厦门大学:《概率论与数理统计 Probability and Statistics for Economists》课程教学资源(课件讲稿)Chapter 10 Big Data, Machine Learning and Statistics

分海總南亞王季大门原章 Big Data,Machine Learning and Statistics Professor Yongmiao Hong Cornell University July8,2020
Big Data, Machine Learning and Statistics Professor Yongmiao Hong Cornell University July 8, 2020
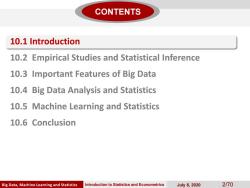
CONTENTS 10.1 Introduction 10.2 Empirical Studies and Statistical Inference 10.3 Important Features of Big Data 10.4 Big Data Analysis and Statistics 10.5 Machine Learning and Statistics 10.6 Conclusion Big Data,Machine Learning and Statistics Introduction to Statistics and Econometrics July8,2020 2170
Big Data, Machine Learning and Statistics Introduction to Statistics and Econometrics July 8, 2020 2/70 10.1 Introduction 10.2 Empirical Studies and Statistical Inference 10.3 Important Features of Big Data 10.4 Big Data Analysis and Statistics 10.5 Machine Learning and Statistics 10.6 Conclusion CONTENTS

Parameter Estimation and Evaluation Introduction Introduction With the rapid development of internet and mobil in- ternet techologies as well as their applications,the rise of Big data together with machine learning,a main computer-based automatic analytic tool for Big data,has profound implications on statistical sciences. Compared with traditional historical data,Big data of- ten has an extraordinarily large volume of data,with structured,semi-structruraled and unstructured formats, which are often produced in real-time or near real-time. Big Data,Machine Learning and Statistics Introduction to Statistics and Econometrics Juy8,2020 3/70
Parameter Estimation and Evaluation Big Data, Machine Learning and Statistics Introduction to Statistics and Econometrics July 8, 2020 3/70 Introduction Introduction

Parameter Estimation and Evaluation Introduction Introduction What is Big data? Has Big data altered the foundation of statistical sciences,such as sampling inference for population,causal analysis,sufficiency principle,data reduction, prediction,and etc? What challenges and opportunities does Big data bring to the theory and practice of statistical modelling and inference? What is machine learning? What are the key differences between machine learning and statistical mod- elling? What is the relationship between machine learning and statistical inference? As is well-known,machine learning often has accurate out-of-sample pre- dictions,but it looks like a black box.Can statistics provide meaningful interpretations for machine learning methods? Can machine learning and statistics be synthesized together,and if so,how this will affect the future development of statistical sciences? Big Data,Machine Learning and Statistics Introduction to Statistics and Econometrics July8,2020 4170
Parameter Estimation and Evaluation Big Data, Machine Learning and Statistics Introduction to Statistics and Econometrics July 8, 2020 4/70 Introduction Introduction
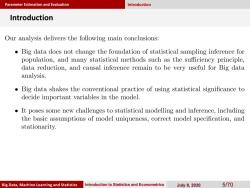
Parameter Estimation and Evaluation Introduction Introduction Our analysis delivers the following main conclusions: Big data does not change the foundation of statistical sampling inference for population,and many statistical methods such as the sufficiency principle, data reduction,and causal inference remain to be very useful for Big data analysis. Big data shakes the conventional practice of using statistical significance to decide important variables in the model. It poses some new challenges to statistical modelling and inference,including the basic assumptions of model uniqueness,correct model specification,and stationarity. Big Data,Machine Learning and Statistics Introduction to Statistics and Econometrics Juy8,2020 5/70
Parameter Estimation and Evaluation Big Data, Machine Learning and Statistics Introduction to Statistics and Econometrics July 8, 2020 5/70 Introduction Introduction

Parameter Estimation and Evaluation Introduction Introduction Machine learning,which arises due to availability of Big data,shares some common grounds as statistical inference,particularly in terms of sampling inference for population.Like any statistical inference methods,machine learning may suffer from sample bias. As an algorithm-based approach,Machine learning is much more general and flexible than statistical parametric modelling,including the determination of the set of important explanatory variables. ● Statistical nonparametric modelling can provide meaningful interpretations for some important machine learning algorithms,such as decision trees and artificial neural networks. The synthesis of machine learning and statistical inference is expected to open several new directions for statistical sciences. Big Data,Machine Learning and Statistics Introduction to Statistics and Econometrics July8,2020 6/70
Parameter Estimation and Evaluation Big Data, Machine Learning and Statistics Introduction to Statistics and Econometrics July 8, 2020 6/70 Introduction Introduction

CONTENTS 10.1 Introduction 10.2 Empirical Studies and Statistical Inference 10.3 Important Features of Big Data 10.4 Big Data Analysis and Statistics 10.5 Machine Learning and Statistics 10.6 Conclusion Big Data,Machine Learning and Statistics Introduction to Statistics and Econometrics July8,2020 7170
Big Data, Machine Learning and Statistics Introduction to Statistics and Econometrics July 8, 2020 7/70 10.1 Introduction 10.2 Empirical Studies and Statistical Inference 10.3 Important Features of Big Data 10.4 Big Data Analysis and Statistics 10.5 Machine Learning and Statistics 10.6 Conclusion CONTENTS
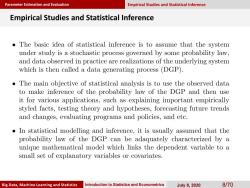
Parameter Estimation and Evaluation Empirical Studies and Statistical Inference Empirical Studies and Statistical Inference The basic idea of statistical inference is to assume that the system under study is a stochastic process governed by some probability law, and data observed in practice are realizations of the underlying system which is then called a data generating process(DGP). The main objective of statistical analysis is to use the observed data to make inference of the probability law of the DGP and then use it for various applications,such as explaining important empirically styled facts,testing theory and hypotheses,forecasting future trends and changes,evaluating programs and policies,and etc. In statistical modelling and inference,it is usually assumed that the probability law of the DGP can be adaquately characterized by a unique mathematical model which links the dependent variable to a small set of explanatory variables or covariates. Big Data,Machine Learning and Statistics Introduction to Statistics and Econometrics July8,2020 8/70
Parameter Estimation and Evaluation Big Data, Machine Learning and Statistics Introduction to Statistics and Econometrics July 8, 2020 8/70 Empirical Studies and Statistical Inference Empirical Studies and Statistical Inference
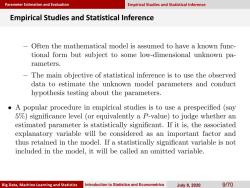
Parameter Estimation and Evaluation Empirical Studies and Statistical Inference Empirical Studies and Statistical Inference -Often the mathematical model is assumed to have a known func- tional form but subject to some low-dimensional unknown pa- rameters. The main objective of statistical inference is to use the observed data to estimate the unknown model parameters and conduct hypothesis testing about the parameters. .A popular procedure in empirical studies is to use a prespecified (say 5%)significance level (or equivalently a P-value)to judge whether an estimated parameter is statistically significant.If it is,the associated explanatory variable will be considered as an important factor and thus retained in the model.If a statistically significant variable is not included in the model,it will be called an omitted variable. Big Data,Machine Learning and Statistics Introduction to Statistics and Econometrics July8,2020 9/70
Parameter Estimation and Evaluation Big Data, Machine Learning and Statistics Introduction to Statistics and Econometrics July 8, 2020 9/70 Empirical Studies and Statistical Inference Empirical Studies and Statistical Inference
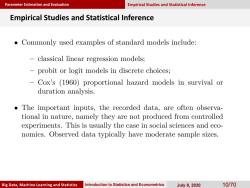
Parameter Estimation and Evaluation Empirical Studies and Statistical Inference Empirical Studies and Statistical Inference Commonly used examples of standard models include: -classical linear regression models; -probit or logit models in discrete choices; Cox's (1960)proportional hazard models in survival or duration analysis. The important inputs,the recorded data,are often observa- tional in nature,namely they are not produced from controlled experiments.This is usually the case in social sciences and eco- nomics.Observed data typically have moderate sample sizes. Big Data,Machine Learning and Statistics Introduction to Statistics and Econometrics Juy8,2020 10/70
Parameter Estimation and Evaluation Big Data, Machine Learning and Statistics Introduction to Statistics and Econometrics July 8, 2020 10/70 Empirical Studies and Statistical Inference Empirical Studies and Statistical Inference
按次数下载不扣除下载券;
注册用户24小时内重复下载只扣除一次;
顺序:VIP每日次数-->可用次数-->下载券;
- 厦门大学:《概率论与数理统计 Probability and Statistics for Economists》课程教学资源(课件讲稿)Chapter 03 Random Variables and Univariate Probability Distributions.pdf
- 厦门大学:《概率论与数理统计 Probability and Statistics for Economists》课程教学资源(课件讲稿)Chapter 02 Foundation of Probability Theory.pdf
- 厦门大学:《概率论与数理统计 Probability and Statistics for Economists》课程教学资源(教学大纲,主讲:洪永淼).pdf
- 中国科学院数学与系统科学研究院:《高级计量经济学》课程教学资源(课件讲稿)第四章 Professor Yongmiao Hong.pdf
- 中国科学院数学与系统科学研究院:《高级计量经济学》课程教学资源(课件讲稿)第三章 Classical Linear Regression Model.pdf
- 中国科学院数学与系统科学研究院:《高级计量经济学》课程教学资源(课件讲稿)第二章 General Regression Analysis.pdf
- 中国科学院数学与系统科学研究院:《高级计量经济学》课程教学资源(课件讲稿)第一章 Introduction to Econometrics.pdf
- 厦门大学:《高级计量经济学》课程教学资源(课件讲稿)Introduction to Statistics and Econometrics.pdf
- 厦门大学:《高级计量经济学》课程教学资源(教学大纲)A Course on Advanced Econometrics(主讲:洪永淼).pdf
- 运城学院:《宏观经济学》课程教学资源(电子教案,打印版,负责人:李吉续).pdf
- 运城学院:《宏观经济学》课程教学资源(各章习题,含答案,打印版)第五章 国际经济的基本知识.pdf
- 运城学院:《宏观经济学》课程教学资源(各章习题,含答案,打印版)第四章 失业与通货膨胀.pdf
- 运城学院:《宏观经济学》课程教学资源(各章习题,含答案,打印版)第三章 凯恩斯的宏观经济政策主张.pdf
- 运城学院:《宏观经济学》课程教学资源(各章习题,含答案,打印版)第二章 凯恩斯的均衡国民收入理论.pdf
- 运城学院:《宏观经济学》课程教学资源(各章习题,含答案,打印版)第一章 福利经济学和微观经济政策.pdf
- 华东理工大学:《商业银行经营学》课程教学资源(PPT课件讲稿)第十四章 商业银行经营发展趋势.ppt
- 华东理工大学:《商业银行经营学》课程教学资源(PPT课件讲稿)第十三章 商业银行经营风险与内部控制.ppt
- 华东理工大学:《商业银行经营学》课程教学资源(PPT课件讲稿)第十二章 商业银行绩效评估.ppt
- 华东理工大学:《商业银行经营学》课程教学资源(PPT课件讲稿)第十章 国际业务.ppt
- 华东理工大学:《商业银行经营学》课程教学资源(PPT课件讲稿)第十一章 商业银行资产负债管理策略.ppt
- 厦门大学:《概率论与数理统计 Probability and Statistics for Economists》课程教学资源(课件讲稿)Chapter 05 Multivariate Probability Distributions.pdf
- 厦门大学:《概率论与数理统计 Probability and Statistics for Economists》课程教学资源(课件讲稿)Chapter 06 Multivariate Probability Distributions.pdf
- 厦门大学:《概率论与数理统计 Probability and Statistics for Economists》课程教学资源(课件讲稿)Chapter 07 Convergences and Limit Theorems.pdf
- 厦门大学:《概率论与数理统计 Probability and Statistics for Economists》课程教学资源(课件讲稿)Chapter 08 Parameter Estimation and Evaluation.pdf
- 厦门大学:《概率论与数理统计 Probability and Statistics for Economists》课程教学资源(课件讲稿)Chapter 09 Hypothesis Testing.pdf
- 厦门大学:《概率论与数理统计 Probability and Statistics for Economists》课程教学资源(课件讲稿)Chapter 01 Introduction to Statistics and Econometrics.pdf
- 厦门大学:《概率论与数理统计 Probability and Statistics for Economists》课程教学资源(课件讲稿)Chapter 04 Important Probability Distributions.pdf
- 厦门大学:《社会主义政治经济学 Socialist Political Economics》课程教学资源(PPT课件讲稿)导论(主讲:洪永淼).pptx
- 厦门大学:《社会主义政治经济学 Socialist Political Economics》课程教学资源(PPT课件讲稿)第二章 资本主义经济发展规律.pptx
- 厦门大学:《社会主义政治经济学 Socialist Political Economics》课程教学资源(PPT课件讲稿)第二章 附录——商品生产基本概念.pptx
- 厦门大学:《社会主义政治经济学 Socialist Political Economics》课程教学资源(PPT课件讲稿)马克思恩格斯社会主义思想的理论来源(主讲:侯金光).pdf
- 厦门大学:《社会主义政治经济学 Socialist Political Economics》课程教学资源(PPT课件讲稿)三十年代苏联党内斗争和大镇压(主讲:侯金光).pptx
- 厦门大学:《社会主义政治经济学 Socialist Political Economics》课程教学资源(PPT课件讲稿)彼得堡大学的经济学家们.pptx
- 厦门大学:《社会主义政治经济学 Socialist Political Economics》课程教学资源(PPT课件讲稿)第八章 西方学者关于社会主义的论争.pptx
- 厦门大学:《社会主义政治经济学 Socialist Political Economics》课程教学资源(PPT课件讲稿)第九章 新民主主义经济与社会主义改造(主讲:张兴祥).pptx
- 厦门大学:《社会主义政治经济学 Socialist Political Economics》课程教学资源(PPT课件讲稿)第十一章 从有计划的商品经济到市场经济.pptx
- 厦门大学:《社会主义政治经济学 Socialist Political Economics》课程教学资源(PPT课件讲稿)第十章 中国计划经济模式.pptx
- 厦门大学:《社会主义政治经济学 Socialist Political Economics》课程教学资源(PPT课件讲稿)第十三章 中国模式特征与发展趋势.pptx
- 国家十一五规划教材:《货币经济学》课程教学资源(讲义,货币银行学)目录(经济科学出版社,主编:姜旭朝、胡金焱,副主编:孔丹凤).doc
- 国家十一五规划教材:《货币经济学》课程教学资源(讲义,货币银行学)第一章 货币基本理论.doc