电子科技大学:《信号检测与估计 Signal Detection and Estimation》课程教学资源(课件讲稿)Chapter 09、10 Bayesian Approach
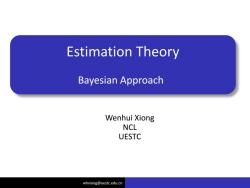
Estimation Theory Bayesian Approach Wenhui Xiong NCL UESTC whxiong@uestc.edu.cn
whxiong@uestc.edu.cn Estimation Theory Bayesian Approach Wenhui Xiong NCL UESTC

Review Estimator CRLB→factorize alnp(x:0) 80 =I(9)(g(x)-8) RBLS: .Use sufficient statistics:T(x)ET(] unbiased estimator over sufficient statistics:g[T(x)] ● BLUE:if the unknown is a linear function of the data ●MLE:known PDF 。Least Square Assumption:unknown is deterministic whxiong@uestc.edu.cn 2
whxiong@uestc.edu.cn Review Estimator 2 CRLB factorize RBLS: Use sufficient statistics: T(x) unbiased estimator over sufficient statistics: g[T(x)] BLUE: if the unknown is a linear function of the data MLE: known PDF Least Square E[µ · jT(x)] Assumption: unknown is deterministic @lnp(x; µ) @µ = I(µ)(g(x) ¡ µ)
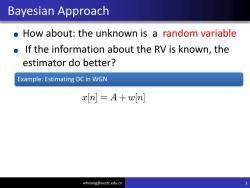
Bayesian Approach How about:the unknown is a random variable 0 If the information about the rV is known,the estimator do better? Example:Estimating DC in WGN cn =A+wln whxiong@uestc.edu.cn 3
whxiong@uestc.edu.cn 3 Bayesian Approach How about: the unknown is a random variable If the information about the RV is known, the estimator do better? Example: Estimating DC in WGN x[n] = A + w[n]

Bayesian Approach How about:the unknown is a random variable If the information about the RV is known,the estimator do better? Example:Estimating DC in WGN xIn =A+wln 。MVU:sample mean A=元AW(A,σ2/N) whxiong@uestc.edu.cn 4
whxiong@uestc.edu.cn 4 Bayesian Approach How about: the unknown is a random variable If the information about the RV is known, the estimator do better? Example: Estimating DC in WGN x[n] = A + w[n] MVU: sample mean A b = x¹ A ^ » N(A; ¾ 2 =N)
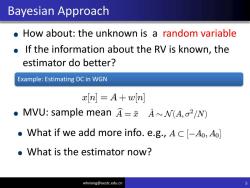
Bayesian Approach How about:the unknown is a random variable If the information about the RV is known,the estimator do better? Example:Estimating DC in WGN xn =A+wln 。MVU:sample mean A=元A~W(A,o2/N) .What if we add more info.e.g.,AC[-A0,Ao] What is the estimator now? whxiong@uestc.edu.cn 5
whxiong@uestc.edu.cn 5 Bayesian Approach How about: the unknown is a random variable If the information about the RV is known, the estimator do better? Example: Estimating DC in WGN x[n] = A + w[n] MVU: sample mean A b = x¹ A ^ » N(A; ¾ 2 =N) What if we add more info. e.g., A ½ [¡A0;A0] What is the estimator now?
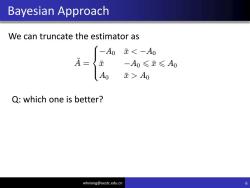
Bayesian Approach We can truncate the estimator as A0元A0 Q:which one is better? whxiong@uestc.edu.cn 6
whxiong@uestc.edu.cn Bayesian Approach 6 We can truncate the estimator as A¸ = 8 >: ¡A0 x¹ A0 Q: which one is better?
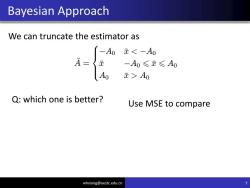
Bayesian Approach We can truncate the estimator as A0元A0 Q:which one is better? Use MSE to compare whxiong@uestc.edu.cn
whxiong@uestc.edu.cn Bayesian Approach 7 We can truncate the estimator as A¸ = 8 >: ¡A0 x¹ A0 Q: which one is better? Use MSE to compare
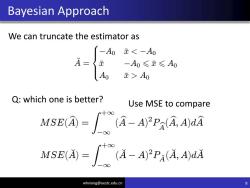
Bayesian Approach We can truncate the estimator as -A0元A0 Q:which one is better? Use MSE to compare MsE④=(④-A2Paa,AdA MSE(A)=(A-A)2PA(A,A)dA whxiong@uestc.edu.cn 8
whxiong@uestc.edu.cn Bayesian Approach 8 We can truncate the estimator as MSE(A b ) = Z +1 ¡1 (A b ¡ A) 2 PA b (A b ; A)dA b A¸ = 8 >: ¡A0 x¹ A0 Q: which one is better? MSE(A ¸ ) = Z +1 ¡1 (A ¸¡ A) 2 PA¸(A ¸ ; A)dA ¸ Use MSE to compare
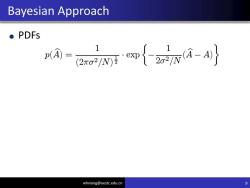
Bayesian Approach 。PDFS i=27a{xA- whxiong@uestc.edu.cn 9
whxiong@uestc.edu.cn PDFs Bayesian Approach 9 p(A b ) = 1 (2¼¾2 =N) 1 2 ¢exp ½ ¡ 1 2¾2 =N (A b ¡ A) ¾
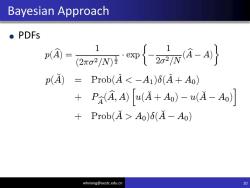
Bayesian Approach 。PDFS () m{wi p(A)Prob(AAo)6(A-Ao)) whxiong@uestc.edu.cn 10
whxiong@uestc.edu.cn PDFs Bayesian Approach 10 p(A b ) = 1 (2¼¾2 =N) 1 2 ¢exp ½ ¡ 1 2¾2 =N (A b ¡ A) ¾ p(A¸) = Prob(A· A0)±(A¸¡ A0)
按次数下载不扣除下载券;
注册用户24小时内重复下载只扣除一次;
顺序:VIP每日次数-->可用次数-->下载券;
- 电子科技大学:《信号检测与估计 Signal Detection and Estimation》课程教学资源(课件讲稿)Chapter 08 Least Square(LS).pdf
- 电子科技大学:《信号检测与估计 Signal Detection and Estimation》课程教学资源(课件讲稿)Chapter 07 Maximum Likelihood Estimator(MLE).pdf
- 电子科技大学:《信号检测与估计 Signal Detection and Estimation》课程教学资源(课件讲稿)Chapter 06 Statistical Detection Theory II.pdf
- 电子科技大学:《信号检测与估计 Signal Detection and Estimation》课程教学资源(课件讲稿)Chapter 05 Random Signal.pdf
- 电子科技大学:《信号检测与估计 Signal Detection and Estimation》课程教学资源(课件讲稿)Chapter 04 Deterministic Signal.pdf
- 电子科技大学:《信号检测与估计 Signal Detection and Estimation》课程教学资源(课件讲稿)Chapter 03 Statistical Detection Theory I.pdf
- 电子科技大学:《中外新闻传播学史 History of Journalism and Communication of China and Foreign Countries》课程教学资源(教学大纲).pdf
- 运城学院:《广播电视概论》课程教学资源(教学大纲,打印版)播音与主持艺术.pdf
- 运城学院:《广播电视概论》课程教学资源(教学大纲,打印版)播音与主持艺术专升本.pdf
- 运城学院:《广播电视概论》课程教学大纲 Radio and television introduction(播音与主持艺术,打印版).pdf
- 运城学院:《广播电视概论》课程教学资源(电子教案,打印版)第九章 广播电视综艺娱乐类节目.pdf
- 运城学院:《广播电视概论》课程教学资源(电子教案,打印版)第八章 广播电视谈话节目.pdf
- 运城学院:《广播电视概论》课程教学资源(电子教案,打印版)第七章 广播电视新闻类节目.pdf
- 运城学院:《广播电视概论》课程教学资源(电子教案,打印版)第六章广播电视传播的语言教案.pdf
- 运城学院:《广播电视概论》课程教学资源(电子教案,打印版)第五章 广播电视传播符号.pdf
- 运城学院:《广播电视概论》课程教学资源(电子教案,打印版)第四章 世界广播电视的体制与发展.pdf
- 运城学院:《广播电视概论》课程教学资源(电子教案,打印版)第三章_港澳台地区广播电视事业发展.pdf
- 运城学院:《广播电视概论》课程教学资源(电子教案,打印版)第二章_中国广播电视事业发展.pdf
- 运城学院:《广播电视概论》课程教学资源(电子教案,打印版)第一章 广播电视发展与现状.pdf
- 长沙医学院:人文传媒学院课程简介.pdf
- 电子科技大学:《信号检测与估计 Signal Detection and Estimation》课程教学资源(课件讲稿)绪论(熊文汇).pdf
- 电子科技大学:《新媒体研究 New Media Studies》课程教学资源(教学大纲,主讲:韩洪).pdf
- 电子科技大学:《新媒体研究 New Media Studies》课程教学资源(课件讲稿,共五部分,主讲:韩洪).pdf
- 电子科技大学:《新闻学理论 Journalism Thoery》课程教学资源(教学大纲,詹恂).pdf
- 电子科技大学:《新闻学理论 Journalism Thoery》课程教学资源(课件讲稿,詹恂).pdf
- 运城学院:《新闻学概论》课程教学资源(教学大纲)Introduction to Journalism(负责人:贠琪).doc
- 《新闻学概论》课程教学资源(参考文献)列宁——党的组织和党的出版物.doc
- 《新闻学概论》课程教学资源(参考文献)马克思恩格斯——摩泽尔记者的辩护.doc
- 《新闻学概论》课程教学资源(参考文献)马克思——第六届莱茵省议会的辩论(第一篇论文).doc
- 《新闻学概论》课程教学资源(参考文献)马克思——对波拿巴的谋杀.doc
- 《新闻学概论》课程教学资源(参考文献)我们对于新闻学的基本观点(陆定一).doc
- 《新闻学概论》课程教学资源(参考文献)马克思——评普鲁士最近的书报检查令.doc
- 《新闻学概论》课程教学资源(参考文献)毛泽东在延安文艺座谈会上的讲话.doc
- 成都大学:文学与新闻传播学院汉语国际教育专业课程教学大纲(汇编).pdf
- 成都大学:文学与新闻传播学院广播电视学专业课程教学大纲(汇编).pdf
- 成都大学:文学与新闻传播学院网络与新媒体专业课程教学大纲(汇编).pdf
- 成都大学:文学与新闻传播学院汉语言文学专业课程教学大纲(汇编).pdf
- 成都大学:影视与动画学院动画专业课程教学大纲合集(汇编).pdf
- 成都大学:影视与动画学院广播电视编导专业课程教学大纲(合集).pdf
- 国家开放大学:2016年春季学期“开放专科”广告(设计与制作)专业广告学概论期末试题(开卷).pdf