电子科技大学:《统计学习理论及应用 Statistical Learning Theory and Applications》课程教学资源(课件讲稿,英文版)Lecture 10 Unsupervised Learning
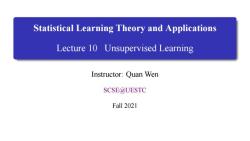
Statistical Learning Theory and Applications Lecture 10 Unsupervised Learning Instructor:Quan Wen SCSE@UESTC Fall 2021
Statistical Learning Theory and Applications Lecture 10 Unsupervised Learning Instructor: Quan Wen SCSE@UESTC Fall 2021

Outline (Level 1) DK-Means Clustering Algorithm ②PageRank 1/32
Outline (Level 1) 1 K-Means Clustering Algorithm 2 PageRank 1 / 32

Outline (Level 1) K-Means Clustering Algorithm PageRank 2/32
Outline (Level 1) 1 K-Means Clustering Algorithm 2 PageRank 2 / 32

1.K-Means Clustering Algorithm In the clustering problem,we are given a training set {x(),...x(,x()ER", and want to group the data into a few cohesive"clusters."No labels(are given. The k-means clustering algorithm is as follows: Initialize cluster centroidsE R",j=1,2,...,k,randomly. 2 Repeat until convergence: For each training samplex(),set c0=argmin|lr0-马l2 2 For every cluster centroid i,set ∑l(c0=r0 ∑1I(c0=) } I()is the indicator function. 3/32
1. K-Means Clustering Algorithm ▶ In the clustering problem, we are given a training set {x (1) , . . . , x (m)}, x (i) ∈ R n , and want to group the data into a few cohesive “clusters.” No labels y (i) are given. ▶ The k-means clustering algorithm is as follows: 1 Initialize cluster centroids µj ∈ R n , j = 1, 2, . . . , k, randomly. 2 Repeat until convergence: { 1 For each training sample x (i) , set c (i) = arg min j ∥x (i) − µj∥2 2 For every cluster centroid µj , set µj = Pm i=1 I(c (i) = j)x (i) Pm i=1 I(c (i) = j) } • I(·) is the indicator function. 3 / 32
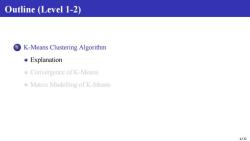
Outline (Level 1-2) K-Means Clustering Algorithm 。Explanation Convergence of K-Means o Matrix Modelling of K-Means 4/32
Outline (Level 1-2) 1 K-Means Clustering Algorithm Explanation Convergence of K-Means Matrix Modelling of K-Means 4 / 32

1.1.Explanation k(hyper-parameter of the algorithm)is the number of clusters we want to find Cluster centroids urepresent our current guesses for the positions of the centers of the clusters. To initialize the cluster centroids we could choose k training sample randomly, and set the cluster centroids to be equal to the values of these k examples. The inner-loop of the algorithm repeatedly carries out two steps: .Assigning each training samplex(to the closest cluster centroid Moving each cluster centroid to the mean of the samples assigned to it. 5/32
1.1. Explanation ▶ k (hyper-parameter of the algorithm) is the number of clusters we want to find ▶ Cluster centroids µj represent our current guesses for the positions of the centers of the clusters. To initialize the cluster centroids we could choose k training sample randomly, and set the cluster centroids to be equal to the values of these k examples. ▶ The inner-loop of the algorithm repeatedly carries out two steps: • Assigning each training sample x (i) to the closest cluster centroid µj . • Moving each cluster centroid µj to the mean of the samples assigned to it. 5 / 32

(a) (b) (c) (d) (e) () Training samples (dots)and cluster centroids(crosses).(a)Original dataset.(b)Random initial cluster centroids (isn't equal to training samples).(c-f)Illustration of running two iterations of 2-means. 6/32
▶ Training samples (dots) and cluster centroids (crosses). (a) Original dataset. (b) Random initial cluster centroids (isn’t equal to training samples). (c-f) Illustration of running two iterations of 2-means. 6 / 32

Outline (Level 1-2) DK-Means Clustering Algorithm Explanation Convergence of K-Means o Matrix Modelling of K-Means 7/32
Outline (Level 1-2) 1 K-Means Clustering Algorithm Explanation Convergence of K-Means Matrix Modelling of K-Means 7 / 32
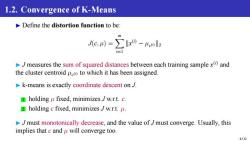
1.2.Convergence of K-Means Define the distortion function to be: m Jlc,)=∑lr0-4enl2 i=1 Jmeasures the sum of squared distances between each training samplex(and the cluster centroid u(o to which it has been assigned. k-means is exactly coordinate descent onJ. holding u fixed,minimizesJ w.r.t.c. 2 holding c fixed,minimizesJw.r.t.u. Jmust monotonically decrease,and the value ofJ must converge.Usually,this implies that c and u will converge too. 8/32
1.2. Convergence of K-Means ▶ Define the distortion function to be: J(c, µ) = Xm i=1 ∥x (i) − µc (i)∥2 ▶ J measures the sum of squared distances between each training sample x (i) and the cluster centroid µc (i) to which it has been assigned. ▶ k-means is exactly coordinate descent on J. 1 holding µ fixed, minimizes J w.r.t. c. 2 holding c fixed, minimizes J w.r.t. µ. ▶ J must monotonically decrease, and the value of J must converge. Usually, this implies that c and µ will converge too. 8 / 32

Local Minima of K-Means Distortion functionJ is a non-convex function,and so coordinate descent onJ is not guaranteed to converge to the global minimum. k-means can be susceptible to local optima. To avoid getting stuck in bad local minima,one common thing to do is run k-means many times(using different random initial values for the cluster centroids ui).Then,pick the one that gives the lowest distortion J(c,u). 9/32
Local Minima of K-Means ▶ Distortion function J is a non-convex function, and so coordinate descent on J is not guaranteed to converge to the global minimum. ▶ k-means can be susceptible to local optima. ▶ To avoid getting stuck in bad local minima, one common thing to do is run k-means many times (using different random initial values for the cluster centroids µj). Then, pick the one that gives the lowest distortion J(c, µ). 9 / 32
按次数下载不扣除下载券;
注册用户24小时内重复下载只扣除一次;
顺序:VIP每日次数-->可用次数-->下载券;
- 电子科技大学:《统计学习理论及应用 Statistical Learning Theory and Applications》课程教学资源(课件讲稿,英文版)Lecture 09 Data Representation — Non-Parametric Model.pdf
- 电子科技大学:《统计学习理论及应用 Statistical Learning Theory and Applications》课程教学资源(课件讲稿,英文版)Lecture 08 Data Representation - Parametric Model.pdf
- 电子科技大学:《统计学习理论及应用 Statistical Learning Theory and Applications》课程教学资源(课件讲稿,英文版)Lecture 07 Non-Linear Classification Model - Ensemble Methods.pdf
- 电子科技大学:《统计学习理论及应用 Statistical Learning Theory and Applications》课程教学资源(课件讲稿,英文版)Lecture 06 Multilayer Perceptron.pdf
- 电子科技大学:《统计学习理论及应用 Statistical Learning Theory and Applications》课程教学资源(课件讲稿,英文版)Lecture 05 Support Vector Machine.pdf
- 电子科技大学:《统计学习理论及应用 Statistical Learning Theory and Applications》课程教学资源(课件讲稿,英文版)Lecture 04 Perceptron.pdf
- 电子科技大学:《统计学习理论及应用 Statistical Learning Theory and Applications》课程教学资源(课件讲稿,英文版)Lecture 03 Regression Models.pdf
- 电子科技大学:《统计学习理论及应用 Statistical Learning Theory and Applications》课程教学资源(课件讲稿,英文版)Lecture 02 Review of Linear Algebra and Probability Theory.pdf
- 电子科技大学:《统计学习理论及应用 Statistical Learning Theory and Applications》课程教学资源(课件讲稿,英文版)Lecture 01 Introduction.pdf
- 安顺学院:《经济统计学》专业新增学士学位授予权评审汇报PPT(吴永武).ppt
- 对外经济贸易大学:《应用统计 Applied Statistics》课程教学资源(教案讲稿).pdf
- 对外经济贸易大学:《应用统计 Applied Statistics》课程教学资源(教学大纲).pdf
- 上海交通大学:《统计原理 Principal of statistics》课程教学资源_大脑衰老与吃兴奋功能食品关系研究(调查问卷).doc
- 上海交通大学:《统计原理 Principal of statistics》课程教学资源_课后作业答案.doc
- 上海交通大学:《统计原理 Principal of statistics》课程教学资源_课后习题解答.doc
- 《统计原理 Principal of statistics》课程教学资源(统计软件教程)北京大学《统计软件SAS教程》(李东风).pdf
- 《统计原理 Principal of statistics》课程教学资源(统计软件教程)数据分析与EVIEWS应用(易丹辉).pdf
- 《统计原理 Principal of statistics》课程教学资源(统计软件教程)SPSS18.0教程(SPSS统计与分析).pdf
- 《统计原理 Principal of statistics》课程教学资源(统计软件教程)R语言实战(中文完整版).pdf
- 《统计原理 Principal of statistics》课程教学资源(统计软件教程)Matlab基础及其应用教程.pdf
- 电子科技大学:《统计学习理论及应用 Statistical Learning Theory and Applications》课程教学资源(课件讲稿)第一讲 概述(文泉、陈娟).pdf
- 电子科技大学:《统计学习理论及应用 Statistical Learning Theory and Applications》课程教学资源(课件讲稿)第二讲 概率与线性代数回顾.pdf
- 电子科技大学:《统计学习理论及应用 Statistical Learning Theory and Applications》课程教学资源(课件讲稿)第三讲 回归模型.pdf
- 电子科技大学:《统计学习理论及应用 Statistical Learning Theory and Applications》课程教学资源(课件讲稿)第四讲 感知机.pdf
- 电子科技大学:《统计学习理论及应用 Statistical Learning Theory and Applications》课程教学资源(课件讲稿)第五讲 支持向量机.pdf
- 电子科技大学:《统计学习理论及应用 Statistical Learning Theory and Applications》课程教学资源(课件讲稿)第六讲 非线性分类模型——多层感知机.pdf
- 电子科技大学:《统计学习理论及应用 Statistical Learning Theory and Applications》课程教学资源(课件讲稿)第七讲 非线性分类模型——集成方法.pdf
- 电子科技大学:《统计学习理论及应用 Statistical Learning Theory and Applications》课程教学资源(课件讲稿)第八讲 数据表示——含参模型.pdf
- 电子科技大学:《统计学习理论及应用 Statistical Learning Theory and Applications》课程教学资源(课件讲稿)第九讲 数据表示——不含参模型.pdf
- 电子科技大学:《统计学习理论及应用 Statistical Learning Theory and Applications》课程教学资源(课件讲稿)第十讲 非监督学习.pdf
- 中国人民大学:《应用随机过程 Applied Stochastic Processes》课程教学资源(课件讲稿)第10章 随机过程在保险精算中的应用.pdf
- 中国人民大学:《应用随机过程 Applied Stochastic Processes》课程教学资源(课件讲稿)第11章 Markov链Monte Carlo方法.pdf
- 中国人民大学:《应用随机过程 Applied Stochastic Processes》课程教学资源(课件讲稿)第1章 预备知识(张波、商豪、邓军).pdf
- 中国人民大学:《应用随机过程 Applied Stochastic Processes》课程教学资源(课件讲稿)第2章 随机过程的基本概念和类型.pdf
- 中国人民大学:《应用随机过程 Applied Stochastic Processes》课程教学资源(课件讲稿)第3章 Poisson过程.pdf
- 中国人民大学:《应用随机过程 Applied Stochastic Processes》课程教学资源(课件讲稿)第4章 更新过程.pdf
- 中国人民大学:《应用随机过程 Applied Stochastic Processes》课程教学资源(课件讲稿)第5章 Markov链.pdf
- 中国人民大学:《应用随机过程 Applied Stochastic Processes》课程教学资源(课件讲稿)第6章 鞅.pdf
- 中国人民大学:《应用随机过程 Applied Stochastic Processes》课程教学资源(课件讲稿)第7章 Brown运动.pdf
- 中国人民大学:《应用随机过程 Applied Stochastic Processes》课程教学资源(课件讲稿)第8章 随机积分.pdf