电子科技大学:《统计学习理论及应用 Statistical Learning Theory and Applications》课程教学资源(课件讲稿,英文版)Lecture 06 Multilayer Perceptron
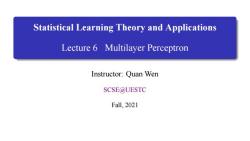
Statistical Learning Theory and Applications Lecture 6 Multilayer Perceptron Instructor:Quan Wen SCSE@UESTC Fal,2021
Statistical Learning Theory and Applications Lecture 6 Multilayer Perceptron Instructor: Quan Wen SCSE@UESTC Fall, 2021
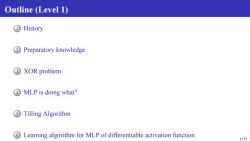
Outline (Level 1) ①History 2Preparatory knowledge ③XOR problem 4 MLP is doing what? ⑤Tilling Algorithm 6 Learning algorithm for MLP of differentiable activation function 1/77
Outline (Level 1) 1 History 2 Preparatory knowledge 3 XOR problem 4 MLP is doing what? 5 Tilling Algorithm 6 Learning algorithm for MLP of differentiable activation function 1 / 77
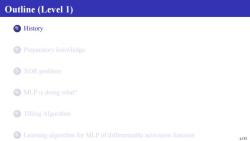
Outline (Level 1) ●History Preparatory knowledge XOR problem MLP is doing what? Tilling Algorithm Learning algorithm for MLP of differentiable activation function 2/77
Outline (Level 1) 1 History 2 Preparatory knowledge 3 XOR problem 4 MLP is doing what? 5 Tilling Algorithm 6 Learning algorithm for MLP of differentiable activation function 2 / 77
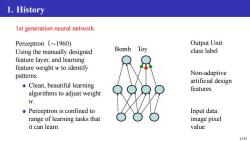
1.History Ist generation neural network Perceptron (~1960) Output Unit: Using the manually designed Bomb Toy class label feature layer,and learning feature weight w to identify patterns. Non-adaptive Clean,beautiful learning artificial design features algorithms to adjust weight W. o Perceptron is confined to Input data: range of learning tasks that image pixel it can learn. value 3/77
1. History 1st generation neural network Perceptron(∼1960) Using the manually designed feature layer, and learning feature weight w to identify patterns. Clean, beautiful learning algorithms to adjust weight w. Perceptron is confined to range of learning tasks that it can learn. Bomb Toy Output Unit: class label Non-adaptive artificial design features Input data: image pixel value 3 / 77
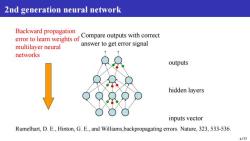
2nd generation neural network Backward propagation error to learn weights of Compare outputs with correct multilayer neural answer to get error signal networks outputs hidden layers inputs vector Rumelhart,D.E.,Hinton,G.E.,and Williams,backpropagating errors.Nature,323,533-536. 4/77
2nd generation neural network Backward propagation error to learn weights of multilayer neural networks Compare outputs with correct answer to get error signal outputs hidden layers inputs vector Rumelhart, D. E., Hinton, G. E., and Williams,backpropagating errors. Nature, 323, 533-536. 4 / 77
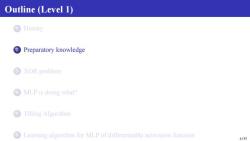
Outline (Level 1) History Preparatory knowledge XOR problem MLP is doing what? Tilling Algorithm Learning algorithm for MLP of differentiable activation function 5/77
Outline (Level 1) 1 History 2 Preparatory knowledge 3 XOR problem 4 MLP is doing what? 5 Tilling Algorithm 6 Learning algorithm for MLP of differentiable activation function 5 / 77

2.Preparatory knowledge The Simple Perceptron I 5 n=1 6/77
2. Preparatory knowledge The Simple Perceptron I o = fact( P 5 n=1 in · wn) 6 / 77
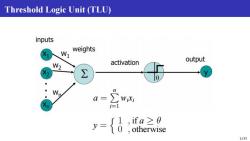
Threshold Logic Unit (TLU) inputs X1 N weights activation output W2 X ∑ o n a= WiXi y= {0 ,ifa≥0 otherwise 7/77
Threshold Logic Unit (TLU) a = P n i=1 wixi y = n 1 , if a ≥ θ 0 , otherwise 7 / 77
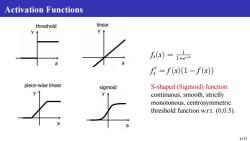
Activation Functions threshold linear )=+ =f(x)(1-f(x)) piece-wise linear sigmoid S-shaped(Sigmoid)function: continuous,smooth,strictly monotonous,centrosymmetric threshold function w.r.t.(0,0.5). 8/77
Activation Functions fs(x) = 1 1+e−x f ′ s = f (x)(1 − f (x)) S-shaped (Sigmoid) function: continuous, smooth, strictly monotonous, centrosymmetric threshold function w.r.t. (0,0.5). 8 / 77
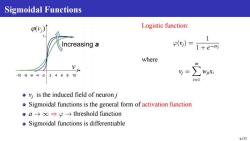
Sigmoidal Functions Logistic function: Increasing a p(y)=1+e-y where 108642246810 ∑ WjiXi i=1 v is the induced field of neuron j oSigmoidal functions is the general form of activation function ●a→o→p→threshold function Sigmoidal functions is differentiable 9177
Sigmoidal Functions Logistic function: φ(vj) = 1 1 + e −avj where vj = Xm i=1 wjixi vj is the induced field of neuron j Sigmoidal functions is the general form of activation function a → ∞ ⇒ φ → threshold function Sigmoidal functions is differentiable 9 / 77
按次数下载不扣除下载券;
注册用户24小时内重复下载只扣除一次;
顺序:VIP每日次数-->可用次数-->下载券;
- 电子科技大学:《统计学习理论及应用 Statistical Learning Theory and Applications》课程教学资源(课件讲稿,英文版)Lecture 05 Support Vector Machine.pdf
- 电子科技大学:《统计学习理论及应用 Statistical Learning Theory and Applications》课程教学资源(课件讲稿,英文版)Lecture 04 Perceptron.pdf
- 电子科技大学:《统计学习理论及应用 Statistical Learning Theory and Applications》课程教学资源(课件讲稿,英文版)Lecture 03 Regression Models.pdf
- 电子科技大学:《统计学习理论及应用 Statistical Learning Theory and Applications》课程教学资源(课件讲稿,英文版)Lecture 02 Review of Linear Algebra and Probability Theory.pdf
- 电子科技大学:《统计学习理论及应用 Statistical Learning Theory and Applications》课程教学资源(课件讲稿,英文版)Lecture 01 Introduction.pdf
- 安顺学院:《经济统计学》专业新增学士学位授予权评审汇报PPT(吴永武).ppt
- 对外经济贸易大学:《应用统计 Applied Statistics》课程教学资源(教案讲稿).pdf
- 对外经济贸易大学:《应用统计 Applied Statistics》课程教学资源(教学大纲).pdf
- 上海交通大学:《统计原理 Principal of statistics》课程教学资源_大脑衰老与吃兴奋功能食品关系研究(调查问卷).doc
- 上海交通大学:《统计原理 Principal of statistics》课程教学资源_课后作业答案.doc
- 上海交通大学:《统计原理 Principal of statistics》课程教学资源_课后习题解答.doc
- 《统计原理 Principal of statistics》课程教学资源(统计软件教程)北京大学《统计软件SAS教程》(李东风).pdf
- 《统计原理 Principal of statistics》课程教学资源(统计软件教程)数据分析与EVIEWS应用(易丹辉).pdf
- 《统计原理 Principal of statistics》课程教学资源(统计软件教程)SPSS18.0教程(SPSS统计与分析).pdf
- 《统计原理 Principal of statistics》课程教学资源(统计软件教程)R语言实战(中文完整版).pdf
- 《统计原理 Principal of statistics》课程教学资源(统计软件教程)Matlab基础及其应用教程.pdf
- 《统计原理 Principal of statistics》课程教学资源(统计软件教程)MATLAB2013超强教程.pdf
- 《统计原理 Principal of statistics》课程教学资源(统计软件教程)Excel统计分析实例精讲.pdf
- 上海交通大学:《统计原理 Principal of statistics》课程教学资源_统计原理练习题(放大解答).pdf
- 上海交通大学:《统计原理 Principal of statistics》课程教学资源_统计原理练习题.pdf
- 电子科技大学:《统计学习理论及应用 Statistical Learning Theory and Applications》课程教学资源(课件讲稿,英文版)Lecture 07 Non-Linear Classification Model - Ensemble Methods.pdf
- 电子科技大学:《统计学习理论及应用 Statistical Learning Theory and Applications》课程教学资源(课件讲稿,英文版)Lecture 08 Data Representation - Parametric Model.pdf
- 电子科技大学:《统计学习理论及应用 Statistical Learning Theory and Applications》课程教学资源(课件讲稿,英文版)Lecture 09 Data Representation — Non-Parametric Model.pdf
- 电子科技大学:《统计学习理论及应用 Statistical Learning Theory and Applications》课程教学资源(课件讲稿,英文版)Lecture 10 Unsupervised Learning.pdf
- 电子科技大学:《统计学习理论及应用 Statistical Learning Theory and Applications》课程教学资源(课件讲稿)第一讲 概述(文泉、陈娟).pdf
- 电子科技大学:《统计学习理论及应用 Statistical Learning Theory and Applications》课程教学资源(课件讲稿)第二讲 概率与线性代数回顾.pdf
- 电子科技大学:《统计学习理论及应用 Statistical Learning Theory and Applications》课程教学资源(课件讲稿)第三讲 回归模型.pdf
- 电子科技大学:《统计学习理论及应用 Statistical Learning Theory and Applications》课程教学资源(课件讲稿)第四讲 感知机.pdf
- 电子科技大学:《统计学习理论及应用 Statistical Learning Theory and Applications》课程教学资源(课件讲稿)第五讲 支持向量机.pdf
- 电子科技大学:《统计学习理论及应用 Statistical Learning Theory and Applications》课程教学资源(课件讲稿)第六讲 非线性分类模型——多层感知机.pdf
- 电子科技大学:《统计学习理论及应用 Statistical Learning Theory and Applications》课程教学资源(课件讲稿)第七讲 非线性分类模型——集成方法.pdf
- 电子科技大学:《统计学习理论及应用 Statistical Learning Theory and Applications》课程教学资源(课件讲稿)第八讲 数据表示——含参模型.pdf
- 电子科技大学:《统计学习理论及应用 Statistical Learning Theory and Applications》课程教学资源(课件讲稿)第九讲 数据表示——不含参模型.pdf
- 电子科技大学:《统计学习理论及应用 Statistical Learning Theory and Applications》课程教学资源(课件讲稿)第十讲 非监督学习.pdf
- 中国人民大学:《应用随机过程 Applied Stochastic Processes》课程教学资源(课件讲稿)第10章 随机过程在保险精算中的应用.pdf
- 中国人民大学:《应用随机过程 Applied Stochastic Processes》课程教学资源(课件讲稿)第11章 Markov链Monte Carlo方法.pdf
- 中国人民大学:《应用随机过程 Applied Stochastic Processes》课程教学资源(课件讲稿)第1章 预备知识(张波、商豪、邓军).pdf
- 中国人民大学:《应用随机过程 Applied Stochastic Processes》课程教学资源(课件讲稿)第2章 随机过程的基本概念和类型.pdf
- 中国人民大学:《应用随机过程 Applied Stochastic Processes》课程教学资源(课件讲稿)第3章 Poisson过程.pdf
- 中国人民大学:《应用随机过程 Applied Stochastic Processes》课程教学资源(课件讲稿)第4章 更新过程.pdf