电子科技大学:《贝叶斯学习与随机矩阵及在无线通信中的应用 BI-RM-AWC》课程教学资源(课件讲稿)02 Types of Matrices and Local Non-Asymptotic Results
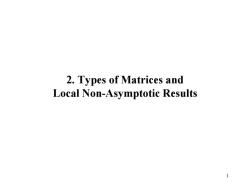
2.Types of Matrices and Local Non-Asymptotic Results 1
2. Types of Matrices and Local Non-Asymptotic Results 1
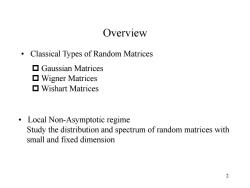
Overview Classical Types of Random Matrices ▣Gaussian Matrices ▣Wigner Matrices ▣Wishart Matrices Local Non-Asymptotic regime Study the distribution and spectrum of random matrices with small and fixed dimension 2
2 Overview • Classical Types of Random Matrices • Local Non-Asymptotic regime Study the distribution and spectrum of random matrices with small and fixed dimension Gaussian Matrices Wigner Matrices Wishart Matrices
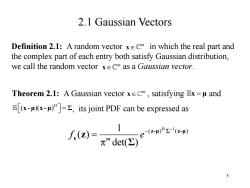
2.1 Gaussian Vectors Definition 2.1:A random vector xeCT in which the real part and the complex part of each entry both satisfy Gaussian distribution, we call the random vector xe CT as a Gaussian vector. Theorem 2.1:A Gaussian vector xeCT,satisfying Ex=u and E(x-)(x-)"=,its joint PDF can be expressed as 人ai e(z-)HΣ'(z-) 3
3 2.1 Gaussian Vectors Definition 2.1: A random vector in which the real part and the complex part of each entry both satisfy Gaussian distribution, we call the random vector as a Gaussian vector. m x m x Theorem 2.1: A Gaussian vector , satisfying and , its joint PDF can be expressed as m x x μ H ( )( ) x -μ x -μ Σ H 1 1 ( ) ( ) ( ) det( ) m f e z -μ Σ z -μ x z Σ
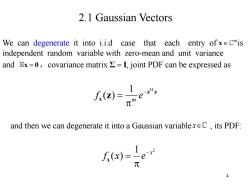
2.1 Gaussian Vectors We can degenerate it into i.i.d case that each entry of x∈C"is independent random variable with zero-mean and unit variance and Ex=0,covariance matrix >=I,joint PDF can be expressed as e and then we can degenerate it into a Gaussian variablexEC,its PDF: L(x)=Ie 元 4
4 2.1 Gaussian Vectors We can degenerate it into i.i.d case that each entry of is independent random variable with zero-mean and unit variance and x 0 ,covariance matrix , joint PDF can be expressed as 1 H ( ) m f e z z x z Σ I and then we can degenerate it into a Gaussian variable , its PDF: x 1 2 ( ) x f x e x m x
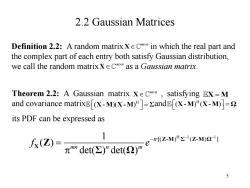
2.2 Gaussian Matrices Definition 2.2:A random matrix XeCIRX in which the real part and the complex part of each entry both satisfy Gaussian distribution, we call the random matrixXe Cx"as a Gaussian matrix. Theorem 2.2:A Gaussian matrix X eCTX,satisfying EX=M and covariance matrix (X-M)(X-M)"]=zandE(X-M)"(X-M)]= its PDF can be expressed as 1 ATet四Ydet2eo'z.Wp 5
5 2.2 Gaussian Matrices Definition 2.2: A random matrix in which the real part and the complex part of each entry both satisfy Gaussian distribution, we call the random matrix as a Gaussian matrix. m n X m n X Theorem 2.2: A Gaussian matrix , satisfying and covariance matrix and , its PDF can be expressed as X M m n X H ( )( ) X - M X - M Σ H ( ) ( ) X - M X - M Ω H 1 1 1 [( ) ( ) ] ( ) det( ) det( ) tr mn n m f e Z-M Σ Z-M Ω X Z Σ Ω

2.2 Gaussian Matrices We can degenerate it into i.i.d case that each entry of xecTR is independent random variable with zero-mean and unit variance whose covariance matrix Xx"]=I and X"X]=I its joint PDF can be expressed as 人-点e and then we can degenerate it into a Gaussian vector xeC,its PDF: 人-e 6
6 2.2 Gaussian Matrices We can degenerate it into i.i.d case that each entry of is independent random variable with zero-mean and unit variance whose covariance matrix and , its joint PDF can be expressed as H XX I H X X I H 1 ( ) ( ) tr mn f e Z Z X Z m n X and then we can degenerate it into a Gaussian vector , its PDF: m x 1 H ( ) m f e z z x z

2.3 Wigner Matrices Definition 2.3:An nXn Hermitian matrix W is a Wigner matrix if its upper-triangular entries are independent zero-mean random variables with identical variance.If the variance is 1/n,then w is a standard Wigner matrix. Theorem 2.3:Let W be an n x n complex Wigner matrix whose (diagonal and upper-triangle)entries are i.i.d.zero-mean Gaussian with unit variance.Then,its PDF is rIw2] 2m2元n2e 2 while the joint PDF of its ordered eigenvalues,≥入2≥…入nis 2 i<i 7
7 2.3 Wigner Matrices Definition 2.3: An n×n Hermitian matrix W is a Wigner matrix if its upper-triangular entries are independent zero-mean random variables with identical variance. If the variance is 1/n, then W is a standard Wigner matrix. Theorem 2.3: Let W be an n × n complex Wigner matrix whose (diagonal and upper-triangle) entries are i.i.d. zero-mean Gaussian with unit variance. Then, its PDF is 2 2 [ ] /2 /2 2 2 tr n n e W while the joint PDF of its ordered eigenvalues is 1 2 n 2 1 1 1 2 2 / 2 1 1 1 ( ) 2 ! n i i n n n i j i i j e i
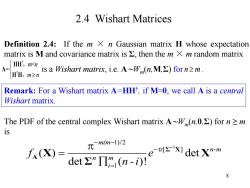
2.4 Wishart Matrices Definition 2.4:If the m x n Gaussian matrix H whose expectation matrix is M and covariance matrix is >then the m X m random matrix A= H,m”is a Wishart matrix,ie.A~Wmn,M,)orn≥m. H'H,m≥n Remark:For a Wishart matrix A=HH',if M=0,we call A is a central Wishart matrix. The PDF of the central complex Wishart matrix A~W,(n,0,>)for n=m iS 元-mm-l)/2 X)= e-txl det xn-m detΣ"Π,(n-i)! 8
8 2.4 Wishart Matrices Definition 2.4: If the m × n Gaussian matrix H whose expectation matrix is M and covariance matrix is Σ, then the m × m random matrix is a Wishart matrix, i.e. A∼Wm (n,M,Σ) for . 1 1)/2 tr[ ] 1 ( ) det det ( )! m(m n-m n m i f e n - i Σ X A X X Σ Remark: For a Wishart matrix A=HH† , if M=0, we call A is a central Wishart matrix. † † < = m n m n HH A H H , , The PDF of the central complex Wishart matrix A∼Wm (n,0,Σ) for n ≥ m is n m
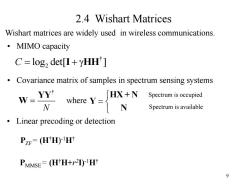
2.4 Wishart Matrices Wishart matrices are widely used in wireless communications. ·MIMO capacity C=log,det[I+yHH'] Covariance matrix of samples in spectrum sensing systems YY HX+N Spectrum is occupied N where YN Spectrum is available Linear precoding or detection PZF=(HH)H PMMSE=(HTH+r21)H? 9
9 2.4 Wishart Matrices Wishart matrices are widely used in wireless communications. • MIMO capacity † 2 C log det[ ] I HH • Covariance matrix of samples in spectrum sensing systems † N YY W where HX + N Y N Spectrum is occupied Spectrum is available • Linear precoding or detection PZF= (H†H) -1H† PMMSE= (H†H+r 2 I)-1H†

2.4 Wishart Matrices Theorem 2.4.1:The entry of HE CTRx be i.i.d.Gaussian variable with zero mean and unit variance,with n zm.So Complex central Wishart matrix HH=W.EHH=I.,EH'H=I then we have for ks m and k≤n E[det(H2%)det(H月 k!ifi=vh=uik=VxJk=uk 0 otherwise where det()is a minor determinant ofX 10
10 2.4 Wishart Matrices Theorem 2.4.1: The entry of be i.i.d. Gaussian variable with zero mean and unit variance, with n ≥m. So Complex central Wishart matrix HH†= W, , m n H † HH I m † H H I n , then we have for and k m k n 1 2, 1 2, 1 2, 1 2, , , † , , 1 1 1 1 [det( )det( )] ! , , 0 k k k k i i i u u u j j j v v v k k k k k if i v j u i v j u otherwise H H where is a minor determinant of 1 2, 1 2, X , , det( ) k k i i i Xj j j
按次数下载不扣除下载券;
注册用户24小时内重复下载只扣除一次;
顺序:VIP每日次数-->可用次数-->下载券;
- 电子科技大学:《贝叶斯学习与随机矩阵及在无线通信中的应用 BI-RM-AWC》课程教学资源(课件讲稿)01 Introduction of Wireless Channel and Random Matrices(陈智).pdf
- 《贝叶斯学习与随机矩阵及在无线通信中的应用 BI-RM-AWC》课程教学资源(文献书籍)Random Matrix Theory and Wireless Communications.pdf
- 《贝叶斯学习与随机矩阵及在无线通信中的应用 BI-RM-AWC》课程教学资源(文献书籍)PRML中文版——模式识别与机器学习.pdf
- 《贝叶斯学习与随机矩阵及在无线通信中的应用 BI-RM-AWC》课程教学资源(文献书籍)Pattern Recognition and Machine Learning.pdf
- 电子科技大学:《时域测试技术综合实验 Comprehensive Experiment of Time Domain Testing Technology》课程教学资源(课件讲稿)实验十四 虚拟数字示波器实验.pdf
- 电子科技大学:《时域测试技术综合实验 Comprehensive Experiment of Time Domain Testing Technology》课程教学资源(课件讲稿)实验十三 基于FPGA的地址译码实验.pdf
- 电子科技大学:《时域测试技术综合实验 Comprehensive Experiment of Time Domain Testing Technology》课程教学资源(课件讲稿)实验十二 数字示波器信号调理通道实验.pdf
- 电子科技大学:《时域测试技术综合实验 Comprehensive Experiment of Time Domain Testing Technology》课程教学资源(课件讲稿)实验十一 数字示波器协议触发与解码应用测试.pdf
- 电子科技大学:《时域测试技术综合实验 Comprehensive Experiment of Time Domain Testing Technology》课程教学资源(课件讲稿)实验十 时域反射实验.pdf
- 电子科技大学:《时域测试技术综合实验 Comprehensive Experiment of Time Domain Testing Technology》课程教学资源(课件讲稿)实验九 参数测量实验.pdf
- 电子科技大学:《时域测试技术综合实验 Comprehensive Experiment of Time Domain Testing Technology》课程教学资源(课件讲稿)实验八 数字示波器中的信号插值.pdf
- 电子科技大学:《时域测试技术综合实验 Comprehensive Experiment of Time Domain Testing Technology》课程教学资源(课件讲稿)实验七 信号采集抽取功能设计实验.pdf
- 电子科技大学:《时域测试技术综合实验 Comprehensive Experiment of Time Domain Testing Technology》课程教学资源(课件讲稿)实验六 信号采集触发功能设计实验.pdf
- 电子科技大学:《时域测试技术综合实验 Comprehensive Experiment of Time Domain Testing Technology》课程教学资源(课件讲稿)实验五 数据采集动态性能评估方法.pdf
- 电子科技大学:《时域测试技术综合实验 Comprehensive Experiment of Time Domain Testing Technology》课程教学资源(课件讲稿)实验四 基于FIFO采样与存储.pdf
- 电子科技大学:《时域测试技术综合实验 Comprehensive Experiment of Time Domain Testing Technology》课程教学资源(课件讲稿)实验三 信号产生实验.pdf
- 电子科技大学:《时域测试技术综合实验 Comprehensive Experiment of Time Domain Testing Technology》课程教学资源(课件讲稿)实验二 FPGA开发环境与基本设计流程.pdf
- 电子科技大学:《时域测试技术综合实验 Comprehensive Experiment of Time Domain Testing Technology》课程教学资源(课件讲稿)实验一 时域测试仪器原理及典型应用.pdf
- 电子科技大学:《时域测试技术综合实验 Comprehensive Experiment of Time Domain Testing Technology》课程教学资源(课件讲稿)概述.pdf
- 电子科技大学:《时域测试技术综合实验 Comprehensive Experiment of Time Domain Testing Technology》课程教学资源(教学大纲,邱渡裕).pdf
- 电子科技大学:《贝叶斯学习与随机矩阵及在无线通信中的应用 BI-RM-AWC》课程教学资源(课件讲稿)03 Transforms.pdf
- 电子科技大学:《贝叶斯学习与随机矩阵及在无线通信中的应用 BI-RM-AWC》课程教学资源(课件讲稿)04 Asymptotic Spectrum Theorems(1/4).pdf
- 《贝叶斯学习与随机矩阵及在无线通信中的应用 BI-RM-AWC》课程教学资源(文献书籍)An Introduction to Random Matrices(Greg W. Anderson、Alice Guionnet).pdf
- 电子科技大学:《贝叶斯学习与随机矩阵及在无线通信中的应用 BI-RM-AWC》课程教学资源(课件讲稿)04 Asymptotic Spectrum Theorems(2/4).pdf
- 电子科技大学:《贝叶斯学习与随机矩阵及在无线通信中的应用 BI-RM-AWC》课程教学资源(课件讲稿)04 Asymptotic Spectrum Theorems(3/4).pdf
- 电子科技大学:《贝叶斯学习与随机矩阵及在无线通信中的应用 BI-RM-AWC》课程教学资源(课件讲稿)04 Asymptotic Spectrum Theorems(4/4).pdf
- 电子科技大学:《贝叶斯学习与随机矩阵及在无线通信中的应用 BI-RM-AWC》课程教学资源(课件讲稿)06 Non-asymptotic Analysis for Large Random Matrix.pdf
- 电子科技大学:《贝叶斯学习与随机矩阵及在无线通信中的应用 BI-RM-AWC》课程教学资源(课件讲稿)07 Analysis of neural networks - a random matrix approach.pdf
- 电子科技大学:《贝叶斯学习与随机矩阵及在无线通信中的应用 BI-RM-AWC》课程教学资源(课件讲稿)05 Free Probability.pdf
- 《贝叶斯学习与随机矩阵及在无线通信中的应用 BI-RM-AWC》课程教学资源(学习资料)Random Matrix Theory and Wireless Communications.pdf
- 电子科技大学:《贝叶斯学习与随机矩阵及在无线通信中的应用 BI-RM-AWC》课程教学资源(课件讲稿)08 Linear Regression.pdf
- 电子科技大学:《贝叶斯学习与随机矩阵及在无线通信中的应用 BI-RM-AWC》课程教学资源(课件讲稿)09 Sparse Signal Recovery.pdf
- 电子科技大学:《贝叶斯学习与随机矩阵及在无线通信中的应用 BI-RM-AWC》课程教学资源(学习资料)贝叶斯学习补充材料.pdf
- 电子科技大学:《贝叶斯学习与随机矩阵及在无线通信中的应用 BI-RM-AWC》课程教学资源(学习资料)随机矩阵补充材料 Analysis of neural networks - a random matrix approach.pdf
- 广东海洋大学:《数字信号处理 Digital Signal Processing》课程教学资源(电子教案).doc
- 电子科技大学:《ASIC设计 Application Specific Integrated Circuit Design(ASIC)》课程教学资源(课件讲稿)Topic 1 Introduction(About IC technology).pdf
- 电子科技大学:《ASIC设计 Application Specific Integrated Circuit Design(ASIC)》课程教学资源(课件讲稿)Topic 1 Introduction(About ASIC Design).pdf
- 电子科技大学:《ASIC设计 Application Specific Integrated Circuit Design(ASIC)》课程教学资源(课件讲稿)Topic1 Introduction(About Our Course).pdf
- 电子科技大学:《ASIC设计 Application Specific Integrated Circuit Design(ASIC)》课程教学资源(课件讲稿)Topic 2 FPGA Design with Verilog(FPGA Design Method、Design Examples).pdf
- 电子科技大学:《ASIC设计 Application Specific Integrated Circuit Design(ASIC)》课程教学资源(课件讲稿)Topic 2 FPGA Design with Verilog(Supplementary).pdf