南京大学:《并行处理技术——分布式与并行计算 Distributed and Parallel computing(并行计算——结构、算法、编程)》课程教学资源(课件讲稿)专题一 MapReduce的概念、原理与应用

专题l:MapReduce的概念、原理 与应用 谢磊博士 南京大学计算机科学与技术系
专题1: MapReduce的概念、原理 与应用 谢磊 博士 南京大学计算机科学与技术系
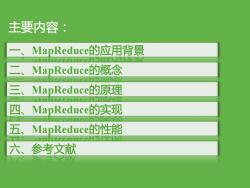
主要内容: 一、MapReduce的应用背景 二 MapReduce的概念 三、MapReduce的原理 四、MapReduce的实现 五、MapReduce的性能 六、参考文献
一、MapReduce的应用背景 三、MapReduce的原理 主要内容: 二、MapReduce的概念 五、MapReduce的性能 四、MapReduce的实现 六、参考文献
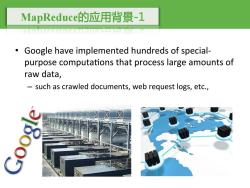
MapReduce的应用背景-l Google have implemented hundreds of special- purpose computations that process large amounts of raw data, such as crawled documents,web request logs,etc
MapReduce的应用背景-1 • Google have implemented hundreds of specialpurpose computa6ons that process large amounts of raw data, – such as crawled documents, web request logs, etc
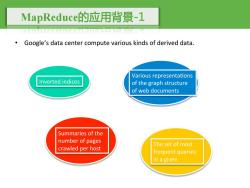
MapReduce的应用背景-1 Google's data center compute various kinds of derived data. Various representations Inverted indices of the graph structure of web documents Summaries of the number of pages The set of most crawled per host frequent queries in a given
MapReduce的应用背景-1 • Google’s data center compute various kinds of derived data. Inverted indices Various representa6ons of the graph structure of web documents Summaries of the number of pages crawled per host The set of most frequent queries in a given
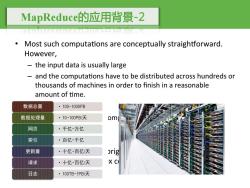
MapReduce的应用背景-2 Most such computations are conceptually straightforward. However, the input data is usually large and the computations have to be distributed across hundreds or thousands of machines in order to finish in a reasonable amount of time. 数据总量 ·100~1000PB 数据处理量 ·10~100PB/天 oml 网页 ·千亿万亿 索引 ·百亿千亿 更新量 ·十亿~百亿天 orig 请求 ·十亿~百亿/天 X C 日志 100TB~1PB/天
MapReduce的应用背景-2 • Most such computa6ons are conceptually straighDorward. However, – the input data is usually large – and the computa6ons have to be distributed across hundreds or thousands of machines in order to finish in a reasonable amount of 6me. • The issues of – how to parallelize the computa6on, – distribute the data, – and handle failures • conspire to obscure the original simple computa6on with large amounts of complex code to deal with these issues
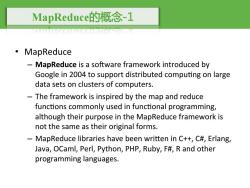
MapReduce的概念-1 ·MapReduce MapReduce is a software framework introduced by Google in 2004 to support distributed computing on large data sets on clusters of computers. The framework is inspired by the map and reduce functions commonly used in functional programming, although their purpose in the MapReduce framework is not the same as their original forms. MapReduce libraries have been written in C++,C#,Erlang, Java,OCaml,Perl,Python,PHP,Ruby,F#,R and other programming languages
MapReduce的概念-1 • MapReduce – MapReduce is a soJware framework introduced by Google in 2004 to support distributed compu6ng on large data sets on clusters of computers. – The framework is inspired by the map and reduce func6ons commonly used in func6onal programming, although their purpose in the MapReduce framework is not the same as their original forms. – MapReduce libraries have been wriNen in C++, C#, Erlang, Java, OCaml, Perl, Python, PHP, Ruby, F#, R and other programming languages

MapReduce的概念-2 MapReduce is a framework for processing huge datasets on certain kinds of distributable problems using a large number of computers(nodes). The nodes collectively are referred to as a cluster (if all nodes use the same hardware) or a grid (if the nodes use different hardware). Computational processing can occur on data stored either in a filesystem(unstructured)or within a database(structured)
MapReduce的概念-2 • MapReduce is a framework for processing huge datasets on certain kinds of distributable problems using a large number of computers (nodes). • The nodes collec6vely are referred to as – a cluster (if all nodes use the same hardware) – or a grid (if the nodes use different hardware). • Computa6onal processing can occur on data stored either in a filesystem (unstructured) or within a database (structured)
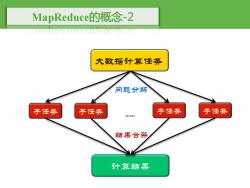
MapReduce的概念-2 大数据计算任务 问题分解 子任务 子任务 子任务 子任务 结果合 计算结果
MapReduce的概念-2 大数据计算任务 子任务 子任务 子任务 子任务 …… 问题分解 计算结果 结果合并
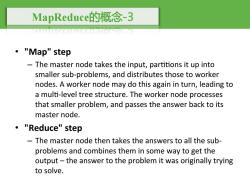
MapReduce的概念-3 ·"Map"step The master node takes the input,partitions it up into smaller sub-problems,and distributes those to worker nodes.A worker node may do this again in turn,leading to a multi-level tree structure.The worker node processes that smaller problem,and passes the answer back to its master node. 。"Reduce"step The master node then takes the answers to all the sub- problems and combines them in some way to get the output-the answer to the problem it was originally trying to solve
MapReduce的概念-3 • "Map" step – The master node takes the input, par66ons it up into smaller sub-problems, and distributes those to worker nodes. A worker node may do this again in turn, leading to a mul6-level tree structure. The worker node processes that smaller problem, and passes the answer back to its master node. • "Reduce" step – The master node then takes the answers to all the subproblems and combines them in some way to get the output – the answer to the problem it was originally trying to solve
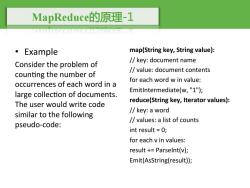
MapReduce的原理-I 。Example map(String key,String value): /key:document name Consider the problem of /value:document contents counting the number of for each word w in value: occurrences of each word in a EmitIntermediate(w,"1"); large collection of documents. reduce(String key,Iterator values): The user would write code /key:a word similar to the following /values:a list of counts pseudo-code: int result 0; for each v in values: result +Parselnt(v); Emit(AsString(result));
MapReduce的原理-1 • Example Consider the problem of coun6ng the number of occurrences of each word in a large collec6on of documents. The user would write code similar to the following pseudo-code: map(String key, String value): // key: document name // value: document contents for each word w in value: EmitIntermediate(w, "1"); reduce(String key, Iterator values): // key: a word // values: a list of counts int result = 0; for each v in values: result += ParseInt(v); Emit(AsString(result));
按次数下载不扣除下载券;
注册用户24小时内重复下载只扣除一次;
顺序:VIP每日次数-->可用次数-->下载券;
- 南京大学:《并行处理技术——分布式与并行计算 Distributed and Parallel computing(并行计算——结构、算法、编程)》课程教学资源(课件讲稿)第九章 并行数值算法(稠密矩阵运算).pdf
- 南京大学:《并行处理技术——分布式与并行计算 Distributed and Parallel computing(并行计算——结构、算法、编程)》课程教学资源(课件讲稿)第八章 并行数值算法(基本通信操作).pdf
- 南京大学:《并行处理技术——分布式与并行计算 Distributed and Parallel computing(并行计算——结构、算法、编程)》课程教学资源(课件讲稿)第七章 并行算法的一般设计过程.pdf
- 南京大学:《并行处理技术——分布式与并行计算 Distributed and Parallel computing(并行计算——结构、算法、编程)》课程教学资源(课件讲稿)第六章 并行算法的基本设计技术.pdf
- 南京大学:《并行处理技术——分布式与并行计算 Distributed and Parallel computing(并行计算——结构、算法、编程)》课程教学资源(课件讲稿)第五章 并行算法的一般设计方法.pdf
- 南京大学:《并行处理技术——分布式与并行计算 Distributed and Parallel computing(并行计算——结构、算法、编程)》课程教学资源(课件讲稿)第四章 并行算法的设计基础.pdf
- 南京大学:《并行处理技术——分布式与并行计算 Distributed and Parallel computing(并行计算——结构、算法、编程)》课程教学资源(课件讲稿)第三章 并行计算硬件结构基础(并行计算性能评测).pdf
- 南京大学:《并行处理技术——分布式与并行计算 Distributed and Parallel computing(并行计算——结构、算法、编程)》课程教学资源(课件讲稿)专题二 云计算的概念、技术与应用.pdf
- 南京大学:《并行处理技术——分布式与并行计算 Distributed and Parallel computing(并行计算——结构、算法、编程)》课程教学资源(课件讲稿)第二章 并行计算硬件结构基础——当代并行机系统(SMP、MPP和Cluster).pdf
- 南京大学:《并行处理技术——分布式与并行计算 Distributed and Parallel computing(并行计算——结构、算法、编程)》课程教学资源(课件讲稿)第一章 并行计算硬件结构基础(并行计算机系统及其结构模型).pdf
- 南京大学:《并行处理技术——分布式与并行计算 Distributed and Parallel computing(并行计算——结构、算法、编程)》课程教学资源(课件讲稿)引论 Introduction(谢磊).pdf
- 计算机科学与技术(参考文献)Focus and Shoot - Efficient Identification over RFID Tags in the Specified Area.pdf
- 计算机科学与技术(参考文献)Search for a Needle in a Haystack - an RFID-based Approach for Efficiently Locating Objects.pdf
- 计算机科学与技术(参考文献)Efficient Route Guidance in Vehicular Wireless Networks.pdf
- 计算机科学与技术(参考文献)FootStep-Tracker:An Anchor-Free Indoor Localization System via Sensing Foot Steps.pdf
- 计算机科学与技术(参考文献)TouchID - User Authentication on Mobile Devices via Inertial-Touch Gesture Analysis.pdf
- 计算机科学与技术(参考文献)RF-ECG - Heart Rate Variability Assessment Based on COTS RFID Tag Array.pdf
- 计算机科学与技术(参考文献)RF-Kinect - A Wearable RFID-based Approach Towards 3D Body Movement Tracking.pdf
- 计算机科学与技术(参考文献)Tremor Detection Using Smartphone-based Acoustic Sensing.pdf
- 计算机科学与技术(参考文献)Tell Me What I See - Recognize RFID Tagged Objects in Augmented Reality Systems.pdf
- 南京大学:《并行处理技术——分布式与并行计算 Distributed and Parallel computing(并行计算——结构、算法、编程)》课程教学资源(课件讲稿)专题三 边缘智能(边缘计算时代的人工智能).pdf
- 南京大学:《物联网技术导论 Introduction of Internet of Things》课程教学资源(课件讲稿)课程介绍 Introduction(谢磊).pdf
- 南京大学:《物联网技术导论 Introduction of Internet of Things》课程教学资源(课件讲稿)第一章 物联网概述.pdf
- 南京大学:《物联网技术导论 Introduction of Internet of Things》课程教学资源(课件讲稿)第二章 智能感知技术概述.pdf
- 南京大学:《物联网技术导论 Introduction of Internet of Things》课程教学资源(课件讲稿)第三章 传感器感知技术.pdf
- 南京大学:《物联网技术导论 Introduction of Internet of Things》课程教学资源(课件讲稿)第六章 自动识别技术与RFID(RFID防冲突协议与无源感知技术).pdf
- 南京大学:《物联网技术导论 Introduction of Internet of Things》课程教学资源(课件讲稿)第四章 非传感器感知技术.pdf
- 南京大学:《物联网技术导论 Introduction of Internet of Things》课程教学资源(课件讲稿)专题——RFID的识别与估算机制.pdf
- 南京大学:《物联网技术导论 Introduction of Internet of Things》课程教学资源(课件讲稿)专题——从识别到感知(基于RFID的可标记无源感知机制研究).pdf
- 南京大学:《物联网技术导论 Introduction of Internet of Things》课程教学资源(课件讲稿)第七章 传感器技术(传感器网络).pdf
- 南京大学:《物联网技术导论 Introduction of Internet of Things》课程教学资源(课件讲稿)第八章 定位系统(定位技术).pdf
- 南京大学:《物联网技术导论 Introduction of Internet of Things》课程教学资源(课件讲稿)专题——物联网定位机制(概念、原理与前沿技术)以及基于位置的服务.pdf
- 南京大学:《物联网技术导论 Introduction of Internet of Things》课程教学资源(课件讲稿)专题——基于RFID的感知机制研究(RFID as a Sensing Tool).pdf
- 南京大学:《物联网技术导论 Introduction of Internet of Things》课程教学资源(课件讲稿)第九章 物联网信息安全与隐私保护.pdf
- RFID标签识别机制-冲突以及防冲突算法研究(参考文献)Efficient Tag Identification in Mobile RFID Systems.pdf
- RFID标签识别机制-冲突以及防冲突算法研究(参考文献)P-MTI - Physical-layer Missing Tag Identification via Compressive Sensing.pdf
- RFID标签识别机制-冲突以及防冲突算法研究(参考文献)Probabilistic Optimal Tree Hopping for RFID Identification.pdf
- RFID标签识别机制-冲突以及防冲突算法研究(参考文献)Season Shelving Interference and Joint Identification in Large-scale RFID Systems.pdf
- RFID标签识别机制-冲突以及防冲突算法研究(参考文献)Using Analog Network Coding to Improve the RFID Reading Throughput.pdf
- RFID标签数目估算机制研究(参考文献)An Efficient Protocol for RFID Multigroup Threshold-based Classification.pdf