电子科技大学:《机器学习 Machine Learning》课程教学资源(课件讲稿)第16讲 生成对抗网络 GAN

电子科技大学研究生《机器学习》精品课程 第16讲生成对抗网络 16 GAN 郝家胜(Jiasheng Hao) Ph.D.,Associate Professor Email:hao@uestc.edu.cn School of Automation Engineering University of Electronic Science and Technology of China,Chengdu 611731 Aug.2015第一稿;Apr2021第四稿
电子科技大学研究生《机器学习》精品课程 Email: hao@uestc.edu.cn School of Automation Engineering University of Electronic Science and Technology of China, Chengdu 611731 郝家胜 (Jiasheng Hao) Ph.D., Associate Professor Aug. 2015 第一稿;Apr. 2021 第四稿 第16讲 生成对抗网络 16 GAN
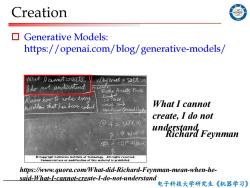
Creation /956 Generative Models: https://openai.com/blog/generative-models/ √hy ernat soi.Po Bathe Amar本fd K人力 3-0 Hale c以,70mh What I cannot Non Lonkan sl且d create,I do not 9()u) understand Richard Feynman t=arallua Copyright California Institute of Technology.All rights reserved. Commercial use or modification of this material is prohibited. https://www.quora.com/What-did-Richard-Feynman-mean-when-he- said-What-I-cannot-create-I-do-not-understand 电子科技大学研究生《机器学习》
电子科技大学研究生《机器学习》 Creation o Generative Models: https://openai.com/blog/generative-models/ https://www.quora.com/What-did-Richard-Feynman-mean-when-he- said-What-I-cannot-create-I-do-not-understand What I cannot create, I do not understand. Richard Feynman
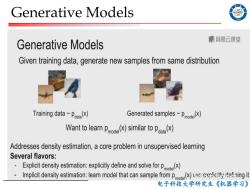
Generative models 号5不 夏网易云课堂 Generative models Given training data,generate new samples from same distribution Training dataP) Generated samples~Pd() Wanttoea Pde)similar to Pax) Addresses density estimation,a core problem in unsupervised learning Several flavors: Explicit densitytimation:expictly define an or P) Implicit density stimation:l mode that can sample from Ping it 电子科技大学研究生《机器学习》
电子科技大学研究生《机器学习》 Generative Models
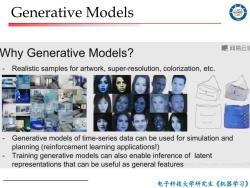
Generative models 号5 Why Generative Models? 网易云识 Realistic samples for artwork,super-resolution,colorization,etc. Generative models of time-series data can be used for simulation and planning(reinforcement learning applications!) Training generative models can also enable inference of latent representations that can be useful as general features ttps://blog.csdn.net/poulang578 电子科技大学研究生《机器学习》
电子科技大学研究生《机器学习》 Generative Models
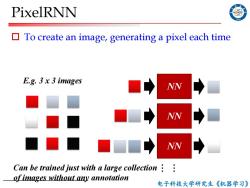
PixelRNN To create an image,generating a pixel each time E.g.3 x 3 images NN NN NN Can be trained just with a large collection: of images without any annotation 电子科技大学研究生《机器学习》
电子科技大学研究生《机器学习》 PixelRNN o To create an image, generating a pixel each time E.g. 3 x 3 images NN NN NN … … Can be trained just with a large collection of images without any annotation
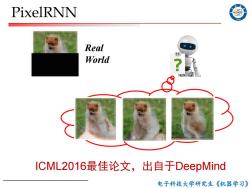
PixelRNN 956 Real World ICML2016最佳论文,出自于DeepMind 电子科技大学研究生《机器学习》
电子科技大学研究生《机器学习》 PixelRNN Real World ICML2016最佳论文,出自于DeepMind
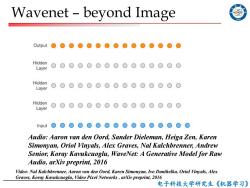
Wavenet beyond Image 56 Output Hidden Layer Hidden Layer Hidden Layer Audio:Aaron van den Oord,Sander Dieleman,Heiga Zen,Karen Simonyan,Oriol Vinyals,Alex Graves,Nal Kalchbrenner,Andrew Senior,Koray Kavukcuoglu,WaveNet:A Generative Model for Raw Audio,arXiv preprint,2016 Video:Nal Kalchbrenner,Aaron van den Oord,Karen Simonyan,Ivo Danihelka,Oriol Vinyals,Alex Graves,Koray Kavukcuoglu,Video Pixel Networks,arXiv preprint,2016 电子科技大学研究生《机器学习》
电子科技大学研究生《机器学习》 Wavenet – beyond Image Audio: Aaron van den Oord, Sander Dieleman, Heiga Zen, Karen Simonyan, Oriol Vinyals, Alex Graves, Nal Kalchbrenner, Andrew Senior, Koray Kavukcuoglu, WaveNet: A Generative Model for Raw Audio, arXiv preprint, 2016 Video: Nal Kalchbrenner, Aaron van den Oord, Karen Simonyan, Ivo Danihelka, Oriol Vinyals, Alex Graves, Koray Kavukcuoglu, Video Pixel Networks , arXiv preprint, 2016

例 Any other ideas? 电子科技大学研究生《模式识别》
电子科技大学研究生《模式识别》 Any other ideas?

PixelCNN Still generate image pixels starting from Softmax loss at each pixel corner Dependency on previous pixels now modeled using a CNN over context region Training:maximize likelihood of training images n p(x)=p(xc1,,ci-1) i=1 电子科技大学研究生《机器学习》
电子科技大学研究生《机器学习》 PixelCNN
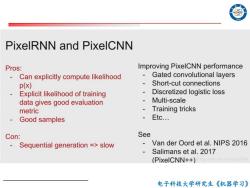
PixelRNN and PixelCNN Pros: Improving PixelCNN performance Can explicitly compute likelihood - Gated convolutional layers p(x) Short-cut connections Explicit likelihood of training Discretized logistic loss data gives good evaluation Multi-scale metric Training tricks Good samples Etc.… Con: See Sequential generation =slow Van der Oord et al.NIPS 2016 Salimans et al.2017 (PixelCNN++) log.csdn.net/poulang5786 电子科技大学研究生《机器学习》
电子科技大学研究生《机器学习》
按次数下载不扣除下载券;
注册用户24小时内重复下载只扣除一次;
顺序:VIP每日次数-->可用次数-->下载券;
- 电子科技大学:《机器学习 Machine Learning》课程教学资源(课件讲稿)第14讲 深度CNN Deep CNN.pdf
- 电子科技大学:《机器学习 Machine Learning》课程教学资源(课件讲稿)第13讲 卷积神经网络 Convolution Neural Nets.pdf
- 电子科技大学:《机器学习 Machine Learning》课程教学资源(课件讲稿)第12讲 特征学习 Feature Learning.pdf
- 电子科技大学:《机器学习 Machine Learning》课程教学资源(课件讲稿)第11讲 特征提取 Feature Extraction.pdf
- 电子科技大学:《机器学习 Machine Learning》课程教学资源(课件讲稿)第10讲 特征提取 Feature Extraction.pdf
- 电子科技大学:《机器学习 Machine Learning》课程教学资源(课件讲稿)第9讲 特征选择 Feature Selection.pdf
- 电子科技大学:《机器学习 Machine Learning》课程教学资源(课件讲稿)第8讲 非监督学习 Unsupervised Learning.pdf
- 电子科技大学:《机器学习 Machine Learning》课程教学资源(课件讲稿)第7讲 其他分类方法 Classifiers for More.pdf
- 电子科技大学:《机器学习 Machine Learning》课程教学资源(课件讲稿)第6讲 近邻法与Logist回归 Nearest Neighbors & Logist Regression.pdf
- 电子科技大学:《机器学习 Machine Learning》课程教学资源(课件讲稿)第5讲 人工神经网络分类器 Classifiers with ANN.pdf
- 电子科技大学:《机器学习 Machine Learning》课程教学资源(课件讲稿)第4讲 支持向量机 Support Vector Machines.pdf
- 电子科技大学:《机器学习 Machine Learning》课程教学资源(课件讲稿)第3讲 线性模型 Linear Models.pdf
- 电子科技大学:《机器学习 Machine Learning》课程教学资源(课件讲稿)第2讲 模型评估与选择 Evaluation and Selection of Models.pdf
- 电子科技大学:《机器学习 Machine Learning》课程教学资源(课件讲稿)第1讲 概论 Introduction(主讲:郝家胜).pdf
- 《机器学习 Machine Learning》课程教学资源(书籍文献)SVM Tutorial.pdf
- 《机器学习 Machine Learning》课程教学资源(书籍文献)A random forest guided tour.pdf
- 《机器学习 Machine Learning》课程教学资源(书籍文献)Real-Time Human Pose Recognition in Parts from Single Depth Images.pdf
- 《机器学习 Machine Learning》课程教学资源(书籍文献)An introduction to neural networks.pdf
- 《机器学习 Machine Learning》课程教学资源(书籍文献)An introduction to neural networks for beginners.pdf
- 《机器学习 Machine Learning》课程教学资源(书籍文献)Handwritten Digit Recognition with a Back-Propagation Network.pdf
- 电子科技大学:《机器学习 Machine Learning》课程教学资源(课件讲稿)第17讲 循环神经网络 Recurrent Neural Networks.pdf
- 电子科技大学:《机器学习 Machine Learning》课程教学资源(课件讲稿)第18讲 强化学习 Reinforcement Learning.pdf
- 电子科技大学:《机器学习 Machine Learning》课程教学资源(课件讲稿)第12讲 超参数优化与自动学习 Hyperparameters Optimization & AutoML.pdf
- 《C++程序设计》课程教学资源(课件讲稿)第三篇 基于对象的程序设计 第9章 关于类和对象的进一步讨论.pdf
- 杭州电子科技大学:《计算机视觉》课程教学资源(PPT课件讲稿)第五讲 目标分割.pdf
- 杭州电子科技大学:《人工智能导论》课程教学资源(PPT课件讲稿)第六讲 机器学习基础(机器学习与知识发现).pdf
- 杭州电子科技大学:《人工智能导论》课程教学资源(PPT课件讲稿)第三讲 搜索与求解.pdf
- 杭州电子科技大学:《人工智能导论》课程教学资源(PPT课件讲稿)第二讲 人工智能概述.pdf
- 杭州电子科技大学:《人工智能与模式识别》课程教学资源(讲稿)第二讲 基础知识(概念).pdf
- 杭州电子科技大学:《人工智能与模式识别》课程教学资源(讲稿)第一讲 绪论(主讲:周文晖).pdf
- 杭州电子科技大学:《人工智能导论》课程教学资源(PPT课件讲稿)第一讲 绪论(主讲:周文晖).pdf
- Deep Learning-Based CT Radiomics for Feature Representation and Analysis of Aging Characteristics of Asian Bony Orbit.pdf
- 杭州电子科技大学:《计算机视觉》课程教学资源(PPT课件讲稿)第一讲 绪论(主讲:周文晖).pdf
- 杭州电子科技大学:《计算机视觉》课程教学资源(PPT课件讲稿)第三讲 图像预处理.pdf
- 杭州电子科技大学:《计算机视觉》课程教学资源(PPT课件讲稿)第二讲 图像采集.pdf
- 杭州电子科技大学:《计算机视觉》课程教学资源(PPT课件讲稿)第四讲 基元检测.pdf
- 杭州电子科技大学:《计算机视觉》课程教学资源(PPT课件讲稿)第六讲 立体视觉.pdf
- 杭州电子科技大学:《人工智能导论》课程教学资源(PPT课件讲稿)第四讲 遗传算法.pdf
- 杭州电子科技大学:《人工智能导论》课程教学资源(PPT课件讲稿)第五讲 不确定性知识的表示与推理.pdf
- 杭州电子科技大学:《人工智能导论》课程教学资源(PPT课件讲稿)第七讲 决策树学习.pdf