电子科技大学:《模拟集成电路分析与设计 Analysis and Design of Analog Integrated Circuit》课程教学资源(课件讲稿)Chapter 02 Amplifiers, source followers and cascodes.pdf
- 川北医学院:《模拟电子技术》课程电子教案(课件讲稿)第四章 集成运算放大器 integrated operational amplifier.pdf
- 川北医学院:《模拟电子技术》课程电子教案(课件讲稿)第四章 双极结型三极管及放大电路基础.pdf
- 《信号与系统》课程教学资源(课件讲稿)第2章 线性时不变系统的时域分析.pdf
- 川北医学院:《模拟电子技术》课程电子教案(课件讲稿)第五章 场效应管放大电路.pdf
- 川北医学院:《模拟电子技术》课程电子教案(课件讲稿)第三章 二极管及其基本电路.pdf
- 《电子技术基础》课程教学资源(课件讲稿)第五章 振荡电路 oscillator.pdf
- 《单片机原理及应用》课程教学资源(课件讲稿)第7章 并行扩展技术.pdf