《金融经济学原理》课程教学资源(文献资料)Nonparametric risk management and implied risk aversion
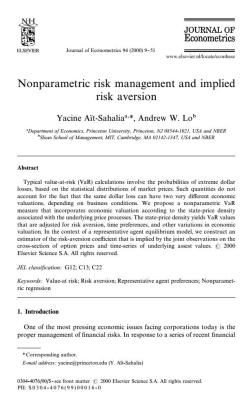
H JOURNAL OF Econometrics ELSEVIER Journal of Econometrics 94(2000)9-51 www.elsevier.nl/locate/econbase Nonparametric risk management and implied risk aversion Yacine Ait-Sahalia2.*.Andrew W.Lob Abstract me dollar ical value-at-risk (vae the probabilities of extrem based statistical dis ns of m ount for r the fact that th epending on business condit measure that incorporates economic valuation according to the stat density that are adjusted for risk aversion,time preferences,and other variations in economic valuation.In the context of a representative agent equilibrium model,we construct an estimator of the risk-aversion coefficient that is implied by the joint observations on the cross-section of option prices and time-series of underlying assest values.C 2000 Elsevier Science S.A.All rights reserved. JEL classification:G12:C13:C22 Keywords:Value-at risk;Risk aversion;Representative agent preferences;Nonparamet- ric regression 1.Introduction One of the most pressing issues facing corporations today is the proper management of financial risks.In response to a series of recent financial *Corresponding author E-mail address:yacine@princeton.edu(Y.Ait-Sahalia 0304-4076/00/S-see front matter 2000 Elsevier Science S.A.All rights reserved. P:S0304-4076(99)00016-0
Journal of Econometrics 94 (2000) 9}51 Nonparametric risk management and implied risk aversion Yacine AmKt-Sahalia!,*, Andrew W. Lo" !Department of Economics, Princeton University, Princeton, NJ 08544-1021, USA and NBER "Sloan School of Management, MIT, Cambridge, MA 02142-1347, USA and NBER Abstract Typical value-at-risk (VaR) calculations involve the probabilities of extreme dollar losses, based on the statistical distributions of market prices. Such quantities do not account for the fact that the same dollar loss can have two very di!erent economic valuations, depending on business conditions. We propose a nonparametric VaR measure that incorporates economic valuation according to the state-price density associated with the underlying price processes. The state-price density yields VaR values that are adjusted for risk aversion, time preferences, and other variations in economic valuation. In the context of a representative agent equilibrium model, we construct an estimator of the risk-aversion coe$cient that is implied by the joint observations on the cross-section of option prices and time-series of underlying assest values. ( 2000 Elsevier Science S.A. All rights reserved. JEL classixcation: G12; C13; C22 Keywords: Value-at risk; Risk aversion; Representative agent preferences; Nonparametric regression 1. Introduction One of the most pressing economic issues facing corporations today is the proper management of "nancial risks. In response to a series of recent "nancial * Corresponding author. E-mail address: yacine@princeton.edu (Y. AmKt-Sahalia) 0304-4076/00/$ - see front matter ( 2000 Elsevier Science S.A. All rights reserved. PII: S 0 3 0 4 - 4 0 7 6 ( 9 9 ) 0 0 0 1 6 - 0

10 Y.Ai-Sahalia,A.W.Lo/Journal of Econometrics 94(2000)9-51 catastrophes,regulators,investment bankers,and chief executive officers have now embraced the notion of risk management as one of the primary fiduciary responsibilities of the corporate manager.Because financial risks often manifest themselves in subtle and nonlinear ways in corporate balance sheets and income statements,recent attention has focused on quantifying the fluctuations of market valuations in a statistical sense.These value-at-risk(VaR)measures lie at the heart of mo current risk management systems and protocols.For example JP Morgan's(1995)RiskMetrics system documentation describes VaR in the following way: Value at Risk is an estimate,with a predefined confidence interval,of how much one can lose from holding a position over a set horizon.Potential horizons may be one day for typical trading activities or a month or longer for e methods described in our documentation useis volatilities and correlations that are then used to estimate the market risk.These statistics can be applied across a set of asset classes covering products used by financial institutions,corporations,and insti- tutional investors. By modeling the price fluctuations of securities held in one's portfolio,an estimate and confidence interval of how much one can lose is readily derived from the basic principles of statistical inference.However,in this paper we argue that statistical notions of value-at-risk are,at best,inco omplete measures of the true risks facing investors.In particular,while statistical measures do provide some information about the range of uncertainty that a portfolio exhibits,they have little to do with the economic valuation of such uncertainty.For example, a typical VaR statistic might indicate a 5%probability of a $15M loss for a S10M portfolio over the next month,which seems to be a substantial risk exposure at first glance.But if this 15%loss occurs only when other investments of similar characteristics suffer losses of 25%or more,such a risk may seem rather mild after all.This simplistic example suggests that a one-dollar loss is not always worth the same,and that circumstances s urrounding the loss can affect its economic valuation,something that is completely ignored by purely statistical measures of risk In this paper,we propose an alternative to statistical VaR(henceforth S-VaR) that is based on economic valuations of value-at-risk,and which incorporate many other aspects of market risk that are central to the practice of risk management.Our alternative is based on the seminal ideas of Arrow (1964)and Debreu(1959),who first formalized the economics of uncertainty by introducing elementary securities each paying $1 in one specific state of nature and nothing
catastrophes,1 regulators, investment bankers, and chief executive o$cers have now embraced the notion of risk management as one of the primary "duciary responsibilities of the corporate manager. Because "nancial risks often manifest themselves in subtle and nonlinear ways in corporate balance sheets and income statements, recent attention has focused on quantifying the #uctuations of market valuations in a statistical sense. These value-at-risk (VaR) measures lie at the heart of most current risk management systems and protocols. For example, JP Morgan's (1995) RiskMetrics system documentation describes VaR in the following way: Value at Risk is an estimate, with a prede"ned con"dence interval, of how much one can lose from holding a position over a set horizon. Potential horizons may be one day for typical trading activities or a month or longer for portfolio management. The methods described in our documentation use historical returns to forecast volatilities and correlations that are then used to estimate the market risk. These statistics can be applied across a set of asset classes covering products used by "nancial institutions, corporations, and institutional investors. By modeling the price #uctuations of securities held in one's portfolio, an estimate and con"dence interval of how much one can lose is readily derived from the basic principles of statistical inference. However, in this paper we argue that statistical notions of value-at-risk are, at best, incomplete measures of the true risks facing investors. In particular, while statistical measures do provide some information about the range of uncertainty that a portfolio exhibits, they have little to do with the economic valuation of such uncertainty. For example, a typical VaR statistic might indicate a 5% probability of a $15M loss for a $100M portfolio over the next month, which seems to be a substantial risk exposure at "rst glance. But if this 15% loss occurs only when other investments of similar characteristics su!er losses of 25% or more, such a risk may seem rather mild after all. This simplistic example suggests that a one-dollar loss is not always worth the same, and that circumstances surrounding the loss can a!ect its economic valuation, something that is completely ignored by purely statistical measures of risk. In this paper, we propose an alternative to statistical VaR (henceforth S-VaR) that is based on economic valuations of value-at-risk, and which incorporates many other aspects of market risk that are central to the practice of risk management. Our alternative is based on the seminal ideas of Arrow (1964) and Debreu (1959), who "rst formalized the economics of uncertainty by introducing elementary securities each paying $1 in one speci"c state of nature and nothing 1For example, the multimillion-dollar losses su!ered by Gibson Greetings, Metallgesellschaft, Orange County, Proctor and Gamble, Barings Securities, etc. 10 Y. An(t-Sahalia, A.W. Lo / Journal of Econometrics 94 (2000) 9}51
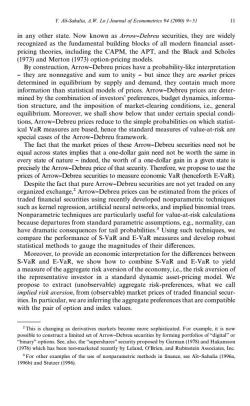
Y.Ait-Sahalia,A.W.Lo Journal of Econometrics 94 (2000)9-51 in any other state.Now known as Arrow-Debreu securities,they are widely recognized as the fundamental building blocks of all modern financial asset pricing theories,including the CAPM,the APT,and the Black and Scholes (1973)and Merton(1973)option-pricing models. By construction,Arrow-Debreu prices have a probability-like interpretation -they are nonnegative and sum to unity but since they are market prices determined in equilibrium by supply and demand,they contain much more information than statistical models of prices.Arrow-Debreu prices are deter- amics,informa- ined by the combination of investors pckec nn and the sitio general equilibrium.Moreover,we shall show below that under certain special condi tions,Arrow-Debreu prices reduce to the simple probabilities on which statist- ical VaR measures are based,hence the standard measures of value-at-risk are cial case s of the Arrov w-Debreu f amework. The fact that the market prices of these Arrow-Debreu securities need not be equal across states implies that a one-dollar gain need not be worth the same in every state of nature-indeed,the worth of a one-dollar gain in a given state is w-Debreu pri ice of that security.Therefo Debreu sites to msur ono VaR he we prop e to use the Despite the fact that pure Arrow-Debreu securities are not yet traded on any organized exchange,2 Arrow-Debreu prices can be estimated from the prices of traded financial recently developed no rictechniques such as kernel regression,artificial neural networks,and implied binomial trees. Nonparametric techniques are particularly useful for value-at-risk calculations because departures from standard parametric assumptions,e.g.,normality,can have dramatic consequences for tail probabilities Using such techniques,we compare the performance of s-VaR and E-VaR measures and develop robust statistical methods to gauge the magnitudes of their differences. Moreover,to provide an economic interpretation for the differences between S-VaR and E-VaR,we show how to con nbine S-VaR and E-VaR to yield a measure of the aggregate risk aversion of the economy,i.e,the risk aversion the representative investor in a standard dynamic asset-pricing model.We propose to extract (unobservable)aggregate risk-preferences,what we call mplied risk aversion,from (observable)market prices of traded financial secur- ities.n particur,the aggregate preferences that are compatible with the pair of option and index values. 2This is changing as derivatives markets become more sophisticated.For example,it is now le to construct a limited set of Arrow-Debreu securitie (97 whih s be man(19 tes ios o Hakan markete recently by 31e a Forther em of thes of mcthods in fnance,Ait-Sahalia 1996b)and Stutzer (1996)
in any other state. Now known as Arrow}Debreu securities, they are widely recognized as the fundamental building blocks of all modern "nancial assetpricing theories, including the CAPM, the APT, and the Black and Scholes (1973) and Merton (1973) option-pricing models. By construction, Arrow}Debreu prices have a probability-like interpretation } they are nonnegative and sum to unity } but since they are market prices determined in equilibrium by supply and demand, they contain much more information than statistical models of prices. Arrow}Debreu prices are determined by the combination of investors' preferences, budget dynamics, information structure, and the imposition of market-clearing conditions, i.e., general equilibrium. Moreover, we shall show below that under certain special conditions, Arrow}Debreu prices reduce to the simple probabilities on which statistical VaR measures are based, hence the standard measures of value-at-risk are special cases of the Arrow}Debreu framework. The fact that the market prices of these Arrow}Debreu securities need not be equal across states implies that a one-dollar gain need not be worth the same in every state of nature } indeed, the worth of a one-dollar gain in a given state is precisely the Arrow}Debreu price of that security. Therefore, we propose to use the prices of Arrow}Debreu securities to measure economic VaR (henceforth E-VaR). Despite the fact that pure Arrow}Debreu securities are not yet traded on any organized exchange,2 Arrow}Debreu prices can be estimated from the prices of traded "nancial securities using recently developed nonparametric techniques such as kernel regression, arti"cial neural networks, and implied binomial trees. Nonparametric techniques are particularly useful for value-at-risk calculations because departures from standard parametric assumptions, e.g., normality, can have dramatic consequences for tail probabilities.3 Using such techniques, we compare the performance of S-VaR and E-VaR measures and develop robust statistical methods to gauge the magnitudes of their di!erences. Moreover, to provide an economic interpretation for the di!erences between S-VaR and E-VaR, we show how to combine S-VaR and E-VaR to yield a measure of the aggregate risk aversion of the economy, i.e., the risk aversion of the representative investor in a standard dynamic asset-pricing model. We propose to extract (unobservable) aggregate risk-preferences, what we call implied risk aversion, from (observable) market prices of traded "nancial securities. In particular, we are inferring the aggregate preferences that are compatible with the pair of option and index values. 2This is changing as derivatives markets become more sophisticated. For example, it is now possible to construct a limited set of Arrow}Debreu securities by forming portfolios of `digitala or `binarya options. See, also, the `supersharesa security proposed by Garman (1978) and Hakansson (1976) which has been test-marketed recently by Leland, O'Brien, and Rubinstein Associates, Inc. 3For other examples of the use of nonparametric methods in "nance, see AmKt}Sahalia (1996a, 1996b) and Stutzer (1996). Y. An(t-Sahalia, A.W. Lo / Journal of Econometrics 94 (2000) 9}51 11
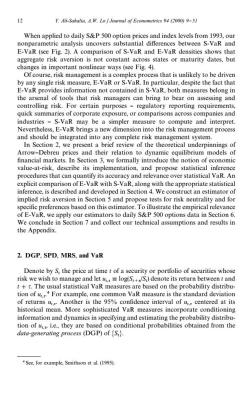
12 Y.Ai-Sahalia,A.W.Lo Journal of Econometrics 94(2000)9-5 When applied to daily S&P 500 option prices and index levels from 1993,our nonparametric analysis uncovers substantial differences between S-VaR and E-VaR(see Fig.2).A comparison of S-VaR and E-VaR densities shows that aggregate risk aversion is not constant across states or maturity dates,but changes in important nonlinear wavs (see Fig.4). Of course,risk management is a complex process that is unlikely to be driven by any sine risk measure E-VaR-Vak.In particular.despite the fact that E-VaR provides information not contained in S-VaR,both measures belong in the arsenal of tools that risk managers can bring to bear on assessing and controlling risk.For certain purposes-regulatory reporting requirements. quick summaries of corporate exposure,r comparisons across companies and industries-S-VaR may be a simpler measure to compute and interpret. Nevertheless,E-VaR brings a new dimension into the risk management process and should be integrated into any complete risk management system. In Se ection 2,we present a brief review of the theoretical underpinnings of Arrow-Debreu prices and their relation to dynamic equilibrium models of financial markets.In Section 3,we formally introduce the notion of economic value-at-risk,describe its implementation,and propose statistical inference procedures that can quantify its accuracy and relevanc over statistical VaR.An explicit comparison of E-VaR with S-VaR,along with the appropriate statistical inference,is described and developed in Section 4.We construct an estimator of implied risk aversion in Section 5 and propose tests for risk neutrality and for ic preferences based on this estimato trate the empirical relevanc of E-VaR,we apply our estimators to daily500 options data in Section6. We conclude in Section 7 and collect our technical assumptions and results in the Appendix. 2.DGP,SPD,MRS,and VaR Denote by S,the price at time t of a security or portfolio of securities whose risk we wish to manage and let u,=log(S,/S,)denote its return between t and .The usual statistical VaR measures are based on the probability distribu- tion ofFor example,one common VaR measure is the standard deviatior of returns u.Another is the 95%confidence interval of u centered at its historical mean.More sophisticated VaR measures incorporate conditioning information and dynamics in specifying and estimating the probability distribu- tion of ur.ie they are based on conditional probabilities obtained from the data-generating process DGP)ofS. +See.for example.Smithson et al.(1995)
When applied to daily S&P 500 option prices and index levels from 1993, our nonparametric analysis uncovers substantial di!erences between S-VaR and E-VaR (see Fig. 2). A comparison of S-VaR and E-VaR densities shows that aggregate risk aversion is not constant across states or maturity dates, but changes in important nonlinear ways (see Fig. 4). Of course, risk management is a complex process that is unlikely to be driven by any single risk measure, E-VaR or S-VaR. In particular, despite the fact that E-VaR provides information not contained in S-VaR, both measures belong in the arsenal of tools that risk managers can bring to bear on assessing and controlling risk. For certain purposes } regulatory reporting requirements, quick summaries of corporate exposure, or comparisons across companies and industries } S-VaR may be a simpler measure to compute and interpret. Nevertheless, E-VaR brings a new dimension into the risk management process and should be integrated into any complete risk management system. In Section 2, we present a brief review of the theoretical underpinnings of Arrow}Debreu prices and their relation to dynamic equilibrium models of "nancial markets. In Section 3, we formally introduce the notion of economic value-at-risk, describe its implementation, and propose statistical inference procedures that can quantify its accuracy and relevance over statistical VaR. An explicit comparison of E-VaR with S-VaR, along with the appropriate statistical inference, is described and developed in Section 4. We construct an estimator of implied risk aversion in Section 5 and propose tests for risk neutrality and for speci"c preferences based on this estimator. To illustrate the empirical relevance of E-VaR, we apply our estimators to daily S&P 500 options data in Section 6. We conclude in Section 7 and collect our technical assumptions and results in the Appendix. 2. DGP, SPD, MRS, and VaR Denote by S t the price at time t of a security or portfolio of securities whose risk we wish to manage and let u t,q ,log(S t`q /S t ) denote its return between t and t#q. The usual statistical VaR measures are based on the probability distribution of u t,q .4 For example, one common VaR measure is the standard deviation of returns u t,q . Another is the 95% con"dence interval of u t,q centered at its historical mean. More sophisticated VaR measures incorporate conditioning information and dynamics in specifying and estimating the probability distribution of u t,q , i.e., they are based on conditional probabilities obtained from the data-generating process (DGP) of MS t N. 4 See, for example, Smithson et al. (1995). 12 Y. An(t-Sahalia, A.W. Lo / Journal of Econometrics 94 (2000) 9}51
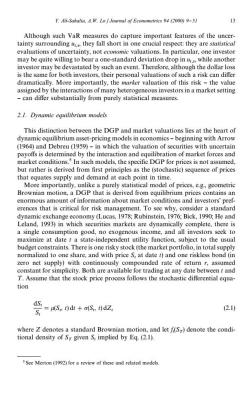
Y.Ait-Sahalia,A.W.Lo Journal of Econometrics 94 (2000)9-51 3 Although such VaR measures do capture important features of the uncer- tainty surroun fall short in one crucial respect:they are statistica evaluations of uncertainty,not economic valuations.In particular,one investor may be quite willing to bear a one-standard deviation drop in ur while another investor may be devastated by such an event.Therefore,although the dollar loss is the same for both investors,their personal valuationsof such a risk can differ dramatically.More importantly,the market valuation of this risk-the value assigned by the interactions of many heterogeneous investors in a market setting -can differ substantially from purely statistical measures. 2.1.Dynamic equilibrium models This distinction between the DGP and market valuations lies at the heart of dynamic equilibrium asset-pricing models in economics-beginning with Arrow (1964)and Debreu(1959)-in which the valuation of securities with uncertain ined by the inter ilibration of market forces and tionsIn such models theDOP for prices is not assumed but rather is derived from first principles as the (stochastic)sequence of prices that equates supply and demand at each point in time. More importantly,unlike a purely statistical model of prices,e.g,geometric Brownian motion,a DGP that is derived from prices contains an enormous amount of information about market conditions and investors'pref- erences that is critical for risk management.To see why,consider a standard ange economy (Lucas,197:Rubinstein,1976:Bick,19:He and which securities markets ars dynamically complete.there is a single consumption good,no exogenous income,and all investors seek to maximize at date t a state-independent utility function,subject to the usual budget constraints.There is one risky stock(the market portfolio,in total supply normalized to one share,and with price S,at date t)and one riskless bond (i zero net supply)with continuously compounded rate of return r,assumed constant for simplicity.Both are available for trading at any date between t and T.Assume that the stock price process follows the stochastic differential equa- tion ds=dS.)dt+as,d☑, (2.1) S. where Z denotes a standard Brownian motion,and let f(S)denote the condi- tional density of Sr given S,implied by Eq.(2.1). See Merton(1992)for a review of these and related models
Although such VaR measures do capture important features of the uncertainty surrounding u t,q , they fall short in one crucial respect: they are statistical evaluations of uncertainty, not economic valuations. In particular, one investor may be quite willing to bear a one-standard deviation drop in u t,q , while another investor may be devastated by such an event. Therefore, although the dollar loss is the same for both investors, their personal valuations of such a risk can di!er dramatically. More importantly, the market valuation of this risk } the value assigned by the interactions of many heterogeneous investors in a market setting } can di!er substantially from purely statistical measures. 2.1. Dynamic equilibrium models This distinction between the DGP and market valuations lies at the heart of dynamic equilibrium asset-pricing models in economics } beginning with Arrow (1964) and Debreu (1959) } in which the valuation of securities with uncertain payo!s is determined by the interaction and equilibration of market forces and market conditions.5 In such models, the speci"c DGP for prices is not assumed, but rather is derived from "rst principles as the (stochastic) sequence of prices that equates supply and demand at each point in time. More importantly, unlike a purely statistical model of prices, e.g., geometric Brownian motion, a DGP that is derived from equilibrium prices contains an enormous amount of information about market conditions and investors' preferences that is critical for risk management. To see why, consider a standard dynamic exchange economy (Lucas, 1978; Rubinstein, 1976; Bick, 1990; He and Leland, 1993) in which securities markets are dynamically complete, there is a single consumption good, no exogenous income, and all investors seek to maximize at date t a state-independent utility function, subject to the usual budget constraints. There is one risky stock (the market portfolio, in total supply normalized to one share, and with price S t at date t) and one riskless bond (in zero net supply) with continuously compounded rate of return r, assumed constant for simplicity. Both are available for trading at any date between t and ¹. Assume that the stock price process follows the stochastic di!erential equation dS t S t "k(S t , t) dt#p(S t , t) dZt (2.1) where Z denotes a standard Brownian motion, and let f t (S T ) denote the conditional density of S T given S t implied by Eq. (2.1). 5 See Merton (1992) for a review of these and related models. Y. An(t-Sahalia, A.W. Lo / Journal of Econometrics 94 (2000) 9}51 13

14 Y.Ai-Sahalia,A.W.Lo/Journal of Econometrics 94(2000)9-51 Under suitable assumptions for preferences and endowment shocks,it is well-known that market completeness allows us to introduce a representative investor with utility function U [see,for example,Constantinides (1982)] Assuming that he can consume at date t and at the fixed future date T(allowing intermediate consumption or labor income does not affect the basic results),and that he receives one share of the stock as endowment at date t,the representative nvestor adjusts the dollar amount invested in the stock at each intermediary date t to solve the Merton(1971)optimization problem max E[U(W] {q:|t≤s≤T} subject to dWs=rWs +gsu(Ss,s)-r)ds +gso(Ss,s)dzs W.≥0,t≤s≤T where W,denotes his wealth at date s In equilibrium,the investor optimally invests all his wealth in the risky stock at every instant prior to T(W,=S,for all tss sT)and then consumes the terminal value of the stock at T(Cr=Wr=ST).Let J(W,S,t)denote the investor's indirect utility function.The first-order condition for the investor's problem takes the form aJ(W,Ss,母 =e-n-nel(W S.D aw (2.2) with terminal condition at date s=T U(WT)=e-HT-OU(WT (2.3) where =ep.-)) (2.4) Clearly,Eq.(2.2)implies that the right-hand-side of Eq.(2.4)must be path independent,i.e,it depends only on the values of the stock price at t and s and not on the path taken by the stock price in between these dates.The price at date t of a security with a single date-T liquidating payoff of(Wr)is then given by E,[(WT)M.T] (2.5)
Under suitable assumptions for preferences and endowment shocks, it is well-known that market completeness allows us to introduce a representative investor with utility function ; [see, for example, Constantinides (1982)]. Assuming that he can consume at date t and at the "xed future date ¹ (allowing intermediate consumption or labor income does not a!ect the basic results), and that he receives one share of the stock as endowment at date t, the representative investor adjusts the dollar amount q q invested in the stock at each intermediary date q to solve the Merton (1971) optimization problem max M qs @ txsxTN E[;(=T )] subject to d=s "Mr=s #q s (k(S s , s)!r)N ds#q s p(S s , s) dZs , =s *0, t)s)¹ where =s denotes his wealth at date s. In equilibrium, the investor optimally invests all his wealth in the risky stock at every instant prior to ¹ (=s "S s for all t)s)¹) and then consumes the terminal value of the stock at ¹ (CT "=T "ST ). Let J(=, S, t) denote the investor's indirect utility function. The "rst-order condition for the investor's problem takes the form RJ(=s , Ss , s) R= "e~r(s~t) RJ(=t , S t , t) R= f s (2.2) with terminal condition at date s"¹ ;@(=T )"e~r(T~t);@(=t )f T (2.3) where f s ,expGP s t A k(S u , u)!r p(S u , u) B dZu !1 2P s t A k(S u , u)!r p(S u , u) B 2 du H . (2.4) Clearly, Eq. (2.2) implies that the right-hand-side of Eq. (2.4) must be path independent, i.e., it depends only on the values of the stock price at t and s and not on the path taken by the stock price in between these dates. The price at date t of a security with a single date-¹ liquidating payo! of t(=T ) is then given by Et [t(=T )Mt,T ] (2.5) 14 Y. An(t-Sahalia, A.W. Lo / Journal of Econometrics 94 (2000) 9}51
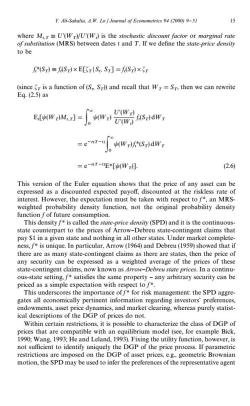
Y.Ait-Sahalia,A.W.Lo Journal of Econometrics 94 (2000)9-51 where MU(W)U(W)is the stochastic discount factor or marginal rate of substitution(MRS)between dates t and T.If we define the state-price density to be f*(Sr)=fS)×E[5rlS,Sr]=fS)×Sr (since is a function of(S ST))and recall that Wr=ST,then we can rewrite Eq.(2.5)as E[(Wr)M.r]= (WT) U(Wf(SpdWr U'(W) =e-mr-n[ (WT)f (ST)dWT =eT-E*[(Wr门. (2.6) This version of the Euler equation shows that the price of any asset can be expressed as a discounted expected payoff,discounted at the riskless rate of rest.However,the expectation must be take en with respect toan MRS- weighted probability density function,not the original probability density function f of future consumption. This density f*is called the state-price density(SPD)and it is the continuous- ness,f*is unique.In particular,Arrow(1964)and Debreu (1959)showed that if there are as many state-contingent claims as there are states.then the price of any security can be expressed as a weighted average of th prices ofthe state-contingent claims,now known as Arrow-Debreu state prices.In a continu- ous-state setting,f*satisfies the same property-any arbitrary security can be priced as a simple expectation with respect to f*. This underscores the importance off*for risk management:the SPD aggre- gates all economically pertinent information regarding investors'preferences endowments,asset price dynamics,and market clearing,whereas purely statist- ical descriptions of the DGP of prices do not. Within certain restrictions,it is possible to characterize the class of DGP of prices that are compatible with an equilibrium model (see,for example Bick 1990;Wang,1993;He and Leland,1993).Fixing the utility function,however,is not sufficient to identify uniquely the DGP of the price process.If parametric restrictions are imposed on the DGP of asset prices,e.g.,geometric Brownian motion,the SPD may be used toinfer the preferences of the representative agent
where Mt,T ,;@(=T )/;@(=t ) is the stochastic discount factor or marginal rate of substitution (MRS) between dates t and ¹. If we de"ne the state-price density to be f H t (S T ),f t (S T )]E[f T D S t , S T ]"f t (S T )]f T (since f T is a function of (S t , S T )) and recall that =T "S T , then we can rewrite Eq. (2.5) as Et [t(=T )Mt,T ]"P = 0 t(=T ) ;@(=T ) ;@(=t ) f t (S T ) d=T "e~r(T~t) P = 0 t(=T ) f H t (S T ) d=T "e~r(T~t)EH[t(=T )]. (2.6) This version of the Euler equation shows that the price of any asset can be expressed as a discounted expected payo!, discounted at the riskless rate of interest. However, the expectation must be taken with respect to f H, an MRSweighted probability density function, not the original probability density function f of future consumption. This density f H is called the state-price density (SPD) and it is the continuousstate counterpart to the prices of Arrow}Debreu state-contingent claims that pay $1 in a given state and nothing in all other states. Under market completeness, f H is unique. In particular, Arrow (1964) and Debreu (1959) showed that if there are as many state-contingent claims as there are states, then the price of any security can be expressed as a weighted average of the prices of these state-contingent claims, now known as Arrow}Debreu state prices. In a continuous-state setting, f H satis"es the same property } any arbitrary security can be priced as a simple expectation with respect to f H. This underscores the importance of f H for risk management: the SPD aggregates all economically pertinent information regarding investors' preferences, endowments, asset price dynamics, and market clearing, whereas purely statistical descriptions of the DGP of prices do not. Within certain restrictions, it is possible to characterize the class of DGP of prices that are compatible with an equilibrium model (see, for example Bick, 1990; Wang, 1993; He and Leland, 1993). Fixing the utility function, however, is not su$cient to identify uniquely the DGP of the price process. If parametric restrictions are imposed on the DGP of asset prices, e.g., geometric Brownian motion, the SPD may be used to infer the preferences of the representative agent Y. An(t-Sahalia, A.W. Lo / Journal of Econometrics 94 (2000) 9}51 15

16 Y.Ait-Sahalia,A.W.Lo/Journal of Econometrics 94(2000)9-5 in an equilibrium model of asset prices (see,for example,Bick,1987).Alterna- tively,if specific preferences are imposed,e.g.logarithmic utility,the SPD may be used to infer the DGP of asset prices.Indeed,two of the following imply the third:(1)the representative agent's preferences;(2)asset price dynamics;and(3)the SPD. 2.2.No-arbitrage models The practical relevance of SPDs for derivative pricing and hedging applica- tions has also become apparent in no-arbitrage or dynamically complete-markets models in which sophisticated dynamic trading strategies involving a set of "fundamental"securities can perfectly replicate the payoffs of more complex "derivative"securities.For example,suppose that we observe a set of n asset prices following Ito diffusions driven byindependent Brownian motions dS,=u dt +a:dz (2.7) where S,and u,are (n x 1)-vectors.Z,is an (n2 x 1)-vector of independent Brownian motions,a,i 2.Supp oose that there derivative securities on an asset with payoff function (ST)are spanned by certain dynamic trading strategies,i.e,derivatives are redundant assets hence they may be priced such applications the asset price dynamics are specified explicitly and conditions are imposed to ensure the existence of an SPD and dynamic completeness of markets(Harrison and Kreps,1979;Duffie and Huang,1985:Duffie,1996). For example,the system of asset prices S,in Eq.(2.7)supports an SPD if and only if the system of linear equations=admits at every date a solutior A,such that exp d2 has finite expectation,and oxpd nL973.19920r 197
in an equilibrium model of asset prices (see, for example, Bick, 1987). Alternatively, if speci"c preferences are imposed, e.g., logarithmic utility, the SPD may be used to infer the DGP of asset prices. Indeed, in equilibrium, any two of the following imply the third: (1) the representative agent's preferences; (2) asset price dynamics; and (3) the SPD. 2.2. No-arbitrage models The practical relevance of SPDs for derivative pricing and hedging applications has also become apparent in no-arbitrage or dynamically complete-markets models in which sophisticated dynamic trading strategies involving a set of `fundamentala securities can perfectly replicate the payo!s of more complex `derivativea securities. For example, suppose that we observe a set of n 1 asset prices following Ito( di!usions driven by n 2 independent Brownian motions: dS t "l t dt#r t dZt (2.7) where S t and l t are (n 1 ]1) -vectors, Zt is an (n 2 ]1)-vector of independent Brownian motions, r t is an (n 1 ]n 2 )-matrix, and n 1 *n 2 . Suppose that there exists a riskless asset with instantaneous rate of return r. Then path-independent derivative securities on an asset with payo! function t(S T ) are spanned by certain dynamic trading strategies, i.e., derivatives are redundant assets hence they may be priced by arbitrage.6 In such applications the asset price dynamics are speci"ed explicitly and conditions are imposed to ensure the existence of an SPD and dynamic completeness of markets (Harrison and Kreps, 1979; Du$e and Huang, 1985; Du$e, 1996). For example, the system of asset prices S t in Eq. (2.7) supports an SPD if and only if the system of linear equations r t ) k t "l t admits at every date a solution k t such that expCP T t k q ) k q dq/2D has "nite expectation, and expC !P T t k q dZq !P T t k q ) k q dq/2D 6 Additional assumptions are, of course, required such as frictionless markets, unlimited riskless borrowing and lending opportunities at the same instantaneous rate r t,q , a known di!usion coe$cient, etc. See Merton (1973, 1992) for further discussion. 16 Y. An(t-Sahalia, A.W. Lo / Journal of Econometrics 94 (2000) 9}51

Y.Ait-Sahalia.A.W.Lo Journal of Econometrics 94 (2000)9-51 1 has finite variance.In the presence of an SPD,markets are complete if and ony if rank()=n2 almost everywhere.Then the SPD can be characterized explicit ly without reference to preferences.In the particular case of geometric Brownian motion,with constant volatility o,interest rate r and dividend yield over dS=.x-ò,Sdt+oSdZ which is a lognormal distribution with mean((r.t-8.)-2/2)t and variance 石2T More generally,denote by S,the price of an underlying asset and by f*(Sr) the SPD of the asset price ST at a future date T,conditioned on the current price S,[for simplicity,we leave implicit the dependence off*on(S.,t,r.]. Consider now a European-style derivative security with a single liquidat- equal to e-me't (ST)f*(ST)dSr (2.8) Jo For example,a European call option with maturity date T and strike price X has a payoff function (Sr)=max[ST-X,0]hence its date-t price H,is simply ”+00 H(S X,t,r1.)=e rat naxST-X.Of,*(Sr)dSr (2.9) Even the most complex path-independent derivative security can be priced and hedged according toE(8). 3.Economic VaR The relevance of the SPD for risk management is clear:the MRS-weighted probability density ically complete mea of value-at-risk-economic value-than the probability density function fof the DGP.Therefore,f*can be used in the same ways that statistical VaR measures such as standard deviation,95%confidence intervals,tail probabilities,etc.are used.To distinguish the more traditional method of risk management from this 7See also Cox and Ros(1976),Goldberger(1991),Madan and Milne(1994)and Rady (1994)
has "nite variance. In the presence of an SPD, markets are complete if and only if rank(r t )"n 2 almost everywhere. Then the SPD can be characterized explicitly without reference to preferences. In the particular case of geometric Brownian motion, with constant volatility p, interest rate r t,q and dividend yield d t,q over the interval (t, t#q), the SPD or risk-neutral pricing density is given by the conditional distribution of the risk-neutral stochastic process with dynamics dSH t "(r t,q !d t,q )SH t dt#pSH t dZt which is a lognormal distribution with mean ((r t,q !d t,q )!p2/2)q and variance p2q. More generally, denote by S t the price of an underlying asset and by f H t (S T ) the SPD of the asset price S T at a future date ¹, conditioned on the current price S t [for simplicity, we leave implicit the dependence of f H t on (S t , q, r t,q , d t,q )]. Consider now a European-style derivative security with a single liquidating payo! t(S T ). To rule out arbitrage opportunities among the asset, the derivative and a risk-free cash account, the price of the derivative at t must be equal to e~rt,q>q P `= 0 t(S T ) f H t (S T ) dS T . (2.8) For example, a European call option with maturity date ¹ and strike price X has a payo! function t(S T )"max[S T !X, 0] hence its date-t price Ht is simply H(S t , X, q, r t,q )"e~rt,qq P `= 0 max[S T !X, 0] f H t (S T ) dS T . (2.9) Even the most complex path-independent derivative security can be priced and hedged according to Eq. (2.8).7 3. Economic VaR The relevance of the SPD for risk management is clear: the MRS-weighted probability density function f H provides a more economically complete measure of value-at-risk } economic value } than the probability density function f of the DGP. Therefore, f H can be used in the same ways that statistical VaR measures such as standard deviation, 95% con"dence intervals, tail probabilities, etc. are used. To distinguish the more traditional method of risk management from this 7 See also Cox and Ros (1976), Goldberger (1991), Madan and Milne (1994) and Rady (1994). Y. An(t-Sahalia, A.W. Lo / Journal of Econometrics 94 (2000) 9}51 17

18 Y.Ai-Sahalia,A.W.Lo/Journal of Econometrics 94(2000)9-51 approach,we shall refer to the statistical measure of value-at-risk as 'S-VaR' well Now if the MRS in Eq.(2.5)were observable,implementing E-VaR measures and comparing them to S-VaR measures would be a simple matter.However. in practice obtaining f*can be quite a challenge,especially for markets that are more complex than the pure-exchange economy described in Section 2.1.Fortunately,several accurate and computationally efficient estimators of f*have been developed recently and we provide a brief review of these stimatorsin derive their.1 of Appendix A.With these estimators in hand,we show in Section 4 how to gauge the relative importance of E-VaR empirically by examining the ratio ff 3.1.SPDs and option prices Banz and Miller (1978),Breeden and Litzenberger(1978),and Ross (1976) were among the first to suggest that Arrow-Debreu prices may be estimated ices of traded financial securities.In particular ight that ontions can be uset to ereate pure Arrow-Debreu state-contingent securities,Banz and Miller(1978)and Breeden and Litzenberger(1978)provide an elegant method for obtaining an explicit expression for the SPD from option prices:the SPD is the second derivative (normalized to integrate to unity)of a call option pricing formula with respect to the strike price. To see why.consider the portfolio obtained by selling two call options struck at X and buying one struck at -sand one atX+Consider 1/e2 shares of this called because of the shape of its payof function (ST)which pays nothing outside the interval [X-8,X +&]Letting tend to zero,the payoff function of the butterfly tends to a Dirac delta function with mass at X,i.e,in the limit the butterfly becomes an elementary Ar- row-Debreu security payingf-and nothing otherwise.The limit ofits price as a tends to zero should therefore be equal to e'f*(X).Now denote by H(S,.X,t)the market price of a call option at time t with strike price X, time-to-maturity and underlying asset price S Then,by construction,the price of the butterfly spread must be -2HSnX,)+HS。X-6)+HS。X+6功 (3.1) which has,as its limit as→0,a2HS,X,t)/aX2
approach, we shall refer to the statistical measure of value-at-risk as &S-VaR' since it is based on a purely statistical model of the DGP, and call the SPD-based measure `E-VaRa since it is based on economic considerations as well. Now if the MRS in Eq. (2.5) were observable, implementing E-VaR measures and comparing them to S-VaR measures would be a simple matter. However, in practice obtaining f H can be quite a challenge, especially for markets that are more complex than the pure-exchange economy described in Section 2.1. Fortunately, several accurate and computationally e$cient estimators of f H have been developed recently and we provide a brief review of these estimators in Section 3.1 and derive their asymptotic distributions in Section A.1 of Appendix A. With these estimators in hand, we show in Section 4 how to gauge the relative importance of E-VaR empirically by examining the ratio f H/f. 3.1. SPDs and option prices Banz and Miller (1978), Breeden and Litzenberger (1978), and Ross (1976) were among the "rst to suggest that Arrow}Debreu prices may be estimated or approximated from the prices of traded "nancial securities. In particular, building on Ross's (1976) insight that options can be used to create pure Arrow}Debreu state-contingent securities, Banz and Miller (1978) and Breeden and Litzenberger (1978) provide an elegant method for obtaining an explicit expression for the SPD from option prices: the SPD is the second derivative (normalized to integrate to unity) of a call option pricing formula with respect to the strike price. To see why, consider the portfolio obtained by selling two call options struck at X and buying one struck at X!e and one at X#e. Consider 1/e2 shares of this portfolio, often called a &butter#y' spread because of the shape of its payo! function t(S T ) which pays nothing outside the interval [X!e, X#e]. Letting e tend to zero, the payo! function of the butter#y tends to a Dirac delta function with mass at X, i.e., in the limit the butter#y becomes an elementary Arrow}Debreu security paying $1 if S T "X and nothing otherwise. The limit of its price as e tends to zero should therefore be equal to ert,qqf H(X). Now denote by H(S t , X, q) the market price of a call option at time t with strike price X, time-to-maturity q, and underlying asset price S t . Then, by construction, the price of the butter#y spread must be 1 e2 [!2H(S t , X, q)#H(S t , X!e, q)#H(S t , X#e, q)] (3.1) which has, as its limit as eP0, R2H(S t , X, q)/RX2. 18 Y. An(t-Sahalia, A.W. Lo / Journal of Econometrics 94 (2000) 9}51
按次数下载不扣除下载券;
注册用户24小时内重复下载只扣除一次;
顺序:VIP每日次数-->可用次数-->下载券;
- 《金融经济学原理》课程教学资源(文献资料)Implied risk aversion and pricing kernel in the FTSE 100 index.pdf
- 中国人民大学出版社:《金融经济学》教材书籍PDF电子版 Financial Economics(著:王江).pdf
- 北京大学:《金融经济学》课程教学资源(教案讲义,共二十五讲,授课教师:徐高).pdf
- 《金融经济学原理》课程教学课件(PPT讲稿)一般均衡(2).pptx
- 《金融经济学原理》课程教学课件(PPT讲稿)CCAPM.pptx
- 《金融经济学原理》课程教学课件(PPT讲稿)均值方差分析.pptx
- 《金融经济学原理》课程教学课件(PPT讲稿)无套利定价蝶式策略.pptx
- 《金融经济学原理》课程教学课件(PPT讲稿)无套利定价之美式期权.pptx
- 《金融经济学原理》课程教学课件(PPT讲稿)无套利定价之欧式期权(1).pptx
- 《金融经济学原理》课程教学课件(PPT讲稿)关于期权,你所需要知道的2.pptx
- 《金融经济学原理》课程教学课件(PPT讲稿)资产定价基本原理(1).pptx
- 《金融经济学原理》课程教学课件(PPT讲稿)Arbitrage Pricing Theory-鞅定价原理.pptx
- 《金融经济学原理》课程教学课件(PPT讲稿)资产定价基本原理.pptx
- 《金融经济学原理》课程教学课件(PPT讲稿)无套利定价原则.pptx
- 《金融经济学原理》课程教学课件(PPT讲稿)完备市场的等价描述.pptx
- 《金融经济学原理》课程教学课件(PPT讲稿)期望效用.pptx
- 石河子大学:《经济地理学》课程教学资源(PPT课件)第四章 中心地理论.ppt
- 石河子大学:《经济地理学》课程教学资源(PPT课件)第十章 经济活动全球化发展过程及影响因素.ppt
- 石河子大学:《经济地理学》课程教学资源(PPT课件)第十二章 经济活动全球化的区域影响.ppt
- 石河子大学:《经济地理学》课程教学资源(PPT课件)第十三章 经济地理研究方法.ppt
- 《金融经济学原理》课程教学资源(文献资料)Option-implied risk aversion estimation.pdf
- 《金融经济学原理》课程教学资源(文献资料)Utility-implied term structures of equity risk premia.pdf
- 《金融经济学原理》课程教学资源(文献资料)When uncertainty and volatility are disconnected - Implications for asset pricing and portfolio performance.pdf
- 《商业银行综合业务处理》课程教学大纲 Business practice of Commercial Bank.doc
- 中南财经政法大学:《金融会计》课程教学课件(PPT讲稿)第一章 金融会计基础理论(1/2).ppt
- 中南财经政法大学:《金融会计》课程教学课件(PPT讲稿)第一章 金融会计基础理论(2/2).ppt
- 中南财经政法大学:《金融会计》课程教学课件(PPT讲稿)第二章 存款业务的会计核算 Deposit accounting.ppt
- 中南财经政法大学:《金融会计》课程教学课件(PPT讲稿)第十八章 所有者权益的核算与管理.ppt
- 中南财经政法大学:《金融会计》课程教学课件(PPT讲稿)第十九章 收入、成本费用及利润的核算与管理.ppt
- 中南财经政法大学:《金融会计》课程教学课件(讲稿)第八章 银行间支付系统的核算与管理.pdf
- 中南财经政法大学:《金融会计》课程教学课件(PPT讲稿)第十五章 财务报告与分析.ppt
- 中南财经政法大学:《金融会计》课程教学课件(讲稿)第六章 外汇业务会计核算.pdf
- 中南财经政法大学:《金融会计》课程教学课件(PPT讲稿)第九章 证券业务会计核算.pptx
- 中南财经政法大学:《金融会计》课程教学课件(讲稿)金融资产的核算.pdf
- 中南财经政法大学:《创业投资》课程教学课件(PPT讲稿)第一章 创业投资概述(主讲:张家峰).ppt
- 中南财经政法大学:《创业投资》课程教学课件(PPT讲稿)第二章 创业投资的运作原理.ppt
- 中南财经政法大学:《创业投资》课程教学课件(PPT讲稿)第三章 创业投资机构及其组建.ppt
- 中南财经政法大学:《创业投资》课程教学课件(PPT讲稿)第五章 创业投资价值评估.ppt
- 中南财经政法大学:《创业投资》课程教学课件(PPT讲稿)第八章 创业投资管理与增值服务.pptx
- 中南财经政法大学:《创业投资》课程教学课件(PPT讲稿)第四章 创业投资项目选择.ppt