电子科技大学:《统计学习理论及应用 Statistical Learning Theory and Applications》课程教学资源(课件讲稿)第七讲 非线性分类模型——集成方法
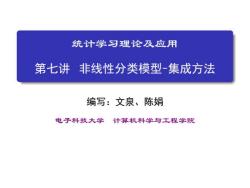
统计学习理论及应用 第七讲非线性分类模型-集成方法 编写:文泉、陈娟 电子科技大学计算机科学与工程学院
统计学习理论及应用 第七讲 非线性分类模型–集成方法 编写:文泉、陈娟 电子科技大学 计算机科学与工程学院
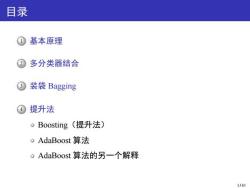
目录 ①基本原理 2 多分类器结合 装袋Bagging 提升法 。Boosting(提升法) 。AdaBoost算法 o AdaBoost算法的另一个解释 1/41
目录 1 基本原理 2 多分类器结合 3 装袋 Bagging 4 提升法 Boosting(提升法) AdaBoost 算法 AdaBoost 算法的另一个解释 1 / 41
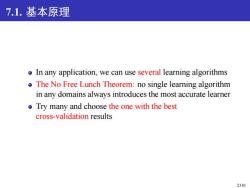
7.1.基本原理 o In any application,we can use several learning algorithms o The No Free Lunch Theorem:no single learning algorithm in any domains always introduces the most accurate learner o Try many and choose the one with the best cross-validation results 2/41
7.1. 基本原理 In any application, we can use several learning algorithms The No Free Lunch Theorem: no single learning algorithm in any domains always introduces the most accurate learner Try many and choose the one with the best cross-validation results 2 / 41
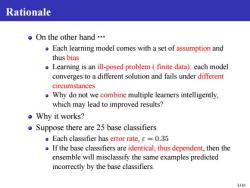
Rationale o On the other hand.. Each learning model comes with a set of assumption and thus bias Learning is an ill-posed problem finite data):each model converges to a different solution and fails under different circumstances Why do not we combine multiple learners intelligently, which may lead to improved results? Why it works? Suppose there are 25 base classifiers Each classifier has error rate,e=0.35 o If the base classifiers are identical,thus dependent,then the ensemble will misclassify the same examples predicted incorrectly by the base classifiers. 3/41
Rationale On the other hand … Each learning model comes with a set of assumption and thus bias Learning is an ill-posed problem ( finite data): each model converges to a different solution and fails under different circumstances Why do not we combine multiple learners intelligently, which may lead to improved results? Why it works? Suppose there are 25 base classifiers Each classifier has error rate, ε = 0.35 If the base classifiers are identical, thus dependent, then the ensemble will misclassify the same examples predicted incorrectly by the base classifiers. 3 / 41
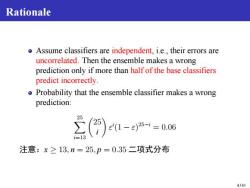
Rationale o Assume classifiers are independent,i.e.,their errors are uncorrelated.Then the ensemble makes a wrong prediction only if more than half of the base classifiers predict incorrectly. o Probability that the ensemble classifier makes a wrong prediction: 25 25 e(1-e)25-=0.06 i=13 注意:x≥13,n=25,p=0.35二项式分布 4/41
Rationale Assume classifiers are independent, i.e., their errors are uncorrelated. Then the ensemble makes a wrong prediction only if more than half of the base classifiers predict incorrectly. Probability that the ensemble classifier makes a wrong prediction: X 25 i=13 25 i ε i (1 − ε) 25−i = 0.06 注意:x ≥ 13, n = 25, p = 0.35 二项式分布 4 / 41
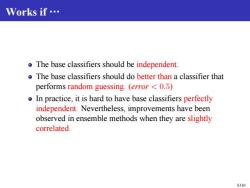
Works if... o The base classifiers should be independent o The base classifiers should do better than a classifier that performs random guessing.(error 0.5) o In practice,it is hard to have base classifiers perfectly independent.Nevertheless,improvements have been observed in ensemble methods when they are slightly correlated. 5/41
Works if … The base classifiers should be independent. The base classifiers should do better than a classifier that performs random guessing. (error < 0.5) In practice, it is hard to have base classifiers perfectly independent. Nevertheless, improvements have been observed in ensemble methods when they are slightly correlated. 5 / 41
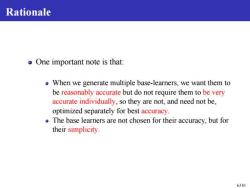
Rationale o One important note is that: When we generate multiple base-learners,we want them to be reasonably accurate but do not require them to be very accurate individually,so they are not,and need not be, optimized separately for best accuracy. The base learners are not chosen for their accuracy,but for their simplicity. 6/41
Rationale One important note is that: When we generate multiple base-learners, we want them to be reasonably accurate but do not require them to be very accurate individually, so they are not, and need not be, optimized separately for best accuracy. The base learners are not chosen for their accuracy, but for their simplicity. 6 / 41
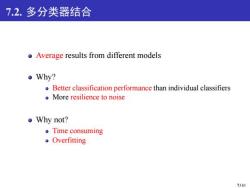
7.2.多分类器结合 o Average results from different models o Why? Better classification performance than individual classifiers More resilience to noise o Why not? Time consuming o Overfitting 7/41
7.2. 多分类器结合 Average results from different models Why? Better classification performance than individual classifiers More resilience to noise Why not? Time consuming Overfitting 7 / 41
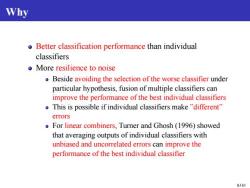
Why o Better classification performance than individual classifiers o More resilience to noise Beside avoiding the selection of the worse classifier under particular hypothesis,fusion of multiple classifiers can improve the performance of the best individual classifiers o This is possible if individual classifiers make"different" errors For linear combiners,Turner and Ghosh (1996)showed that averaging outputs of individual classifiers with unbiased and uncorrelated errors can improve the performance of the best individual classifier 8/41
Why Better classification performance than individual classifiers More resilience to noise Beside avoiding the selection of the worse classifier under particular hypothesis, fusion of multiple classifiers can improve the performance of the best individual classifiers This is possible if individual classifiers make ”different” errors For linear combiners, Turner and Ghosh (1996) showed that averaging outputs of individual classifiers with unbiased and uncorrelated errors can improve the performance of the best individual classifier 8 / 41
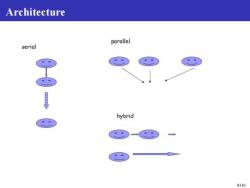
Architecture parallel serial hybrid 9/41
Architecture 9 / 41
按次数下载不扣除下载券;
注册用户24小时内重复下载只扣除一次;
顺序:VIP每日次数-->可用次数-->下载券;
- 电子科技大学:《统计学习理论及应用 Statistical Learning Theory and Applications》课程教学资源(课件讲稿)第六讲 非线性分类模型——多层感知机.pdf
- 电子科技大学:《统计学习理论及应用 Statistical Learning Theory and Applications》课程教学资源(课件讲稿)第五讲 支持向量机.pdf
- 电子科技大学:《统计学习理论及应用 Statistical Learning Theory and Applications》课程教学资源(课件讲稿)第四讲 感知机.pdf
- 电子科技大学:《统计学习理论及应用 Statistical Learning Theory and Applications》课程教学资源(课件讲稿)第三讲 回归模型.pdf
- 电子科技大学:《统计学习理论及应用 Statistical Learning Theory and Applications》课程教学资源(课件讲稿)第二讲 概率与线性代数回顾.pdf
- 电子科技大学:《统计学习理论及应用 Statistical Learning Theory and Applications》课程教学资源(课件讲稿)第一讲 概述(文泉、陈娟).pdf
- 电子科技大学:《统计学习理论及应用 Statistical Learning Theory and Applications》课程教学资源(课件讲稿,英文版)Lecture 10 Unsupervised Learning.pdf
- 电子科技大学:《统计学习理论及应用 Statistical Learning Theory and Applications》课程教学资源(课件讲稿,英文版)Lecture 09 Data Representation — Non-Parametric Model.pdf
- 电子科技大学:《统计学习理论及应用 Statistical Learning Theory and Applications》课程教学资源(课件讲稿,英文版)Lecture 08 Data Representation - Parametric Model.pdf
- 电子科技大学:《统计学习理论及应用 Statistical Learning Theory and Applications》课程教学资源(课件讲稿,英文版)Lecture 07 Non-Linear Classification Model - Ensemble Methods.pdf
- 电子科技大学:《统计学习理论及应用 Statistical Learning Theory and Applications》课程教学资源(课件讲稿,英文版)Lecture 06 Multilayer Perceptron.pdf
- 电子科技大学:《统计学习理论及应用 Statistical Learning Theory and Applications》课程教学资源(课件讲稿,英文版)Lecture 05 Support Vector Machine.pdf
- 电子科技大学:《统计学习理论及应用 Statistical Learning Theory and Applications》课程教学资源(课件讲稿,英文版)Lecture 04 Perceptron.pdf
- 电子科技大学:《统计学习理论及应用 Statistical Learning Theory and Applications》课程教学资源(课件讲稿,英文版)Lecture 03 Regression Models.pdf
- 电子科技大学:《统计学习理论及应用 Statistical Learning Theory and Applications》课程教学资源(课件讲稿,英文版)Lecture 02 Review of Linear Algebra and Probability Theory.pdf
- 电子科技大学:《统计学习理论及应用 Statistical Learning Theory and Applications》课程教学资源(课件讲稿,英文版)Lecture 01 Introduction.pdf
- 安顺学院:《经济统计学》专业新增学士学位授予权评审汇报PPT(吴永武).ppt
- 对外经济贸易大学:《应用统计 Applied Statistics》课程教学资源(教案讲稿).pdf
- 对外经济贸易大学:《应用统计 Applied Statistics》课程教学资源(教学大纲).pdf
- 上海交通大学:《统计原理 Principal of statistics》课程教学资源_大脑衰老与吃兴奋功能食品关系研究(调查问卷).doc
- 电子科技大学:《统计学习理论及应用 Statistical Learning Theory and Applications》课程教学资源(课件讲稿)第八讲 数据表示——含参模型.pdf
- 电子科技大学:《统计学习理论及应用 Statistical Learning Theory and Applications》课程教学资源(课件讲稿)第九讲 数据表示——不含参模型.pdf
- 电子科技大学:《统计学习理论及应用 Statistical Learning Theory and Applications》课程教学资源(课件讲稿)第十讲 非监督学习.pdf
- 中国人民大学:《应用随机过程 Applied Stochastic Processes》课程教学资源(课件讲稿)第10章 随机过程在保险精算中的应用.pdf
- 中国人民大学:《应用随机过程 Applied Stochastic Processes》课程教学资源(课件讲稿)第11章 Markov链Monte Carlo方法.pdf
- 中国人民大学:《应用随机过程 Applied Stochastic Processes》课程教学资源(课件讲稿)第1章 预备知识(张波、商豪、邓军).pdf
- 中国人民大学:《应用随机过程 Applied Stochastic Processes》课程教学资源(课件讲稿)第2章 随机过程的基本概念和类型.pdf
- 中国人民大学:《应用随机过程 Applied Stochastic Processes》课程教学资源(课件讲稿)第3章 Poisson过程.pdf
- 中国人民大学:《应用随机过程 Applied Stochastic Processes》课程教学资源(课件讲稿)第4章 更新过程.pdf
- 中国人民大学:《应用随机过程 Applied Stochastic Processes》课程教学资源(课件讲稿)第5章 Markov链.pdf
- 中国人民大学:《应用随机过程 Applied Stochastic Processes》课程教学资源(课件讲稿)第6章 鞅.pdf
- 中国人民大学:《应用随机过程 Applied Stochastic Processes》课程教学资源(课件讲稿)第7章 Brown运动.pdf
- 中国人民大学:《应用随机过程 Applied Stochastic Processes》课程教学资源(课件讲稿)第8章 随机积分.pdf
- 中国人民大学:《应用随机过程 Applied Stochastic Processes》课程教学资源(课件讲稿)第9章 随机过程在金融中的应用.pdf
- 贵州医科大学:《医学统计学 Medical Statistics》课程教学资源(教学大纲,打印版).pdf
- 贵州医科大学:《医学统计学 Medical Statistics》课程教学资源(习题,打印版).pdf
- 贵州医科大学:《医学统计学 Medical Statistics》课程教学资源(毕业实习指导,打印版).pdf
- 贵州医科大学:《医学统计学 Medical Statistics》课程教学资源(电子教案,打印版)01 绪论.pdf
- 贵州医科大学:《医学统计学 Medical Statistics》课程教学资源(电子教案,打印版)02 计量资料描述.pdf
- 贵州医科大学:《医学统计学 Medical Statistics》课程教学资源(电子教案,打印版)03 总体均数估计和假设检验.pdf