中国科学院数学与系统科学研究院:《高级计量经济学》课程教学资源(课件讲稿)第三章 Classical Linear Regression Model
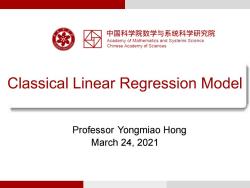
中国科学院数学与系统科学研究院 Academy of Mathematics and Systems Science Chinese Academy of Sciences Classical Linear Regression Model Professor Yongmiao Hong March 24.2021
Classical Linear Regression Model Professor Yongmiao Hong March 24, 2021

CONTENTS 3.1 Framework and Assumptions 3.2 Ordinary Least Squares (OLS)Estimation 3.3 Goodness of Fit and Model Selection Criteria 3.4 Consistency and Efficiency of OLS 3.5 Sampling Distribution of OLS 3.6 Variance Estimation for OLS 3.7 Hypothesis Testing ADVANCED ECONOMETRICS Classical Linear Regression Model May11,2021 2
ADVANCED ECONOMETRICS Classical Linear Regression Model May 11, 2021 2 3.1 Framework and Assumptions 3.2 Ordinary Least Squares (OLS) Estimation 3.3 Goodness of Fit and Model Selection Criteria 3.4 Consistency and Efficiency of OLS 3.5 Sampling Distribution of OLS 3.6 Variance Estimation for OLS 3.7 Hypothesis Testing CONTENTS
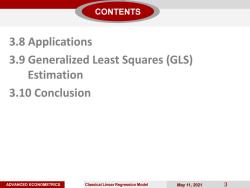
CONTENTS 3.8 Applications 3.9 Generalized Least Squares (GLS) Estimation 3.10 Conclusion ADVANCED ECONOMETRICS Classical Linear Regression Model May11,2021 3
ADVANCED ECONOMETRICS Classical Linear Regression Model May 11, 2021 3 3.8 Applications 3.9 Generalized Least Squares (GLS) Estimation 3.10 Conclusion CONTENTS
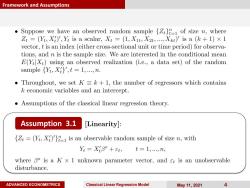
Framework and Assumptions Suppose we have an observed random sample {Z1 of size n,where Zt =(Yt;X)',Yt is a scalar,Xt =(1,Xit;X2t,..Xit)'is a (k+1)x 1 vector,t is an index(either cross-sectional unit or time period)for observa- tions,and n is the sample size.We are interested in the conditional mean E(YiXt)using an observed realization (i.e.,a data set)of the random sample (rt,xi',t =1,....n. Throughout,we set K=k+1,the number of regressors which contains k economic variables and an intercept. Assumptions of the classical linear regression theory. Assumption 3.1 [Linearity]: Z=(Yi,X)')1 is an observable random sample of size n,with Y=XtB°+et, t=1,,n, where Bo is a k x 1 unknown parameter vector,and et is an unobservable disturbance. ADVANCED ECONOMETRICS Classical Linear Regression Model May11,2021 4
ADVANCED ECONOMETRICS Classical Linear Regression Model May 11, 2021 4 Framework and Assumptions Assumption 3.1

Framework and Assumptions Questions: Does Assumption 3.1 imply a causal relationship from Xt to Yi? Not necessarily.As Kendall and Stuart (1961,Vol.2,Ch.26,p.279)point out,"a statistical relationship,however strong and however suggestive, can never establish causal connection.Our ideas of causation must come from outside statistics ultimately,from some theory or other." Denote Y= (Yi,,Yn)', n×1, E= (e1,,en)/, n×1, X=(X1,,Xm), n×K. where the t-th row of X isX=(1,Xit,...,Xit). With these matrix notations,we have a compact expression for Assumption 3.1: Y= XB°+e, n×1=(n×K)(K×1)+n×1. ADVANCED ECONOMETRICS Classical Linear Regression Model May11,2021 5
ADVANCED ECONOMETRICS Classical Linear Regression Model May 11, 2021 5 Framework and Assumptions Questions:
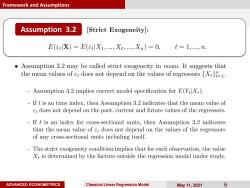
Framework and Assumptions Assumption 3.2 [Strict Exogeneity]: E(et X)=E(et X1;...Xi;...,Xn)=0, t=1,,n. Assumption 3.2 may be called strict exogeneity in mean.It suggests that the mean values of et does not depend on the values of regressors {X1. Assumption 3.2 implies correct model specification for E(YXt). If t is an time index,then Assumption 3.2 indicates that the mean value of et does not depend on the past,current and future values of the regressors. If t is an index for cross-sectional units,then Assumption 3.2 indicates that the mean value of et does not depend on the values of the regressors of any cross-sectional units including itself. The strict exogeneity condition implies that for each observation,the value Xt is determined by the factors outside the regression model under study. ADVANCED ECONOMETRICS Classical Linear Regression Model May11,2021 6
ADVANCED ECONOMETRICS Classical Linear Regression Model May 11, 2021 6 Framework and Assumptions Assumption 3.2

Framework and Assumptions Assumption 3.2 [Strict Exogeneity]: E(et X)=E(et X1,...,Xt;..,Xn)=0,t=1,...,n. - Under Assumption 3.2,we have E(Xset)=0 for any (t,s),where t,s E 1,...,n.This follows because E(XsEt) =EE(Xset X)] EXgE(et X E(Xs·0) 三 0. Given E(et)=0,E(Xset)=0 implies cov(Xs,Et)=0 for all t,s E {1,,n Because X contains regressors {Xs}for both s t,Assumption 3.2 essentially requires that the error et do not depend on both the past and future values of regressors if t is a time index.This rules out dynamic time series models for which et may be correlated with the future values of regressors. ADVANCED ECONOMETRICS Classical Linear Regression Model May11,2021 7
ADVANCED ECONOMETRICS Classical Linear Regression Model May 11, 2021 7 Framework and Assumptions Assumption 3.2
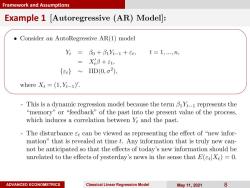
Framework and Assumptions Example 1 [Autoregressive (AR)Model]: .Consider an AutoRegressive AR(1)model Yi Bo+B1Yi-1+6t, t=1,,n, XB+Et, {et}~IID(0,o2), where Xt=(1,Yi-1)'. This is a dynamic regression model because the term BiY-1 represents the “memory”or“feedback'”of the past into the present value of the process, which induces a correlation between Yt and the past. The disturbance et can be viewed as representing the effect of "new infor- mation"that is revealed at time t.Any information that is truly new can- not be anticipated so that the effects of today's new information should be unrelated to the effects of yesterday's news in the sense that E(tXt)=0. ADVANCED ECONOMETRICS Classical Linear Regression Model May11,2021 8
ADVANCED ECONOMETRICS Classical Linear Regression Model May 11, 2021 8 Framework and Assumptions Example 1
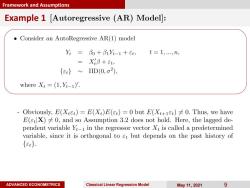
Framework and Assumptions Example 1 [Autoregressive (AR)Model]: .Consider an AutoRegressive AR(1)model Yt Bo+B1Yi-1+Et, t=1,,n, XB+Et, {et}~IID(0,o2), where Xt =(1,Yi-1). Obviously,E(Xiet)=E(X:)E(et)=0 but E(Xt+1et)0.Thus,we have E(etX)0,and so Assumption 3.2 does not hold.Here,the lagged de- pendent variable Yi-1 in the regressor vector X:is called a predetermined variable,since it is orthogonal to st but depends on the past history of {et}. ADVANCED ECONOMETRICS Classical Linear Regression Model May11,2021 9
ADVANCED ECONOMETRICS Classical Linear Regression Model May 11, 2021 9 Framework and Assumptions Example 1
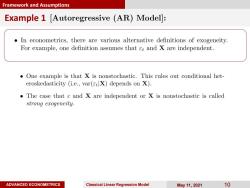
Framework and Assumptions Example 1 [Autoregressive (AR)Model]: In econometrics,there are various alternative definitions of exogeneity. For example,one definition assumes that st and X are independent. One example is that X is nonstochastic.This rules out conditional het- eroskedasticity (i.e.,var(et X)depends on X). The case that s and X are independent or X is nonstochastic is called strong exogeneity. ADVANCED ECONOMETRICS Classical Linear Regression Model May11,2021 10
ADVANCED ECONOMETRICS Classical Linear Regression Model May 11, 2021 10 Framework and Assumptions Example 1
按次数下载不扣除下载券;
注册用户24小时内重复下载只扣除一次;
顺序:VIP每日次数-->可用次数-->下载券;
- 中国科学院数学与系统科学研究院:《高级计量经济学》课程教学资源(课件讲稿)第二章 General Regression Analysis.pdf
- 中国科学院数学与系统科学研究院:《高级计量经济学》课程教学资源(课件讲稿)第一章 Introduction to Econometrics.pdf
- 厦门大学:《高级计量经济学》课程教学资源(课件讲稿)Introduction to Statistics and Econometrics.pdf
- 厦门大学:《高级计量经济学》课程教学资源(教学大纲)A Course on Advanced Econometrics(主讲:洪永淼).pdf
- 运城学院:《宏观经济学》课程教学资源(电子教案,打印版,负责人:李吉续).pdf
- 运城学院:《宏观经济学》课程教学资源(各章习题,含答案,打印版)第五章 国际经济的基本知识.pdf
- 运城学院:《宏观经济学》课程教学资源(各章习题,含答案,打印版)第四章 失业与通货膨胀.pdf
- 运城学院:《宏观经济学》课程教学资源(各章习题,含答案,打印版)第三章 凯恩斯的宏观经济政策主张.pdf
- 运城学院:《宏观经济学》课程教学资源(各章习题,含答案,打印版)第二章 凯恩斯的均衡国民收入理论.pdf
- 运城学院:《宏观经济学》课程教学资源(各章习题,含答案,打印版)第一章 福利经济学和微观经济政策.pdf
- 华东理工大学:《商业银行经营学》课程教学资源(PPT课件讲稿)第十四章 商业银行经营发展趋势.ppt
- 华东理工大学:《商业银行经营学》课程教学资源(PPT课件讲稿)第十三章 商业银行经营风险与内部控制.ppt
- 华东理工大学:《商业银行经营学》课程教学资源(PPT课件讲稿)第十二章 商业银行绩效评估.ppt
- 华东理工大学:《商业银行经营学》课程教学资源(PPT课件讲稿)第十章 国际业务.ppt
- 华东理工大学:《商业银行经营学》课程教学资源(PPT课件讲稿)第十一章 商业银行资产负债管理策略.ppt
- 华东理工大学:《商业银行经营学》课程教学资源(PPT课件讲稿)第九章 其他业务.ppt
- 华东理工大学:《商业银行经营学》课程教学资源(PPT课件讲稿)第八章 表外业务.ppt
- 华东理工大学:《商业银行经营学》课程教学资源(PPT课件讲稿)第七章 租赁与信托.ppt
- 华东理工大学:《商业银行经营学》课程教学资源(PPT课件讲稿)第六章 银行证券投资业务.ppt
- 华东理工大学:《商业银行经营学》课程教学资源(PPT课件讲稿)第四章 现金资产业务.ppt
- 中国科学院数学与系统科学研究院:《高级计量经济学》课程教学资源(课件讲稿)第四章 Professor Yongmiao Hong.pdf
- 厦门大学:《概率论与数理统计 Probability and Statistics for Economists》课程教学资源(教学大纲,主讲:洪永淼).pdf
- 厦门大学:《概率论与数理统计 Probability and Statistics for Economists》课程教学资源(课件讲稿)Chapter 02 Foundation of Probability Theory.pdf
- 厦门大学:《概率论与数理统计 Probability and Statistics for Economists》课程教学资源(课件讲稿)Chapter 03 Random Variables and Univariate Probability Distributions.pdf
- 厦门大学:《概率论与数理统计 Probability and Statistics for Economists》课程教学资源(课件讲稿)Chapter 10 Big Data, Machine Learning and Statistics.pdf
- 厦门大学:《概率论与数理统计 Probability and Statistics for Economists》课程教学资源(课件讲稿)Chapter 05 Multivariate Probability Distributions.pdf
- 厦门大学:《概率论与数理统计 Probability and Statistics for Economists》课程教学资源(课件讲稿)Chapter 06 Multivariate Probability Distributions.pdf
- 厦门大学:《概率论与数理统计 Probability and Statistics for Economists》课程教学资源(课件讲稿)Chapter 07 Convergences and Limit Theorems.pdf
- 厦门大学:《概率论与数理统计 Probability and Statistics for Economists》课程教学资源(课件讲稿)Chapter 08 Parameter Estimation and Evaluation.pdf
- 厦门大学:《概率论与数理统计 Probability and Statistics for Economists》课程教学资源(课件讲稿)Chapter 09 Hypothesis Testing.pdf
- 厦门大学:《概率论与数理统计 Probability and Statistics for Economists》课程教学资源(课件讲稿)Chapter 01 Introduction to Statistics and Econometrics.pdf
- 厦门大学:《概率论与数理统计 Probability and Statistics for Economists》课程教学资源(课件讲稿)Chapter 04 Important Probability Distributions.pdf
- 厦门大学:《社会主义政治经济学 Socialist Political Economics》课程教学资源(PPT课件讲稿)导论(主讲:洪永淼).pptx
- 厦门大学:《社会主义政治经济学 Socialist Political Economics》课程教学资源(PPT课件讲稿)第二章 资本主义经济发展规律.pptx
- 厦门大学:《社会主义政治经济学 Socialist Political Economics》课程教学资源(PPT课件讲稿)第二章 附录——商品生产基本概念.pptx
- 厦门大学:《社会主义政治经济学 Socialist Political Economics》课程教学资源(PPT课件讲稿)马克思恩格斯社会主义思想的理论来源(主讲:侯金光).pdf
- 厦门大学:《社会主义政治经济学 Socialist Political Economics》课程教学资源(PPT课件讲稿)三十年代苏联党内斗争和大镇压(主讲:侯金光).pptx
- 厦门大学:《社会主义政治经济学 Socialist Political Economics》课程教学资源(PPT课件讲稿)彼得堡大学的经济学家们.pptx
- 厦门大学:《社会主义政治经济学 Socialist Political Economics》课程教学资源(PPT课件讲稿)第八章 西方学者关于社会主义的论争.pptx
- 厦门大学:《社会主义政治经济学 Socialist Political Economics》课程教学资源(PPT课件讲稿)第九章 新民主主义经济与社会主义改造(主讲:张兴祥).pptx