电子科技大学:《贝叶斯学习与随机矩阵及在无线通信中的应用 BI-RM-AWC》课程教学资源(课件讲稿)05 Free Probability
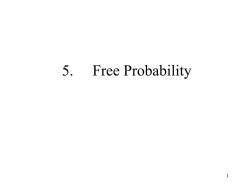
5. Free Probability 1
1 5. Free Probability
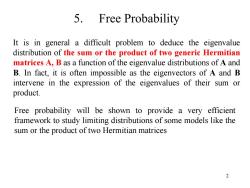
5. Free Probability It is in general a difficult problem to deduce the eigenvalue distribution of the sum or the product of two generic Hermitian matrices A,B as a function of the eigenvalue distributions of A and B.In fact,it is often impossible as the eigenvectors of A and B intervene in the expression of the eigenvalues of their sum or product. Free probability will be shown to provide a very efficient framework to study limiting distributions of some models like the sum or the product of two Hermitian matrices 2
2 5. Free Probability It is in general a difficult problem to deduce the eigenvalue distribution of the sum or the product of two generic Hermitian matrices A, B as a function of the eigenvalue distributions of A and B. In fact, it is often impossible as the eigenvectors of A and B intervene in the expression of the eigenvalues of their sum or product. Free probability will be shown to provide a very efficient framework to study limiting distributions of some models like the sum or the product of two Hermitian matrices
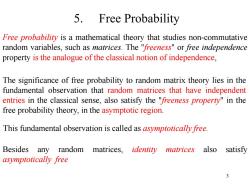
5. Free Probability Free probability is a mathematical theory that studies non-commutative random variables,such as matrices.The "freeness"or free independence property is the analogue of the classical notion of independence, The significance of free probability to random matrix theory lies in the fundamental observation that random matrices that have independent entries in the classical sense,also satisfy the "freeness property"in the free probability theory,in the asymptotic region. This fundamental observation is called as asymptotically free. Besides any random matrices, identity matrices also s satisfy asymptotically free 3
3 5. Free Probability Free probability is a mathematical theory that studies non-commutative random variables, such as matrices. The "freeness" or free independence property is the analogue of the classical notion of independence, The significance of free probability to random matrix theory lies in the fundamental observation that random matrices that have independent entries in the classical sense, also satisfy the "freeness property" in the free probability theory, in the asymptotic region. This fundamental observation is called as asymptotically free. Besides any random matrices, identity matrices also satisfy asymptotically free

5. Free Probability The definition of free probability is analogous to the concept of independence in classical probability.The definition of freeness is as follows: a)The free probability developed as a probability theory can be applied for noncommutative random variables like matrices; b)These random variables are the elements in a non-commutative probability space that can be defined by a pair (A,φ) where A is a unital algebra,and o is a normalized linear functional on A.In random matrix backgound,A is a IXI matrix and o is the normalized monent for the matrix,which is defined as 4
4 5. Free Probability The definition of free probability is analogous to the concept of independence in classical probability. The definition of freeness is as follows: a)The free probability developed as a probability theory can be applied for noncommutative random variables like matrices; b)These random variables are the elements in a non-commutative probability space that can be defined by a pair ( , ) A where A is a unital algebra, and φ is a normalized linear functional on A. In random matrix backgound, A is a l×l matrix and φ is the normalized monent for the matrix, which is defined as 1 ( ) ( ) tr l A A
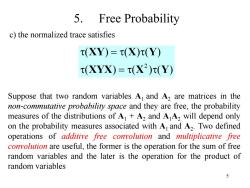
5. Free Probability c)the normalized trace satisfies (XY)=(X)(Y) (XYX)=(X(Y) Suppose that two random variables A and A,are matrices in the non-commutative probability space and they are free,the probability measures of the distributions of A+A2 and A A2 will depend only on the probability measures associated with A and A2.Two defined operations of additive free convolution and multiplicative free convolution are useful,the former is the operation for the sum of free random variables and the later is the operation for the product of random variables 5
5 5. Free Probability c) the normalized trace satisfies 2 ( ) ( ) ( ) ( ) ( ) ( ) XY X Y XYX X Y Suppose that two random variables A1 and A2 are matrices in the non-commutative probability space and they are free, the probability measures of the distributions of A1 + A2 and A1A2 will depend only on the probability measures associated with A1 and A2 . Two defined operations of additive free convolution and multiplicative free convolution are useful, the former is the operation for the sum of free random variables and the later is the operation for the product of random variables
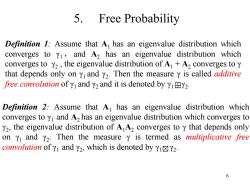
5. Free Probability Definition 1:Assume that A has an eigenvalue distribution which converges to Y,and A2 has an eigenvalue distribution which converges to Y2,the eigenvalue distribution of A+A2 converges to y that depends only on Y and Y2.Then the measure y is called additive free convolution of y and y2 and it is denoted by YY2. Definition 2:Assume that A has an eigenvalue distribution which converges to Y and A2 has an eigenvalue distribution which converges to Y2,the eigenvalue distribution of A A2 converges to y that depends only on Y and Y2.Then the measure y is termed as multiplicative free convolution of y and Y2,which is denoted by YY2. 6
6 5. Free Probability Definition 1: Assume that A1 has an eigenvalue distribution which converges to γ1,and A2 has an eigenvalue distribution which converges to γ2 , the eigenvalue distribution of A1 + A2 converges to γ that depends only on γ1 and γ2 . Then the measure γ is called additive free convolution of γ1 and γ2 and it is denoted by γ1 γ2 . Definition 2: Assume that A1 has an eigenvalue distribution which converges to γ1 and A2 has an eigenvalue distribution which converges to γ2 , the eigenvalue distribution of A1A2 converges to γ that depends only on γ1 and γ2 . Then the measure γ is termed as multiplicative free convolution of γ1 and γ2 , which is denoted by γ1 γ2
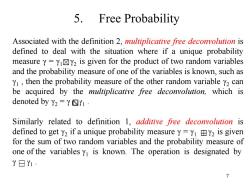
5. Free Probability Associated with the definition 2,multiplicative free deconvolution is defined to deal with the situation where if a unique probability measure y=YY2 is given for the product of two random variables and the probability measure of one of the variables is known,such as Y,then the probability measure of the other random variable y2 can be acquired by the multiplicative free deconvolution,which is denoted by Y2=YㄖYi· Similarly related to definition 1,additive free deconvolution is defined to get Y2 if a unique probability measure y =Y1 Y2 is given for the sum of two random variables and the probability measure of one of the variables y is known.The operation is designated by Y日Y1· 7
7 5. Free Probability Associated with the definition 2, multiplicative free deconvolution is defined to deal with the situation where if a unique probability measure γ = γ1 γ2 is given for the product of two random variables and the probability measure of one of the variables is known, such as γ1 , then the probability measure of the other random variable γ2 can be acquired by the multiplicative free deconvolution, which is denoted by γ2 = γ γ1 . Similarly related to definition 1, additive free deconvolution is defined to get γ2 if a unique probability measure γ = γ1 γ2 is given for the sum of two random variables and the probability measure of one of the variables γ1 is known. The operation is designated by γ γ1
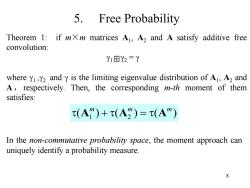
5.Free Probability Theorem 1:if mXm matrices A1,A2 and A satisfy additive free convolution: Y1田Y2=Y where Y1,Y2 and y is the limiting eigenvalue distribution of A,A2 and A,respectively.Then,the corresponding m-th moment of them satisfies: (A")+(A)=(A") In the non-commutative probability space,the moment approach can uniquely identify a probability measure. 8
8 5. Free Probability Theorem 1: if m×m matrices A1 , A2 and A satisfy additive free convolution: γ1 γ2 = γ where γ1 ,γ2 and γ is the limiting eigenvalue distribution of A1 , A2 and A,respectively. Then, the corresponding m-th moment of them satisfies: 1 2 ( ) ( ) ( ) m m m A A A In the non-commutative probability space, the moment approach can uniquely identify a probability measure
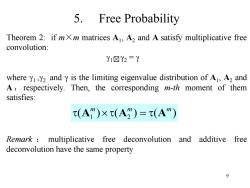
5.Free Probability Theorem 2:if mXm matrices A,A2 and A satisfy multiplicative free convolution: Y1☒Y2=Y where Y1,Y2 and y is the limiting eigenvalue distribution of A1,A2 and A,respectively.Then,the corresponding m-th moment of them satisfies: (A")×(A)=(A") Remark multiplicative free deconvolution and additive free deconvolution have the same property 9
9 5. Free Probability Theorem 2: if m×m matrices A1 , A2 and A satisfy multiplicative free convolution: γ1 γ2 = γ where γ1 ,γ2 and γ is the limiting eigenvalue distribution of A1 , A2 and A,respectively. Then, the corresponding m-th moment of them satisfies: 1 2 ( ) ( ) ( ) m m m A A A Remark : multiplicative free deconvolution and additive free deconvolution have the same property
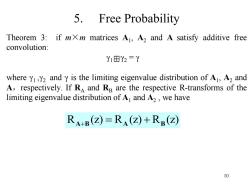
5.Free Probability Theorem 3:if mXm matrices A,A2 and A satisfy additive free convolution: Y1田Y2=Y where Y1,Y2 and y is the limiting eigenvalue distribution of A,A2 and A,respectively.If Ra and Re are the respective R-transforms of the limiting eigenvalue distribution of A and A2,we have RAB=RA+REZ) 10
10 5. Free Probability Theorem 3: if m×m matrices A1 , A2 and A satisfy additive free convolution: γ1 γ2 = γ where γ1 ,γ2 and γ is the limiting eigenvalue distribution of A1 , A2 and A,respectively. If RA and RB are the respective R-transforms of the limiting eigenvalue distribution of A1 and A2 , we have R (z) R (z) R (z) A B A B
按次数下载不扣除下载券;
注册用户24小时内重复下载只扣除一次;
顺序:VIP每日次数-->可用次数-->下载券;
- 电子科技大学:《贝叶斯学习与随机矩阵及在无线通信中的应用 BI-RM-AWC》课程教学资源(课件讲稿)07 Analysis of neural networks - a random matrix approach.pdf
- 电子科技大学:《贝叶斯学习与随机矩阵及在无线通信中的应用 BI-RM-AWC》课程教学资源(课件讲稿)06 Non-asymptotic Analysis for Large Random Matrix.pdf
- 电子科技大学:《贝叶斯学习与随机矩阵及在无线通信中的应用 BI-RM-AWC》课程教学资源(课件讲稿)04 Asymptotic Spectrum Theorems(4/4).pdf
- 电子科技大学:《贝叶斯学习与随机矩阵及在无线通信中的应用 BI-RM-AWC》课程教学资源(课件讲稿)04 Asymptotic Spectrum Theorems(3/4).pdf
- 电子科技大学:《贝叶斯学习与随机矩阵及在无线通信中的应用 BI-RM-AWC》课程教学资源(课件讲稿)04 Asymptotic Spectrum Theorems(2/4).pdf
- 《贝叶斯学习与随机矩阵及在无线通信中的应用 BI-RM-AWC》课程教学资源(文献书籍)An Introduction to Random Matrices(Greg W. Anderson、Alice Guionnet).pdf
- 电子科技大学:《贝叶斯学习与随机矩阵及在无线通信中的应用 BI-RM-AWC》课程教学资源(课件讲稿)04 Asymptotic Spectrum Theorems(1/4).pdf
- 电子科技大学:《贝叶斯学习与随机矩阵及在无线通信中的应用 BI-RM-AWC》课程教学资源(课件讲稿)03 Transforms.pdf
- 电子科技大学:《贝叶斯学习与随机矩阵及在无线通信中的应用 BI-RM-AWC》课程教学资源(课件讲稿)02 Types of Matrices and Local Non-Asymptotic Results.pdf
- 电子科技大学:《贝叶斯学习与随机矩阵及在无线通信中的应用 BI-RM-AWC》课程教学资源(课件讲稿)01 Introduction of Wireless Channel and Random Matrices(陈智).pdf
- 《贝叶斯学习与随机矩阵及在无线通信中的应用 BI-RM-AWC》课程教学资源(文献书籍)Random Matrix Theory and Wireless Communications.pdf
- 《贝叶斯学习与随机矩阵及在无线通信中的应用 BI-RM-AWC》课程教学资源(文献书籍)PRML中文版——模式识别与机器学习.pdf
- 《贝叶斯学习与随机矩阵及在无线通信中的应用 BI-RM-AWC》课程教学资源(文献书籍)Pattern Recognition and Machine Learning.pdf
- 电子科技大学:《时域测试技术综合实验 Comprehensive Experiment of Time Domain Testing Technology》课程教学资源(课件讲稿)实验十四 虚拟数字示波器实验.pdf
- 电子科技大学:《时域测试技术综合实验 Comprehensive Experiment of Time Domain Testing Technology》课程教学资源(课件讲稿)实验十三 基于FPGA的地址译码实验.pdf
- 电子科技大学:《时域测试技术综合实验 Comprehensive Experiment of Time Domain Testing Technology》课程教学资源(课件讲稿)实验十二 数字示波器信号调理通道实验.pdf
- 电子科技大学:《时域测试技术综合实验 Comprehensive Experiment of Time Domain Testing Technology》课程教学资源(课件讲稿)实验十一 数字示波器协议触发与解码应用测试.pdf
- 电子科技大学:《时域测试技术综合实验 Comprehensive Experiment of Time Domain Testing Technology》课程教学资源(课件讲稿)实验十 时域反射实验.pdf
- 电子科技大学:《时域测试技术综合实验 Comprehensive Experiment of Time Domain Testing Technology》课程教学资源(课件讲稿)实验九 参数测量实验.pdf
- 电子科技大学:《时域测试技术综合实验 Comprehensive Experiment of Time Domain Testing Technology》课程教学资源(课件讲稿)实验八 数字示波器中的信号插值.pdf
- 《贝叶斯学习与随机矩阵及在无线通信中的应用 BI-RM-AWC》课程教学资源(学习资料)Random Matrix Theory and Wireless Communications.pdf
- 电子科技大学:《贝叶斯学习与随机矩阵及在无线通信中的应用 BI-RM-AWC》课程教学资源(课件讲稿)08 Linear Regression.pdf
- 电子科技大学:《贝叶斯学习与随机矩阵及在无线通信中的应用 BI-RM-AWC》课程教学资源(课件讲稿)09 Sparse Signal Recovery.pdf
- 电子科技大学:《贝叶斯学习与随机矩阵及在无线通信中的应用 BI-RM-AWC》课程教学资源(学习资料)贝叶斯学习补充材料.pdf
- 电子科技大学:《贝叶斯学习与随机矩阵及在无线通信中的应用 BI-RM-AWC》课程教学资源(学习资料)随机矩阵补充材料 Analysis of neural networks - a random matrix approach.pdf
- 广东海洋大学:《数字信号处理 Digital Signal Processing》课程教学资源(电子教案).doc
- 电子科技大学:《ASIC设计 Application Specific Integrated Circuit Design(ASIC)》课程教学资源(课件讲稿)Topic 1 Introduction(About IC technology).pdf
- 电子科技大学:《ASIC设计 Application Specific Integrated Circuit Design(ASIC)》课程教学资源(课件讲稿)Topic 1 Introduction(About ASIC Design).pdf
- 电子科技大学:《ASIC设计 Application Specific Integrated Circuit Design(ASIC)》课程教学资源(课件讲稿)Topic1 Introduction(About Our Course).pdf
- 电子科技大学:《ASIC设计 Application Specific Integrated Circuit Design(ASIC)》课程教学资源(课件讲稿)Topic 2 FPGA Design with Verilog(FPGA Design Method、Design Examples).pdf
- 电子科技大学:《ASIC设计 Application Specific Integrated Circuit Design(ASIC)》课程教学资源(课件讲稿)Topic 2 FPGA Design with Verilog(Supplementary).pdf
- 电子科技大学:《ASIC设计 Application Specific Integrated Circuit Design(ASIC)》课程教学资源(课件讲稿)Topic 3 Verification and Test.pdf
- 电子科技大学:《ASIC设计 Application Specific Integrated Circuit Design(ASIC)》课程教学资源(课件讲稿)Topic 4 VLSI for DSP.pdf
- 电子科技大学:《高等数字集成电路设计 Advanced Digital Integrated Circuits Design》课程教学资源(教学大纲,负责人:贺雅娟).pdf
- 电子科技大学:《高等数字集成电路设计 Advanced Digital Integrated Circuits Design》课程教学资源(课件讲稿)Lecture 1 Introduction & The Fabrics.pdf
- 电子科技大学:《模拟集成电路分析与设计 Analysis and Design of Analog Integrated Circuit》课程教学资源(教学大纲,负责人:罗萍).pdf
- 电子科技大学:《模拟集成电路分析与设计 Analysis and Design of Analog Integrated Circuit》课程教学资源(课件讲稿)Chapter 01 Introduction、Models and comparison of integrated-circuit active devices.pdf
- 电子科技大学:《模拟集成电路分析与设计 Analysis and Design of Analog Integrated Circuit》课程教学资源(课件讲稿)Chapter 02 Amplifiers, source followers and cascodes.pdf
- 川北医学院:《模拟电子技术》课程电子教案(课件讲稿)第四章 集成运算放大器 integrated operational amplifier.pdf
- 川北医学院:《模拟电子技术》课程电子教案(课件讲稿)第四章 双极结型三极管及放大电路基础.pdf