上海交通大学:《随机模拟方法与应用 Stochastic Simulation Methods and Its Applications》课程教学资源(学生作业)Distribution Analysis of Spring Festival Travel Based on Gravity Model and MCMC Methodology
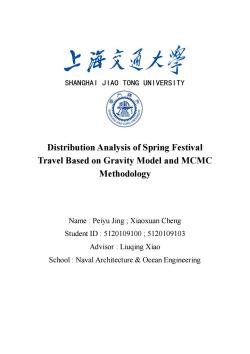
上游充通大 SHANGHAI JIAO TONG UNIVERSITY 卧h IAO TONG Distribution Analysis of Spring Festival Travel Based on Gravity Model and MCMC Methodology Name Peiyu Jing Xiaoxuan Cheng Student ID:5120109100;5120109103 Advisor Liuqing Xiao School Naval Architecture Ocean Engineering
SHANGHAI JIAO TONG UNIVERSITY Distribution Analysis of Spring Festival Travel Based on Gravity Model and MCMC Methodology Name : Peiyu Jing ; Xiaoxuan Cheng Student ID : 5120109100 ; 5120109103 Advisor : Liuqing Xiao School : Naval Architecture & Ocean Engineering

Distribution Analysis of Spring Festival Travel Based on Gravity Model and MCMC Methodology ABSTRACT Spring Festival travel,the largest national transportation activity in China,has reached its summit in the number of man-time transmitted during 2014 Lunar Chinese New Year.With the application of"Baidu Migration",a Location-Based Social Networking backed by Baidu Positioning,we were enabled to analyze certain numerical features of the traffic flows,which were utilized in this paper to establish an origin/destination matrix by unit of city.We selected Beijing,Shanghai,and Guangzhou as cells in the matrix and regarded positioning data collected as traffic volumes between locations.Three forms of gravity model (i.e.,basic gravity model,modified gravity model,and doubly restrained gravity model)were discussed respectively to develop the matrices.To calibrate the coefficients,we used the least square method(LSM)and trial method.We finally generated O/D prediction matrices with three different models but statistical tests invalidated the basic gravity model for this application. Further,with hypothetical data,we proposed possible usage of Markov Chain Monte Carlo (MCMC)methodology for predicting trip attractions to the three cities.These attempts may well provide valuable transportation information for the coming Spring Festival travel. However,the insufficiency of accessible data could have compromised the accuracy of the analysis,which requires further discussion with the collection of a larger number of traffic data as well as an improved methodology. Key Words:Spring Festival travel;Gravity Model;Origin/Destination Matrix;MCMC methodology 1.INTRODUCTION Spring Festival travel,the largest national transportation activity in China,has reached its summit in the number of man-time transmitted during 2014 Lunar Chinese New Year.In February,2014,China's Development and Reform Commission announced that the total traffic volume was approximately 3.62 billion man-time,which was 0.2 billion more than the number last year.The surprising large-scale migration lasted from January 16th to February 24h.During the forty days,it was estimated that national traffic volume of highway transportation,waterborne transportation,railway transportation,and air transportation was 3.2 billion,0.043 billion,0.2578 billion,and 0.042 billion man-time respectively,all presenting increases in number During 2014 Spring Festival,Baidu publicized a commercial application platform named "Baidu Migration".On the basis of"Baidu Map:Spring Festival Population Migration Big
1 Distribution Analysis of Spring Festival Travel Based on Gravity Model and MCMC Methodology ABSTRACT Spring Festival travel, the largest national transportation activity in China, has reached its summit in the number of man-time transmitted during 2014 Lunar Chinese New Year. With the application of “Baidu Migration”, a Location-Based Social Networking backed by Baidu Positioning, we were enabled to analyze certain numerical features of the traffic flows, which were utilized in this paper to establish an origin/destination matrix by unit of city. We selected Beijing, Shanghai, and Guangzhou as cells in the matrix and regarded positioning data collected as traffic volumes between locations. Three forms of gravity model (i.e., basic gravity model, modified gravity model, and doubly restrained gravity model) were discussed respectively to develop the matrices. To calibrate the coefficients, we used the least square method (LSM) and trial method. We finally generated O/D prediction matrices with three different models but statistical tests invalidated the basic gravity model for this application. Further, with hypothetical data, we proposed possible usage of Markov Chain Monte Carlo (MCMC) methodology for predicting trip attractions to the three cities. These attempts may well provide valuable transportation information for the coming Spring Festival travel. However, the insufficiency of accessible data could have compromised the accuracy of the analysis, which requires further discussion with the collection of a larger number of traffic data as well as an improved methodology. Key Words: Spring Festival travel; Gravity Model; Origin/Destination Matrix; MCMC methodology 1. INTRODUCTION Spring Festival travel, the largest national transportation activity in China, has reached its summit in the number of man-time transmitted during 2014 Lunar Chinese New Year. In February, 2014, China’s Development and Reform Commission announced that the total traffic volume was approximately 3.62 billion man-time, which was 0.2 billion more than the number last year. The surprising large-scale migration lasted from January 16th to February 24th. During the forty days, it was estimated that national traffic volume of highway transportation, waterborne transportation, railway transportation, and air transportation was 3.2 billion, 0.043 billion, 0.2578 billion, and 0.042 billion man-time respectively, all presenting increases in number [1] . During 2014 Spring Festival, Baidu publicized a commercial application platform named “Baidu Migration”. On the basis of “Baidu Map: Spring Festival Population Migration Big
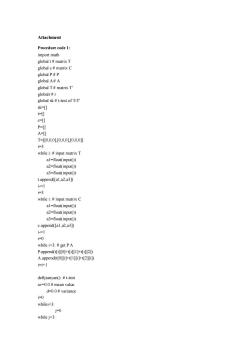
Attachment Procedure code 1: import math global t#matrix T global c#matrix C global P#P global A#A global T#matrix T globalr #r global sk t-test of T-T' sk=[] =0 c=0 P=0 A=0 T=[0,0,01,0,0,01,0,0,0] i=3 while i:input matrix T al=float(input()) a2=float(input()) a3=float(input()) t.append[al,a2,a3]) i=1 i=3 while i:input matrix C al=float(input()) a2=float(input()) a3=float(input()) c.append([al,a2,a3]) i=1 0 while i<3:get PA P.append(t[i][0]+ti][1]+t[i][2)) A.append(t[o][i]+t[1][i]+t[2]i]) i=计1 deftjianyan():t-test av=0.0 mean value d=0.0#variance =0 whilei<3: j=0 while j<3:
Attachment Procedure code 1: import math global t # matrix T global c # matrix C global P # P global A # A global T # matrix T' globalr # r global sk # t-test of T-T' sk=[] t=[] c=[] P=[] A=[] T=[[0,0,0],[0,0,0],[0,0,0]] i=3 while i: # input matrix T a1=float(input()) a2=float(input()) a3=float(input()) t.append([a1,a2,a3]) i-=1 i=3 while i: # input matrix C a1=float(input()) a2=float(input()) a3=float(input()) c.append([a1,a2,a3]) i-=1 i=0 while i<3: # get P A P.append(t[i][0]+t[i][1]+t[i][2]) A.append(t[0][i]+t[1][i]+t[2][i]) i=i+1 deftjianyan(): # t-test av=0.0 # mean value d=0.0 # variance i=0 whilei<3: j=0 while j<3:

if i=j:except the leading diagonal j+1 continue sk.append(t[i][j]-T[i]j]) av+=t(i](j]-T[i](j] j+=1 i+=1 i=0 av/=6#mean value whilei0.05 Or abs(k][t]-k][(t+1)%2]/k][tt)>0.05: tt=(tt+1)%2 k[[(tt+1)%2]=t[[]*P*A]/cc/k][t] k][(t+1)%2]=t0]*P*A]/cc/k[[(t+1)%2] T[0]=P[*A]*cc/k[[t/k][t] j+=1 i+=1 iftjianyan()-0: return 0
if i==j: # except the leading diagonal j+=1 continue sk.append(t[i][j]-T[i][j]) av+=t[i][j]-T[i][j] j+=1 i+=1 i=0 av/=6 # mean value whilei0.05 or abs((k[j][tt]-k[j][(tt+1)%2])/k[j][tt])>0.05: tt=(tt+1)%2 k[i][(tt+1)%2]=t[i][j]*P[i]*A[j]/cc/k[j][tt] k[j][(tt+1)%2]=t[i][j]*P[i]*A[j]/cc/k[i][(tt+1)%2] T[i][j]=P[i]*A[j]*cc/k[i][tt]/k[j][tt] j+=1 i+=1 iftjianyan()==0: return 0
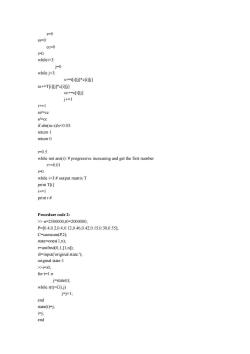
S=0 ss=0 cc=0 =0 whilei>n=2500000:t0=2000000: P=0.4,0.2,0.4,0.12,0.46,0.42,0.15,0.30,0.551 C=cumsum(P,2); state=ones(1,n); r=unifrnd(0,1,1,n) i0=input('original state:) original state:I >i=i0: for t=l:n j=state(t): while r(t)>C(i,j) j-j+l; end state(t)=j; i end
s=0 ss=0 cc=0 i=0 whilei> n=2500000;t0=2000000; P=[0.4,0.2,0.4;0.12,0.46,0.42;0.15,0.30,0.55]; C=cumsum(P,2); state=ones(1,n); r=unifrnd(0,1,[1,n]); i0=input('original state:'); original state:1 >>i=i0; for t=1:n j=state(t); while r(t)>C(i,j) j=j+1; end state(t)=j; i=j; end

h=hist(state(to+1:n),[1,2,3]); p=h/(n-t0) p= 0.1852 0.3369 0.4779
h=hist(state(t0+1:n),[1,2,3]); p=h/(n-t0) p = 0.1852 0.3369 0.4779
按次数下载不扣除下载券;
注册用户24小时内重复下载只扣除一次;
顺序:VIP每日次数-->可用次数-->下载券;
- 《随机模拟方法与应用 Stochastic Simulation Methods and Its Applications》教学资源(论文资料)09 基于Bayesian-MCMC方法的水体污染识别反问题.pdf
- 《随机模拟方法与应用 Stochastic Simulation Methods and Its Applications》教学资源(论文资料)08 基于AM-MCMC算法的贝叶斯概率洪水预报模型(东北农业大学、河海大学).pdf
- 《随机模拟方法与应用 Stochastic Simulation Methods and Its Applications》教学资源(论文资料)07 国内外非同步期货交易市场之间的跳跃溢出行为——基于风险事件的视角(复旦大学).pdf
- 《随机模拟方法与应用 Stochastic Simulation Methods and Its Applications》教学资源(论文资料)06 管理浮动机制下的人民币汇率行为分析——基于贝叶斯MCMC推断法与ESVDJ模型(厦门大学、中山大学).pdf
- 《随机模拟方法与应用 Stochastic Simulation Methods and Its Applications》教学资源(论文资料)60 IRT模型参数估计的新方法——MCMC算法(江西师范大学).pdf
- 《随机模拟方法与应用 Stochastic Simulation Methods and Its Applications》教学资源(论文资料)05 Metropolis-Hastings自适应算法及其应用(东南大学、俄亥俄州立大学).pdf
- 《随机模拟方法与应用 Stochastic Simulation Methods and Its Applications》教学资源(论文资料)59 小样本下两阶段MCMC参数估计方法——基于信用风险强度模型的研究(大连理工大学).pdf
- 《随机模拟方法与应用 Stochastic Simulation Methods and Its Applications》教学资源(论文资料)58 MCMC方法下最优Copula的估计及选取(安徽财经大学).pdf
- 《随机模拟方法与应用 Stochastic Simulation Methods and Its Applications》教学资源(论文资料)57 Bayes网络学习的MCMC方法(西安电子科技大学).pdf
- 《随机模拟方法与应用 Stochastic Simulation Methods and Its Applications》教学资源(论文资料)56 基于广义极值分布和Metropolis-Hastings抽样算法的贝叶斯MCMC洪水频率分析方法.pdf
- 《随机模拟方法与应用 Stochastic Simulation Methods and Its Applications》教学资源(论文资料)55 全源NT技术的接入网链路丢包率推断(西北工业大学计算机学院).pdf
- 《随机模拟方法与应用 Stochastic Simulation Methods and Its Applications》教学资源(论文资料)54 双指数跳跃扩散模型的McMC估计.pdf
- 《随机模拟方法与应用 Stochastic Simulation Methods and Its Applications》教学资源(论文资料)53 树木位置空间模式建模与预测(东北林业大学).pdf
- 《随机模拟方法与应用 Stochastic Simulation Methods and Its Applications》教学资源(论文资料)52 正态逆高斯扩散模型的MCMC估计(天津大学管理学院).pdf
- 《随机模拟方法与应用 Stochastic Simulation Methods and Its Applications》教学资源(论文资料)51 一种基于MCMC稳态模拟的贝叶斯索赔校正模型(南京理工大学、湖南大学).pdf
- 《随机模拟方法与应用 Stochastic Simulation Methods and Its Applications》教学资源(论文资料)50 一种基于IS-MCMC的多目标跟踪算法(国防科学技术大学).pdf
- 《随机模拟方法与应用 Stochastic Simulation Methods and Its Applications》教学资源(论文资料)04 MCMC粒子滤波的GPS定位数据处理算法(沈阳航空航天大学).pdf
- 《随机模拟方法与应用 Stochastic Simulation Methods and Its Applications》教学资源(论文资料)49 研制阶段系统可靠性增长的Bayesian评估与预测.pdf
- 《随机模拟方法与应用 Stochastic Simulation Methods and Its Applications》教学资源(论文资料)48 中国商业银行操作风险损失分布甄别与分析——基于贝叶斯MCMC频率方法.pdf
- 《随机模拟方法与应用 Stochastic Simulation Methods and Its Applications》教学资源(论文资料)47 我国能源消费需求的时变弹性分析(西安交通大学:刘东霖、张俊瑞).pdf
- 上海交通大学:《随机模拟方法与应用 Stochastic Simulation Methods and Its Applications》课程教学资源(学生作业)LOL战绩铭——李金滨.doc
- 上海交通大学:《随机模拟方法与应用 Stochastic Simulation Methods and Its Applications》课程教学资源(学生作业)MCMC方法求解生物逆问题——周东旭.pdf
- 上海交通大学:《随机模拟方法与应用 Stochastic Simulation Methods and Its Applications》课程教学资源(学生作业)基于GA-MCMC的粒子滤波图像恢复算法——胡卓然、吴迪舟、焦一航.docx
- 上海交通大学:《随机模拟方法与应用 Stochastic Simulation Methods and Its Applications》课程教学资源(学生作业)基于MCMC方法的中国象棋等级分系统模型分析——梁久阳,谭明.pdf
- 上海交通大学:《随机模拟方法与应用 Stochastic Simulation Methods and Its Applications》课程教学资源(学生作业)基于MCMC的创业公司估值问题研究——马欣楠.pdf
- 上海交通大学:《随机模拟方法与应用 Stochastic Simulation Methods and Its Applications》课程教学资源(学生作业)基于元胞自动机的车载设备对驾驶员行为影响的研究——王浩衡.doc
- 上海交通大学:《随机模拟方法与应用 Stochastic Simulation Methods and Its Applications》课程教学资源(学生作业)基于控制变量方法的蒙特卡洛方法探究及其在期权定价中的应用——王思远.pdf
- 上海交通大学:《随机模拟方法与应用 Stochastic Simulation Methods and Its Applications》课程教学资源(学生作业)对MCMC 方法在瑞利分布样本采集及一元线性回归模型参数估计中应用的思考与研究——吴凯斌.pdf
- 上海交通大学:《随机模拟方法与应用 Stochastic Simulation Methods and Its Applications》课程教学资源(学生作业)对流-扩散方程源项识别反问题的MCMC方法论文研读——孙密广.doc
- 上海交通大学:《随机模拟方法与应用 Stochastic Simulation Methods and Its Applications》课程教学资源(学生作业)非合作博弈与纳什均衡——李忠睿 王大伟 张正强.docx
- 上海交通大学:《随机模拟方法与应用 Stochastic Simulation Methods and Its Applications》课程教学资源(学生作业)音乐类型的数学统计——周逸芃.pdf
- 上海交通大学:《数学与科技进步》课程教学资源(参考文献)从“格致”到“科学”.pdf
- 《数学与科技进步》课程教学资源参考文献:《数学文化论十九讲》PDF电子书(孔令兵).pdf
- 上海交通大学:《数学与科技进步》课程教学资源(教学PPT)第1、2、3、4、5章(沈灏).ppt
- 上海交通大学:《数学与科技进步》课程教学资源(教学PPT)第1、2、3、4、5、6、7章.ppt
- 上海交通大学:《数学与科技进步》课程教学资源(参考资料)数学家言行录.docx
- 上海交通大学:《数学与科技进步》课程教学资源(参考资料)读书摘录——中国近三百年学术史(梁启超).docx
- 上海交通大学:《数学与科技进步》课程教学资源(参考资料)读书摘录——中国近三百年学术史(梁启超).docx
- 《微分方程》课程教学资源(书籍资料)DENNIS G. ZILL&MICHAEL R. CULLEN《Differential Equations with Boundary-Value Problems》(SEVENTH EDITION).pdf
- 《微分方程》课程教学资源(电子书籍)NAKHLE H.ASMAR《Partial Differential Equations》with FOURIER SERIES and BOUNDARY VALUE PROBLEMS(Second Edition).pdf