电子科技大学:《GPU并行编程 GPU Parallel Programming》课程教学资源(课件讲稿)Lecture 04 Performance considerations
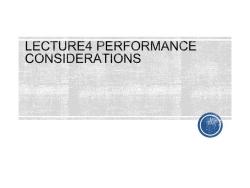
LECTURE4 PERFORMANCE CONSIDERATIONS
Warps and SIMD Hardware
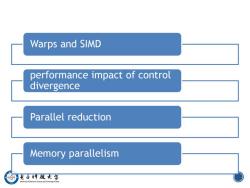
Warps and SIMD performance impact of control divergence Parallel reduction Memory parallelism 电子件做女字
2 Warps and SIMD performance impact of control divergence Parallel reduction Memory parallelism
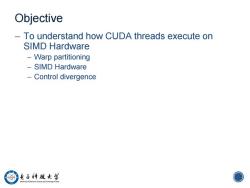
Objective To understand how CUDA threads execute on SIMD Hardware Warp partitioning -SIMD Hardware - Control divergence 电子科妓女学 O
3 Objective – To understand how CUDA threads execute on SIMD Hardware – Warp partitioning – SIMD Hardware – Control divergence
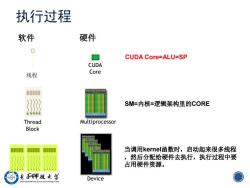
执行过程 软件 硬件 0 □ CUDA Core=ALU=SP CUDA 线程 Core 瑙 SM=内核=逻辑架构里的CORE Thread Multiprocessor Block 当调用kerneli函数时,启动起来很多线程 ,然后分配给硬件去执行,执行过程中要 占用硬件资源。 电F神线女学 Device
4 软件 硬件 CUDA Core=ALU=SP 线程 CUDA Core Thread Block Multiprocessor Grid 执行过程 Device SM=内核=逻辑架构里的CORE 当调用kernel函数时,启动起来很多线程 ,然后分配给硬件去执行,执行过程中要 占用硬件资源
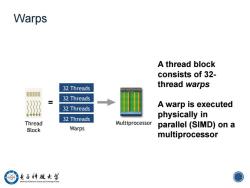
Warps A thread block consists of 32- 32 Threads thread warps 32 Threads 汹 日 32 Threads A warp is executed physically in 32 Threads Thread Multiprocessor parallel (SIMD)on a Block Warps multiprocessor 电子科妓女学 O
5 Multiprocessor 32 Threads Warps A thread block consists of 32- thread warps A warp is executed physically in parallel (SIMD) on a multiprocessor = Warps Thread Block 32 Threads 32 Threads 32 Threads
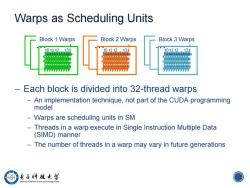
Warps as Scheduling Units Block 1 Warps Block 2 Warps Block 3 Warps t0t1t2.t31 0.t1t to t1 t2...t31 一 Each block is divided into 32-thread warps An implementation technique,not part of the CUDA programming model - Warps are scheduling units in SM Threads in a warp execute in Single Instruction Multiple Data (SIMD)manner The number of threads in a warp may vary in future generations 电子科妓女学 O
6 Warps as Scheduling Units – Each block is divided into 32-thread warps – An implementation technique, not part of the CUDA programming model – Warps are scheduling units in SM – Threads in a warp execute in Single Instruction Multiple Data (SIMD) manner – The number of threads in a warp may vary in future generations … …t0 t1 t2 … t31 … …t0 t1 t2 … t31 Block 1 Warps Block 2 Warps … …t0 t1 t2 … t31 Block 3 Warps
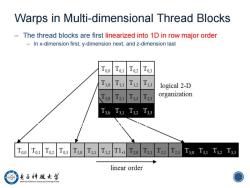
Warps in Multi-dimensional Thread Blocks The thread blocks are first linearized into 1D in row major order In x-dimension first,y-dimension next,and z-dimension last Too Tol T02 T03 T10 T11 T12 T13 logical 2-D T20 T21 T22 T23 organization T30T31T32T33 T00 T01 T02T031 T2T13T20 T21T22T23T30T31T32T33 linear order 例 电子神越女学 O
7 Warps in Multi-dimensional Thread Blocks – The thread blocks are first linearized into 1D in row major order – In x-dimension first, y-dimension next, and z-dimension last 7 Figure 6.1: Placing 2D threads into linear order
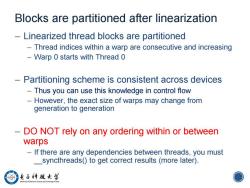
Blocks are partitioned after linearization Linearized thread blocks are partitioned Thread indices within a warp are consecutive and increasing Warp 0 starts with Thread 0 -Partitioning scheme is consistent across devices Thus you can use this knowledge in control flow However,the exact size of warps may change from generation to generation DO NOT rely on any ordering within or between warps If there are any dependencies between threads,you must _syncthreads()to get correct results(more later). 电子科妓女学
8 Blocks are partitioned after linearization – Linearized thread blocks are partitioned – Thread indices within a warp are consecutive and increasing – Warp 0 starts with Thread 0 – Partitioning scheme is consistent across devices – Thus you can use this knowledge in control flow – However, the exact size of warps may change from generation to generation – DO NOT rely on any ordering within or between warps – If there are any dependencies between threads, you must __syncthreads() to get correct results (more later)
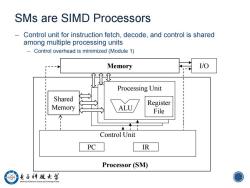
SMs are SIMD Processors Control unit for instruction fetch,decode,and control is shared among multiple processing units Control overhead is minimized (Module 1) Memory I/O Processing Unit Shared Memory ALU/ Register File Control Unit PC IR Processor (SM) 电子神越女学 Uaimraity at Eleetreie Scieeand Teclegd O
9 SMs are SIMD Processors – Control unit for instruction fetch, decode, and control is shared among multiple processing units – Control overhead is minimized (Module 1) Memory Processing Unit I/O ALU Processor (SM) Shared Memory Register File Control Unit PC IR
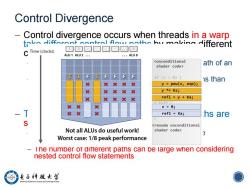
Control Divergence Control divergence occurs when threads in a warp toko Nifforont control flownth hy mokin Nifferent C Time(clocks) ALU1 ALU2 .. ·ALU8 TT F T FFF P 1f(×>0)( is than X y pow(x,exp); X XX然 y *Ks; X XXXX refl =y Ka; else X然 X X=0; x refl Ka; hs are Worst case:1/8 peak performance i ne numper ot ditterent patns can be large when considering nested control flow statements 电子神越女学 0
10 Control Divergence – Control divergence occurs when threads in a warp take different control flow paths by making different control decisions – Some take the then-path and others take the else-path of an if-statement – Some threads take different number of loop iterations than others – The execution of threads taking different paths are serialized in current GPUs – The control paths taken by the threads in a warp are traversed one at a time until there is no more. – The number of different paths can be large when considering nested control flow statements
按次数下载不扣除下载券;
注册用户24小时内重复下载只扣除一次;
顺序:VIP每日次数-->可用次数-->下载券;
- 电子科技大学:《GPU并行编程 GPU Parallel Programming》课程教学资源(课件讲稿)Lecture 03 MEMORY AND DATA LOCALITY.pdf
- 电子科技大学:《GPU并行编程 GPU Parallel Programming》课程教学资源(课件讲稿)Lecture 02 CUDA PARALLELISM MODEL.pdf
- 电子科技大学:《GPU并行编程 GPU Parallel Programming》课程教学资源(课件讲稿)Lecture 01 Introduction To Cuda C.pdf
- 《GPU并行编程 GPU Parallel Programming》课程教学资源(参考文献)NVIDIA CUDA C Programming Guide(Design Guide,June 2017).pdf
- 《GPU并行编程 GPU Parallel Programming》课程教学资源(参考文献)Methods of conjugate gradients for solving linear systems.pdf
- 《GPU并行编程 GPU Parallel Programming》课程教学资源(参考文献)NVIDIA Parallel Prefix Sum(Scan)with CUDA(April 2007).pdf
- 《GPU并行编程 GPU Parallel Programming》课程教学资源(参考文献)Single-pass Parallel Prefix Scan with Decoupled Look-back.pdf
- 《GPU并行编程 GPU Parallel Programming》课程教学资源(参考文献)Program Optimization Space Pruning for a Multithreaded GPU.pdf
- 《GPU并行编程 GPU Parallel Programming》课程教学资源(参考文献)Optimization Principles and Application Performance Evaluation of a Multithreaded GPU Using CUDA.pdf
- 《GPU并行编程 GPU Parallel Programming》课程教学资源(参考文献)Some Computer Organizations and Their Effectiveness.pdf
- 《GPU并行编程 GPU Parallel Programming》课程教学资源(参考文献)Software and the Concurrency Revolution.pdf
- 《GPU并行编程 GPU Parallel Programming》课程教学资源(参考文献)An Asymmetric Distributed Shared Memory Model for Heterogeneous Parallel Systems.pdf
- 《GPU并行编程 GPU Parallel Programming》课程教学资源(参考文献)MPI A Message-Passing Interface Standard(Version 2.2).pdf
- 南京大学:《网络安全与入侵检测 Network Security and Intrusion Detection》课程教学资源(课件讲稿)19 Firewall Design Methods.pdf
- 南京大学:《网络安全与入侵检测 Network Security and Intrusion Detection》课程教学资源(课件讲稿)18 Web Security(SQL Injection and Cross-Site Request Forgery).pdf
- 南京大学:《网络安全与入侵检测 Network Security and Intrusion Detection》课程教学资源(课件讲稿)17 Web Security(Cookies and Cross Site Scripting,XSS).pdf
- 南京大学:《网络安全与入侵检测 Network Security and Intrusion Detection》课程教学资源(课件讲稿)16 Bloom Filter for Network Security.pdf
- 南京大学:《网络安全与入侵检测 Network Security and Intrusion Detection》课程教学资源(课件讲稿)15 Bloom Filters and its Variants.pdf
- 南京大学:《网络安全与入侵检测 Network Security and Intrusion Detection》课程教学资源(课件讲稿)14 Buffer Overflow Attacks.pdf
- 南京大学:《网络安全与入侵检测 Network Security and Intrusion Detection》课程教学资源(课件讲稿)13 Human Authentication.pdf
- 电子科技大学:《GPU并行编程 GPU Parallel Programming》课程教学资源(课件讲稿)Lecture 05 PARALLEL COMPUTATION PATTERNS(HISTOGRAM).pdf
- 电子科技大学:《GPU并行编程 GPU Parallel Programming》课程教学资源(课件讲稿)Lecture 06 PARALLEL COMPUTATION PATTERNS(SCAN).pdf
- 电子科技大学:《GPU并行编程 GPU Parallel Programming》课程教学资源(课件讲稿)Lecture 07 JOINT CUDA-MPI PROGRAMMING.pdf
- 电子科技大学:《GPU并行编程 GPU Parallel Programming》课程教学资源(课件讲稿)Lecture 08 Parallel Sparse Methods.pdf
- 电子科技大学:《GPU并行编程 GPU Parallel Programming》课程教学资源(课件讲稿)Lecture 09 Parallel patterns(MERGE SORT).pdf
- 电子科技大学:《GPU并行编程 GPU Parallel Programming》课程教学资源(课件讲稿)Lecture 10 Computational Thinking.pdf
- 电子科技大学:《有限元理论与建模方法 Finite Element Analysis and Modeling》研究生课程教学资源(课件讲稿)课程简介(杜平安).pdf
- 电子科技大学:《有限元理论与建模方法 Finite Element Analysis and Modeling》研究生课程教学资源(课件讲稿)第一章 绪论.pdf
- 电子科技大学:《有限元理论与建模方法 Finite Element Analysis and Modeling》研究生课程教学资源(课件讲稿)第二章 有限元法的基本原理(平面问题有限元法).pdf
- 电子科技大学:《有限元理论与建模方法 Finite Element Analysis and Modeling》研究生课程教学资源(课件讲稿)第七章 动态分析有限元法 FEM of Dynamic Analysis.pdf
- 电子科技大学:《有限元理论与建模方法 Finite Element Analysis and Modeling》研究生课程教学资源(课件讲稿)第3~6章 其他问题有限元法.pdf
- 电子科技大学:《有限元理论与建模方法 Finite Element Analysis and Modeling》研究生课程教学资源(课件讲稿)第八章 热分析有限元法 FEM of Thermal Analysis.pdf
- 电子科技大学:《有限元理论与建模方法 Finite Element Analysis and Modeling》研究生课程教学资源(课件讲稿)第二篇 有限元建模方法 第十二章 有限元建模概述 Overview of Finite Element Modeling.pdf
- 电子科技大学:《有限元理论与建模方法 Finite Element Analysis and Modeling》研究生课程教学资源(课件讲稿)第二篇 有限元建模方法 第十一章 有限元建模的基本原则.pdf
- 电子科技大学:《有限元理论与建模方法 Finite Element Analysis and Modeling》研究生课程教学资源(课件讲稿)第二篇 有限元建模方法 第十四章 几何模型的建立.pdf
- 电子科技大学:《有限元理论与建模方法 Finite Element Analysis and Modeling》研究生课程教学资源(课件讲稿)第二篇 有限元建模方法 第十五章 单元类型及特性定义.pdf
- 电子科技大学:《有限元理论与建模方法 Finite Element Analysis and Modeling》研究生课程教学资源(课件讲稿)第二篇 有限元建模方法 第十六章 网格划分方法.pdf
- 电子科技大学:《有限元理论与建模方法 Finite Element Analysis and Modeling》研究生课程教学资源(课件讲稿)第二篇 有限元建模方法 第十七章 模型检查与处理 Model Checking and Processing.pdf
- 电子科技大学:《有限元理论与建模方法 Finite Element Analysis and Modeling》研究生课程教学资源(课件讲稿)第二篇 有限元建模方法 第十八章 边界条件的建立 Creation of Boundary Condition.pdf
- 南京大学:《高级算法 Advanced Algorithms》课程教学资源(课件讲稿)Fingerprinting.pdf