《Artificial Intelligence:A Modern Approach》教学资源(PPT课件,英文版)Chapter 18-Learning from Observations
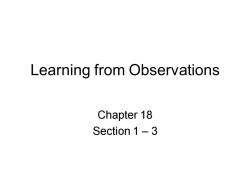
Learning from Observations Chapter 18 Section 1 -3
Learning from Observations Chapter 18 Section 1 – 3
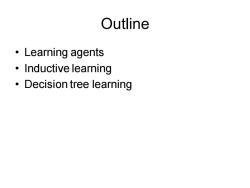
Outline ·Learning agents ·Inductive learning Decision tree learning
Outline • Learning agents • Inductive learning • Decision tree learning
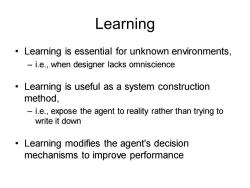
Learning Learning is essential for unknown environments, i.e.,when designer lacks omniscience Learning is useful as a system construction method, -i.e.,expose the agent to reality rather than trying to write it down Learning modifies the agent's decision mechanisms to improve performance
Learning • Learning is essential for unknown environments, – i.e., when designer lacks omniscience • Learning is useful as a system construction method, – i.e., expose the agent to reality rather than trying to write it down • Learning modifies the agent's decision mechanisms to improve performance
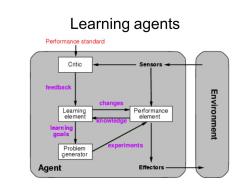
Learning agents Performance standard Critic Sensors feedback changes Learning Performance element element knowledge learn ing Environment goals Problem experiments generator Agent Effectors
Learning agents
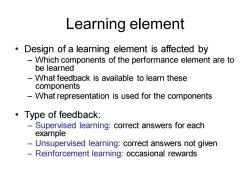
Learning element Design of a learning element is affected by -Which components of the performance element are to be learned What feedback is available to learn these components -What representation is used for the components ·Type of feedback: Supervised learning:correct answers for each example Unsupervised learning:correct answers not given Reinforcement learning:occasional rewards
Learning element • Design of a learning element is affected by – Which components of the performance element are to be learned – What feedback is available to learn these components – What representation is used for the components • Type of feedback: – Supervised learning: correct answers for each example – Unsupervised learning: correct answers not given – Reinforcement learning: occasional rewards
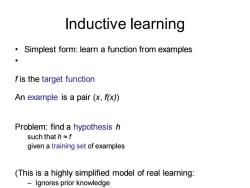
Inductive learning Simplest form:learn a function from examples f is the target function An example is a pair (x,f(x)) Problem:find a hypothesis h such that h≈f given a training set of examples (This is a highly simplified model of real learning: Ignores prior knowledge
Inductive learning • Simplest form: learn a function from examples • f is the target function An example is a pair (x, f(x)) Problem: find a hypothesis h such that h ≈ f given a training set of examples (This is a highly simplified model of real learning: – Ignores prior knowledge – Assumes examples are given)
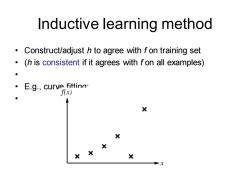
Inductive learning method Construct/adjust h to agree with f on training set (h is consistent if it agrees with fon all examples) E.g.,curve fittinn f(x) × X X X X
Inductive learning method • Construct/adjust h to agree with f on training set • (h is consistent if it agrees with f on all examples) • • E.g., curve fitting: •

Inductive learning method Construct/adjust h to agree with f on training set (h is consistent if it agrees with f on all examples) E.g.,curve fittinn. f(x)
Inductive learning method • Construct/adjust h to agree with f on training set • (h is consistent if it agrees with f on all examples) • • E.g., curve fitting: •
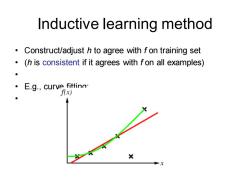
Inductive learning method Construct/adjust h to agree with f on training set (h is consistent if it agrees with fon all examples) ● E.g.,curve fittinn. f(x)
Inductive learning method • Construct/adjust h to agree with f on training set • (h is consistent if it agrees with f on all examples) • • E.g., curve fitting: •
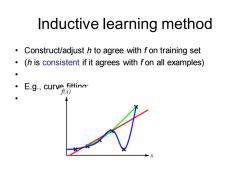
Inductive learning method Construct/adjust h to agree with f on training set (h is consistent if it agrees with f on all examples) E.g.,curve fittinn. f(x)
Inductive learning method • Construct/adjust h to agree with f on training set • (h is consistent if it agrees with f on all examples) • • E.g., curve fitting: •
按次数下载不扣除下载券;
注册用户24小时内重复下载只扣除一次;
顺序:VIP每日次数-->可用次数-->下载券;
- 《Artificial Intelligence:A Modern Approach》教学资源(PPT课件,英文版)Chapter 14-Bayesian networks.ppt
- 《Artificial Intelligence:A Modern Approach》教学资源(PPT课件,英文版)Chapter 13-Uncertainty.ppt
- 《Artificial Intelligence:A Modern Approach》教学资源(PPT课件,英文版)Chapter 1-Introduction.ppt
- 《Artificial Intelligence:A Modern Approach》教学资源(讲义,英文版)chapter25.pdf
- 《Artificial Intelligence:A Modern Approach》教学资源(讲义,英文版)chapter25-6pp.pdf
- 《Artificial Intelligence:A Modern Approach》教学资源(讲义,英文版)chapter22.pdf
- 《Artificial Intelligence:A Modern Approach》教学资源(讲义,英文版)chapter22-6pp.pdf
- 《Artificial Intelligence:A Modern Approach》教学资源(讲义,英文版)chapter20b.pdf
- 《Artificial Intelligence:A Modern Approach》教学资源(讲义,英文版)chapter20b-6pp.pdf
- 《Artificial Intelligence:A Modern Approach》教学资源(讲义,英文版)chapter20a.pdf
- 《Artificial Intelligence:A Modern Approach》教学资源(讲义,英文版)chapter20a-6pp.pdf
- 《Artificial Intelligence:A Modern Approach》教学资源(讲义,英文版)chapter18.pdf
- 《Artificial Intelligence:A Modern Approach》教学资源(讲义,英文版)chapter18-6pp.pdf
- 《Artificial Intelligence:A Modern Approach》教学资源(讲义,英文版)chapter16.pdf
- 《Artificial Intelligence:A Modern Approach》教学资源(讲义,英文版)chapter16-6pp.pdf
- 《Artificial Intelligence:A Modern Approach》教学资源(讲义,英文版)chapter15b.pdf
- 《Artificial Intelligence:A Modern Approach》教学资源(讲义,英文版)chapter15b-6pp.pdf
- 《Artificial Intelligence:A Modern Approach》教学资源(讲义,英文版)chapter15a.pdf
- 《Artificial Intelligence:A Modern Approach》教学资源(讲义,英文版)chapter15a-6pp.pdf
- 《Artificial Intelligence:A Modern Approach》教学资源(讲义,英文版)chapter14b.pdf
- 《Artificial Intelligence:A Modern Approach》教学资源(PPT课件,英文版)Chapter 2-Intelligent Agents.ppt
- 《Artificial Intelligence:A Modern Approach》教学资源(PPT课件,英文版)Chapter 3-Solving problems by searching.ppt
- 《Artificial Intelligence:A Modern Approach》教学资源(PPT课件,英文版)Chapter 4-Informed search algorithms.ppt
- 《Artificial Intelligence:A Modern Approach》教学资源(PPT课件,英文版)Chapter 5-Constraint Satisfaction Problems.ppt
- 《Artificial Intelligence:A Modern Approach》教学资源(PPT课件,英文版)Chapter 6-Adversarial Search.ppt
- 《Artificial Intelligence:A Modern Approach》教学资源(PPT课件,英文版)Chapter 7-Logical Agents.ppt
- 《Artificial Intelligence:A Modern Approach》教学资源(PPT课件,英文版)Chapter 8-First-Order Logic.ppt
- 《Artificial Intelligence:A Modern Approach》教学资源(PPT课件,英文版)Chapter 9-Inference in first-order logic.ppt
- 中国科学技术大学:《人工智能基础》课程教学资源(课件讲稿)Lecture 02 Intelligent Agents.pdf
- 中国科学技术大学:《人工智能基础》课程教学资源(课件讲稿)Lecture 03 Solving Problems by Searching.pdf
- 中国科学技术大学:《人工智能基础》课程教学资源(课件讲稿)Lecture 04 Informed Search.pdf
- 中国科学技术大学:《人工智能基础》课程教学资源(课件讲稿)Lecture 05 Constraint Satisfaction Problems.pdf
- 中国科学技术大学:《人工智能基础》课程教学资源(课件讲稿)Lecture 06 Game Playing.pdf
- 中国科学技术大学:《人工智能基础》课程教学资源(课件讲稿)Lecture 07 Logical Agents.pdf
- 中国科学技术大学:《人工智能基础》课程教学资源(课件讲稿)Lecture 10 Uncertainty and Bayesian Networks.pdf
- 中国科学技术大学:《人工智能基础》课程教学资源(课件讲稿)Lecture 11 马尔可夫决策过程.pdf
- 中国科学技术大学:《人工智能基础》课程教学资源(课件讲稿)Lecture 08 First-Order Logic and Inference in FOL.pdf
- 中国科学技术大学:《人工智能基础》课程教学资源(课件讲稿)Lecture 09 AI Planning.pdf
- 中国科学技术大学:《人工智能基础》课程教学资源(课件讲稿)Lecture 13 神经网络与深度学习.pdf
- 中国科学技术大学:《人工智能基础》课程教学资源(课件讲稿)Lecture 14 Reinforcement Learning.pdf