《机器学习》课程教学资源(讲稿)对(文本)聚类中一些问题的讨论(Thinking in Clustering)
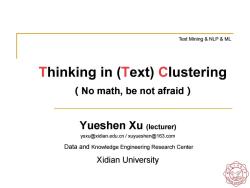
Text Mining NLP ML Thinking in (Text)Clustering No math,be not afraid Yueshen Xu (lecturer) ysxu@xidian.edu.cn/xuyueshen@163.com Data and Knowledge Engineering Research Center Xidian University
Thinking in (Text) Clustering (No math, be not afraid) Yueshen Xu (lecturer) ysxu@xidian.edu.cn / xuyueshen@163.com Data and Knowledge Engineering Research Center Xidian University Text Mining & NLP & ML
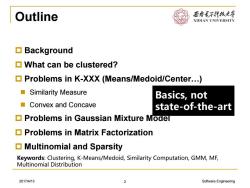
Outline 历些毛子代拔大》 XIDIAN UNIVERSITY ▣Background What can be clustered? Problems in K-XXX(Means/Medoid/Center...) ■Similarity Measure Basics,not ■Convex and Concave state-of-the-art Problems in Gaussian Mixture Model Problems in Matrix Factorization Multinomial and Sparsity Keywords:Clustering,K-Means/Medoid,Similarity Computation,GMM,MF, Multinomial Distribution 2017/4/13 Software Engineering
2017/4/13 Software Engineering Outline Background What can be clustered? Problems in K-XXX (Means/Medoid/Center…) Similarity Measure Convex and Concave Problems in Gaussian Mixture Model Problems in Matrix Factorization Multinomial and Sparsity 2 Keywords: Clustering, K-Means/Medoid, Similarity Computation, GMM, MF, Multinomial Distribution Basics, not state-of-the-art
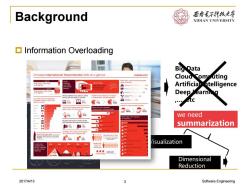
Background 历忠毛子代枚大学 XIDIAN UNIVERSITY Information Overloading Big Data Chinese International Travel Monitor 2015 at a glance Hotels.com Cloud Com uting Artificiatelligence Deep Kearnng n we need 8o0oa summarization isualization 人盘 Dimensional Reduction 2017/4/13 Software Engineering
2017/4/13 Software Engineering Background Information Overloading 3 we need summarization Visualization Dimensional Reduction Big Data Cloud Computing Artificial Intelligence Deep Learning ,…, etc
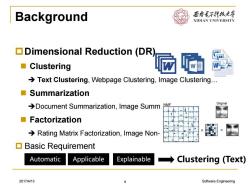
Background 历些毫子种拔大” XIDIAN UNIVERSITY Dimensional Reduction (DR) ■Clustering >Text Clustering,Webpage Clustering,Image Clustering... ■Summarization NMF ●nigina >Document Summarization,Image Summ ■Factorization >Rating Matrix Factorization,Image Non- ▣Basic Requirement Automatic Applicable Explainable →Clustering(Text) 2017/14/13 Software Engineering
2017/4/13 Software Engineering Background Dimensional Reduction (DR) Clustering Text Clustering, Webpage Clustering, Image Clustering… Summarization Document Summarization, Image Summarization… Factorization Rating Matrix Factorization, Image Non-negative Factorization 4 Automatic Applicable Explainable Basic Requirement Clustering (Text)
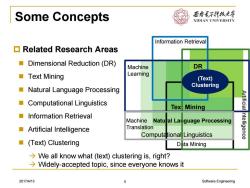
Some Concepts 历些毛子种技大学 XIDIAN UNIVERSITY Information Retrieval Related Research Areas Dimensional Reduction(DR) Machine DR ■Text Mining Learning (Text) Clustering Natural Language Processing Computational Linguistics Tex Mining Artificial Information Retrieval Machine Natu al Language Processing Artificial Intelligence Translation Computational Linguistics ntelligence (Text)Clustering Data Mining >We all know what(text)clustering is,right? >Widely-accepted topic,since everyone knows it 2017/4/13 Software Engineering
2017/4/13 Software Engineering Related Research Areas Dimensional Reduction (DR) Text Mining Natural Language Processing Computational Linguistics Information Retrieval Artificial Intelligence (Text) Clustering Some Concepts 5 Information Retrieval Computational Linguistics Natural Language Processing LSA/Topic Model Text Mining DR Data Mining Artificial Intelligence Machine Learning Machine Translation (Text) Clustering We all know what (text) clustering is, right? Widely-accepted topic, since everyone knows it
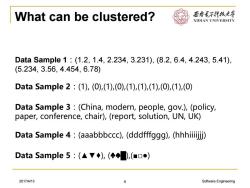
What can be clustered? 历些毛子种枝大” XIDIAN UNIVERSITY Data Sample1:(1.2,1.4,2.234,3.231),(8.2,6.4,4.243,5.41), (5.234,3.56,4.454,6.78) Data Sample2:(1),(0),(1),(0),(1),(1),(1),(0),(1),(0) Data Sample 3:(China,modern,people,gov.),(policy, paper,conference,chair),(report,solution,UN,UK) Data Sample 4:(aaabbbccc),(dddfffggg),(hhhiiiijj) Data Sample5:(Av◆),(,(ao●) 2017/14/13 6 Software Engineering
2017/4/13 Software Engineering What can be clustered? 6 Data Sample 1:(1.2, 1.4, 2.234, 3.231), (8.2, 6.4, 4.243, 5.41), (5.234, 3.56, 4.454, 6.78) Data Sample 2:(1), (0),(1),(0),(1),(1),(1),(0),(1),(0) Data Sample 3:(China, modern, people, gov.), (policy, paper, conference, chair), (report, solution, UN, UK) Data Sample 4:(aaabbbccc), (dddfffggg), (hhhiiiijjj) Data Sample 5:(▲▼♦), (♣♠█),(■□●)
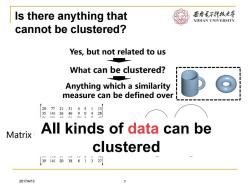
Is there anything that 历粤莞子代找大学 XIDIAN UNIVERSITY cannot be clustered? Yes,but not related to us What can be clustered? Anything which a similarity measure can be defined over 207721 31 451 14126 46 904 28 All kinds of data can be Matrix clustered 3916i2088i;2 2017/4/13
2017/4/13 Software Engineering Is there anything that cannot be clustered? 7 Yes, but not related to us What can be clustered? Anything which a similarity measure can be defined over Matrix topology All kinds of data can be clustered
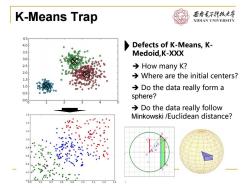
K-Means Trap 历些毛子代枝大等 XIDIAN UNIVERSITY 4.5 4.0 Defects of K-Means,K- 3.5 Medoid,K-XXX 3.0 →How many K? 20 Where are the initial centers? 1.5 >Do the data really form a 0.5 sphere? 0.0 >Do the data really follow Minkowski /Euclidean distance? 12 1.0 0.6
2017/4/13 Software Engineering K-Means Trap 8 Defects of K-Means, KMedoid,K-XXX How many K? Where are the initial centers? Do the data really form a sphere? Do the data really follow Minkowski /Euclidean distance?
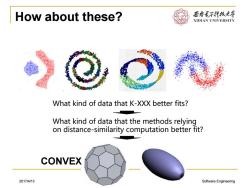
How about these? 历些毛子种枚大学 XIDIAN UNIVERSITY What kind of data that K-XXX better fits? What kind of data that the methods relying on distance-similarity computation better fit? CONVEX 2017/4/13 Software Engineering
2017/4/13 Software Engineering How about these? What kind of data that K-XXX better fits? What kind of data that the methods relying on distance-similarity computation better fit? CONVEX
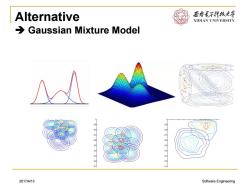
Alternative 历些毛子代枝大等 XIDIAN UNIVERSITY >Gaussian Mixture Model 2017/14/13 Software Engineering
2017/4/13 Software Engineering Alternative Gaussian Mixture Model
按次数下载不扣除下载券;
注册用户24小时内重复下载只扣除一次;
顺序:VIP每日次数-->可用次数-->下载券;
- 《机器学习》课程教学资源(讲稿)基于上下文的服务推荐 Context-Aware Service Recommendation.pdf
- 《机器学习》课程教学资源(讲稿)自然语言理解、主题建模与基于NN的语言生成 Natural Language Processing, Topic Modeling and Neural Text Generation.pdf
- 《机器学习》课程教学资源(讲稿)推荐系统入门——任务、特征与方法概述(Recommender System).pdf
- 《机器学习》课程教学资源(讲稿)The Structure of an Academic Paper in CS(for Starters).pdf
- 《机器学习》课程教学资源(讲稿)Academic Paper Writing for Starters.pdf
- 西安电子科技大学:《信息检索》课程教学资源(讲义)文本挖掘中的概率图模型、矩阵方法与变量求解.pdf
- 西安电子科技大学:《数据通信与计算机网络》课程教学资源(PPT课件)数字数据通信技术——异步与同步传输.pptx
- 西安电子科技大学:《数据通信与计算机网络》课程教学资源(PPT课件)HDLC帧结构——高级数据链路控制协议(帧结构).pptx
- 西安电子科技大学:《并行计算》课程教学资源(课件讲稿)Python并发编程部分(Python并发程序设计).pdf
- 西安电子科技大学:《并行计算》课程教学资源(课件讲稿)Python并发与并行程序设计(语言基础部分).pdf
- 西安电子科技大学:《并行计算》课程教学资源(课件讲稿)Java并发与并行程序设计.pdf
- 西安电子科技大学:《并行计算》课程教学资源(课件讲稿)Java并发程序设计(并行程序设计基础与样例).pdf
- 西安电子科技大学:《并行计算》课程教学资源(课件讲稿)并行程序设计基础与样例(第二部分,交互问题与计算圆周率).pdf
- 西安电子科技大学:《并行计算》课程教学资源(课件讲稿)并行程序设计基础与样例(第一部分).pdf
- 西安电子科技大学:《并行计算》课程教学资源(课件讲稿)并行计算机性能测评.pdf
- 西安电子科技大学:《并行计算》课程教学资源(课件讲稿)并行计算机系统结构(第二部分).pdf
- 西安电子科技大学:《并行计算》课程教学资源(课件讲稿)并行计算机系统结构模型(第一部分,含第一次作业).pdf
- 西安电子科技大学:《并行计算》课程教学资源(课件讲稿)课程概述 Parallel Computing(主讲:徐悦甡).pdf
- 西安电子科技大学:《信息检索》课程教学资源(课件讲稿)推荐系统(Recommender System).pdf
- 西安电子科技大学:《信息检索》课程教学资源(课件讲稿)文本分类(Text Classification).pdf
- 《机器学习》课程教学资源(讲稿)主题模型与层次主题模型(Topic Model and Hierarchical Topic Model).pdf
- 长沙理工大学:《大学计算机基础》课程教学资源(课件讲稿)第1章 计算机系统基础.pdf
- 长沙理工大学:《大学计算机基础》课程教学资源(课件讲稿)第3章 文字处理软件Word 2010.pdf
- 长沙理工大学:《大学计算机基础》课程教学资源(课件讲稿)第2章 操作系统基础.pdf
- 长沙理工大学:《大学计算机基础》课程教学资源(课件讲稿)第4章 电子表格处理软件Excel 2010.pdf
- 长沙理工大学:《大学计算机基础》课程教学资源(课件讲稿)第5章 演示文稿制作软件PowerPoint 2010.pdf
- 长沙理工大学:《大学计算机基础》课程教学资源(课件讲稿)第6章 计算机网络基础.pdf
- 长沙理工大学:《大学计算机基础》课程教学资源(课件讲稿)第7章 多媒体技术基础.pdf
- 长沙理工大学:《大学计算机基础》课程教学资源(课件讲稿)第8章 数据库技术基础.pdf
- 中国人民大学:《移动平台应用开发》课程教学资源(教案讲义)智能移动平台应用开发教学大纲(主讲:杨刚).pdf
- 中国人民大学:《移动平台应用开发》课程教学资源(课件讲义)第10章 Widget组件开发.pdf
- 中国人民大学:《移动平台应用开发》课程教学资源(课件讲义)第9章 位置服务与地图应用.pdf
- 中国人民大学:《移动平台应用开发》课程教学资源(课件讲义)第8章 数据存储与访问.pdf
- 中国人民大学:《移动平台应用开发》课程教学资源(课件讲义)第7章 后台服务.pdf
- 中国人民大学:《移动平台应用开发》课程教学资源(课件讲义)组件通信——消息机制和广播.pdf
- 中国人民大学:《移动平台应用开发》课程教学资源(课件讲义)第5章 Android组件通信.pdf
- 中国人民大学:《移动平台应用开发》课程教学资源(课件讲义)第4章 Android用户界面.pdf
- 中国人民大学:《移动平台应用开发》课程教学资源(课件讲义)Android应用程序生命周期.pdf
- 中国人民大学:《移动平台应用开发》课程教学资源(课件讲义)第3章 Android系统框架.pdf
- 中国人民大学:《移动平台应用开发》课程教学资源(课件讲义)第0章 前言(主讲:杨刚).pdf