香港中文大学:《Probability and Statistics for Engineers》课程教学资源(课件讲稿)Bayesian Statistical Inference
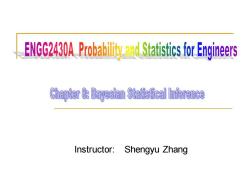
ENGG430Protaistics onnrs Chapter 8:Bayesian Statistical Inference Instructor: Shengyu Zhang
Instructor: Shengyu Zhang
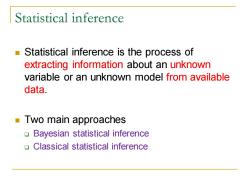
Statistical inference Statistical inference is the process of extracting information about an unknown variable or an unknown model from available data. Two main approaches o Bayesian statistical inference Classical statistical inference
Statistical inference ◼ Statistical inference is the process of extracting information about an unknown variable or an unknown model from available data. ◼ Two main approaches ❑ Bayesian statistical inference ❑ Classical statistical inference
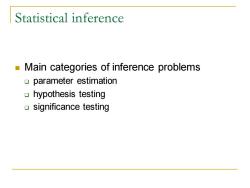
Statistical inference Main categories of inference problems oparameter estimation hypothesis testing significance testing
Statistical inference ◼ Main categories of inference problems ❑ parameter estimation ❑ hypothesis testing ❑ significance testing

Statistical inference Most important methodologies o I maximum a posteriori(MAP) probability rule, least mean squares estimation, maximum likelihood. ▣regression, likelihood ratio tests
Statistical inference ◼ Most important methodologies ❑ maximum a posteriori (MAP) ❑ probability rule, ❑ least mean squares estimation, ❑ maximum likelihood, ❑ regression, ❑ likelihood ratio tests
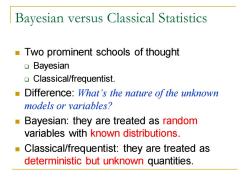
Bayesian versus Classical Statistics Two prominent schools of thought Bayesian Classical/frequentist. Difference:What's the nature of the unknown models or variables? Bayesian:they are treated as random variables with known distributions. Classical/frequentist:they are treated as deterministic but unknown quantities
Bayesian versus Classical Statistics ◼ Two prominent schools of thought ❑ Bayesian ❑ Classical/frequentist. ◼ Difference: What’s the nature of the unknown models or variables? ◼ Bayesian: they are treated as random variables with known distributions. ◼ Classical/frequentist: they are treated as deterministic but unknown quantities
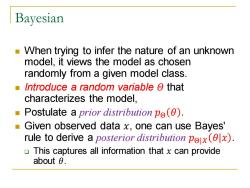
Bayesian When trying to infer the nature of an unknown model,it views the model as chosen randomly from a given model class. Introduce a random variable o that characterizes the model, Postulate a prior distribution pe(). Given observed data x,one can use Bayes' rule to derive a posterior distribution px(x). This captures all information that x can provide about 0
Bayesian ◼ When trying to infer the nature of an unknown model, it views the model as chosen randomly from a given model class. ◼ Introduce a random variable 𝛩 that characterizes the model, ◼ Postulate a prior distribution 𝑝Θ 𝜃 . ◼ Given observed data 𝑥, one can use Bayes' rule to derive a posterior distribution 𝑝Θ|𝑋 𝜃|𝑥 . ❑ This captures all information that 𝑥 can provide about 𝜃

Classical/frequentist View the unknown quantity 0 as an unknown constant. Strives to develop an estimate of 0. We are dealing with multiple candidate probabilistic models,one for each possible value of 0
Classical/frequentist ◼ View the unknown quantity 𝜃 as an unknown constant. ◼ Strives to develop an estimate of 𝜃. ◼ We are dealing with multiple candidate probabilistic models, one for each possible value of 𝜃
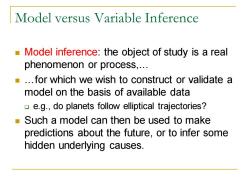
Model versus Variable Inference Model inference:the object of study is a real phenomenon or process,... ..for which we wish to construct or validate a model on the basis of available data e.g.,do planets follow elliptical trajectories? ■、 Such a model can then be used to make predictions about the future,or to infer some hidden underlying causes
Model versus Variable Inference ◼ Model inference: the object of study is a real phenomenon or process,… ◼ …for which we wish to construct or validate a model on the basis of available data ❑ e.g., do planets follow elliptical trajectories? ◼ Such a model can then be used to make predictions about the future, or to infer some hidden underlying causes
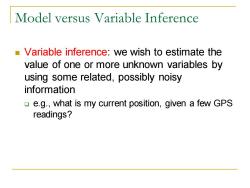
Model versus Variable Inference -Variable inference:we wish to estimate the value of one or more unknown variables by using some related,possibly noisy information e.g.,what is my current position,given a few GPS readings?
Model versus Variable Inference ◼ Variable inference: we wish to estimate the value of one or more unknown variables by using some related, possibly noisy information ❑ e.g., what is my current position, given a few GPS readings?
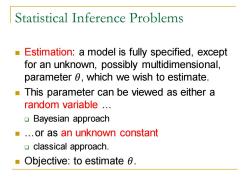
Statistical Inference Problems ■ Estimation:a model is fully specified,except for an unknown,possibly multidimensional, parameter 0,which we wish to estimate. This parameter can be viewed as either a random variable .. Bayesian approach ..or as an unknown constant ▣classical approach. Objective:to estimate 0
Statistical Inference Problems ◼ Estimation: a model is fully specified, except for an unknown, possibly multidimensional, parameter 𝜃, which we wish to estimate. ◼ This parameter can be viewed as either a random variable … ❑ Bayesian approach ◼ …or as an unknown constant ❑ classical approach. ◼ Objective: to estimate 𝜃
按次数下载不扣除下载券;
注册用户24小时内重复下载只扣除一次;
顺序:VIP每日次数-->可用次数-->下载券;
- 香港中文大学:《Probability and Statistics for Engineers》课程教学资源(课件讲稿)Bayesian Statistical Inference.pdf
- 香港中文大学:《Probability and Statistics for Engineers》课程教学资源(课件讲稿)Limit Theorems.pptx
- 香港中文大学:《Probability and Statistics for Engineers》课程教学资源(课件讲稿)Limit Theorems.pdf
- 香港中文大学:《Probability and Statistics for Engineers》课程教学资源(课件讲稿)General random variables.pdf
- 香港中文大学:《Probability and Statistics for Engineers》课程教学资源(课件讲稿)Further Topics on Random Variables.pptx
- 香港中文大学:《Probability and Statistics for Engineers》课程教学资源(课件讲稿)Further Topics on Random Variables.pdf
- 香港中文大学:《Probability and Statistics for Engineers》课程教学资源(课件讲稿)Discrete random variables.pptx
- 香港中文大学:《Probability and Statistics for Engineers》课程教学资源(课件讲稿)Discrete random variables.pdf
- 香港中文大学:《Probability and Statistics for Engineers》课程教学资源(课件讲稿)Sample space and probability.pptx
- 香港中文大学:《Probability and Statistics for Engineers》课程教学资源(课件讲稿)Sample space and probability.pdf
- 香港中文大学:Random Walk on Graphs and its Algorithmic Applications.ppt
- 香港中文大学:An Introduction to Quantum Computing in Theoretical Computer Science.ppt
- 香港中文大学:On the power of lower bound methods for quantum one-way communication complexity.ppt
- 香港中文大学:Quantum strategic game theory.ppt
- 香港中文大学:A quantum protocol for sampling correlated equilibria unconditionally and without a mediator.pptx
- 香港中文大学:On the complexity of trial and error.pptx
- 香港中文大学:Efficient protocols for generating bipartite classical distributions and quantum states.pptx
- 香港中文大学:Fourier sparsity, spectral norm, and the Log-rank conjecture(long).pptx
- 香港中文大学:Fourier sparsity, spectral norm, and the Log-rank conjecture(short).pptx
- 香港中文大学:Fast quantum algorithms for Least Squares Regression and Statistic Leverage Scores.pptx
- 香港中文大学:《Probability and Statistics for Engineers》课程教学资源(课件讲稿)Classical Statistical Inference.pdf
- 香港中文大学:《Probability and Statistics for Engineers》课程教学资源(课件讲稿)General random variables.pptx
- 香港中文大学:《Probability and Statistics for Engineers》课程教学资源(课件讲稿)Classical Statistical Inference.pptx
- 香港中文大学:《Probability and Statistics for Engineers》课程教学资源(辅导材料)Tutorial 1:Sample Space and Probability 1.pdf
- 香港中文大学:《Probability and Statistics for Engineers》课程教学资源(辅导材料)Tutorial 1:Sample Space and Probability 1.pptx
- 香港中文大学:《Probability and Statistics for Engineers》课程教学资源(辅导材料)Tutorial 2:Sample Space and Probability 2.pdf
- 香港中文大学:《Probability and Statistics for Engineers》课程教学资源(辅导材料)Tutorial 2:Sample Space and Probability 2.pptx
- 香港中文大学:《Probability and Statistics for Engineers》课程教学资源(辅导材料)Tutorial 3:Discrete Random Variables 1.pdf
- 香港中文大学:《Probability and Statistics for Engineers》课程教学资源(辅导材料)Tutorial 3:Discrete Random Variables 1.pptx
- 香港中文大学:《Probability and Statistics for Engineers》课程教学资源(辅导材料)Tutorial 4:Discrete Random Variables 2.pdf
- 香港中文大学:《Probability and Statistics for Engineers》课程教学资源(辅导材料)Tutorial 4:Discrete Random Variables 2.pptx
- 香港中文大学:《Probability and Statistics for Engineers》课程教学资源(辅导材料)Tutorial 5:General Random Variables 1.pdf
- 香港中文大学:《Probability and Statistics for Engineers》课程教学资源(辅导材料)Tutorial 5:General Random Variables 1.pptx
- 香港中文大学:《Probability and Statistics for Engineers》课程教学资源(辅导材料)Tutorial 6:General Random Variables 2.pdf
- 香港中文大学:《Probability and Statistics for Engineers》课程教学资源(辅导材料)Tutorial 6:General Random Variables 2.pptx
- 香港中文大学:《Probability and Statistics for Engineers》课程教学资源(辅导材料)Tutorial 7:General Random Variables 3.pdf
- 香港中文大学:《Probability and Statistics for Engineers》课程教学资源(辅导材料)Tutorial 7:General Random Variables 3.pptx
- 香港中文大学:《Probability and Statistics for Engineers》课程教学资源(辅导材料)Tutorial 10:Limit Theorems.pdf
- 香港中文大学:《Probability and Statistics for Engineers》课程教学资源(辅导材料)Tutorial 10:Limit Theorems.pptx
- 香港中文大学:《Probability and Statistics for Engineers》课程教学资源(辅导材料)Tutorial 8:Further Topics on Random Variables 1.pdf