电子科技大学:《信号检测与估计 Signal Detection and Estimation》课程教学资源(课件讲稿)chapter 04 Single Sample Detection of Binary Hypotheses
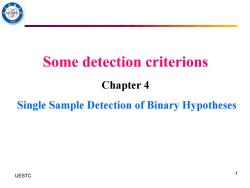
Some detection criterions Chapter 4 Single Sample Detection of Binary Hypotheses UESTC 1
1 UESTC Some detection criterions Chapter 4 Single Sample Detection of Binary Hypotheses
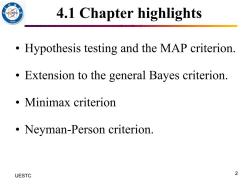
4.1 Chapter highlights Hypothesis testing and the MaP criterion. Extension to the general Bayes criterion. ·inimax criterion Neyman-Person criterion. UESTC 2
2 UESTC 4.1 Chapter highlights • Hypothesis testing and the MAP criterion. • Extension to the general Bayes criterion. • Minimax criterion • Neyman-Person criterion
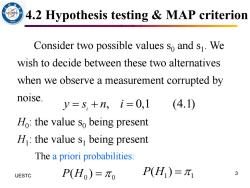
4.2 Hypothesis testing MAP criterion Consider two possible values so and s.We wish to decide between these two alternatives when we observe a measurement corrupted by noise. y=S,+n,i=0,1 (4.1) Ho:the value so being present H:the value s being present The a priori probabilities: UESTC P(Ho)=πo P(H)=π1 3
3 UESTC 4.2 Hypothesis testing & MAP criterion Consider two possible values s0 and s1 . We wish to decide between these two alternatives when we observe a measurement corrupted by noise. H0 : the value s0 being present H1 : the value s1 being present , 0,1 (4.1) i y s n i = + = 0 0 P(H ) = 1 1 P(H ) = The a priori probabilities:

MAP criterion (Maximum A Posteriori):If P(H y)>P(H y) (4.2) we decide so are present,and conversely we decide S if P(H y)>P(H y) (4.3) Using Bayes'theorem,we can write P(Hy)= p(yH)P(H) (4.4) p(y) UESTC 4
4 UESTC 0 1 P H y P H y ( | ) ( | ) (4.2) 1 0 P H y P H y ( | ) ( | ) (4.3) 0 0 0 ( | ) ( ) ( | ) (4.4) ( ) p y H P H P H y p y MAP criterion (Maximum A Posteriori) :If we decide s0 are present, and conversely we decide s1 if Using Bayes’ theorem, we can write
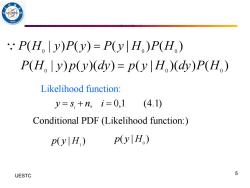
P(H y)P(y)=P(y H)P(H) P(H。Iy)p(y)(y)=p(y|H。)(dy)P(H。) Likelihood function: y=S+n,i=0,1 (4.1) Conditional PDF (Likelihood function: p(yH) p(ylH。) UESTC 5
5 UESTC , 0,1 (4.1) i y s n i = + = 0 p y H ( | ) 1 p y H ( | ) Likelihood function: Conditional PDF (Likelihood function:) 0 0 0 0 0 0 ( | ) ( ) ( | ) ( ) ( | ) ( )( ) ( | )( ) ( ) P H y P y P y H P H P H y p y dy p y H dy P H

Similarly, P(H)=PHDP(H) (4.6) p(y) In order to calculate the a posteriori probabilities, it is necessary to know the a priori probabilities. Let P(Ho)=πo P(H)=π1 UESTC 6
Similarly, In order to calculate the a posteriori probabilities, it is necessary to know the a priori probabilities. Let 6 UESTC 1 1 1 ( | ) ( ) ( | ) (4.6) ( ) p y H P H P H y p y = 0 0 P(H ) = 1 1 P(H ) =

Do:the event associated with decision Ho D:the event associated with decision H D p(y|H1)π1p(y|Ho)πo (4.10) Do p(yHi) 元0 (4.11) p(y|Ho)0元1 Likelihood DI ratio 人L(y) TMAP UESTC DO 7
7 UESTC D0 : the event associated with decision H0 D1 : the event associated with decision H1 1 0 1 1 0 0 ( | ) ( | ) (4.10) D D p y H p y H 1 1 0 0 1 0 1 0 ( | ) (4.11) ( | ) ( ) D D D MAP D p y H p y H L y Likelihood ratio
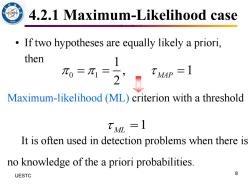
4.2.1 Maximum-Likelihood case If two hypotheses are equally likely a priori, then 元=兀1= 2 Tmap 1 Maximum-likelihood (ML)criterion with a threshold Ev =1 It is often used in detection problems when there is no knowledge of the a priori probabilities. UESTC 8
8 UESTC 4.2.1 Maximum-Likelihood case • If two hypotheses are equally likely a priori, then 0 1 1 , 1 2 = = = MAP Maximum-likelihood (ML) criterion with a threshold It is often used in detection problems when there is no knowledge of the a priori probabilities. ML =1
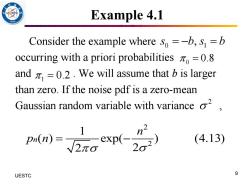
Example 4.1 Consider the example where so =-b,s=b occurring with a priori probabilitieso=0.8 and元,=0.2.We will assume that b is larger than zero.If the noise pdf is a zero-mean Gaussian random variable with variance 902 (4.13) UESTC 9
9 UESTC Example 4.1 Consider the example where occurring with a priori probabilities and . We will assume that b is larger than zero. If the noise pdf is a zero-mean Gaussian random variable with variance , 0 1 s b s b = − = , 0 = 0.8 1 = 0.2 2 2 2 1 ( ) exp( ) (4.13) 2 2 n n p n = −
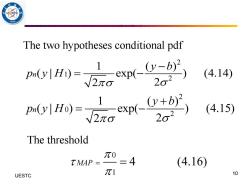
The two hypotheses conditional pdf lHy-" (4.14) (4.15) The threshold 元0 TMAP = 4 (4.16) UESTC π1 10
10 UESTC 2 1 2 1 ( ) ( | ) exp( ) (4.14) 2 2 n y b p y H − = − 2 0 2 1 ( ) ( | ) exp( ) (4.15) 2 2 n y b p y H + = − 0 1 MAP 4 (4.16) = = The two hypotheses conditional pdf The threshold
按次数下载不扣除下载券;
注册用户24小时内重复下载只扣除一次;
顺序:VIP每日次数-->可用次数-->下载券;
- 电子科技大学:《信号检测与估计 Signal Detection and Estimation》课程教学资源(课件讲稿)Introduction、Chapter 01 Preparations.pdf
- 电子科技大学:《信号检测与估计 Signal Detection and Estimation》课程教学资源(教学大纲,何子述).pdf
- 电子科技大学:《敏感材料与传感器 Sensitive Materials and Sensors》课程教学资源(课件讲稿)第八章 电子聚合物基湿度传感器 第二节 电子聚合物基电阻式湿度传感器.pdf
- 电子科技大学:《敏感材料与传感器 Sensitive Materials and Sensors》课程教学资源(课件讲稿)第八章 电子聚合物基湿度传感器 第三节 电子聚合物基电容式湿度传感器.pdf
- 电子科技大学:《敏感材料与传感器 Sensitive Materials and Sensors》课程教学资源(课件讲稿)第八章 电子聚合物基湿度传感器 第一节 电子聚合物基湿度传感器概述.pdf
- 电子科技大学:《敏感材料与传感器 Sensitive Materials and Sensors》课程教学资源(课件讲稿)第九章 专题讲座 第二节 柔性传感器 Flexible Sensors.pdf
- 电子科技大学:《敏感材料与传感器 Sensitive Materials and Sensors》课程教学资源(课件讲稿)第九章 专题讲座 第三节 电子皮肤 E-skin.pdf
- 电子科技大学:《敏感材料与传感器 Sensitive Materials and Sensors》课程教学资源(课件讲稿)第九章 专题讲座 第一节 柔性电子学 Flexible Electronics.pdf
- 电子科技大学:《敏感材料与传感器 Sensitive Materials and Sensors》课程教学资源(课件讲稿)第七章 新型气体传感器 第三节 电子聚合物薄膜气体传感器(质量敏感型有毒有害气体传感器研究).pdf
- 电子科技大学:《敏感材料与传感器 Sensitive Materials and Sensors》课程教学资源(课件讲稿)第七章 新型气体传感器 第二节 无机半导体气体传感器.pdf
- 电子科技大学:《敏感材料与传感器 Sensitive Materials and Sensors》课程教学资源(课件讲稿)第七章 新型气体传感器 第三节 电子聚合物薄膜气体传感器(有机薄膜晶体管气体传感器的制备及特性研究).pdf
- 电子科技大学:《敏感材料与传感器 Sensitive Materials and Sensors》课程教学资源(课件讲稿)第七章 新型气体传感器 第三节 电子聚合物薄膜气体传感器(聚苯胺复合薄膜电阻型气体传感器的制备及特性研究).pdf
- 电子科技大学:《敏感材料与传感器 Sensitive Materials and Sensors》课程教学资源(课件讲稿)第七章 新型气体传感器 第一节 新型气体传感器概述.pdf
- 电子科技大学:《敏感材料与传感器 Sensitive Materials and Sensors》课程教学资源(课件讲稿)第六章 新型红外传感器 第3节 非致冷红外传感器.pdf
- 电子科技大学:《敏感材料与传感器 Sensitive Materials and Sensors》课程教学资源(课件讲稿)第六章 新型红外传感器 第2节 红外传感器分类及性能参数.pdf
- 电子科技大学:《敏感材料与传感器 Sensitive Materials and Sensors》课程教学资源(课件讲稿)第六章 新型红外传感器 第1节 红外辐射的基本知识.pdf
- 电子科技大学:《敏感材料与传感器 Sensitive Materials and Sensors》课程教学资源(课件讲稿)第六章 新型红外传感器.pdf
- 电子科技大学:《敏感材料与传感器 Sensitive Materials and Sensors》课程教学资源(课件讲稿)第五章 有机敏感材料.pdf
- 电子科技大学:《敏感材料与传感器 Sensitive Materials and Sensors》课程教学资源(课件讲稿)第四章 半导体材料.pdf
- 电子科技大学:《敏感材料与传感器 Sensitive Materials and Sensors》课程教学资源(课件讲稿)第三章 陶瓷敏感材料.pdf
- 电子科技大学:《信号检测与估计 Signal Detection and Estimation》课程教学资源(课件讲稿)Chapter 05 Multiple Sample Detection of Binary Hypotheses.pdf
- 电子科技大学:《信号检测与估计 Signal Detection and Estimation》课程教学资源(课件讲稿)Chapter 06 Detection of signals with random parameters.pdf
- 电子科技大学:《信号检测与估计 Signal Detection and Estimation》课程教学资源(课件讲稿)Chapter 07 Multiple pulse detection with random parameters.pdf
- 电子科技大学:《信号检测与估计 Signal Detection and Estimation》课程教学资源(课件讲稿)Chapter 09 Nonparametric detection.pdf
- 电子科技大学:《信号检测与估计 Signal Detection and Estimation》课程教学资源(课件讲稿)Chapter 10 Fundamentals of Estimation Theory.pdf
- 电子科技大学:《信号检测与估计 Signal Detection and Estimation》课程教学资源(课件讲稿)Chapter 11 Estimation of Specific Parameters.pdf
- 电子科技大学:《射频集成电路 RF Integrated Circuits》课程教学资源(课件讲稿)第一讲 CMOS工艺(游飞).pdf
- 电子科技大学:《射频集成电路 RF Integrated Circuits》课程教学资源(课件讲稿)第三讲 单级放大器.pdf
- 电子科技大学:《射频集成电路 RF Integrated Circuits》课程教学资源(课件讲稿)第二讲 CMOS器件.pdf
- 电子科技大学:《射频集成电路 RF Integrated Circuits》课程教学资源(课件讲稿)第四讲 噪声 Noise.pdf
- 电子科技大学:《射频集成电路 RF Integrated Circuits》课程教学资源(课件讲稿)第七讲 混合器 Mixers.pdf
- 电子科技大学:《射频集成电路 RF Integrated Circuits》课程教学资源(课件讲稿)第九讲 Power Amplifiers.pdf
- 电子科技大学:《射频集成电路 RF Integrated Circuits》课程教学资源(课件讲稿)第五讲 Transceiver Architecture.pdf
- 电子科技大学:《射频集成电路 RF Integrated Circuits》课程教学资源(课件讲稿)第八讲 Passive devices.pdf
- 电子科技大学:《射频集成电路 RF Integrated Circuits》课程教学资源(课件讲稿)第六讲 Low-Noise Amplifier.pdf
- 电子科技大学:《射频电路理论与应用》课程教学资源(教学大纲)RF Circuit Design - Theory and Application(主讲:游长江).pdf
- 电子科技大学:《射频电路理论与应用》课程教学资源(课件讲稿)第0章 绪论(主讲:游长江).pdf
- 电子科技大学:《射频电路理论与应用》课程教学资源(课件讲稿)第1章 射频通信系统理论.pdf
- 电子科技大学:《射频电路理论与应用》课程教学资源(课件讲稿)第2章 射频通信系统中电路理论(2/2).pdf
- 电子科技大学:《射频电路理论与应用》课程教学资源(课件讲稿)第3章 射频通信传播和天线基础理论.pdf