北京大学:《信息检索》课程教学资源(PPT课件讲稿)Essential Background
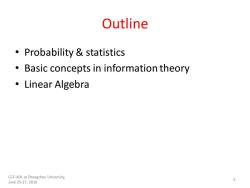
Outline Probability statistics Basic concepts in information theory 。Linear Algebra CCF-ADL at Zhengzhou University, 2 June25-27,2010
Outline • Probability & statistics • Basic concepts in information theory • Linear Algebra 2 CCF-ADL at Zhengzhou University, June 25-27, 2010
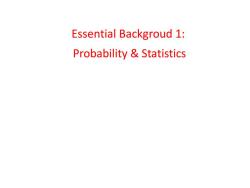
Essential Backgroud 1: Probability Statistics
Essential Backgroud 1: Probability & Statistics

Prob/Statistics Text Management Probability statistics provide a principled way to quantify the uncertainties associated with natural language Allow us to answer questions like: Given that we observe "baseball"three times and "game"once in a news article,how likely is it about "sports"? (text categorization, information retrieval) Given that a user is interested in sports news,how likely would the user use "baseball"in a query? (information retrieval) CCF-ADL at Zhengzhou University, 4 June25-27,2010
CCF-ADL at Zhengzhou University, June 25-27, 2010 4 Prob/Statistics & Text Management • Probability & statistics provide a principled way to quantify the uncertainties associated with natural language • Allow us to answer questions like: – Given that we observe “baseball” three times and “game” once in a news article, how likely is it about “sports”? (text categorization, information retrieval) – Given that a user is interested in sports news, how likely would the user use “baseball” in a query? (information retrieval)

Basic Concepts in Probability Random experiment:an experiment with uncertain outcome (e.g.,tossing a coin,picking a word from text) Sample space:all possible outcomes,e.g., Tossing 2 fair coins,S=[HH,HT,TH,TT} Event:ECS,E happens iff outcome is in E,e.g., E={HH}(all heads) E={HH,TT}(same face) Impossible event ({})certain event (S) 。Probability of Event:1≥P(E)≥0,s.t. -P(S)=1(outcome always in S) -P(AUB)=P(A)+P(B)if (AB)=(e.g.,A=same face,B=different CCF-ADLat University. 5 June25-27,2010
CCF-ADL at Zhengzhou University, June 25-27, 2010 5 Basic Concepts in Probability • Random experiment: an experiment with uncertain outcome (e.g., tossing a coin, picking a word from text) • Sample space: all possible outcomes, e.g., – Tossing 2 fair coins, S ={HH, HT, TH, TT} • Event: ES, E happens iff outcome is in E, e.g., – E={HH} (all heads) – E={HH,TT} (same face) – Impossible event ({}), certain event (S) • Probability of Event : 1P(E) 0, s.t. – P(S)=1 (outcome always in S) – P(A B)=P(A)+P(B) if (AB)= (e.g., A=same face, B=different face)
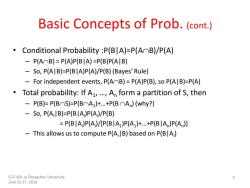
Basic Concepts of Prob.(cont.) .Conditional Probability P(B|A)=P(AB)/P(A) P(AOB)=P(A)P(BIA)=P(B)P(AIB) -So,P(A|B)=P(B|A)P(A)/P(B)(Bayes'Rule) For independent events,P(AB)=P(A)P(B),so P(A|B)=P(A) Total probability:If A1,...,An form a partition of S,then -P(B)=P(BOS)=P(BOA1)+...+P(BOAn)(why?) So,P(A;lB)=P(BIA )P(A:)/P(B) P(BIA)P(A )/[P(BIA)P(A)+...+P(BIA )P(A ) This allows us to compute P(AlB)based on P(B|A) CCF-ADL at Zhengzhou University, 6 June25-27,2010
CCF-ADL at Zhengzhou University, June 25-27, 2010 6 Basic Concepts of Prob. (cont.) • Conditional Probability :P(B|A)=P(AB)/P(A) – P(AB) = P(A)P(B|A) =P(B)P(A|B) – So, P(A|B)=P(B|A)P(A)/P(B) (Bayes’ Rule) – For independent events, P(AB) = P(A)P(B), so P(A|B)=P(A) • Total probability: If A1 , …, An form a partition of S, then – P(B)= P(BS)=P(BA1 )+…+P(B An ) (why?) – So, P(Ai|B)=P(B|Ai )P(Ai )/P(B) = P(B|Ai )P(Ai )/[P(B|A1 )P(A1 )+…+P(B|An )P(An )] – This allows us to compute P(Ai|B) based on P(B|Ai )
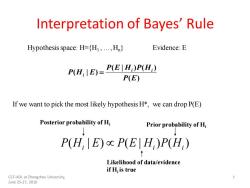
Interpretation of Bayes'Rule Hypothesis space:H=(H1,...,H) Evidence:E P(H,E)=P(EH)P(H P(E) If we want to pick the most likely hypothesis H*,we can drop P(E) Posterior probability of Hi Prior probability of Hi P(H E)P(E H)P(H) Likelihood of data/evidence if H;is true CCF-ADL at Zhengzhou University, June25-27,2010
CCF-ADL at Zhengzhou University, June 25-27, 2010 7 Interpretation of Bayes’ Rule ( ) ( | ) ( ) ( | ) P E P E H P H P H E i i i = Hypothesis space: H={H1 , …,Hn} Evidence: E If we want to pick the most likely hypothesis H*, we can drop P(E) Posterior probability of Hi Prior probability of Hi Likelihood of data/evidence if Hi is true ( | ) ( | ) ( ) P Hi E P E Hi P Hi
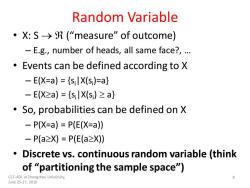
Random Variable ·X:S>gR(“measure”of outcome) -E.g.,number of heads,all same face?,... Events can be defined according to X -E(X=a)={silX(si)=a} -E(X≥a)={slX(s)≥a} So,probabilities can be defined on X -P(X=a)=P(E(X=a)) -P(a≥X)=P(E(a≥X) Discrete vs.continuous random variable(think of "partitioning the sample space") CCF-ADL at Zhengzhou University, June25-27,2010
CCF-ADL at Zhengzhou University, June 25-27, 2010 8 Random Variable • X: S → (“measure” of outcome) – E.g., number of heads, all same face?, … • Events can be defined according to X – E(X=a) = {si|X(si )=a} – E(Xa) = {si|X(si ) a} • So, probabilities can be defined on X – P(X=a) = P(E(X=a)) – P(aX) = P(E(aX)) • Discrete vs. continuous random variable (think of “partitioning the sample space”)
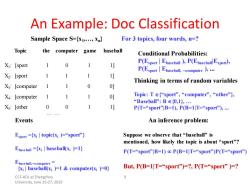
An Example:Doc Classification Sample Space S={x1,...,xn} For 3 topics,four words,n=? Topic the computer game baseball Conditional Probabilities: X:[sport 0 1] P(Esport Epaseball )P(Epaseball Espor) P(Esport|Ebaseball,computer力… X2:[sport 1 1] Thinking in terms of random variables X3:[computer 1 0] X:[computer 1 1 1 0] Topic:T∈{“sport'”,“computer”,“other'”}, “Baseball”:Be{0,l},… Xs:[other 0 0 1] P(T=“sp0rt”IB=1),P(B=1T=“sport”), Events An inference problem: Esport={在|topic(G))=“spot") Suppose we observe that“base ball'”is mentioned,how likely the topic is about "sport"? Ebaseball ={xi baseball(xi )=1) P(T=“spot”IB=1)ocPB=1T=“spot”)P(T=“sport'”) Ebase ball,computer {xi baseball(xi )=1 computer(xi )=0} But,P(B=1T=“sport'”)=?,P(T=“sport'”))=? CCF-ADL at Zhengzhou 9 University,June 25-27,2010
CCF-ADL at Zhengzhou University, June 25-27, 2010 9 An Example: Doc Classification X1 : [sport 1 0 1 1] Topic the computer game baseball X2 : [sport 1 1 1 1] X3 : [computer 1 1 0 0] X4 : [computer 1 1 1 0] X5 : [other 0 0 1 1] … … For 3 topics, four words, n=? Events Esport ={xi | topic(xi )=“sport”} Ebaseball ={xi | baseball(xi )=1} Ebaseball,computer = {xi | baseball(xi )=1 & computer(xi )=0} Sample Space S={x1 ,…, xn } Conditional Probabilities: P(Esport | Ebaseball ), P(Ebaseball|Esport), P(Esport | Ebaseball, computer ), ... An inference problem: Suppose we observe that “baseball” is mentioned, how likely the topic is about “sport”? But, P(B=1|T=“sport”)=?, P(T=“sport” )=? P(T=“sport”|B=1) P(B=1|T=“sport”)P(T=“sport”) Thinking in terms of random variables Topic: T {“sport”, “computer”, “other”}, “Baseball”: B {0,1}, … P(T=“sport”|B=1), P(B=1|T=“sport”),
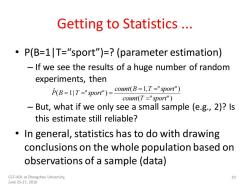
Getting to Statistics .. P(B=1T="sport")=?(parameter estimation) If we see the results of a huge number of random experiments,then P(B=1T-"sport")=cout(B=LT="sport") count(T ="sport") But,what if we only see a small sample (e.g.,2)?Is this estimate still reliable? In general,statistics has to do with drawing conclusions on the whole population based on observations of a sample(data) CCF-ADL at Zhengzhou University, 10 June25-27,2010
CCF-ADL at Zhengzhou University, June 25-27, 2010 10 Getting to Statistics ... • P(B=1|T=“sport”)=? (parameter estimation) – If we see the results of a huge number of random experiments, then – But, what if we only see a small sample (e.g., 2)? Is this estimate still reliable? • In general, statistics has to do with drawing conclusions on the whole population based on observations of a sample (data) ( " ") ( 1, " ") ( 1| " ") ˆ count T sport count B T sport P B T sport = = = = = =
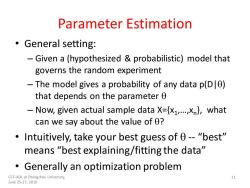
Parameter Estimation 。General setting: -Given a(hypothesized probabilistic)model that governs the random experiment -The model gives a probability of any data p(D) that depends on the parameter 0 -Now,given actual sample data X={x1,..,xn},what can we say about the value of 0? Intuitively,take your best guess of 0--"best" means“best explaining/fitting the data” Generally an optimization problem CCF-ADL at Zhengzhou University, 11 June25-27,2010
CCF-ADL at Zhengzhou University, June 25-27, 2010 11 Parameter Estimation • General setting: – Given a (hypothesized & probabilistic) model that governs the random experiment – The model gives a probability of any data p(D|) that depends on the parameter – Now, given actual sample data X={x1 ,…,xn }, what can we say about the value of ? • Intuitively, take your best guess of -- “best” means “best explaining/fitting the data” • Generally an optimization problem
按次数下载不扣除下载券;
注册用户24小时内重复下载只扣除一次;
顺序:VIP每日次数-->可用次数-->下载券;
- 北京大学:《信息检索》课程教学资源(PPT课件讲稿)Retrieval Models.ppt
- 北京大学:《信息检索》课程教学资源(PPT课件讲稿)Crawling the Web.ppt
- 北京大学:《信息检索》课程教学资源(PPT课件讲稿)Web Search.ppt
- 北京大学:《信息检索》课程教学资源(PPT课件讲稿)Course Overview(主讲:闫宏飞).ppt
- 中国科学技术大学:《人工智能基础》课程教学资源(课件讲稿)Lecture 01 Introdution(主讲:吉建民).pdf
- 中国科学技术大学:《人工智能基础》课程教学资源(课件讲稿)Lecture 15 智能机器人系统介绍.pdf
- 中国科学技术大学:《人工智能基础》课程教学资源(课件讲稿)Lecture 14 Reinforcement Learning.pdf
- 中国科学技术大学:《人工智能基础》课程教学资源(课件讲稿)Lecture 13 神经网络与深度学习.pdf
- 中国科学技术大学:《人工智能基础》课程教学资源(课件讲稿)Lecture 09 AI Planning.pdf
- 中国科学技术大学:《人工智能基础》课程教学资源(课件讲稿)Lecture 08 First-Order Logic and Inference in FOL.pdf
- 中国科学技术大学:《人工智能基础》课程教学资源(课件讲稿)Lecture 11 马尔可夫决策过程.pdf
- 中国科学技术大学:《人工智能基础》课程教学资源(课件讲稿)Lecture 10 Uncertainty and Bayesian Networks.pdf
- 中国科学技术大学:《人工智能基础》课程教学资源(课件讲稿)Lecture 07 Logical Agents.pdf
- 中国科学技术大学:《人工智能基础》课程教学资源(课件讲稿)Lecture 06 Game Playing.pdf
- 中国科学技术大学:《人工智能基础》课程教学资源(课件讲稿)Lecture 05 Constraint Satisfaction Problems.pdf
- 中国科学技术大学:《人工智能基础》课程教学资源(课件讲稿)Lecture 04 Informed Search.pdf
- 中国科学技术大学:《人工智能基础》课程教学资源(课件讲稿)Lecture 03 Solving Problems by Searching.pdf
- 中国科学技术大学:《人工智能基础》课程教学资源(课件讲稿)Lecture 02 Intelligent Agents.pdf
- 《Artificial Intelligence:A Modern Approach》教学资源(PPT课件,英文版)Chapter 9-Inference in first-order logic.ppt
- 《Artificial Intelligence:A Modern Approach》教学资源(PPT课件,英文版)Chapter 8-First-Order Logic.ppt
- 哈尔滨工业大学:《信息检索》课程教学资源(课件讲义)文本分类 Text Categorization(主讲:刘挺).pdf
- 哈尔滨工业大学:《信息检索》课程教学资源(课件讲义)信息过滤(主讲:刘挺).pdf
- 哈尔滨工业大学:《信息检索》课程教学资源(课件讲义)信息检索模型 IRModel.pdf
- 哈尔滨工业大学:《信息检索》课程教学资源(课件讲义)信息检索概述.pdf
- 哈尔滨工业大学:《信息检索》课程教学资源(课件讲义)搜索引擎技术 SearchEngine.pdf
- 《统计自然语言处理》课程教学资源(PPT课件讲稿)第7章 汉语自动分词与词性标注.ppt
- 北京大学:《信息检索》课程PPT课件讲稿(自然语言处理)01 Introduction(主讲:彭波)The CCF Advanced Disciplines Lectures.ppt
- 北京大学:《信息检索》课程PPT课件讲稿(自然语言处理)02 Link Analysis.ppt
- 北京大学:《信息检索》课程PPT课件讲稿(自然语言处理)03 Web Spam.ppt
- 北京大学:《信息检索》课程PPT课件讲稿(自然语言处理)04 Recommendation System.ppt
- 北京大学:《信息检索》课程PPT课件讲稿(自然语言处理)05 Infrastructure and Cloud.ppt
- 河南科技学院:信息工程学院本科课程教学大纲汇编(计算机科学与技术专业).pdf
- 广东茂名农林科技职业学院:计算机网络技术人才培养方案(2020级).pdf
- 广东茂名农林科技职业学院:计算机网络技术专业人才培养方案(2021级).pdf
- 广东茂名农林科技职业学院:动漫制作技术专业人才培养方案(2020级).pdf
- 南京农业大学:《面向对象程序设计实验》课程教学大纲 Experiment in Object-Oriented Programming.pdf
- 广东茂名农林科技职业学院:电子商务专业人才培养方案(2019级).pdf
- 中国科学技术大学:《数据库基础》课程教学资源(PPT课件讲稿)第一章 绪论(主讲:袁平波).pps
- 中国科学技术大学:《数据库基础》课程教学资源(PPT课件讲稿)第二章 关系数据库.pps
- 中国科学技术大学:《数据库基础》课程教学资源(PPT课件讲稿)第四章 关系数据库设计理论.pps