中国科学院:CERN专题计算学校《T-CSC数据存储》课程教学资源(讲义)Optimizing existing large codebase-booklet
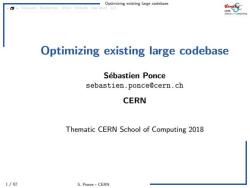
Optimizing existing large codebase Measire Modemise Mem threads lon Optimizing existing large codebase Sebastien Ponce sebastien.ponce@cern.ch CERN Thematic CERN School of Computing 2018 1/62 S.Ponce-CERN
Optimizing existing large codebase 1 / 62 S. Ponce - CERN Measure Modernize Mem threads low level c/c Optimizing existing large codebase S´ebastien Ponce sebastien.ponce@cern.ch CERN Thematic CERN School of Computing 2018
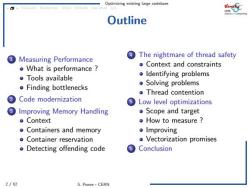
Optimizing existing large codebase Measuare Mem threads low Outline Measuring Performance The nightmare of thread safety o What is performance oContext and constraints Tools available ldentifying problems o Finding bottlenecks o Solving problems ●Thread contention ②Code modernization 6 Low level optimizations Improving Memory Handling Scope and target o Context o How to measure 。Containers and memory ●Improving o Container reservation o Vectorization promises o Detecting offending code Conclusion 2/62 S.Ponce-CERN
Optimizing existing large codebase 2 / 62 S. Ponce - CERN Measure Modernize Mem threads low level c/c Outline 1 Measuring Performance What is performance ? Tools available Finding bottlenecks 2 Code modernization 3 Improving Memory Handling Context Containers and memory Container reservation Detecting offending code 4 The nightmare of thread safety Context and constraints Identifying problems Solving problems Thread contention 5 Low level optimizations Scope and target How to measure ? Improving Vectorization promises 6 Conclusion
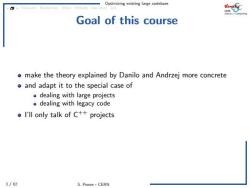
Optimizing existing large codebase 4 Measiare Modemis世Mem thread Goal of this course make the theory explained by Danilo and Andrzej more concrete and adapt it to the special case of dealing with large projects o dealing with legacy code I'll only talk of C+projects 3/62 S.Ponce-CERN
Optimizing existing large codebase 3 / 62 S. Ponce - CERN Measure Modernize Mem threads low level c/c Goal of this course make the theory explained by Danilo and Andrzej more concrete and adapt it to the special case of dealing with large projects dealing with legacy code I’ll only talk of C++ projects
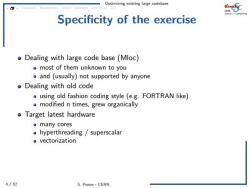
Optimizing existing large codebase Measire Modemise Mem threads Specificity of the exercise Dealing with large code base(Mloc) o most of them unknown to you and(usually)not supported by anyone 。Dealing with old code using old fashion coding style(e.g.FORTRAN like) modified n times,grew organically Target latest hardware many cores hyperthreading superscalar ●vectorization 4/62 S.Ponce-CERN
Optimizing existing large codebase 4 / 62 S. Ponce - CERN Measure Modernize Mem threads low level c/c Specificity of the exercise Dealing with large code base (Mloc) most of them unknown to you and (usually) not supported by anyone Dealing with old code using old fashion coding style (e.g. FORTRAN like) modified n times, grew organically Target latest hardware many cores hyperthreading / superscalar vectorization
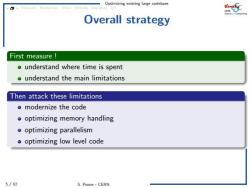
Optimizing existing large codebase 4 Measuare Mo6en世Mem threads fo Overall strategy First measure o understand where time is spent o understand the main limitations Then attack these limitations 。modernize the code o optimizing memory handling o optimizing parallelism optimizing low level code 5/62 S.Ponce-CERN
Optimizing existing large codebase 5 / 62 S. Ponce - CERN Measure Modernize Mem threads low level c/c Overall strategy First measure ! understand where time is spent understand the main limitations Then attack these limitations modernize the code optimizing memory handling optimizing parallelism optimizing low level code
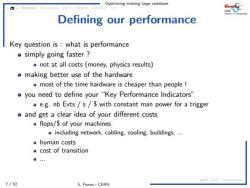
Optimizing existing large codebase Measure Mem threads Defining our performance Key question is:what is performance o simply going faster not at all costs(money,physics results) o making better use of the hardware most of the time hardware is cheaper than people o you need to define your "Key Performance Indicators" e.g.nb Evts/s/S with constant man power for a trigger o and get a clear idea of your different costs flops/S of your machines including network,cabling,cooling,buildings,... ◆human costs ●cost of transition perf tools bottlenecks 7/62 S.Ponce-CERN
Optimizing existing large codebase 7 / 62 S. Ponce - CERN Measure Modernize Mem threads low level c/c perf tools bottlenecks Defining our performance Key question is : what is performance simply going faster ? not at all costs (money, physics results) making better use of the hardware most of the time hardware is cheaper than people ! you need to define your “Key Performance Indicators” e.g. nb Evts / s / ✩ with constant man power for a trigger and get a clear idea of your different costs flops/✩ of your machines including network, cabling, cooling, buildings, ... human costs cost of transition
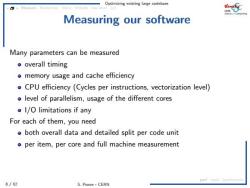
Optimizing existing large codebase Measuring our software Many parameters can be measured o overall timing o memory usage and cache efficiency CPU efficiency (Cycles per instructions,vectorization level) level of parallelism,usage of the different cores I/O limitations if any For each of them,you need both overall data and detailed split per code unit o per item,per core and full machine measurement perf tools bottlenecks 8/62 S.Ponce-CERN
Optimizing existing large codebase 8 / 62 S. Ponce - CERN Measure Modernize Mem threads low level c/c perf tools bottlenecks Measuring our software Many parameters can be measured overall timing memory usage and cache efficiency CPU efficiency (Cycles per instructions, vectorization level) level of parallelism, usage of the different cores I/O limitations if any For each of them, you need both overall data and detailed split per code unit per item, per core and full machine measurement
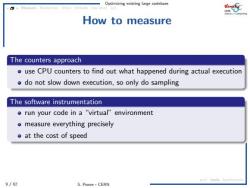
Optimizing existing large codebase How to measure The counters approach o use CPU counters to find out what happened during actual execution o do not slow down execution,so only do sampling The software instrumentation o run your code in a "virtual"environment o measure everything precisely o at the cost of speed perf tools bottlenecks 9/62 S.Ponce-CERN
Optimizing existing large codebase 9 / 62 S. Ponce - CERN Measure Modernize Mem threads low level c/c perf tools bottlenecks How to measure The counters approach use CPU counters to find out what happened during actual execution do not slow down execution, so only do sampling The software instrumentation run your code in a “virtual” environment measure everything precisely at the cost of speed
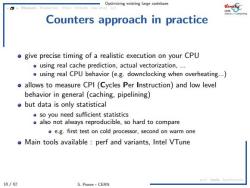
Optimizing existing large codebase Measuire Modemiss Mem threads low Counters approach in practice o give precise timing of a realistic execution on your CPU ousing real cache prediction,actual vectorization,... using real CPU behavior(e.g.downclocking when overheating...) o allows to measure CPI (Cycles Per Instruction)and low level behavior in general (caching,pipelining) but data is only statistical so you need sufficient statistics o also not always reproducible,so hard to compare e.g.first test on cold processor,second on warm one Main tools available:perf and variants,Intel VTune ef tools bottlenecks 10/62 S.Ponce-CERN
Optimizing existing large codebase 10 / 62 S. Ponce - CERN Measure Modernize Mem threads low level c/c perf tools bottlenecks Counters approach in practice give precise timing of a realistic execution on your CPU using real cache prediction, actual vectorization, ... using real CPU behavior (e.g. downclocking when overheating...) allows to measure CPI (Cycles Per Instruction) and low level behavior in general (caching, pipelining) but data is only statistical so you need sufficient statistics also not always reproducible, so hard to compare e.g. first test on cold processor, second on warm one Main tools available : perf and variants, Intel VTune
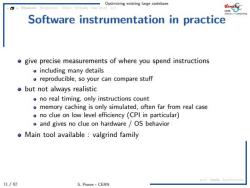
Optimizing existing large codebase Measure Mem threads low Software instrumentation in practice o give precise measurements of where you spend instructions including many details oreproducible,so your can compare stuff but not always realistic no real timing,only instructions count memory caching is only simulated,often far from real case no clue on low level efficiency (CPI in particular) and gives no clue on hardware /OS behavior o Main tool available:valgrind family ef tools bottlenecks 11/62 S.Ponce-CERN
Optimizing existing large codebase 11 / 62 S. Ponce - CERN Measure Modernize Mem threads low level c/c perf tools bottlenecks Software instrumentation in practice give precise measurements of where you spend instructions including many details reproducible, so your can compare stuff but not always realistic no real timing, only instructions count memory caching is only simulated, often far from real case no clue on low level efficiency (CPI in particular) and gives no clue on hardware / OS behavior Main tool available : valgrind family
按次数下载不扣除下载券;
注册用户24小时内重复下载只扣除一次;
顺序:VIP每日次数-->可用次数-->下载券;
- 中国科学院:CERN专题计算学校《T-CSC数据存储》课程教学资源(讲义)Optimizing existing large codebase-pres.pdf
- 中国科学院:CERN专题计算学校《T-CSC数据存储》课程教学资源(讲义)Preserving data-pres.pdf
- 中国科学院:CERN专题计算学校《T-CSC数据存储》课程教学资源(讲义)Many ways to store data-booklet.pdf
- 中国科学院:CERN专题计算学校《T-CSC数据存储》课程教学资源(讲义)Many ways to store data-pres.pdf
- 中国科学院:CERN专题计算学校《T-CSC数据存储》课程教学资源(讲义)Structuring data for efficient I/O-booklet.pdf
- 中国科学院:CERN专题计算学校《T-CSC数据存储》课程教学资源(讲义)Structuring data for efficient I/O-pres.pdf
- 中国科学院:CERN专题计算学校《T-CSC数据存储》课程教学资源(讲义)Optimizing existing large codebase-booklet.pdf
- 中国科学院:CERN专题计算学校《T-CSC数据存储》课程教学资源(讲义)Optimizing existing large codebase-pres.pdf
- 中国科学院:CERN专题计算学校《T-CSC数据存储》课程教学资源(讲义)Modern programming languages for HEP-booklet.pdf
- 中国科学院:CERN专题计算学校《T-CSC数据存储》课程教学资源(讲义)Modern programming languages for HEP-pres.pdf
- 中国科学院:CERN专题计算学校《T-CSC数据存储》课程教学资源(讲义)Practical vectorization-booklet.pdf
- 中国科学院:CERN专题计算学校《T-CSC数据存储》课程教学资源(讲义)Practical vectorization-pres.pdf
- 中国科学院:CERN专题计算学校《T-CSC数据存储》课程教学资源(讲义)Writing Parallel software(booklet).pdf
- 中国科学院:CERN专题计算学校《T-CSC数据存储》课程教学资源(讲义)Writing Parallel software(pres).pdf
- 中国科学院高能所计算中心:数据技术上机 Data Technologies – CERN School of Computing 2019.pdf
- 中国科学院高能所计算中心:数据技术课程 CSC 2018 Data Technologies Exercises(CSC DT 2018 Introduction).pdf
- 中国科学院高能所计算中心:高能物理数据的存储和管理(汪璐).pptx
- 南京大学:《数据结构 Data Structures》课程教学资源(PPT课件讲稿)第九章 排序.ppt
- 南京大学:《数据结构 Data Structures》课程教学资源(PPT课件讲稿)第八章 图.ppt
- 南京大学:《数据结构 Data Structures》课程教学资源(PPT课件讲稿)第七章 搜索结构.ppt
- 中国科学院:CERN专题计算学校《T-CSC数据存储》课程教学资源(讲义)Preserving data-booklet.pdf
- 中国科学院:CERN专题计算学校《T-CSC数据存储》课程教学资源(讲义)Key ingredients to achieve effective I/O-pres.pdf
- 中国科学院:CERN专题计算学校《T-CSC数据存储》课程教学资源(讲义)Key ingredients to achieve effective I/O-booklet.pdf
- 中国科学院:CERN专题计算学校《T-CSC数据存储》课程教学资源(讲义)Data storage and preservation-pres.pdf
- 中国科学院:CERN专题计算学校《T-CSC数据存储》课程教学资源(讲义)Data storage and preservation-booklet.pdf
- 西安电子科技大学:《数学图像处理 Digital Image Processing Digital Image Processing》课程教学资源(授课教案)第1章 绪论(许录平).pdf
- 西安电子科技大学:《数学图像处理 Digital Image Processing Digital Image Processing》课程教学资源(授课教案)第2章 数字图像处理基础.pdf
- 西安电子科技大学:《数学图像处理 Digital Image Processing Digital Image Processing》课程教学资源(授课教案)第3章 图像变换.pdf
- 西安电子科技大学:《数学图像处理 Digital Image Processing Digital Image Processing》课程教学资源(授课教案)第4章 图像增强.pdf
- 西安电子科技大学:《数学图像处理 Digital Image Processing Digital Image Processing》课程教学资源(授课教案)第5章 图象恢复.pdf
- 西安电子科技大学:《数学图像处理 Digital Image Processing Digital Image Processing》课程教学资源(授课教案)第6章 图像压缩编码.pdf
- 西安电子科技大学:《数学图像处理 Digital Image Processing Digital Image Processing》课程教学资源(授课教案)第7章 图像分割.pdf
- 西安电子科技大学:《数学图像处理 Digital Image Processing Digital Image Processing》课程教学资源(授课教案)第8章 图像描述.pdf
- 西安电子科技大学:《数学图像处理 Digital Image Processing Digital Image Processing》课程教学资源(授课教案)第9章 图像分类识别.pdf
- 西安电子科技大学:《数学图像处理 Digital Image Processing Digital Image Processing》课程教学资源(作业习题)各章要求及必做题参考答案.pdf
- 西安电子科技大学:《数学图像处理 Digital Image Processing Digital Image Processing》课程教学资源(实验指导)数字图像处理与Matlab.pdf
- 西安电子科技大学:《数学图像处理 Digital Image Processing Digital Image Processing》课程教学资源(实验指导)上机辅导讲义 - Matlab简介.pdf
- 西安电子科技大学:《数学图像处理 Digital Image Processing Digital Image Processing》课程教学资源(实验指导)数字图像处理上机实验题.pdf
- 对外经济贸易大学:《计算机应用基础》课程教学大纲 Fundamentals of Computer Application(打印版).pdf
- 对外经济贸易大学:《计算机应用基础》课程授课教案 Fundamentals of Computer Application(打印版).pdf