西安电子科技大学:《信息论基础》课程教学资源(学习资料)MIMO信道容量 Channel
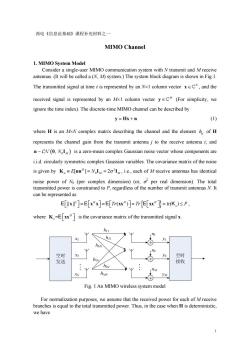
西电《信息论基础》课程补充材料之 MIMO Channel 1.MIMO System Model Consider a single-user MIMO communication system with N transmit and M receive antennas.(It will be called a(N,M)system.)The system block diagram is shown in Fig.1 The transmitted signal at time tis represented by an Nx1 column vectorxC,and the received signal is represented by an MxI column vector yCM (For simplicity,we ignore the time index).The discrete-time MIMO channel can be described by y=Hx+n (1) where H is an MxN complex matrix describing the channel and the elementh of H represents the channel gain from the transmit antennato the receive antenna i:and n~CN(0,N)is a zero-mean complex Gaussian noise vector whose components are i.id.circularly symmetric complex Gaussian variables.The covariance matrix of the noise is given by KE[nn"]=N=2,i.e.,each of Mreceive antennas has identical plex dimension)(or per real dimension).The total ransmited power constrained toP.regardles of the number of transmit antens N.I com can be represented as ElxIP=E x"x=E Tr(xx")=TrE xx"=tr(K,)sP, where K,=E xx"is the covariance matrix of the transmitted signal x. 、hI 空的 空时 接收 Fig.1An MIMO wireless system model For normalization purposes,we assume that the received power for each of Mreceive branches is equal to the total transmitted power.Thus.in the case when H is deterministic. we have
1 西电《信息论基础》课程补充材料之一 MIMO Channel 1. MIMO System Model Consider a single-user MIMO communication system with N transmit and M receive antennas. (It will be called a (N, M) system.) The system block diagram is shown in Fig.1. The transmitted signal at time t is represented by an N×1 column vector N x ∈C , and the received signal is represented by an M×1 column vector M y ∈C (For simplicity, we ignore the time index). The discrete-time MIMO channel can be described by y = Hx n + (1) where H is an M×N complex matrix describing the channel and the element ij h of H represents the channel gain from the transmit antenna j to the receive antenna i; and ( ) 0 , n 0I ∼ CN N M is a zero-mean complex Gaussian noise vector whose components are i.i.d. circularly symmetric complex Gaussian variables. The covariance matrix of the noise is given by 2 0 [] 2 H K nn I I n MM ≡ == E N σ , i.e., each of M receive antennas has identical noise power of N0 (per complex dimension) (or, σ 2 per real dimension). The total transmitted power is constrained to P, regardless of the number of transmit antennas N. It can be represented as { } 2 || || ( ) tr( ) HHH Tr Tr P x ⎡ ⎤⎡⎤⎡ ⎤ ⎡⎤ = = = =≤ ⎣ ⎦⎣⎦⎣ ⎦ ⎣⎦ E EE E K x x x xx xx , where = H x ⎡ ⎤ K xx ⎣ ⎦ E is the covariance matrix of the transmitted signal x. + + + Fig. 1 An MIMO wireless system model For normalization purposes, we assume that the received power for each of M receive branches is equal to the total transmitted power. Thus, in the case when H is deterministic, we have
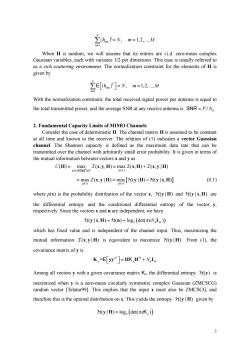
SIhf=N,m=12.M When H is random,we will assume that its entries are i.i.d.zero-mean complex Gaussian variables,each with variance 1/2 per dimension.This case is usually referred to as a rich scattering environment.The normalization constraint for the elements of H is given by 2[kf门=Nm=l2M With the normalization constraint,the total received signal power per antenna is equal to the total transmitted power,and the average SNR at any receive antenna is SNR=P/N 2.Fundamental Capacity Limits of MIMO Channels Consider the case of deterministic H.The channel matrix H is assumed to be constant at all time and known to the receiver The relation of (1)indicates a vector gaussian channel.The Shann acity is defined as the max data rate that can be transmitted over the channel with arbitrarily small error probability.It is given in terms o the mutual information between vectors x and y as C(H)= aZy=maT(x.H)+I(x:y|H) max T(x;y |H)max[H(y |H)-H(ylx,H) (0.1) where p(x)is the probability distribution of the vector x,H(yH)and H(yx,H)are the differential entropy and the conditional differential entropy of the vector y. respectively.Since the vectorsx and nare independent,we have Hylx,HD=H()=log2(det(πeN,lw) which has fixed value and is independent of the channel input.Thus,maximizing the mutual information T(x;y|H)is equivalent to maximize H(y|H).From (1),the covariance matrix ofy is K,=Eyy"=HK,H#+NIv Among all vectors y with a given covariance matrix K,the differential entropy H(y)is maximied when yis ircularly complex Gaussian (ZMCSCG) random vector [Telatar99].This implies that the inputx must also be ZMCSCG and therefore this is the optimal distribution onx.This yields the entropy H(yH)given by H(yl田)=log2(det(πek,)
2 2 1 | | , 1,2,., N mn n h Nm M = ∑ = = When H is random, we will assume that its entries are i.i.d. zero-mean complex Gaussian variables, each with variance 1/2 per dimension. This case is usually referred to as a rich scattering environment. The normalization constraint for the elements of H is given by 2 1 | | , 1,2,., N mn n h Nm M = ⎡ ⎤ = = ∑ ⎣ ⎦ E With the normalization constraint, the total received signal power per antenna is equal to the total transmitted power, and the average SNR at any receive antenna is 0 SNR = P N/ . 2. Fundamental Capacity Limits of MIMO Channels Consider the case of deterministic H. The channel matrix H is assumed to be constant at all time and known to the receiver. The relation of (1) indicates a vector Gaussian channel. The Shannon capacity is defined as the maximum data rate that can be transmitted over the channel with arbitrarily small error probability. It is given in terms of the mutual information between vectors x and y as 2 ( ): [|| || ] ( ) ( ) max ( ; , ) max ( ; ) ( ; | ) p P p x C ≤ = =+ x x H x I II y H xH x y H E [ ] () () max ( ; | ) max ( | ) ( | , ) px px = I HH x y H = − y H y x H (0.1) where p(x) is the probability distribution of the vector x, H H ( | ) and ( | , ) y H y x H are the differential entropy and the conditional differential entropy of the vector y, respectively. Since the vectors x and n are independent, we have H H ( | , ) ( ) log det( ) y xH n I = = 2 0 ( πeN M ) which has fixed value and is independent of the channel input. Thus, maximizing the mutual information ( ; | ) I x y H is equivalent to maximize ( | ) H y H . From (1), the covariance matrix of y is = 0 H H y xM ⎡ ⎤ = + N ⎣ ⎦ K E K yy HH I Among all vectors y with a given covariance matrix Ky, the differential entropy ( ) H y is maximized when y is a zero-mean circularly symmetric complex Gaussian (ZMCSCG) random vector [Telatar99]. This implies that the input x must also be ZMCSCG, and therefore this is the optimal distribution on x. This yields the entropy ( | ) H y H given by H( | ) log det( ) y H = 2 ( πeK y )

The mutual information then reduces to Z(x;yH)=H(y|H)-H(n) (0.2) where we have used the fact that det(AB)=det(A)det(B)and det(A-)=[det(A) And the MIMO capacity is given by maximizing the mutual information (0.2)over all input covariance matrces Kx satisfying the power constraint: c国=腮,oea,+Kr bits per channel use (0.3) 殿ea+是Kr where the last equality follows from the fact that det(I+AB)=det(I +BA)for matrices A (mxn)and B(nxm) Clearly,the optimization relative to K will depend on whether or not H is known at the transmitter.We now discuss this maximizing under different assumptions about transmitter CSI by decomposing the vector channel into a set of parallel,independent scalar Gaussian sub-cha nels 2.3 Channel Unknown to the Transmitter If the channel is known to the receiver.but not to the transmitter,then the transmitter cannot optimize its power allocation or input covariance structure across antennas.This that if the disrbution of Holo he ero-mean spatiall hannel gai odel,the signals independent and the power should be equally divided among the transmit antennas,resulting an input covariance matriK.It is shown in tar]that this K,indeed maxm the mutual information.Thus,the capacity in such a case is log:detSNR HH" ifM<N C= bits per channel use (0.4) e[+)MN where SNR=P/No 2.1 Parallel Decomposition of the MIMO Channel
3 The mutual information then reduces to I HH (; | ) ( | ) () x y H = − y H n 2 0 1 log det H M x N ⎛ ⎞ ⎛ ⎞ = + ⎜ ⎟ ⎜ ⎟ ⎝ ⎠ ⎝ ⎠ I HH K (0.2) where we have used the fact that det( ) det( )det( ) AB A B = and [ ] 1 1 det( ) det( ) − − A A = . And the MIMO capacity is given by maximizing the mutual information (0.2) over all input covariance matrces Kx satisfying the power constraint: 2 :( ) 0 1 ( ) max log det x x H M x Tr P C = N ⎛ ⎞ ⎛ ⎞ = + ⎜ ⎟ ⎜ ⎟ ⎝ ⎠ ⎝ ⎠ H I HH K K K bits per channel use (0.3) 2 :( ) 0 1 max log det x x H N x Tr P= N ⎛ ⎞ ⎛ ⎞ = + ⎜ ⎟ ⎜ ⎟ ⎝ ⎠ ⎝ ⎠ I HH K K K where the last equality follows from the fact that det det (I AB I BA m n += + )( ) for matrices ( ) and ( ) A B mn nm × × . Clearly, the optimization relative to Kx will depend on whether or not H is known at the transmitter. We now discuss this maximizing under different assumptions about transmitter CSI by decomposing the vector channel into a set of parallel, independent scalar Gaussian sub-channels. 2.3 Channel Unknown to the Transmitter If the channel is known to the receiver, but not to the transmitter, then the transmitter cannot optimize its power allocation or input covariance structure across antennas. This implies that if the distribution of H follows the zero-mean spatially white (ZMSW) channel gain model, the signals transmitted from N antennas should be independent and the power should be equally divided among the transmit antennas, resulting an input covariance matrix x N P N K = I . It is shown in [Telatar99] that this Kx indeed maximize the mutual information. Thus, the capacity in such a case is 2 2 log det , if log det , if H M H N M N N C M N N ⎧ ⎡ ⎤ ⎛ ⎞ ⎪ ⎜ ⎟ + < ⎢ ⎥ ⎪⎣ ⎦ ⎝ ⎠ = ⎨ ⎪ ⎡ ⎤ ⎛ ⎞ ⎜ ⎟ + ≥ ⎪ ⎢ ⎥ ⎩ ⎣ ⎦ ⎝ ⎠ I HH I HH SNR SNR bits per channel use (0.4) where 0 SNR = P N/ . 2.1 Parallel Decomposition of the MIMO Channel
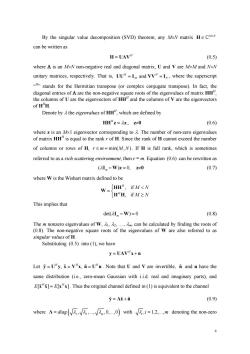
By the singular value decomposition(SVD)theorem,any MxN matrix HC can be written as H=UAV (0.5) where A is an MxN non-negative real and diagonal matrix,U and V are MxM and NxN unitary matrices,respectively.That is,UU=I and VV#=I,where the superscript stands for the Hermitian transpose (or complex conjugate transpose).In fact,the diagonal entries of A are the non-negative square roots of the eigenvalues of matrix HH, the columns of U are the eigenvectors of HH and the columns of V are the eigenvectors of HH Denote bythe eigemalues of HH which are defined by HH“z=z,Z0 (0.6) where z is an Mxl eigenvector corresponding to The number of non-zero eigenvalues of matrixHH is equal to the rankrof H.Since the rank of H cannot exceed the number of columns or rows of H.rsm=min(M,N).If H is full rank,which is sometimes referred to as a rich scattering environment,then r=m.Equation (0.6)can be rewritten as (L.-W)z=0,z0 (0.7) where W is the Wishart matrix defined to be W=H"ifM<N HH,ifM≥N This implies that det(l -W)=0 (0.8) The m nonzero eigenvalues of W, .can be calculated by finding the roots of (0.8).The non-negative square roots of the eigenvalues of W are also referred to as singular values of H. Substituting (0.5)into(1).we have y=UAV"x+n Let y=U"y,=V"x,n=U"n.Note that U and V are invertible,n and n have the same distribution (i.e.,zero-mean Gaussian with i.i.d.real and imaginary parts),and E[=E[xx].Thus the original channel defined in(1)is equivalent to the channel y=A+ (0.9) where A=diag(V,√5,√,0,0)with√万,i=l2,denoting the non-zero 4
4 By the singular value decomposition (SVD) theorem, any M×N matrix M ×N H∈C can be written as H H U= ΛV (0.5) where Λ is an M×N non-negative real and diagonal matrix, U and V are M×M and N×N unitary matrices, respectively. That is, and H H UU I VV I = M = N , where the superscript “ H” stands for the Hermitian transpose (or complex conjugate transpose). In fact, the diagonal entries of Λ are the non-negative square roots of the eigenvalues of matrix HHH, the columns of U are the eigenvectors of HHH and the columns of V are the eigenvectors of HHH. Denote by λ the eigenvalues of HHH, which are defined by H HH z z = λ , z≠0 (0.6) where z is an M×1 eigenvector corresponding to λ. The number of non-zero eigenvalues of matrix HHH is equal to the rank r of H. Since the rank of H cannot exceed the number of columns or rows of H, min( , ) r m MN ≤ = . If H is full rank, which is sometimes referred to as a rich scattering environment, then r = m. Equation (0.6) can be rewritten as ( ) 0, λI Wz m − = z≠0 (0.7) where W is the Wishart matrix defined to be , if , if H H M N M N ⎧ < = ⎨ ⎩ ≥ HH W H H This implies that det( ) 0 λI W m − = (0.8) The m nonzero eigenvalues of W, λ1, λ2, ., λm, can be calculated by finding the roots of (0.8). The non-negative square roots of the eigenvalues of W are also referred to as singular values of H. Substituting (0.5) into (1), we have H y U= ΛVx n + Let , , HHH y === U y x Vxn Un . Note that U and V are invertible, n and n have the same distribution (i.e., zero-mean Gaussian with i.i.d. real and imaginary parts), and [][] H H E E xx xx = . Thus the original channel defined in (1) is equivalent to the channel y = Λx n + (0.9) where ( ) 1 2 , ,., ,0,.,0 m Λ = diag λλ λ with , 1,2,., i λ i m = denoting the non-zero

singular values of H.The equivalence is summarized in Fig.2.From (0.9).we obtain for the received signal components 元=V元+i,1≤i≤m (0.10) y=i,m+1≤i≤M It is seen that received components,i>m,do not depend on the transmitted signal.On the other hand,received components=1,2.m depend only the transmitted component Thus the equivalent MIMO channel in (0.9)can be considered as consisting of m uncoupled parallel Gaussian sub-channels.Specifically. If N>M,(0.10)indicates that there will be at most M non-zero attenuation subchar If M-N,there will be at most N non-zero attenuation subchannels in the equivalent MIMO channel. channel H Figure 2 Converting the MIMO channel into a parallel channel through the SVD. 0 Fig.3 Block diagram of an equivalent MIMO channel for N>M
5 singular values of H. The equivalence is summarized in Fig. 2. From (0.9), we obtain for the received signal components , 1 , m+1 i ii i i i y x n im y n iM = + ≤≤ λ = ≤≤ (0.10) It is seen that received components , i y i m> , do not depend on the transmitted signal. On the other hand, received components , 1,2,., i y i m = depend only the transmitted component i x . Thus the equivalent MIMO channel in (0.9) can be considered as consisting of m uncoupled parallel Gaussian sub-channels. Specifically, If N>M, (0.10) indicates that there will be at most M non-zero attenuation subchannels in the equivalent MIMO channel. See Fig. 3. If M>N, there will be at most N non-zero attenuation subchannels in the equivalent MIMO channel. V U x H V . × × + + H U λ1 λm 1 n mn y 预处理 后处理 channel H x y Figure 2 Converting the MIMO channel into a parallel channel through the SVD. Fig. 3 Block diagram of an equivalent MIMO channel for N>M

With the above model (parallel orthogonal channels),the fundamental capacity of ar as follows. 2.2 Channel Known to the Transmitter When the perfect channel knowledge is available at both the transmitter and the the transmiter can optimize its ower input covariancematri across antennas according to the"water-filling"rule (in space)to maximize the capacity formula(0.3).Substituting the matrix SVD(0.5)into (0.3)and using properties of unitary matrices,we get the MIMO capacity with CSIT and CSIR as C-恩+K,刃 -2e+ optimization leads to a 1sism,is given parametrically by (0.11) where denotes max(0,a),and uis chosen to satisfy EA-P (0.12) The resulting capacity is then bits/channel use (0.13) which is achieved by choosing each component according to an independent Gaussian distribution with power P The covariance matrix of the capacity-achieving transmitted signal is given by K,=VPV# where P=diag(P.P.P.0.0)is an NxN matrix. Water-filling algorithm: The power allocation in (0.11)can be determined iteratively using the water-filling algorithm.We now describe it. We first set the iteration count p to 1 and assume that所有(m-p叶l)个并行子信道都 6
6 With the above model (parallel orthogonal channels), the fundamental capacity of an MIMO channel can be calculated in terms of the positive eigenvalues of the matrix HHH as follows. 2.2 Channel Known to the Transmitter When the perfect channel knowledge is available at both the transmitter and the receiver, the transmitter can optimize its power allocation or input covariance matrix across antennas according to the “water-filling” rule (in space) to maximize the capacity formula (0.3). Substituting the matrix SVD (0.5) into (0.3) and using properties of unitary matrices, we get the MIMO capacity with CSIT and CSIR as 2 :( ) 0 1 max log det x x H N x Tr P C = N ⎛ ⎞ ⎛ ⎞ = + ⎜ ⎟ ⎜ ⎟ ⎝ ⎠ ⎝ ⎠ I Λ Λ K K K 2 : 1 0 max log 1 i i i m i i P PP i P N λ ≤ = ⎛ ⎞ = + ⎜ ⎟ ∑ ⎝ ⎠ ∑ where Pi is the transmit power in the ith sub-channel. Solving the optimization leads to a water-filling power allocation over the parallel channels. The power allocated to channel i, 1≤i≤m, is given parametrically by 0 i i N P μ λ + ⎛ ⎞ = − ⎜ ⎟ ⎝ ⎠ (0.11) where a+ denotes max(0, a), and μ is chosen to satisfy 1 m i i P P = ∑ = (0.12) The resulting capacity is then WF 2 0 2 ( ) 1 1 0 0 1 1 log 1 log m m i i i i C N N N μλ μλ + + = = ⎡ ⎤ ⎛⎞ = + −= ⎢ ⎥ ⎜ ⎟ ⎣ ⎦ ⎝⎠ ∑ ∑ bits/channel use (0.13) which is achieved by choosing each component i x according to an independent Gaussian distribution with power Pi. The covariance matrix of the capacity-achieving transmitted signal is given by H Kx = VPV where ( ) 1 2 diag , ,., ,0,.,0 P = PP Pm is an N×N matrix. Water-filling algorithm: The power allocation in (0.11) can be determined iteratively using the water-filling algorithm. We now describe it. We first set the iteration count p to 1 and assume that 所有(m-p+1)个并行子信道都
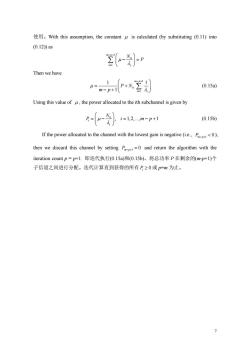
With this assumption,the constant u is calculated (by substituting (0.11)into (0.12)as Then we have a"a-pP+空 (0.15a) Using this value of u,the power allocated to the ith subchannel is given by -(-) i=1,2,m-p+1 (0.15b) If the power allocated to the channel with the lowest gain is negative (i.e.P
7 使用。With this assumption, the constant μ is calculated (by substituting (0.11) into (0.12)) as 1 0 1 m p i i N μ P λ − + = ⎛ ⎞ ⎜ ⎟ − = ⎝ ⎠ ∑ Then we have 1 0 1 1 1 1 m p i i P N m p μ λ − + = ⎛ ⎞ = + ⎜ ⎟ − + ⎝ ⎠ ∑ (0.15a) Using this value of μ , the power allocated to the ith subchannel is given by 0 , 1,2,., 1 i i N P i mp μ λ ⎛ ⎞ = − = −+ ⎜ ⎟ ⎝ ⎠ (0.15b) If the power allocated to the channel with the lowest gain is negative (i.e., 1 0 P m p − + < ), then we discard this channel by setting 1 0 P m p − + = and return the algorithm with the iteration count p = p+1. 即迭代执行(0.15a)和(0.15b),将总功率 P 在剩余的(m-p+1)个 子信道之间进行分配。迭代计算直到获得的所有 0 Pi ≥ 或 p=m 为止
按次数下载不扣除下载券;
注册用户24小时内重复下载只扣除一次;
顺序:VIP每日次数-->可用次数-->下载券;
- 西安电子科技大学:《信息论基础》课程教学资源(学习资料)随机编码错误指数(Gallager bound).pdf
- 兰州交通大学:《通信原理》课程教学资源(实验指导)通信原理仿真实验指导.doc
- 兰州交通大学:《通信原理》课程教学大纲 Principles of Communication(负责人:伍忠东).pdf
- 兰州交通大学:《通信原理》课程各章作业习题(含参考答案).doc
- 《世界流行单片机技术手册》参考书籍(PDF)世界流行单片机技术手册——日本系列(主编:余永权,2002).pdf
- 《电子材料及元器件》参考教材PDF电子书(共十二章,含六个实验).pdf
- 兰州交通大学:《电工学》课程实验指导(打印版)电子技术实验指导.pdf
- 兰州交通大学:《电工学》课程实验指导(打印版)电工技术实验指导.pdf
- 兰州交通大学:《电工学》课程实验指导(打印版)电工电子实验指导.pdf
- 兰州交通大学:《电工学》课程授课教案(打印版)电工电子技术教案(二).pdf
- 兰州交通大学:《电工学》课程授课教案(打印版)电工电子技术教案(一).pdf
- 西安电子科技大学:《通信网络基础》课程教学资源(讲义)第五章 路由算法 5.3 最短路由算法.pdf
- 西安电子科技大学:《通信网络基础》课程教学资源(讲义)第五章 路由算法 路由算法概述.pdf
- 西安电子科技大学:《通信网络基础》课程教学资源(讲义)第四章 多址技术(多址接入协议)4.4 冲突分解算法.pdf
- 西安电子科技大学:《通信网络基础》课程教学资源(讲义)第四章 多址技术(多址接入协议)4.3.2 载波侦听型多址协议.pdf
- 西安电子科技大学:《通信网络基础》课程教学资源(讲义)第三章 网络的时延分析(时延模型)3.3.2 服务员有休假的M/G/1排队模型.pdf
- 西安电子科技大学:《通信网络基础》课程教学资源(讲义)第四章 多址技术(多址接入协议)4.2 随机多址接入协议.pdf
- 西安电子科技大学:《通信网络基础》课程教学资源(讲义)第四章 多址技术(多址接入协议)4.1 多址协议概述.pdf
- 西安电子科技大学:《通信网络基础》课程教学资源(讲义)第三章 网络的时延分析(时延模型)3.2 Basic Queue Theory.pdf
- 西安电子科技大学:《通信网络基础》课程教学资源(讲义)第三章 网络的时延分析(时延模型)3.1 Introduction to Queues and Queueing Theory.pdf
- 西安电子科技大学:《现代数字通信与编码理论》课程教学资源(讲义)Chapter 01 Introduction(主讲:白宝明).pdf
- 西安电子科技大学:《现代数字通信与编码理论》课程教学资源(讲义)信息论与编码理论基础 Introduction to Channel Coding.pdf
- 西安电子科技大学:《现代数字通信与编码理论》课程教学资源(讲义)Chapter 02 Modulation and Coding for Ideal Gaussian channels.pdf
- 西安电子科技大学:《现代数字通信与编码理论》课程教学资源(讲义)Chapter 03 Performance Bounds of Coded Communication Systems.pdf
- 西安电子科技大学:《现代数字通信与编码理论》课程教学资源(讲义)Chapter 04 Binary Capacity-Approaching Codes(4.1-4.5).pdf
- 西安电子科技大学:《现代数字通信与编码理论》课程教学资源(讲义)Chapter 04 Binary Capacity-Approaching Codes(4.6-4.7).pdf
- 西安电子科技大学:《现代数字通信与编码理论》课程教学资源(讲义)Chapter 04 Binary Capacity-Approaching Codes(4.8)Low-Density Parity-Check Codes.pdf
- 西安电子科技大学:《现代数字通信与编码理论》课程教学资源(讲义)Chapter 05 Coded-Modulation for Band-Limited AWGN Channels(5.1-5.8).pdf
- 西安电子科技大学:《现代数字通信与编码理论》课程教学资源(讲义)Polar codes.pdf
- 西安电子科技大学:《现代数字通信与编码理论》课程教学资源(讲义)Chapter 05 Coded-Modulation for Band-Limited AWGN Channels(5.9-5.14).pdf
- 西安电子科技大学:《现代数字通信与编码理论》课程教学资源(讲义)Chapter 07 Coding and Modulation for Fading Channels.pdf
- 西安电子科技大学:《现代数字通信与编码理论》课程教学资源(讲义)Chapter 08 Space-Time Coded-Modulation for Multiple Antenna Systems(for MIMO Channels).pdf
- 西安电子科技大学:《信息论与编码》课程教学资源(讲义,Information Theory and Coding,共八章,主讲:白宝明).pdf
- 石河子大学:《模拟电子技术》课程教学大纲 Analog electronic technique foundation(负责人:张宁).doc
- 石河子大学:《模拟电子技术》课程授课教案.doc
- 《模拟电子技术》课程教学资源(章节习题)半导体二极管及其应用(无答案).doc
- 《模拟电子技术》课程教学资源(章节习题)半导体三极管及其放大电路(无答案).doc
- 《模拟电子技术》课程教学资源(章节习题)场效应管及其放大电路(无答案).doc
- 《模拟电子技术》课程教学资源(章节习题)集成运算放大器(无答案).doc
- 《模拟电子技术》课程教学资源(习题集)第一章 半导体器件(含答案).doc