南京大学:《概率与计算 Probability and Computing》课程教学资源(课件讲稿)random12

Mixing Time Markoy chain:t=(,P) ● mixing time:time to be close to the stationary distribution
Mixing Time Markov chain: M = (⌦, P) • mixing time: time to be close to the stationary distribution
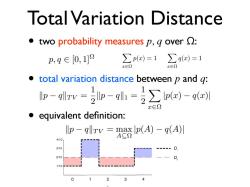
Total Variation Distance two probability measures p,g over p,9∈[0,1]0 ∑p(x)=1∑q(c)=1 x∈2 x∈2 total variation distance between p and g: Ilp-allrv jllp-all >lp(z)-a(s) x∈2 equivalent definition: ID-grv=Rlp(A)-g(A 4/10 3/10 D 2/10 1/10 0 2 3 4
Total Variation Distance • two probability measures p, q over Ω: • total variation distance between p and q: • equivalent definition: p, q 2 [0, 1]⌦ X x2⌦ p(x)=1 X x2⌦ q(x)=1 kp qkT V = 1 2 kp qk1 = 1 2 X x2⌦ |p(x) q(x)| COUPLING OF MARKOV CHAINS 4/10 r-----. I I I I I I r----- I I I - + - -:- --- -{ -- I I I ____ -' L __________ I 3/10 2/10 1/10 o 2 3 4 x Figure 11.1: Example of variation distance. The areas shaded by upward diagonal lines correspond to values x where DI (x) D 2(x). The total area shaded by upward diagonal lines must equal the total area shaded by downward diagonal lines, and the variation distance equals one of these two areas. we run the chain for a finite number of steps. If we want to use this Markov chain to shuffle the deck, how many steps are necessary before we obtain a shuffle that is to uniformly distributed? To quantify what we mean by "close to uniform", we must introduce a distance measure. Definition 11.1: The variation distance between two distributions D, and D2 on (f countable state space S is given by 1 liD, - D211 = 2: LID,(x) - D2(x)l. XES A pictorial example of the variation distance is given in Figure 11.1. The factor 1/2 in the definition of variation distance guarantees that the variation distance is between 0 and l. It also allows the following useful alternative characterization. Lemma 11.1: Foran), A S, let Di(A) = LXEA Di(x)jori = 1,2. Then A careful examination of Figure 11.1 helps make the proof of this lemma transparent. Proof: Let S+ S be the set of states such that D,(x) D2(X), and let S- S the set of states such that D2 (x) > D, (x). Clearly, and But since D,(S) = D2(S) = 1, we have D,(S+) + D,(S-) = D2(S+) + D2(S-) = 1, 272 kp qkT V = max A✓⌦ |p(A) q(A)|

Mixing Time Markov chain:t=(,P) stationary distribution: p):distribution at time when initial state is △(t)=lp-π‖Tv △(t)=max△x(t) x∈2 Tx(e)=min{t|△x(t)≤e}T(e)=max T(e) x∈2 mixing time: Tmix =T(1/2e) rapid mixing: Tmix=(log2)0(1) △(k·Tmix)≤e-and(e)≤Tmix, In
Mixing Time Markov chain: M = (⌦, P) • mixing time: x(t) = kp(t) x ⇡kT V (t) = max x2⌦ x(t) ⌧x(✏) = min{t | x(t) ✏} ⌧ (✏) = max x2⌦ ⌧x(✏) ⌧mix = ⌧ (1/2e) stationary distribution: ⇡ p(t) x : distribution at time t when initial state is x rapid mixing: ⌧mix = (log |⌦|) O(1) (k · ⌧mix) ek ⌧ (✏) ⌧mix · ⇠ ln 1 ✏ ⇡ and
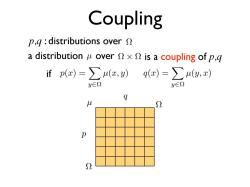
Coupling p,g:distributions over a distribution u over x is a coupling of p,q ifp(x)=>μ(,) q(c)=∑(y,x) y∈2 y∈2 9 0 2 p 2
Coupling p(x) = X y2⌦ µ(x, y) q(x) = X y2⌦ µ(y, x) a distribution µ over ⌦ ⇥ ⌦ is a coupling of p,q p,q : distributions over ⌦ if µ ⌦ ⌦ p q

Coupling Lemma Coupling Lemma 1.(X,Y)is a coupling of p,q>Pr[X≠Y]≥lp-qlrv 2.3 a coupling (X,Y)of p,g s.t.Pr[XY]=llp-allrv p() g(x) x
Coupling Lemma 1. (X,Y) is a coupling of p,q Pr[X 6= Y ] kp qkT V 2. ∃ a coupling (X,Y) of p,q s.t. Pr[X 6= Y ] = kp qkT V Coupling Lemma
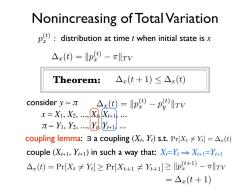
Nonincreasing of Total Variation p):distribution at time t when initial state isx △z(t)=lp-πlrv Theorem: △x(t+1)≤△x(t) consider y~π △(=lp-prv x=X1 X2,....Xn X+1 -Y1,Y2,...YY coupling lemma:3 a coupling (Xi,Y)s.t.Pr[X]=A(t) couple (X1,Y+1)in such a way that:X=YX=Y △x(t)=Pr[X≠Y≥Pr[X+1≠Y+]≥lp+D-πlTv =△x(t+1)
Nonincreasing of Total Variation x(t) = kp(t) x ⇡kT V p(t) x : distribution at time t when initial state is x Theorem: x(t + 1) x(t) consider y ∼ π x(t) = kp(t) x p(t) y kT V coupling lemma: ∃ a coupling (Xt, Yt) s.t. x = X1, X2, ..., Xt, Xt+1, ... π ∼ Y1, Y2, ..., Yt, Yt+1, ... Pr[Xt 6= Yt] = x(t) couple (Xt+1, Yt+1) in such a way that: Xt=Yt ⇒ Xt+1=Yt+1 x(t) = Pr[Xt 6= Yt] Pr[Xt+1 6= Yt+1] kp(t+1) x ⇡kT V = x(t + 1)
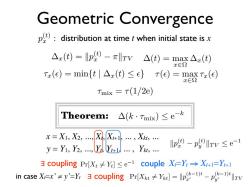
Geometric Convergence p):distribution at time twhen initial state isx △(t)=lp-πlTv△(t)=max△x(t) x∈2 Tx(e)=min{t|△x(t)≤e}r(e)=max Ta(e) x∈2 Tmix =T(1/2e) Theorem:△(k,Tmix)≤ek 三X22%w-g9vse x =X1,X2,... 3 coupling Pr[Xt≠YAl≤e-1 couple X=Y,→X+1=Y+l in caseX=r'≠y'=y子coupling P≠Yl=lpg-t-pg-tIlr
Geometric Convergence x(t) = kp(t) x ⇡kT V p(t) x : distribution at time t when initial state is x Theorem: (k · ⌧mix) ek (t) = max x2⌦ x(t) ⌧x(✏) = min{t | x(t) ✏} ⌧ (✏) = max x2⌦ ⌧x(✏) ⌧mix = ⌧ (1/2e) x = X1, X2, ..., Xt, Xt+1, ... , Xkt, ... y = Y1, Y2, ..., Yt, Yt+1, ... , Ykt, ... kp(t) x p(t) y kT V e1 Pr[Xt 6= Yt] e ∃ coupling 1 couple Xt=Yt ⇒ Xt+1=Yt+1 in case Xt=x’ ≠ y’=Yt ∃ coupling Pr[Xkt 6= Ykt] = kp (k1)t x0 p (k1)t y0 kT V
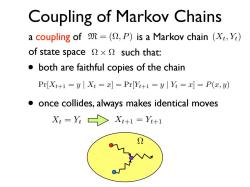
Coupling of Markov Chains a coupling of =(P)is a Markov chain (X,Y) of state space x such that: o l both are faithful copies of the chain Pr[X++1=y Xt=x]=Pr[Yi+1=yYi=x]=P(x,y) once collides,always makes identical moves Xi=Y>Xi+1=Yi+1
• both are faithful copies of the chain • once collides, always makes identical moves Coupling of Markov Chains ⌦ Pr[Xt+1 = y | Xt = x] = Pr[Yt+1 = y | Yt = x] = P(x, y) Xt = Yt Xt+1 = Yt+1 is a Markov chain (Xt, Yt) of state space a coupling of M = (⌦, P) ⌦ ⇥ ⌦ such that:
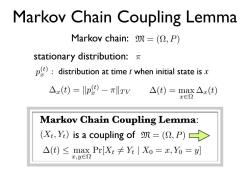
Markov Chain Coupling Lemma Markov chain:=(,P) stationary distribution: p):distribution at time t when initial state isx △z(t)=lp-πlrv △(t)=max△z(t) x∈2 Markov Chain Coupling Lemma: (X:,Yi)is a coupling of=(2,P) △(t)≤nax Pr[Xt≠Yt|Xo=x,Yo=y x,y∈2
Markov Chain Coupling Lemma Markov chain: M = (⌦, P) x(t) = kp(t) x ⇡kT V (t) = max x2⌦ x(t) stationary distribution: ⇡ p(t) x : distribution at time t when initial state is x (Xt, Yt) is a coupling of M = (⌦, P) (t) max x,y2⌦ Pr[Xt 6= Yt | X0 = x, Y0 = y] Markov Chain Coupling Lemma:
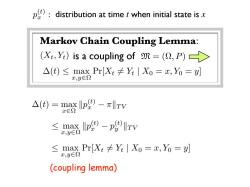
distribution at timewhen initial state isx Markov Chain Coupling Lemma: (X,Y)is a coupling of =(2,P) △(t)≤max Pr[Xt≠Y|Xo=x,Yo=y x,y∈2 △()=竖p-xy ≤max x,y∈2 llsv ≤max Pr[Xt卡Yt|Xo=x,Yo=y c,y∈2 (coupling lemma)
(Xt, Yt) is a coupling of M = (⌦, P) (t) max x,y2⌦ Pr[Xt 6= Yt | X0 = x, Y0 = y] Markov Chain Coupling Lemma: p(t) x : distribution at time t when initial state is x (t) = max x2⌦ kp(t) x ⇡kT V max x,y2⌦ kp(t) x p(t) y kT V max x,y2⌦ Pr[Xt 6= Yt | X0 = x, Y0 = y] (coupling lemma)
按次数下载不扣除下载券;
注册用户24小时内重复下载只扣除一次;
顺序:VIP每日次数-->可用次数-->下载券;
- 南京大学:《概率与计算 Probability and Computing》课程教学资源(课件讲稿)random11.pdf
- 南京大学:《概率与计算 Probability and Computing》课程教学资源(课件讲稿)random10.pdf
- 南京大学:《概率与计算 Probability and Computing》课程教学资源(课件讲稿)random7.pdf
- 南京大学:《概率与计算 Probability and Computing》课程教学资源(课件讲稿)random6.pdf
- 南京大学:《概率与计算 Probability and Computing》课程教学资源(课件讲稿)random5.pdf
- 南京大学:《概率与计算 Probability and Computing》课程教学资源(课件讲稿)random4.pdf
- 南京大学:《概率与计算 Probability and Computing》课程教学资源(课件讲稿)random3.pdf
- 南京大学:《概率与计算 Probability and Computing》课程教学资源(课件讲稿)random2.pdf
- 南京大学:《概率与计算 Probability and Computing》课程教学资源(课件讲稿)random1.pdf
- 南京大学:《组合数学 Combinatorics》课程教学资源(课件讲稿)Linear Programming.pdf
- 南京大学:《组合数学 Combinatorics》课程教学资源(课件讲稿)Flow and Matching.pdf
- 南京大学:《组合数学 Combinatorics》课程教学资源(课件讲稿)Matching Theory.pdf
- 南京大学:《组合数学 Combinatorics》课程教学资源(课件讲稿)Ramsey Theory.pdf
- 南京大学:《组合数学 Combinatorics》课程教学资源(课件讲稿)Ramsey Theory-2.pdf
- 南京大学:《组合数学 Combinatorics》课程教学资源(课件讲稿)Ramsey Theory-1.pdf
- 南京大学:《组合数学 Combinatorics》课程教学资源(课件讲稿)Extremal Combinatorics.pdf
- 南京大学:《组合数学 Combinatorics》课程教学资源(课件讲稿)The Probabilistic Method.pdf
- 南京大学:《组合数学 Combinatorics》课程教学资源(课件讲稿)Existence.pdf
- 南京大学:《组合数学 Combinatorics》课程教学资源(课件讲稿)Extremal Combinatorics.pdf
- 南京大学:《组合数学 Combinatorics》课程教学资源(课件讲稿)The Sieve Methods.pdf
- 南京大学:《概率与计算 Probability and Computing》课程教学资源(课件讲稿)random13.pdf
- 南京大学:《概率与计算 Probability and Computing》课程教学资源(课件讲稿)random8.pdf
- 南京大学:《概率与计算 Probability and Computing》课程教学资源(课件讲稿)random9.pdf
- 运城学院:《抽象代数 Abstract algebra》课程教学资源(习题与试题)2015年1月抽象代数试题及答案(B).pdf
- 运城学院:《抽象代数 Abstract algebra》课程教学资源(习题与试题)2015年1月抽象代数试题及答案(A).pdf
- 运城学院:《抽象代数 Abstract algebra》课程教学资源(习题与试题)2017年1月抽象代数试题及答案(A).pdf
- 运城学院:《抽象代数 Abstract algebra》课程教学资源(习题与试题)2017年1月抽象代数试题及答案(B).pdf
- 运城学院:《抽象代数 Abstract algebra》课程教学资源(习题与试题)2019年1月抽象代数试题及答案(A).pdf
- 运城学院:《抽象代数 Abstract algebra》课程教学资源(习题与试题)2019年1月抽象代数试题及答案(B).pdf
- 运城学院:《抽象代数 Abstract algebra》课程教学资源(习题与试题)2020 年6月抽象代数试题及答案(B).pdf
- 运城学院:《抽象代数 Abstract algebra》课程教学资源(习题与试题)2020年6月抽象代数试题及答案(A).pdf
- 运城学院:《抽象代数 Abstract algebra》课程教学资源(习题与试题)2021年1月抽象代数试题及答案(A).pdf
- 运城学院:《抽象代数 Abstract algebra》课程教学资源(习题与试题)2021年1月抽象代数试题及答案(B).pdf
- 运城学院:《抽象代数 Abstract algebra》课程教学资源(习题与试题)2018年1月抽象代数试题及答案(B).pdf
- 运城学院:《抽象代数 Abstract algebra》课程教学资源(习题与试题)2022年1月抽象代数试题及答案(B).pdf
- 运城学院:《抽象代数 Abstract algebra》课程教学资源(习题与试题)2018年1月抽象代数试题及答案(A).pdf
- 运城学院:《抽象代数 Abstract algebra》课程教学资源(习题与试题)2022年1月抽象代数试题及答案(A).pdf
- 高等教育出版社:《近世代数》课程教材教学资源(电子书籍)近世代数学习辅导与习题选解(编著:杨子胥,共七章).pdf
- 运城学院:《抽象代数 Abstract algebra》课程教学资源(课件讲稿)近世代数 Modern algebra 第一章 基本概念(杨子胥).pdf
- 运城学院:《抽象代数 Abstract algebra》课程教学资源(课件讲稿)近世代数 Modern algebra 第二章 群 2.1-2.3.pdf