上海交通大学:《数字信号处理 Digital Signal Processing(B)》教学资源_Reference Book_DSP of MIT_lec20
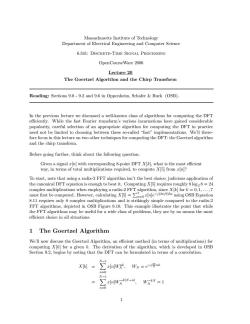
Massachusetts Institute of Technology Department of Electrical Engineering and Computer Science 6.341:DISCRETE-TIME SIGNAL PROCESSING OpenCourseWare 2006 Lecture 20 The Goertzel Algorithm and the Chirp Transform Reading:Sections 9.0-9.2 and 9.6 in Oppenheim,Schafer Buck (OSB). In the previous lecture we discussed a well-known class of algorithms for computing the DFT efficiently.While the fast Fourier transform's various incarnations have gained considerable popularity,careful selection of an appropriate algorithm for computing the DFT in practice need not be limited to choosing between these so-called "fast"implementations.We'll there- fore focus in this lecture on two other techniques for computing the DFT:the Goertzel algorithm and the chirp transform. Before going further,think about the following question: Given a signal x[n]with corresponding 8-point DFT X[k],what is the most efficient way,in terms of total multiplications required,to compute X[5]from xn]? To start,note that using a radix-2 FFT algorithm isn't the best choice;judicious application of the canonical DFT equation is enough to beat it.Computing X[5]requires roughly 810g28=24 complex multiplications when employing a radix-2 FFT algorithm,since Xk fork =0,1,...,7 must first be computed.However,calculating]=e/)5n using OSB Equation 8.11 requires only 8 complex multiplications and is strikingly simple compared to the radix-2 FFT algorithms,depicted in OSB Figure 9.10.This example illustrates the point that while the FFT algorithms may be useful for a wide class of problems,they are by no means the most efficient choice in all situations. 1 The Goertzel Algorithm We'll now discuss the Goertzel Algorithm,an efficient method(in terms of multiplications)for computing Xk for a given k.The derivation of the algorithm,which is developed in OSB Section 9.2,begins by noting that the DFT can be formulated in terms of a convolution. N-1 X[附= xmw, WN=ej装nk n=0 N-1 ∑W-,ww=1
1 Massachusetts Institute of Technology Department of Electrical Engineering and Computer Science 6.341: Discrete-Time Signal Processing OpenCourseWare 2006 Lecture 20 The Goertzel Algorithm and the Chirp Transform Reading: Sections 9.0 - 9.2 and 9.6 in Oppenheim, Schafer & Buck (OSB). In the previous lecture we discussed a well-known class of algorithms for computing the DFT efficiently. While the fast Fourier transform’s various incarnations have gained considerable popularity, careful selection of an appropriate algorithm for computing the DFT in practice need not be limited to choosing between these so-called “fast” implementations. We’ll therefore focus in this lecture on two other techniques for computing the DFT: the Goertzel algorithm and the chirp transform. Before going further, think about the following question: Given a signal x[n] with corresponding 8-point DFT X[k], what is the most efficient way, in terms of total multiplications required, to compute X[5] from x[n]? To start, note that using a radix-2 FFT algorithm isn’t the best choice; judicious application of the canonical DFT equation is enough to beat it. Computing X[5] requires roughly 8 log 2 8 = 24 complex multiplications when employing a radix-2 FFT algorithm, since X[k] for k = 0, 1, . . . , 7 must first be computed. However, calculating X[5] = 7 −j(2�/8)5n using OSB Equation n=0 x[n]e 8.11 requires only 8 complex multiplications and is strikingly simple compared to the radix-2 FFT algorithms, depicted in OSB Figure 9.10. This example illustrates the point that while the FFT algorithms may be useful for a wide class of problems, they are by no means the most efficient choice in all situations. The Goertzel Algorithm We’ll now discuss the Goertzel Algorithm, an efficient method (in terms of multiplications) for computing X[k] for a given k. The derivation of the algorithm, which is developed in OSB Section 9.2, begins by noting that the DFT can be formulated in terms of a convolution. N−1 X[k] = � x[n]Wnk N , WN = e−j 2� N nk n=0 N−1 = � x[n]W−k(N−n) N , W−kN N = 1 n=0 1
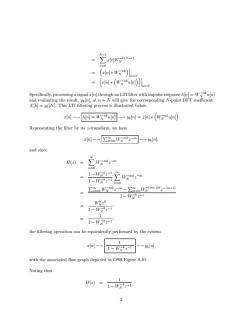
中wn r=0 (回*w儿n=N [-n](Wkin] Specifically,processing a signal n]through an LTI filter with impulse responsehn]=Wun] and evaluating the result,y[n],at n=N will give the corresponding N-point DFT coefficient Xk]=y[N].This LTI filtering process is illustrated below. xm一 hin]WNmkuln] m=刊*(Wku) Representing the filter by its z-transform,we have x[n→ ∑=o WNmkz-m →y[n], and since 00 H(a)=∑Wkzm m=0 1-WNkz-1 1-Wwz之乙 ∑Wmkz-m m=0 ∑=W*2"-∑m=oWm+gm+1 1-Ww2-1 WRz° 1-W*z-1 1 1-W2-1 the filtering operation can be equivalently performed by the system 1 xn] 1- WNE2-I yxn, with the associated flow graph depicted in OSB Figure 9.10. Noting that H(z)= 1-W-1 2
� � �� � � � �� � N−1 = x[r]W−k(N−r) N r=0 = x[n] � W−nk � N � n=N � � ��� = x[n] � W−nk N u[n] � n=N Specifically, processing a signal x[n] through an LTI filter with impulse response h[n] = W −nku[n] N and evaluating the result, yk[n], at n = N will give the corresponding N-point DFT coefficient X[k] = yk[N]. This LTI filtering process is illustrated below. x[n] −� h[n] = W−nku[n] −� yk[n] = x[n] � W−nku[n] N N Representing the filter by its z-transform, we have x[n] −� � W−mkz m=0 −m −� yk[n], N and since H(z) = W−mkz−m N m=0 1 − W−kz−1 � = N W−mk −m N z −1 1 − W−kz N m=0 � W−mk −m − � W−(m+1)k −(m+1) m=0 N z m=0 N z = −1 1 − W−kz N W0 0 N z = 1 − W−kz−1 N 1 = 1 − W−kz−1 , N the filtering operation can be equivalently performed by the system 1 x[n] −� 1 − W−kz −� yk[n], −1 N with the associated flow graph depicted in OSB Figure 9.10. Noting that 1 H(z) = 1 − W−kz−1 N 2
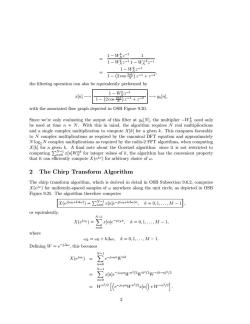
1-W吹21 1 1-W211-w*2-1 1-W啖z-1 1-(2c0s)21+2-21 the filtering operation can also be equivalently performed by xn] 1-W脓2-1 (2c0s)2+22 yhin], 1- with the associated flow graph depicted in OSB Figure 9.20. Since we're only evaluating the output of this filter at y[N],the multiplier-W need only be used at time nN.With this in mind,the algorithm requires N real multiplications and a single complex multiplication to compute Xk for a given k.This compares favorably to N complex multiplications as required by the canonical DFT equation and approximately Nlog2 N complex multiplications as required by the radix-2 FFT algorithms,when computing Xk]for a given k.A final note about the Goertzel algorithm:since it is not restricted to computingfor integer values of the algorithm has the convenient property that it can efficiently compute X(e)for arbitrary choice of w. 2 The Chirp Transform Algorithm The chirp transform algorithm,which is derived in detail in OSB Subsection 9.6.2,computes X(ej)for uniformly-spaced samples of w anywhere along the unit circle,as depicted in OSB Figure 9.25.The algorithm therefore computes X(eeo+kaa)=∑wo rinle--ao+kam,k=0,1,.,M-1 or equivalently, N-1 X(e)=∑xme-u",k=0,1,,M-1, n=0 where wk=w0+k△w,k=0,1,,M-1. Defining W=e-i4,this becomes N-1 X()= e-jwonwnk n=0 N-1 nle-j-onwn2wkp2w-(k-n)2 wmp[(e-owr2pz)*W-n2冈 3
= � � , � � � �� � � 2 −1 1 − Wk N z 1 = N z−1 1 − W−k 1 − Wk z−1 N −1 1 − Wk N z −2 1 − 2 cos 2�k z−1 + z N the filtering operation can also be equivalently performed by −1 1 − Wk x[n] −� 1 − � 2 cos 2�k N � z z− −� yk[n], 1 + −2 z N with the associated flow graph depicted in OSB Figure 9.20. Since we’re only evaluating the output of this filter at yk[N], the multiplier −W k N need only be used at time n = N. With this in mind, the algorithm requires N real multiplications and a single complex multiplication to compute X[k] for a given k. This compares favorably to N complex multiplications as required by the canonical DFT equation and approximately N log2 N complex multiplications as required by the radix-2 FFT algorithms, when computing X[k] for a given k. A final note about the Goertzel algorithm: since it is not restricted to computing N−1 x[n]Wnk for integer values of k, the algorithm has the convenient property n=0 N that it can efficiently compute X(ej�) for arbitrary choice of �. The Chirp Transform Algorithm The chirp transform algorithm, which is derived in detail in OSB Subsection 9.6.2, computes X(ej�) for uniformly-spaced samples of � anywhere along the unit circle, as depicted in OSB Figure 9.25. The algorithm therefore computes N−1 x[n]e X −j(�0+k��)n (ej(�0+k��) ) = , k = 0, 1, . . . , M − 1 , n=0 or equivalently, N−1 X(ej�k ) = x[n]e−j�kn, k = 0, 1, . . . , M − 1, n=0 where �k = �0 + k��, k = 0, 1, . . . , M − 1. Defining W = e−j��, this becomes N−1 j�k X(e ) = e−j�0nWnk n=0 N−1 = x[n]e−j�0nWn2/2Wk2/2W−(k−n)2/2 n=0 −j�0nWn2/2x[n] � W−n2/2 = Wn2/2 e . 3

By defininggn]=eoWnn],the above equation is equivalently represented as X(e)=Wmp(glm*W-n2), which leads to the block diagram depicted in OSB Figure 9.26.This system isn't realizable as-is,since it is neither causal nor stable.If,however,we restrict ourselves to operating on a finite-length causal signal x[n],the system can be implemented,since we're also only interested its output yn]over a given,finite time interval.There are several possible causal realizations of this,and in each case the implementation relies on appropriate bookkeeping. There may also be some question as to how implementing X(e)as in OSB Figure 9.26 could be practically useful.Note however that for a given Aw,the analysis parameter wo appears only at the first modulator block.As long as the frequency sample spacing Aw is fixed,the system can therefore be easily adjusted to compute for frequency samples beginning at any point on the unit circle.Another advantage of this technique is that it computes DTFT samples using a fixed convolution block,a property which is convenient when using discrete-time analog hard- ware,such as charge-coupled devices (CCDs). 4
� � By defining g[n] = e−j�0nWn2/2x[n], the above equation is equivalently represented as 2/2 g[n] � W−n2/2 X(ej�k ) = Wn , which leads to the block diagram depicted in OSB Figure 9.26. This system isn’t realizable as-is, since it is neither causal nor stable. If, however, we restrict ourselves to operating on a finite-length causal signal x[n], the system can be implemented, since we’re also only interested its output y[n] over a given, finite time interval. There are several possible causal realizations of this, and in each case the implementation relies on appropriate bookkeeping. There may also be some question as to how implementing X(ej�k ) as in OSB Figure 9.26 could be practically useful. Note however that for a given ��, the analysis parameter �0 appears only at the first modulator block. As long as the frequency sample spacing �� is fixed, the system can therefore be easily adjusted to compute for frequency samples beginning at any point on the unit circle. Another advantage of this technique is that it computes DTFT samples using a fixed convolution block, a property which is convenient when using discrete-time analog hardware, such as charge-coupled devices (CCDs). 4
按次数下载不扣除下载券;
注册用户24小时内重复下载只扣除一次;
顺序:VIP每日次数-->可用次数-->下载券;
- 上海交通大学:《数字信号处理 Digital Signal Processing(B)》教学资源_Reference Book_DSP of MIT_lec19.pdf
- 上海交通大学:《数字信号处理 Digital Signal Processing(B)》教学资源_Reference Book_DSP of MIT_lec18.pdf
- 上海交通大学:《数字信号处理 Digital Signal Processing(B)》教学资源_Reference Book_DSP of MIT_lec17.pdf
- 上海交通大学:《数字信号处理 Digital Signal Processing(B)》教学资源_Reference Book_DSP of MIT_lec16.pdf
- 上海交通大学:《数字信号处理 Digital Signal Processing(B)》教学资源_Reference Book_DSP of MIT_lec15.pdf
- 上海交通大学:《数字信号处理 Digital Signal Processing(B)》教学资源_Reference Book_DSP of MIT_lec09.pdf
- 上海交通大学:《数字信号处理 Digital Signal Processing(B)》教学资源_Reference Book_DSP of MIT_lec08.pdf
- 上海交通大学:《数字信号处理 Digital Signal Processing(B)》教学资源_Reference Book_DSP of MIT_lec06.pdf
- 上海交通大学:《数字信号处理 Digital Signal Processing(B)》教学资源_Reference Book_DSP of MIT_lec05.pdf
- 上海交通大学:《数字信号处理 Digital Signal Processing(B)》教学资源_Reference Book_DSP of MIT_lec04.pdf
- 上海交通大学:《数字信号处理 Digital Signal Processing(B)》教学资源_Reference Book_DSP of MIT_lec03.pdf
- 上海交通大学:《数字信号处理 Digital Signal Processing(B)》教学资源_Reference Book_DSP of MIT_lec02.pdf
- 上海交通大学:《数字信号处理 Digital Signal Processing(B)》教学资源_Handouts_dsp3-9章中文版.pdf
- 上海交通大学:《数字信号处理 Digital Signal Processing(B)》教学资源_Handouts_ch9 FFT.pdf
- 上海交通大学:《数字信号处理 Digital Signal Processing(B)》教学资源_Handouts_ch9 DFT.pdf
- 上海交通大学:《数字信号处理 Digital Signal Processing(B)》教学资源_Handouts_ch7_Digital Filter Realization.pdf
- 上海交通大学:《数字信号处理 Digital Signal Processing(B)》教学资源_Handouts_ch6_Transfer Functions.pdf
- 上海交通大学:《数字信号处理 Digital Signal Processing(B)》教学资源_Handouts_ch4_FIR filtering and convolution.pdf
- 上海交通大学:《数字信号处理 Digital Signal Processing(B)》教学资源_Handouts_ch3_Discrete-Time Signals and Systems.pdf
- 上海交通大学:《数字信号处理 Digital Signal Processing(B)》教学资源_Handouts_ch1_Review for Signal and System.pdf
- 上海交通大学:《数字信号处理 Digital Signal Processing(B)》教学资源_Reference Book_DSP of MIT_lec21.pdf
- 上海交通大学:《数字信号处理 Digital Signal Processing(B)》教学资源_Reference Book_DSP of MIT_lec22.pdf
- 上海交通大学:《数字信号处理 Digital Signal Processing(B)》教学资源_Reference Book_Introduction to Signal Processing.pdf
- 《数字信号处理 Digital Signal Processing(B)》教学资源(参考资料)Digital Signal Processing Principles, Algorithms, and Applications Third Edition(John G. Proakis、Dimitris G. Manolakis).pdf
- 上海交通大学:《数字信号处理 Digital Signal Processing(B)》教学资源_Reference Book_数字信号处理及其matlab实现.pdf
- 上海交通大学:《工程实践与科技创新》课程教学资源_Altium Designer 电路板设计.ppt
- 上海交通大学:《工程实践与科技创新》课程教学资源_历年优秀作业.pdf
- 上海交通大学:《工程实践与科技创新》课程教学资源_智能小车_焊接.pdf
- 上海交通大学:《工程实践与科技创新》课程教学资源_电子元件介绍.ppt
- 上海交通大学:《工程实践与科技创新》课程教学资源_电源板元件清单.doc
- 上海交通大学:《工程实践与科技创新》课程教学资源_自动控制小车电路原理与演示程序说明.pdf
- 上海交通大学:《工程实践与科技创新》课程教学资源_软件编译与下载(工创1:自动控制小车).doc
- 上海交通大学:《工程实践与科技创新》课程教学资源_自动控制小车焊接.pdf
- 上海交通大学:《工程实践与科技创新》课程教学资源_自动控制小车电路原理与演示程序说明.pdf
- 上海交通大学:《电路与电子技术》课程教学资源(课件讲稿)多级放大电路.ppt
- 上海交通大学:《电路与电子技术》课程教学资源(课件讲稿)常用半导体器件.pdf
- 上海交通大学:《电路与电子技术》课程教学资源(课件讲稿)数字电子技术——门电路和组合逻辑电路.pdf
- 上海交通大学:《电路与电子技术》课程教学资源(课件讲稿)数字电子技术——触发器和时序逻辑电路.pdf
- 上海交通大学:《电路基础》课程教学资源(PPT课件)第一章 基本概念和基本规律 §1.3.1 基尔霍夫定律 §1.3.2 基尔霍夫定律 §1.3.3 从网络到图 §1.3.4 KCL、KVL的矩阵形式 §1.3.5 特勒根定理.ppt
- 上海交通大学:《电路基础》课程教学资源(PPT课件)第一章 基本概念和基本规律 §1.4.1 电阻元件及其约束方程 §1.4.1 功率和能量.ppt