电子科技大学:《统计学习理论及应用 Statistical Learning Theory and Applications》课程教学资源(课件讲稿,英文版)Lecture 01 Introduction
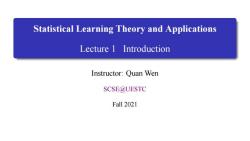
Statistical Learning Theory and Applications Lecture 1 Introduction Instructor:Quan Wen SCSE@UESTC Fall 2021
Statistical Learning Theory and Applications Lecture 1 Introduction Instructor: Quan Wen SCSE@UESTC Fall 2021
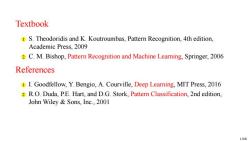
Textbook 1 S.Theodoridis and K.Koutroumbas,Pattern Recognition,4th edition, Academic Press.2009 2 C.M.Bishop,Pattern Recognition and Machine Learning,Springer,2006 References I.Goodfellow,Y.Bengio,A.Courville,Deep Learning,MIT Press,2016 2 R.O.Duda,P.E.Hart,and D.G.Stork,Pattern Classification,2nd edition, John Wiley Sons,Inc.,2001 1/68
Textbook 1 S. Theodoridis and K. Koutroumbas, Pattern Recognition, 4th edition, Academic Press, 2009 2 C. M. Bishop, Pattern Recognition and Machine Learning, Springer, 2006 References 1 I. Goodfellow, Y. Bengio, A. Courville, Deep Learning, MIT Press, 2016 2 R.O. Duda, P.E. Hart, and D.G. Stork, Pattern Classification, 2nd edition, John Wiley & Sons, Inc., 2001 1 / 68
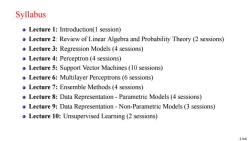
Syllabus Lecture 1:Introduction(1 session) Lecture 2:Review of Linear Algebra and Probability Theory(2 sessions) Lecture 3:Regression Models(4 sessions) Lecture 4:Perceptron(4 sessions) Lecture 5:Support Vector Machines(10 sessions) o Lecture 6:Multilayer Perceptrons(6 sessions) Lecture 7:Ensemble Methods(4 sessions) Lecture 8:Data Representation-Parametric Models(4 sessions) Lecture 9:Data Representation-Non-Parametric Models(3 sessions) o Lecture 10:Unsupervised Learning(2 sessions) 2/68
Syllabus Lecture 1: Introduction(1 session) Lecture 2: Review of Linear Algebra and Probability Theory (2 sessions) Lecture 3: Regression Models (4 sessions) Lecture 4: Perceptron (4 sessions) Lecture 5: Support Vector Machines (10 sessions) Lecture 6: Multilayer Perceptrons (6 sessions) Lecture 7: Ensemble Methods (4 sessions) Lecture 8: Data Representation - Parametric Models (4 sessions) Lecture 9: Data Representation - Non-Parametric Models (3 sessions) Lecture 10: Unsupervised Learning (2 sessions) 2 / 68
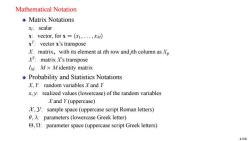
Mathematical Notation o Matrix Notations x:scalar x:vector,for x=(x1,...,xM) xT:vector x's transpose X:matrix,with its element at ith row and jth column asXi XT:matrix X's transpose Iy:Mx Midentity matrix Probability and Statistics Notations X,Y:random variables Y and Y x,y:realized values (lowercase)of the random variables Xand Y(uppercase) sample space(uppercase script Roman letters) 0,A:parameters(lowercase Greek letter) e,:parameter space(uppercase script Greek letters) 4/68
Mathematical Notation Matrix Notations xi : scalar x: vector, for x = (x1, . . . , xM) x T : vector x’s transpose X: matrix,with its element at ith row and jth column as Xij X T : matrix X’s transpose IM: M × M identity matrix Probability and Statistics Notations X, Y: random variables X and Y x, y: realized values (lowercase) of the random variables X and Y (uppercase) X , Y: sample space (uppercase script Roman letters) θ, λ: parameters (lowercase Greek letter) Θ, Ω: parameter space (uppercase script Greek letters) 4 / 68

Outline (Level 1) Definition of Statistical Learning 2 Application Case of Statistical Pattern Recognition 3 SPR System Framework and Design Cycle 4 Basic Concepts 5 Machine Learning in Different AI Disciplines 5/68
Outline (Level 1) 1 Definition of Statistical Learning 2 Application Case of Statistical Pattern Recognition 3 SPR System Framework and Design Cycle 4 Basic Concepts 5 Machine Learning in Different AI Disciplines 5 / 68
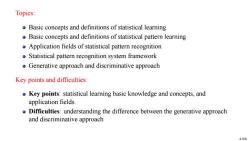
Topics: Basic concepts and definitions of statistical learning Basic concepts and definitions of statistical pattern learning Application fields of statistical pattern recognition Statistical pattern recognition system framework Generative approach and discriminative approach Key points and difficulties: o Key points:statistical learning basic knowledge and concepts,and application fields. oDifficulties:understanding the difference between the generative approach and discriminative approach 6/68
Topics: Basic concepts and definitions of statistical learning Basic concepts and definitions of statistical pattern learning Application fields of statistical pattern recognition Statistical pattern recognition system framework Generative approach and discriminative approach Key points and difficulties: Key points: statistical learning basic knowledge and concepts, and application fields. Difficulties: understanding the difference between the generative approach and discriminative approach 6 / 68
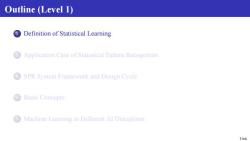
Outline (Level 1) Definition of Statistical Learning Application Case of Statistical Pattern Recognition SPR System Framework and Design Cycle Basic Concepts Machine Learning in Different Al Disciplines 7/68
Outline (Level 1) 1 Definition of Statistical Learning 2 Application Case of Statistical Pattern Recognition 3 SPR System Framework and Design Cycle 4 Basic Concepts 5 Machine Learning in Different AI Disciplines 7 / 68

1.Definition of Statistical Learning data Machine Hypothesis 8/68
1. Definition of Statistical Learning 8 / 68
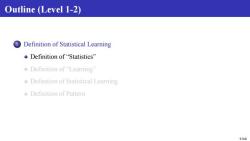
Outline (Level 1-2) Definition of Statistical Learning o Definition of"Statistics" o Definition of"Learning Definition of Statistical Learning o Definition of Pattern 9/68
Outline (Level 1-2) 1 Definition of Statistical Learning Definition of “Statistics” Definition of “Learning” Definition of Statistical Learning Definition of Pattern 9 / 68
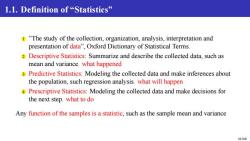
l.l.Definition of“Statistics'” 1"The study of the collection,organization,analysis,interpretation and presentation of data",Oxford Dictionary of Statistical Terms. 2 Descriptive Statistics:Summarize and describe the collected data,such as mean and variance.what happened 3 Predictive Statistics:Modeling the collected data and make inferences about the population,such regression analysis.what will happen 4 Prescriptive Statistics:Modeling the collected data and make decisions for the next step.what to do Any function of the samples is a statistic,such as the sample mean and variance 10/68
1.1. Definition of “Statistics” 1 ”The study of the collection, organization, analysis, interpretation and presentation of data”, Oxford Dictionary of Statistical Terms. 2 Descriptive Statistics: Summarize and describe the collected data, such as mean and variance. what happened 3 Predictive Statistics: Modeling the collected data and make inferences about the population, such regression analysis. what will happen 4 Prescriptive Statistics: Modeling the collected data and make decisions for the next step. what to do Any function of the samples is a statistic, such as the sample mean and variance 10 / 68
按次数下载不扣除下载券;
注册用户24小时内重复下载只扣除一次;
顺序:VIP每日次数-->可用次数-->下载券;
- 安顺学院:《经济统计学》专业新增学士学位授予权评审汇报PPT(吴永武).ppt
- 对外经济贸易大学:《应用统计 Applied Statistics》课程教学资源(教案讲稿).pdf
- 对外经济贸易大学:《应用统计 Applied Statistics》课程教学资源(教学大纲).pdf
- 上海交通大学:《统计原理 Principal of statistics》课程教学资源_大脑衰老与吃兴奋功能食品关系研究(调查问卷).doc
- 上海交通大学:《统计原理 Principal of statistics》课程教学资源_课后作业答案.doc
- 上海交通大学:《统计原理 Principal of statistics》课程教学资源_课后习题解答.doc
- 《统计原理 Principal of statistics》课程教学资源(统计软件教程)北京大学《统计软件SAS教程》(李东风).pdf
- 《统计原理 Principal of statistics》课程教学资源(统计软件教程)数据分析与EVIEWS应用(易丹辉).pdf
- 《统计原理 Principal of statistics》课程教学资源(统计软件教程)SPSS18.0教程(SPSS统计与分析).pdf
- 《统计原理 Principal of statistics》课程教学资源(统计软件教程)R语言实战(中文完整版).pdf
- 《统计原理 Principal of statistics》课程教学资源(统计软件教程)Matlab基础及其应用教程.pdf
- 《统计原理 Principal of statistics》课程教学资源(统计软件教程)MATLAB2013超强教程.pdf
- 《统计原理 Principal of statistics》课程教学资源(统计软件教程)Excel统计分析实例精讲.pdf
- 上海交通大学:《统计原理 Principal of statistics》课程教学资源_统计原理练习题(放大解答).pdf
- 上海交通大学:《统计原理 Principal of statistics》课程教学资源_统计原理练习题.pdf
- 上海交通大学:《统计原理 Principal of statistics》课程教学资源_统计原理练习题.doc
- 上海交通大学:《统计原理 Principal of statistics》课程教学资源_统计原理教材目录.pdf
- 上海交通大学:《统计原理 Principal of statistics》课程教学资源(上课讲义PPT)第五章 统计数据关系的分析.ppt
- 上海交通大学:《统计原理 Principal of statistics》课程教学资源(上课讲义PPT)第三章 统计数据特征的分析 §3.5 总体分布特征的估计.ppt
- 上海交通大学:《统计原理 Principal of statistics》课程教学资源(上课讲义PPT)第四章 统计数据变动的分析.ppt
- 电子科技大学:《统计学习理论及应用 Statistical Learning Theory and Applications》课程教学资源(课件讲稿,英文版)Lecture 02 Review of Linear Algebra and Probability Theory.pdf
- 电子科技大学:《统计学习理论及应用 Statistical Learning Theory and Applications》课程教学资源(课件讲稿,英文版)Lecture 03 Regression Models.pdf
- 电子科技大学:《统计学习理论及应用 Statistical Learning Theory and Applications》课程教学资源(课件讲稿,英文版)Lecture 04 Perceptron.pdf
- 电子科技大学:《统计学习理论及应用 Statistical Learning Theory and Applications》课程教学资源(课件讲稿,英文版)Lecture 05 Support Vector Machine.pdf
- 电子科技大学:《统计学习理论及应用 Statistical Learning Theory and Applications》课程教学资源(课件讲稿,英文版)Lecture 06 Multilayer Perceptron.pdf
- 电子科技大学:《统计学习理论及应用 Statistical Learning Theory and Applications》课程教学资源(课件讲稿,英文版)Lecture 07 Non-Linear Classification Model - Ensemble Methods.pdf
- 电子科技大学:《统计学习理论及应用 Statistical Learning Theory and Applications》课程教学资源(课件讲稿,英文版)Lecture 08 Data Representation - Parametric Model.pdf
- 电子科技大学:《统计学习理论及应用 Statistical Learning Theory and Applications》课程教学资源(课件讲稿,英文版)Lecture 09 Data Representation — Non-Parametric Model.pdf
- 电子科技大学:《统计学习理论及应用 Statistical Learning Theory and Applications》课程教学资源(课件讲稿,英文版)Lecture 10 Unsupervised Learning.pdf
- 电子科技大学:《统计学习理论及应用 Statistical Learning Theory and Applications》课程教学资源(课件讲稿)第一讲 概述(文泉、陈娟).pdf
- 电子科技大学:《统计学习理论及应用 Statistical Learning Theory and Applications》课程教学资源(课件讲稿)第二讲 概率与线性代数回顾.pdf
- 电子科技大学:《统计学习理论及应用 Statistical Learning Theory and Applications》课程教学资源(课件讲稿)第三讲 回归模型.pdf
- 电子科技大学:《统计学习理论及应用 Statistical Learning Theory and Applications》课程教学资源(课件讲稿)第四讲 感知机.pdf
- 电子科技大学:《统计学习理论及应用 Statistical Learning Theory and Applications》课程教学资源(课件讲稿)第五讲 支持向量机.pdf
- 电子科技大学:《统计学习理论及应用 Statistical Learning Theory and Applications》课程教学资源(课件讲稿)第六讲 非线性分类模型——多层感知机.pdf
- 电子科技大学:《统计学习理论及应用 Statistical Learning Theory and Applications》课程教学资源(课件讲稿)第七讲 非线性分类模型——集成方法.pdf
- 电子科技大学:《统计学习理论及应用 Statistical Learning Theory and Applications》课程教学资源(课件讲稿)第八讲 数据表示——含参模型.pdf
- 电子科技大学:《统计学习理论及应用 Statistical Learning Theory and Applications》课程教学资源(课件讲稿)第九讲 数据表示——不含参模型.pdf
- 电子科技大学:《统计学习理论及应用 Statistical Learning Theory and Applications》课程教学资源(课件讲稿)第十讲 非监督学习.pdf
- 中国人民大学:《应用随机过程 Applied Stochastic Processes》课程教学资源(课件讲稿)第10章 随机过程在保险精算中的应用.pdf